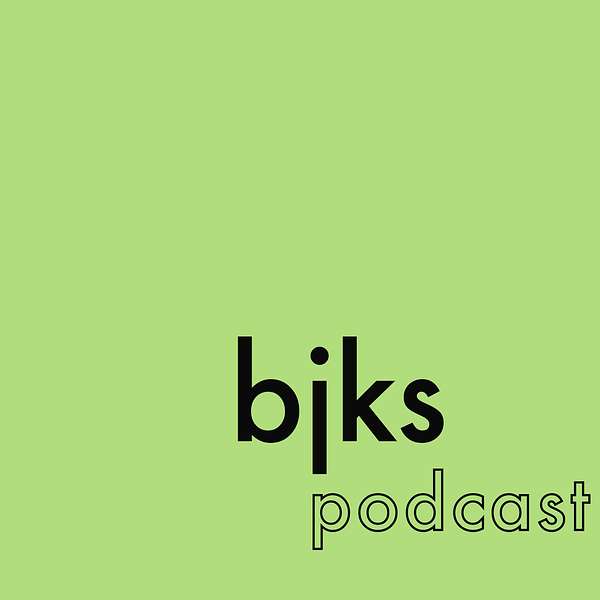
BJKS Podcast
A podcast about neuroscience, psychology, and anything vaguely related. Long-form interviews with people whose work I find interesting.
BJKS Podcast
111. Renzo Huber: Layer-fMRI, high-resolution fMRI, and the delicate balance between gourmet chef and janitor
Renzo Huber is a staff scientist at NIH. We talk about his work on layer-fMRI: what it is, how Renzo got into it, how to do it, when it makes sense to do it, what the future holds, and much more.
Support the show: https://geni.us/bjks-patreon
Timestamps
0:00:00: How Renzo got into high-resolution fMRI
0:11:28: The difference between 3T and 7T fMRI
0:22:46: Is a bigger fMRI scanner always better?
0:33:35: Layer-fMRI
0:56:28: For what types of research is layer-fMRI most useful?
1:02:35: How to do layer-fMRI and make it reproducible
1:19:21: The future of layer-fMRI
1:27:02: A book or paper more people should read
1:30:37: Something Renzo wishes he'd learnt sooner
1:33:11: Advice for PhD students/postdocs
Podcast links
- Website: https://geni.us/bjks-pod
- Twitter: https://geni.us/bjks-pod-twt
Renzo's links
- Website: https://geni.us/huber-web
- Google Scholar: https://geni.us/huber-scholar
- Twitter: https://geni.us/huber-twt
Ben's links
- Website: https://geni.us/bjks-web
- Google Scholar: https://geni.us/bjks-scholar
- Twitter: https://geni.us/bjks-twt
References and links mentioned
Episode with Peter Bandettini: https://geni.us/bjks-bandettini
Episode with Emily Finn: https://geni.us/bjks-finn
Renzo's blog about layer fMRI: https://layerfmri.com/
YouTube channel on layer fMRI: https://www.youtube.com/@layerfmri/
Bastos, ... & Friston (2012). Canonical microcircuits for predictive coding. Neuron.
Bollmann & Barth (2021). New acquisition techniques and their prospects for the achievable resolution of fMRI. Progress in Neurobiology.
Boulant, ... & Le Bihan (2024). In vivo imaging of the human brain with the Iseult 11.7-T MRI scanner. Nature Methods.
Finn, ... & Bandettini (2019). Layer-dependent activity in human prefrontal cortex during working memory. Nature Neuroscience.
Feynman (1985). "Surely you're joking, Mr. Feynman!": adventures of a curious character.
Haarsma, Kok & Browning (2022). The promise of layer-specific neuroimaging for testing predictive coding theories of psychosis. Schizophrenia Research.
Huber, ... & Bandettini (2017). High-resolution CBV-fMRI allows mapping of laminar activity and connectivity of cortical input and output in human M1. Neuron.
Huber, ... & Möller (2019). Non-BOLD contrast for laminar fMRI in humans: CBF, CBV, and CMRO2. Neuroimage.
Huber, ... & Bandettini (2020). Sub-millimeter fMRI reveals multiple topographical digit representations that form action maps in human motor cortex. Neuroimage.
Huber, ... & Kronbichler (2023). Evaluating the capabilities and challenges of layer-fMRI VASO at 3T. Aperture Neuro.
Huber, ... & Horovitz (2023). Laminar VASO fMRI in focal hand dystonia patients. Dystonia.
Persichetti, ... & Martin (2020). Layer-specific contributions to imagined and executed hand movements in human primary motor cortex. Current Biology.
Polimeni, ... & Wald (2010). Laminar analysis of 7 T BOLD using an imposed spatial activation pattern in human V1. Neuroimage.
[This is an automated transcript that contains many errors]
Benjamin James Kuper-Smith: Yeah, I mean, I thought I wanted to start this one fairly in a fairly traditional way. You know, always interested in like how, number one, like just how people got to do what they do. And also I think this can often be a nice way to kind of introduce topics and what makes them interesting and appealing.
And so maybe kind of quite classically you studied physics. I mean, first of all, you're, I believe from Austria and then you studied physics in Germany. So that, those are two choices. Why make both of them in that particular way?
Renzo Huber: Why Germany and why physics? Well, Austria and Germany are kind of a very similar culture. If there wouldn't have been this thing like the Second World War, maybe there would be one country or not. So, I guess in I originally kind of was born in Salzburg. I actually went to school in, in, in Germany.
And then, so, kind of, I don't really see it as two completely different things. When there's a football match of Germany versus Austria, I would root for Germany for that matter. Why physics? I guess it was just something that [00:01:00] I was kind of used to and good at in school. Originally, I come from a background of artists.
Both my parents are artists, and I was kind of in a school that was kind of focusing on art and theater and those kind of things. And so I was kind of the black sheep in, in working in physics. I liked physics simply because it's, you can kind of think very stubbornly in your strict rules And that was something I was kind of good at.
And so I went into physics, even though I was really interested in the brain. So before I went to physics, I even tried to understand how the mind works, how a consciousness works and then how a thought is done. And I had a lot of fun doing physics in Munich. In Germany, specifically, that was an exciting time with the launch Hadron Collider.
So I did a bachelor thesis looking into gas mixtures at the Atlas detector in the ion chambers for the large Hadron Collider at CERN. Which was kind of fun at the time. However, it. It is not really affecting your everyday life. And so I tried to go back into the brain a bit, which was a hard task.
[00:02:00] Like in my master's project, I wanted to do something about intelligence or thoughts or consciousness, which is a difficult endeavor in the sense that there are people who combine physics and the brain or the mind, but It's kind of a tricky territory. Like you can only look into that domain of combining physics at the brain.
If you have a career, that's kind of too big to fail for that matter. Penrose does something like that, or your previous advisor Friston or something, they kind of combine physics with thermodynamics or there are people like looking at quantum. Consciousness and those kind of things where it's very tricky that you end up in the kind of woody section of the bookstore and then not being cited by the wrong people.
So I couldn't find any advice that it would want to work with me on this kind of esoteric weird thing. That's kind of not even kind of true strict science like physics is where there's a kind of ground truth at the end. I found a supervisor that was interested, or at least allowed me to work on artificial neural networks.
That was back in 2010, before kind [00:03:00] of the boom of AI to simulate a robot that has curiosity. It wasn't really his area of expertise. He just allowed me to work on it. And I'm glad I didn't follow through and did my master's with him and kept Googling. And on Google, I typed in neurophysics and Well, I, it turns out that this is actually a thing and there was this Max Planck Institute in Leipzig, far away from Munich, where I lived, where they had a cognitive neuroscience department.
And there was a neurophysics department headed by Bob Turner. So I applied there to do a master project. And then I just went into it. I hadn't, I didn't know that kind of MRI is something where you need physicists or what MRI is, or that there's Something like fMRI, I just try to be useful by being surrounded by people who work on the brain.
And then the rest is kind of just happened from that. I guess I was at I was lucky there was a lot of serendipity that I was at the right place at the right time, because in those days, 2012, they had a seven [00:04:00] Tesla scanner, which is one of those very strong magnets to do kind of high resolution MRI this is much stronger than the conventional, you know, like Kind of three Tesla or clinical 1.
5 Tesla scanners. And in those days, there were already a handful of seven Tesla scanners around the world. So it was a kind of a rather established method already from the engineering perspective. It was not a playground anymore for the engineers to just see if you can build this machine, but it was already starting to get useful in the sense that you could sit there and get an image kind of on a key turn button press basis.
Still it was not really established enough that it was useful for kind of cognitive neuroscience for that matter. So nowadays when you buy a seven tesla scanners when a hospital buys one or Fancy prestigious university buys a seven tesla scanner they rather treat it like a money making machine Like if you want to use it You need to dedicate a lot of your grant money for that seven tesla scanner because it's expensive So in those days it wasn't like that.
It was kind of a new Technology that we just figured out and it wasn't really used that much. And [00:05:00] I could sit there in the nights and evenings to kind of learn about seven Tesla. And after that, it just started to explode. And nowadays there are, I think, 120 seven Tesla scanners kind of sold around the world.
And there's a lot of demand for people with kind of skills that know how to use the seven tesla scanners for that matter So I was lucky to be there and learn the skills that are now under high demand So I that's kind of how I went into brain physics,
Benjamin James Kuper-Smith: But did you have any educational courses in like biophysics or anything like that, or was it Yeah. I mean, was it like you did a, I don't exactly know what it means to study physics in Germany, but typically in Germany, if they ask you to study a thing, you study that thing and not much else.
Yeah, I'm just curious, with what kind of background did you go into this and kind of what were your expectations of the field and what you were going to do and that kind of thing. [00:06:00] Uh,
Renzo Huber: Kind of that I'm kind of where to end up or even to become an academic or anything. It just happened. I like being surrounded by interesting people or intelligent people and people that I learned from physics was just something I was good in school. So in germany, how it does it look like studying physics?
It's pretty Strictly structured so there was physics and basically nothing else. So quantum mechanics and thermodynamics and then kind of a bit of cosmology And those kind of things. It's only then for the kind of bachelor thesis work or the master thesis work where you can kind of specialize a bit more and it was fairly. Flexible with the spec that I could actually, while studying in Munich, could go to a Max Planck Institute in Leipzig and then kind of find a way to, to ask another supervisor to be the official supervisor. And then while I'm actually working on something else in a different city. As I said, I didn't really know a lot about MRI.
It maybe was mentioned in my curriculum once in a [00:07:00] part of a lecture that, hey, you can use these spins of protons to actually produce medical images. And that was it. And in fact, there was a lot of serendipity in that, that they were talking about when I found it on Google at the Max Planck Institute for Cognitive Neuroscience in Leipzig and brain science was looking for master projects.
They were, had a job at saying that they are interested in adiabatic pulses. And I figured adiabaticity, I know adiabaticity, which is basically when you, pump your like in your bicycle pump and it gets hot, right? But when you move something faster than there's a thermodynamic equilibrium. And so I thought I'm working on this.
Okay, fine. I know my thermodynamics, but it turns out that adiabatic pulses are something completely different. Namely, it's when you, in MRI, you kind of sweep your frequency, off resonance frequency in a way, like slower than the usual, frequency of the spins for conventional excitation pulses in MRI.
So it was just a random, I still didn't don't like, I never took formal classes of brain [00:08:00] science. It's something you catch on as you're working in this discipline. And maybe it's also kind of one of the reasons why I haven't left this discipline since 2012 because it's so multidisciplinary.
So there, there's always, It's like you always kind of the smartest person in the room because you're the only expert in that very specific and theme of, and kind of that you're working on and everybody else is know something else and you can learn from them. And it's a great environment to kind of always combine different disciplines and brain and medical imaging and specifically with high resolution fMRI that, you know, That I like to do a lot.
They are kind of the hardware aspect. You need to understand how the hardware is built and how it's in perfectly built. You need to understand the vendors, a bit of kind of industry, what their choices are. There's a lot of kind of vendor research interactions. Then you need to understand your MRI physics, which is, I guess, the only thing that I'm really trained at until you get to kind of software to coding because Being in a kind of new discipline and getting new forms of data means that there is no [00:09:00] standard analysis method out there.
You need to develop it on your own. You need to become a computer programmer until you ultimately kind of end up with a neuro scientific conclusion at the end. So it's highly a multidisciplinary working as a MRI physicist in kind of a neuroscience and settings. And maybe there is no formal course ever.
I think in Maastricht University there are some exotic places now that kind of specialized on advanced neuroimaging methods or cognitive neuroscience method for that matter. And usually that's a small part within psychology departments or sometimes medical departments and so on.,
Benjamin James Kuper-Smith: I mean, so you stayed in Leipzig, I believe you, it seemed to have been good enough at least that you, you didn't want to leave. But it was a different topic though for your PhD, right? Or at least it ended up becoming that or,
Renzo Huber: I think the transition was pretty seamless. So the master, after I finished my master, I kind of formally transitioned to Leipzig to still work on seven Tesla, high resolution, FMRI, the kind of the theme shifted a bit, I was still [00:10:00] working on high resolution, FMRI for seven Tesla specifically blood volume, FMRI.
And that was not really by design or anything, or because I had a five year plan or vision, it was basically the path of least resistant as it happens so often, I guess. And it also, the reason why I'm still kind of in the field that as a master student, I kind of, I learned what I'm capable of and what I'm not capable of.
And the people you work with kind of know what you can and cannot do. And then it's the least risky thing to just take a position. If there's a way one available. And to stay in that field. And then this moving on from there. In Leipzig, I had, I was again, lucky that to be supervised by or co supervised by one kind of charismatic leader, Bob Turner, who was flying around the world and giving keynote lectures.
Kind of showing my results in his slides sometimes. And he kind of put me on the map and then suddenly you build a network and then there are people interested about your work. And then the easiest path of least resistance is then just to stay in the field and do a postdoc because other people know what you do [00:11:00] and so on.
It would have been maybe smarter. I don't know, but it would have been kind of a bigger jump to leave academia, to change the field or to go into industry and kind of jumping in the cold water and trying something completely new. Which I didn't do. So I'm not here today as a layer fMRI research simply because I always wanted this, it's just that it happened to be, and it was always that the path of least resistance and a good chunk of serendipity,
Benjamin James Kuper-Smith: yeah. So, one thing that's probably going to become, abundantly clear as we talk today is that Although i've heard a lot about fMRI and i've occasionally read about fMRI I haven't actually done much fMRI myself yet. It's going to happen at some point. But so I thought I could use that to ask a couple of introductory questions.
As we go along and one of them is now is kind of Okay, so I understand 7Tesla has more Teslas than a 3Tesla scanner. That, that's much I understand. Um, What does I mean, from what I understand, for example it's not [00:12:00] exactly the way that it's just the same thing with a higher resolution but it's a bit more complicated.
Um, So maybe what I kind of, the. I mean, maybe first why try and do 7 tester and kind of what are the main advantages over 3 tester and maybe also some of the stuff that makes it a bit complicated.
Renzo Huber: um, There are multiple angles by seven Tesla is interesting. Like first of all, it's a strong magnetic field strength. So, it increases the signal like substantially. So usually in MRI, we have. We are measuring magnetic fields specifically the protons in the water molecules aligned to the external magnetic field.
And however, it's only a small fraction that kind of aligns. Most of them are still random and because they have temperature and kind of flip. So, the stronger the magnetic field you have, the more of these protons tend to align along the external magnetic field and the more kind of magnetization induced magnetization you have available in diamagnetic water, the higher your Signal that [00:13:00] you do catch at the end and that's an advantage, right?
You have more signal and you can use that signal for all kinds of things in FM. I specifically we are limited by signal strength SNR when we go to high resolution because then that the voxels the three dimensional Pixels are getting smaller and smaller and we have less magnetization per kind of unit of information And then going to higher field strength seven Tesla is kind of is helping a lot.
And so I think actually a lot of the kind of drive for seven Tesla was motivated for high resolution fMRI as it open often. So it's so high resolution fMRI is from the vendor perspective, from the companies of Siemens, she Phillips that built those scanners. They don't really care about high resolution fMRI like at all.
And they, they think the big market is in clinical imaging. And. I'm still kind of seven Tesla as we have it today. Probably only exists in this form because people wanted to do high resolution and FMI. So, so high resolution FMI is kind of a technology [00:14:00] driver because in the early days, for example, in Minnesota, Camille Oglebill and they were interested in high resolution FMI and push the vendors to go to seven Tesla.
And then now seven Tesla is kind of FD year. FDA cleared medical product specifically for applications in MS, like structural imaging, or for cartilage, those kinds of things, even though originally it was coming from the domain of fMRI. The big challenges of fMRI, I guess, are currently that they're more expensive than a three Tesla scanner.
And so it's always kind of a rich man club and they are, I'm keeping track of all the seven Tesla. and ultra high field scanners around the world. And I think they are 126 right now that I have on a little Google map. So it's still not as much as kind of three test line. It's kind of the prestigious institutes.
It's not really a kind of something that's available to the kind of researchers in Africa and other countries. So maybe that's a kind of disadvantage to some degree. It's not really diverse. Another [00:15:00]disadvantage of seven Tesla kind of engineering challenges, which again, brings back maybe the vendors at the end, because at seven Tesla, the higher the field strength you go, the faster the magnetization precesses, like the spins, how they rotate in the external magnetic field and the higher the frequency, the slower the wavelength.
According to Einstein's equation so, so this means that at higher field strength, the wavelength becomes shorter and shorter. And ultimately we are in the range of centimeters where we have microwaves, like microwaves or phone waves are in the range of a few centimeters and day.
They are not ionizing, so they're not dangerous to destroy your molecules, but still they carry a lot of energy, a lot of heat. So at 7 Tesla, as we go to kind of magnetic fields and waves that are in the range of 10 to 10 centimeters to a meter, they start to approach the spatial scale of the head. So sometimes they are kind of constructive and destructive interference that like the center of the brain seems to be much brighter than everything else.
And [00:16:00] that's a problem for medical imaging. Radiologists don't like that. So there's technology around that. Other limits of seven Tesla might be that you just are at a higher risk of heating up the sample. So there are certain legal limits of how much you are allowed to heat a tissue, namely by one degree Celsius, which is the same legal limits for our cell phones.
And this means that we just cannot use as much RF energy, like a signal transmission and reception for MRI as we could at three Tesla, which is something we need to deal with. So they are kind of technical engineering limits that are. Kind of being dealt with. And the reason why the vendors come in here is because the vendors don't really care about that so much because for conventional clinical imaging, it works good enough.
They're not as limited by those factors so much as we are with high resolution fMRI. So, so for, from the vendor perspective, that's basically a solved problem and they don't develop it further, even though it would be easy to use advanced engineering skills to, to solve all the seven test ratios as [00:17:00] we have them.
Benjamin James Kuper-Smith: Okay I just have to interject because the way you phrased it, it sounded like you said Africa was a country, but I'm pretty sure you're aware that it isn't.
Renzo Huber: Yes.
Benjamin James Kuper-Smith: I just, for anyone listening, I'm pretty sure Lance is aware of that, when you said Africa, comma, and other countries. Yeah. The, yeah, I mean, so this is one of those fun things that I did.
And so, I mean, I've started like helping people collect data fair from Ryan, that kind of stuff, because, you know, you need two people in the room and that kind of thing. So, I've started helping some of that. And I, until I did that, I didn't realize that the brain heats up when you memorize kind of, and this was actually been one of the questions.
I mean, so for what I understand, roughly the. Time it takes to heat up a brain with a 3 tester scanner. I think it's also kind of roughly like, to the limit. It's also roughly the time that beyond that you don't really want to have people long in the scanner, because then they just start falling asleep anyway.
So it's not that much of a problem. But I'm curious for a 7 tester, like, how long can someone be scanned there? Before, [00:18:00] before we start steaming their head from the inside. Um,
Renzo Huber: mean, we are
Lucky at 7th Histler that usually though, we are only imaging part of the brain or part of the body. So, if there's energy deposited on the head, that's disseminated across the entire body pretty quickly. And so, from the legal limits which I think there are different like standards in Europe and us.
Overall, there's kind of an overall limit of how much you're allowed to heat the tissue. And there are usually safety factors by a factor 10 or more to really make sure that nothing's happening. So usually it's not that dangerous to deposit a bit of energy in the brain because it's highly perfused and most of the energy is just distributed across the body.
And if you're asking about the legal limits, I think specifically there are, there's a 10 seconds SAR, a SAR is a specific absorption rate. So within 10 seconds, you're allowed to. Put more energy in if you then allowed the heat to be distributed across the body in the next [00:19:00] six minutes, then I think there's also a six minutes and 10 minutes are there.
And you're also not allowed to exceed certain thresholds. And that's kind of supervised on many levels. Usually when I install a new sequence on the scanner it needs to be like, I need to have some energy budgeting. I need to tell the scanner how much energy I expect to be deposited. Then the scanner checks that.
And then also in real time there, they check how much energy is actually going through the cables. They match it with the expectations. And if anything doesn't make sense. it shuts down. And especially for a newer technology on seven Tesla, where you have multiple antennas at the same time, there's also the danger that kind of multiple waves are focusing and you have hotspots and there is a certain way of engineering and safety guards kind of to really make sure that's nothing is happening, which is all a solvable problem because ultimately those legal limits are Really extremely conservative like by a huge margin and nobody wants to kind of risk anything but at the end, I think the better [00:20:00] the models are if more users are really limited by those legal limits, then the models would be improved and the legal limits could be adjusted and fine tuned and they would not just kind of have a ballpark of safety factor 10 or something.
But there's energy deposited at the, yeah,
Benjamin James Kuper-Smith: Yeah, I mean, I guess it didn't occur to me that, I mean, it makes complete sense when you say it that, you know, I mean, related to fMRI, the whole idea of blood flowing through your brain is also that it flows out of your brain again, and then, I guess, you get new blood that then, yeah evens out with the rest of your body, I guess.
Okay, so, but it's not like when you use fMRI that you're necessarily restricted to, you know, much shorter, but Time that you can give people a task to do anything like that. It's more about the sequence the how you run that Okay,
Renzo Huber: two minutes. I think the longest scans that most people do is in the range of two to three hours or so. I think they did stream studies like overnight scans, which are kind of [00:21:00]uncomfortable for other reasons rather than eating up the person.
Benjamin James Kuper-Smith: Do people actually completely fall asleep in there from asking that's
Renzo Huber: Like I was a test subject that was a three Tesla. So though where I think I was in the scanner for eight hours and that was intense. Specifically also because they had EG on my head and then lying on top of that was just uncomfortable. They took out the patient bed and put a very soft mattress there and that was exciting.
There are a lot of kind of big sampling studies. Also kind of overnight studies where people fall asleep and then they're woken up and then they ask, What did you dream? What did you dream? Yeah, but
Benjamin James Kuper-Smith: Okay, but with EEG, I mean assuming they don't put the gel in then because that would just like squish all over for hours. I mean, if you, or do they actually still do the gel with the electrodes? Because I'd imagine just you know, you move a little bit, your head in a way, you know, just like melts your skull.
Renzo Huber: I don't know all the specifics. There are kind of, it is a pretty [00:22:00] big subdisciplines of FMRI, like multimodal Imaging where people do fMRI and EG at the same time. I think there are different kind of electrodes depends on kind of how the contact is. I think in my case it was still with the gel and it dried up and it was uncomfortable.
There are kind of more fancy electrodes specifically with those nets that kind of don't need that gel or different gels, but yeah, it depends. I think there's a lot of room for optimization, specifically the ones at the back of the brain. If you remove those electrodes and you're not directly relying like lying on top of them.
I And you don't feel like they're kind of pressing through your skull.
Benjamin James Kuper-Smith: Yeah, that can't be pleasant. Um, just a kind of final question or two about larger and larger scanners. I mean, is it in theory always better to have a bigger scanner? I mean, taking aside things like costs and that they take up more space and these kind of things. But like from a, you know, let's say cost was not an issue.
[00:23:00] And and space I mean, yeah, basically would you always be in, you know, would it be great for you to always have a bigger scanner? Or is at some point it doesn't make that much of a difference? It's old. The pros and cons kind of start to flip a little bit or I mean, I'm especially thinking because I did part of my master's at Neurospin in Paris where they this year had the whatever it was like 11 and a half full Tesla full body scan or whatever it was.
And I think they've published something very recently about this. Yeah, so I'm just curious is more Teslas always better or?
Renzo Huber: Like in theory, yes. If you would be in full control of the engineering, then yes. And I think it's just a matter of time. Like in, I guess in the early days, people thought that 7 Tesla is kind of this weird playground for physicists, and it's never going to be useful, and there are all these engineering challenges, and now it's a clinical approved scanner, and it just works.
With respect to layer fMRI in Maastricht, where I was, I had access to a 9. 4 Tesla scanner, 7 Tesla and 3 Tesla scanner, and you can run a high [00:24:00] resolution layer dependent fMRI on either field strength, right? At 3 Tesla, it's, you just need to average a lot because the signal is noisier. 7 Tesla is kind of established enough that it just works.
You upload your sequence and it's a turnkey method, mostly in most brain areas, that it just works. So 9. 4 Tesla. You can even have better signal, right? If you have a whole team of engineers working on it and then having prototype self built RF coils and then cables around, and then some of this breaks, and then you have an expert who knows what to fix, and then you get this awesome figures and images out of it.
At the cost of kind of having two successful scans per week so maybe seven tesla for layer fmri is kind of the sweet spot But obviously at the point when 9. 4 tesla or the 11. 7 in paris will be like straightforward enough There's a lot of signal to be harvested like these nature methods and papers and images.
They look like gorgeous Oh, well, they given kind of the surrounding setting, right? They have a lot of issues, of course. And I've [00:25:00] seen nicer images at seven Tesla, but not in the timescale. They acquired it. Like they, they have these multi equity images in four minutes or something that like, it takes half an hour for me at seven Tesla to kind of get to that data quality.
So 11. 7, where we have, I think. Four big projects around the world where they're building 11. 7s. Paris is the fastest one with the first human images and they, or in vivo images in humans, for that matter, they. That there's a lot of signal to obtained. And I think there's even their higher field strength scanners being proposed for the Netherlands at 14 Tesla.
And they had a nice proposal also in Germany. So there, there's always kind of more to further to push. And I'm not sure if it will pay out at some point. It's definitely kind of a new frontier. You will see things that you could not see before. And again, kind of seven Tesla like layer ephemeris. It's a kind of technology driver for seven test and other methodologies.
So seven Tesla is a technology driver for clinical field strength. [00:26:00] Like all this problem of interference of wavelength that we have at seven Tesla was only really well described and acknowledged after seven Tesla's were mainstream. And now a lot of this knowledge is being used at three Tesla to optimize three Tesla and make them more stable as well.
So I think there's this aspect that we, a lot of people just want to see how what they see and then go for it. And of course there is kind of the, who has the biggest, longest, strongest, and who is the biggest institution. And everybody wants to have the highest magnetic field strength.
And it is very challenging specifically. It's also not kind of so sustainable. These scanners need a lot of helium as well, which is kind of scarce, up and down sometimes. I think for layer fMRI, it's not only the field strength itself that matters, but also. What you mentioned, like in Paris, they have a whole body scanner, which is ironic because kind of they will use it for head imaging.
And so most of the seven Tesla scanners out there are whole body scanners with a huge bulky gradient that's kind of optimized to be [00:27:00] flexible because that's what the vendors want. At the end, however, most of those people use it for head imaging. And if you would make smaller gradients, that would just be more efficient.
Kind of be suited for the head only. We could really increase that kind of the performance way beyond. So a seven Tesla scanner with an awesome gradient can. Maybe 4 Tesla scanner. And there are some initiatives about those awesome gradients too. For example, in, in Berkeley, the Feinbergatron, we call it the impulse or next gen 70, which is as low a few strengths, but other components of the scanner are really optimized for head imaging, for fMRI.
And those gradients are very popular and being sold like hotcakes now.
Benjamin James Kuper-Smith: Yeah, I mean, this is something I find quite interesting. I think it might have been in my episode with Peter Bellassini that he mentioned that, you know, the big advantage of fMRI when it started was that the machines already existed. They were everywhere, right? It was just a standard MRI scanner that was in every hospital, more or less.
And I guess the, that's kind of being lost to some [00:28:00] extent because now it's, as you said it's trying to. Develop it as its own thing and take it to places that maybe a clinician who just wants to see I don't know what clinicians want to see but like where the ligament is torn or whatever.
I don't know you know, maybe they don't need and then where they can scan the same thing for a couple of minutes and where they just don't need that kind of resolution and where maybe the extra cost isn't really justified So I think it's kind of really interesting to see kind of what happens now when You I mean I guess it's been going on for a while, but when people really take it into direction for its own sake rather than just because the machine kind of happened to be there.
Renzo Huber: And at the end, I think it's still kind of a tandem, right? We are in this symbiosis with the vendors. They don't care about fMRI and still they're these new scan is being built, but then the vendors kind of do that because they might learn something that they can use and market for clinical environment.
So like a lot of developments, let's say like multiband, maybe you heard about that kind of accelerated imaging aware, which was a big game changer in, in [00:29:00] kind of increasing the spatial temporal resolution was. built for fMRI and then now the vendors are earning a lot in kind of selling licenses and using it for clinical scanners.
So I, it's always kind of this back and forth, even though the technology doesn't really exist yet, still kind of, we build it for kind of that purpose and then another field benefits from it and vice versa. And now, We benefit a lot from the fact that at seven Tesla might be very useful for multiple sclerosis, right?
The, you have these veins very visible at seven Tesla, not for three Tesla. So we can build those, like we can buy those seven Teslas and we don't need to build them ourselves, even though they might originally have been built for layer from right now. They are kind of a factory clinical product and we can just buy that.
And for my time as a physicist, and I learned how complicated it is to When you cannot kind of buy something, right? And when the Large Hadron Collider had a quench, like the superconductivity, it broke down and it tore a part of the accelerator [00:30:00] apart. It you needed an entire team of physicists, quantum mechanics, to know about could prepare superconductivity, repair it.
It took two to three years until they could restart everybody else. Thousands of researchers were waiting. So in MRI, we are lucky enough that we kind of can piggyback on this kind of clinical work. Scheme from the vendors, they benefit from us, but also we can just buy a scanner. Yes, they're expensive, but it would be like orders of magnitude more expensive if every seven Tesla layer at my center would need a team of physicists that basically wire their main magnet and know all the ins and outs and yeah, it would not be as stable as this kind of a factory production. So it's still kind of true that we benefit from them and they benefit from us.
Benjamin James Kuper-Smith: a brief question just because the, from what I understand pretty much, you know, the more, the bigger the magnet, the bigger the machine is that like a necessary thing or is it just we haven't figured out how to do the engineering better? I'm, just curious because like at some point I mean, I [00:31:00] think i've seen the images from Paris it's you know, it's an enormous machine.
You can't just put that into the cellar of a hospital, right? And so i'm curious like is that something that's just like always going to be the case or do you think? We'll be able to I mean, I guess it's always a risky question to say, no, this isn't possible because then there's always going to be some, you know, someone's going to have an idea in 50 years and then do it.
I guess, like with MEG, we didn't think that probably when they started that now you could do it and like the size of a remote control and put a bunch of those around your head. But yeah, so I'm just curious because. Yeah. Yeah. I'm just curious. Do you think that's always going to be the case?
Are we going to figure out how to make smaller magnets? So that you know, we can have a super small fMRI.
Renzo Huber: It depends. It's less a physics question than kind of marketing engineering. The one in Paris is exceptionally huge. The 11. 7 Tesla that we have here in, at NIH, that's not on 11. 7 because it quenched again while ramping up beyond 11, [00:32:00] but not at 11. 7. That is a head scanner and it's way smaller.
It's almost as big as a seven Tesla scanner. Now the plans for this 14 Tesla in the Netherlands is actually as the size of three Tesla scanner, because they're using a completely different technology. They're not using the conventional superconducting wires that are. Kind of cool with liquid helium.
Usually those wires, they lose their superconductivity beyond certain field strengths, maybe 10. 5 or so, where you need to start to pump helium. So at, kind of, at the temperature of the 4 Kelvin, when helium evaporates and cools the magnets, then you cannot keep up with that magnetic field strength, so you need to basically keep pumping helium.
reduce the pressure of the helium, and then it evaporates as soon as you can reduce the temperature even further. So now that the new design on the 11 on the 14 test land in the Netherlands, it's using a different material. These high temperature superconductors where you Like it's a different technology.
Nobody has ever done it. So who knows if it's going to work. But if you look at those marketing pictures and how they envision it, [00:33:00] it's kind of a person and next to it, there's a scanner of the size of a person and it might be kind of more complicated to ramp it up and to build it, but as soon as it's on field, then it might be a kind of more stable.
That's what I've heard in the lectures so far, but it's all kind of in the concept stage. They got the funding. The magnet doesn't exist yet though. But in that case, no, the scan is tiny.
Benjamin James Kuper-Smith: Okay, cool. Yeah. I just was curious. I just didn't, I was curious with whether, you know, sometimes you just have this like physical, Requirements and just that's the way it's going to be, but okay, cool. Yeah, I mean, so I initially invited you to talk about layer fMRI. We haven't done so, so far.
How did layer fMRI enter your career?
Renzo Huber: I think it was always there. Layer fMRI was always part of fMRI in itself. I think like fMRI has the unique feature that it has high spatial resolution, like more so than EEG or MEG or PET even. So I [00:34:00] think among the many disciplines of fMRI method development, high resolution fMRI was always kind of a.
Let's say the discipline supreme or something like every center that was involved in fMRI development world leading center that invented fMRI and kind of are still pushing for high and high resolution because it has the highest promise of showing something completely new. Nobody could ever kind of see the living human brain at this spatial scale with any other method.
So I'm pushing for resolution makes a lot of sense. I am not saying that maybe I should use a metaphor like there are all different disciplines in fMRI method development. Some people look at quantitative fMRI for clinical applications, white matter fMRI or some people look at very challenging spinal cord fMRI and those kind of, which are kind of dedicated people that know the literature and innovative and similarly in high resolution FMI, but high resolution FMI is just always getting more attention.
So since the nineties, people push resolution. And so did I always try to push as much as I could, because [00:35:00] why not have higher Quality high resolution data. So in some sense, it's like in the Olympic games, right? You have the fastest man alive, right? You're saying bold. Everybody knows kind of those disciplines, but do you know the name of the fastest sailor or the fastest disc discus thrower?
So, so, so high resolution FMI is always kind of got more attention than. then other disciplines. So I started on blood volume imaging, which was a bit more quantitative and they just pushed resolution as everybody does and kind of entered into high resolution also because It's a kind of unique selling point of having a high field scanner and it has the highest chances of just seeing something that nobody saw before.
And there's a lot of information at these spatial scales of kind of the few hundred micrometers in the sub millimeter scheme where kind of all this cartography, And way off of doing fMRI is that the conventional field is doing is fails, right? Do you get more information on how the brain areas is involved in a given task at high spatial resolutions?
So that's why I kind of stayed there and it was valuable and perceived as valuable. And [00:36:00] so I could kind of have positions and have a job.
Benjamin James Kuper-Smith: That's nice. So is layer fMRI then, I mean, how is layer fMRI then different from just high resolution fMRI? Is it just a marketing term? Or is there, what's the precise difference between, yeah, just high resolution fMRI and layer
Renzo Huber: There isn't no almost there isn't no it's a marketing. Well, it's a flashy, I think actually high resolution FMI started mostly with columnar imaging. So where people looked at layers, but not see how the layers are different, but basically how the layers are the same. Okay. The dominance columns in 2007, 2008, they were kind of, Iconic images of people also looking at orientation and ocular dominance columns.
Also before that I think only then later that we kind of basic cognitive neuroscientists came along and used it a lot for kind of their circuitry questions. In. layer fMRI, they specifically were looking into things like feed [00:37:00] forward feedback, which is kind of different layers, like feed forward input, like in the visual cortex, then you have input sensory input from the eyes go through thalamus into the middle layers of the visual cortex, whereas upper and deeper layers are mostly receiving feedback from higher cortical areas.
So a lot of people started looking into layer fMRI from that angle. There are different layer models, like there's predictive coding where people predict that Kind of prediction errors are estimated in different layers than others. There is kind of Modulations of brain activity with respect to attention.
There are and less cognitive neuroscience researchers that are more interested in kind of how the biomechanics of the brain works that look at kind of vessels versus non vessel boxes, like superficial kind of stuff that happens above the cortical ribbon versus stuff that's within the cortical ribbon, which is some form of layer fMRI.
Currently it seems that most high resolution fMRI is really done on the layer level. And that's why it kind of, that's a marketing thing. People don't like it because we're looking at, you know, Some people don't like [00:38:00] it and electrophysiologists don't like it because they say, well, it's not true layers, right?
If you look at three layer groups across the cortex, that's not the Brodmann layers, the cytoarchitectonical Brodmann layers. So some people call it laminar fMRI. Which is just a Latin word for it. And some people call it masal scale because it's not at a microscopic scale of the neurons and not the microscopic scale of the brain areas, but it's kind of somewhere in between, but it doesn't mean anything.
Masal scale. It's just middle scale. Like in physics, when you talk about masal scale physics, you're not in the range of planets and not in the range of atoms, but in the range of few kilometers of weather and those kind of things. So every disciplines defines the terms as they want to. I think RI is, it's a kind of catchy enough name and it's kind of aims it shows what we are aiming for. And I have a blog post about the terminology, but it's mostly marketing. And then some people kind of the purist, they call it cortical depth dependent fMRI, but that's a mouthful, right? It's not a cool Twitter handle.
Benjamin James Kuper-Smith: [00:39:00] Yeah. Okay, so that was one question. Like, Laminar and layer fMRI then is the same thing? Oh.
Renzo Huber: Yes.
Benjamin James Kuper-Smith: Okay. , but like presumably you are still focusing on layers rather than, you know, areas of the brain that don't have layers. You know, you could also try and get more precision in the hippocampus or whatever. So, so I mean, it's not just marketing, right?
Renzo Huber: No, it's not. I think that the most valuable immediate additional information that high resolution can give us is by looking at different layers, because these different layers, they have different neural populations, subpopulations that kind of do different things. So, and by looking at layers, we can get a lot of information that we cannot get with kind of looking at the big structures of just the brain areas.
Maybe to give an example, like the study that convinced me that layer fMRI. It gives us more than kind of cartography. Blobology FMRI is by looking at the simple example of the motor cortex, like in a motor cortex, it's close to the antennas. It's perfect signal. It's pretty thick. The superficial layers, [00:40:00]layer two and three, they have a lot of cortical input.
Like when you reach to grasp something, for example, you need to have certain motor planning input, then there's sensory feedback that all comes into the superficial layers to combine that, and then the deeper layers in a motor cortex, they have these. these output circuits, recurrent circuits that have the gigantic pyramidal bed cells that have the direct connections to the spinal cord, and then they trigger the muscles to move.
So now, if you have a motor task, And do fMRI, which area do you see activated? Of course, the motor cortex, right? But it doesn't really tell you that much. It's I like this metaphor of trying to understand how a truck engine works by looking at it with an infrared camera. If you want to understand kind of the truck, it has all these different components.
How does it work? And during driving, we see, okay, the front seems to be heating up, right? It consumes a lot of energy. Yeah, it's cool to see it or when you push the brakes or something else He seems to be heating up and that's kind of the level of kind of an fMRI at some point I am so in layer dependent fMRI we get to a different spatial scale of the kind of microcircuitry We [00:41:00] not only see and kind of if that the front is heating up but more kind of why it's heating up So for example in a motor cortex when we ask people to do a finger tapping.
We see that the corticocortical input and the corticospinal output, both layers are kind of activated, both layer groups. Now when you want to spice this up, make it a bit more neuroscience sexy and ask people to not tap their fingers, but just think about moving their fingers like mentally, they lie there, don't move their fingers.
And then what area would you expect it is sliding up? Of course the motor cortex, right? The circuits are there. It's responsible for hand movement. So of course, in both cases. In conventional resolution fMRI, we see, okay, the motor cortex lights up. So we didn't really learn a lot, right? Maybe the activation strength is different, but overall, it's frustrating how similar the brain looks given this very different behavior.
And this is, I think where layer fMRI comes in because for the imaginary tapping as being done by Andrew Pizzicati, maybe inspired by, by Robert Turner and Robert Rumpel in Leipzig, before they found that, And for imaginary tapping, it's [00:42:00] actually only the superficial layers that are being activated.
There's no output, right? The muscles are not being engaged. So layer fMRI tells us. More than just whether a brain area is involved in a certain task, but kind of how it's differently involved for certain tasks. And there are a lot of features that are interesting for cognitive neuroscientists that always engage the same brain area, but in a different way.
Let's think about attention, right? If I focus on the top right of my screen now and the bottom left of my visual cortex will be differently activated if I pay attention to it. But there's, you cannot answer attention with cartography, right? There's no attention area, right? It's rather individual cortex.
It's kind of the feedback input that activates and enhances the activity in the respective visual cortex. And that is not really driven by feed forward input, like from the eyes, the input from the eyes into the middle layers is the same. It's only the regulatory feedback input in superficial or deeper layers that is being kind of differently activated.
So you can look at those kind of non local features, which is [00:43:00] specifically also interesting if you want to understand something like multibodal integration. There are a lot of areas that are engaged independent of which sensory modality you use. Let's say V5 or planum temporale. Those are areas kind of, responsible for perception of motion.
You can perceive motion with kind of having moving dots on the screen or with auditory tasks. where you hear something moving and then with layer dependent fMRI you can kind of answer those questions on how there's feed forward coming in directly from the sensory areas or if kind of the how much is feedback coming in from another modality and how it's integrated together which we're also looking at with Marco Bavillari in Belgium looking at blind people.
Other applications where lay FMI is particularly useful is kind of things like psychosis, right? When you have hallucinations where there's of course auditory activity for auditory hallucinations in the primary auditory cortex Even though there's no directly forward input from the ears through the brainstem going to the ear It's kind of it's a misbalance between kind of sensory input versus kind of [00:44:00] other cortical areas taking over that area And there are a lot of theories in psychosis You and kind of in, in psychiatric research, whether this is coming from too big feed forward input or too strong feedback input and layer fMRI can ask all those questions beyond just saying whether or not there's a brain area involved in that certain task in like cartography and still having the kind of unique advantage that, that we are still, we can do it in large coverage.
Like many of the things that I mentioned, you can. investigate in kind of an animal models with electrophysiology, right? The so called true neural activity, as they call it sometimes a bit righteously. And layer fMRI is kind of in between those different disciplines. So the true value of layer fMRI might be to kind of bridge the gap between cartography and kind of the cellular circuitry electrophysiology.
And maybe I can use another metaphor here that. Specifically in the context of being at NIMH we know that most of our, like most of psychiatric [00:45:00] diseases are network diseases, right? And so that there, there's, it's not a single area that's kind of screwed up in schizophrenia or autism or whatever.
It's a network that's screwed up. So how do you investigate that? Right. And in, in fMRI, we kind of see that the network and that it might be screwed up, but we don't really know why. Okay. And. Maybe in the metaphor, let's pick a network that everybody knows like the street network of the United States, right?
If we can look at the connectivity of Chicago in New York or whatever and look at the storage halls and how much Connectivity is going on there on the big structures right the cities and then when the network is screwed up. We don't really know why. Is there a traffic jam, for example, and then the connectivity is broken.
So just looking at the big cities and that the network breaks down doesn't really tell us that much. Now, electrophysiology, they are the kind of looking at a true neural signal at the smallest mechanistic level. In my metaphor, that, that's maybe like the brake pedals of the cars, right? The ultimate thing that makes cars not go and not connect those two cities is because people press their brake pedal, right?
So, The true mechanistic reason why the [00:46:00] network breaks down is the network pedal. So we should take them apart and then, and look at them in the microscope, which is absurd, right? So I think that the mesoscopic regime not only looking at the cities and not only looking at the brake pedals but trying to understand where, why we have a traffic jam, right?
This network is corrupted or screwed up in a certain disease. It's the mesoscopic regime. Like in Google Maps, When you look at your traffic pattern, it tells you the parts where it's red and where it's orange. And it tells you which lane, right, which direction. And this is the unique information that allows us to better understand why the network is screwed up.
So in layer dependent fMRI, we not only know if two areas are connected to each other, but we know that the layer that is responsible for this connectivity. So for example, in hierarchical brain systems, we know that like early visual cortex sends feed forward input to the mid layers of higher order areas.
And the feedback from higher order areas to. Lower top down feedback is coming in upper and deeper cortical layer. So we learn something about the causality of this connectivity. We not only learn if they're connected or not, and how strong this connection is, but we learn something if [00:47:00] kind of it's the forward or the backward connection that's kind of screwed up.
We still don't know how to fix it, but we kind of, it gives us a unique spatial regime to kind of bridge the gap between, you know. human neuroscience and kind of the cellular neuroscience. And they don't really talk to each other a lot. And it's a huge opportunity to kind of fill this gap of this medium mesoscale regime, let's say of a few hundred micrometers.
Benjamin James Kuper-Smith: how well does the precise imaging of layers work then? I mean, is it, you know, I think you mentioned earlier that some people might say it's not exactly layer friendly because it's not the, all the layers you might want. So like how well does it work already?
Renzo Huber: Well, I use the term layer as a loose term and maybe I should say called layer groups. And I guess it, it always works as, as precise as your model is. So I mentioned a lot that we have this kind of feed forward [00:48:00] model, filament on S model, which I think is also used in predictive coding. For instance, I picked that up that kind of a certain feed forward and feedback is coming into middle or up and deeper layers.
That's a three layer model. And of course I can only be precise as this three layer model. Now when we have 0. 8 millimeter resolution for a two millimeter thick cortex, that gives us roughly around kind of the right spatial scale to kind of answer anything about the three layer model. We are not really solving the six layer model, but we don't have a good model kind of for the six individual layers differently.
And there are other models like in the frontal cortex and actually with Emily Finn, your previous guest in the podcast, we were looking at layer fMRI in the TLPFC, dorsolateral prefrontal cortex, where we are looking at a more input output model. There are these upper layers that have the Q cells, delay cells that kind of keep a string of letters, or think about your phone number, they keep that information in your working memory for a few seconds, and then the deeper layers and send the output to the motor cortex if you need to press a button [00:49:00] or if the monkey needs to do it.
So there's kind of the input output model. There's the. Feed forward feedback in the, you mentioned hippocampus, it has layers. It's not a new cortex. It has three layers, right? And typically there are these kind of like in the endorhine cortex, which is not hippocampus, but kind of the relay station we have kind of the upper layers on the deeper layers to different things with respect to memory.
There's the memory encoding and there's the memory retrieval in hippocampus for the different sub areas. They also did different upper. Or deeper, or let's call it inner and outer layers in hippocampus and that are more responsible for different pathway of kind of pattern completion or pattern recognition of kind of events or pictures or whatever.
So, in that sense, How precise is it kind of, it can be precise as your model is. And sometimes I got like a curse and it's a curse and a blessing to work in something new. And the curse is that you need to defend your stuff to other [00:50:00] disciplines that are kind of established. And sometimes when I'm getting towards kind of circuitry, I get review of feedback.
That people say, yes, you have reduced your three millimeter voxels of 700, 000 neurons now to 1500 neurons of one voxel, but you cannot be so naive to say that all these neurons are doing the same thing. So it's in that aspect, they're right, right? It's still not exact. And even kind of, if I would be able to resolve every of the six different layers I cannot be so naive to, to assume that all the neurons in the given layer are doing the same things.
They're at different populations in, in, in every layer. Anyway, so at the end, I guess the question is kind of the model that makes sense for you. And I think these standard models, input output, feedforward feedback are quite successful right now. Ultimately, there are still simplifications.
That the big advantage is that They are better than just assuming that every neuron in the entire brain area is doing the same thing, or that every neuron across the entire cortical depth is doing the same thing. Kind of the one layer model is obviously not as great as a three layer [00:51:00] model, even though it's kind of not super precise just yet.
And ultimately, I think it's we are maybe lucky that layers are just kind of a basic neural building block. If you resolve it further and further to kind of then the cortical columns or the cortical layer. I'm not sure if you would gain that much kind of on a, kind of looking at the whole brain perspective.
Layer from my sometimes it's criticized as FMI that it's an indirect neural, like measure of neural activity. You're not measuring the neurons directly, but also I'm not sure if we really want to like being a physicist here. I think. Whenever people call their kind of what they're working with signal or activity Without even a physical unit attached to it they don't know what they're talking about And we call it signal bold signal or neural like fmi activity and then I think that's right But what is the unit?
the ultimate truth. Neuro as a physicist, I think the ultimate unit that we are interested in is energy. It always comes down to energy in the universe and in the [00:52:00] brain. And I think FMRI just gives us that, right? FMRI is a hemodynamic response that's triggered by oxygen consumption of the neurons, right?
So if we would have, In kind of 100 years in the future, if we would be able to measure the neural activity of every single neuron in the entire brain, and not only looking at multi unit activity and looking at frequencies and coherence, if we have all information about every neuron in the entire brain, what would it give us?
Right? How would we be able to like make, take advantage of that how we distill it down to a kind of a meaningful understanding of how the brain works. And I think ideally we would want to have a conversion function that converts all these different measures of spiking of different frequencies and the slow waves, faster waves into something that kind of tells us something about how much information is processed.
So we would like a conversion from kind of all these different things that the neurons do where we don't really know which one of those measures has the [00:53:00] most information in there. Some of it might just be smoke from kind of actual other work that's happening. So I think energy is a good unit of activity and FMRI just gives us that at the spatial scale of the basic neural building blocks the layers and the columns.
So yeah, to answer your question, how precise is it? It's precise as your model. And I think that the model of the layers as kind of basic neural building blocks and looking at the energy, how much those neural building blocks use seems intuitive and implausible to me without needing to discredit kind of electrophysiologists.
Sorry, it's very valuable research and somebody should do it.
Benjamin James Kuper-Smith: So, I mean, you said it's as precise as your model, but you can't do all six, right? Did I misunderstand that then? I mean, it's, there's still like a limit to this, right? 200
Renzo Huber: There's this Moore's law of FMRI resolution where I believe so far it's right that every decade we get an order of magnitude in voxel volume reduction. So in the early studies from Peter [00:54:00] Panettini, I think they had 200 milliliter voxels, like huge slices for other reasons, because they didn't know where as, as he explained in your podcast as well.
And then there was a lot of hardware improvements in the last, maybe 20 years, not so much hardware. It was mostly. Kind of sequences and reconstruction improvement, but still we are kind of getting high and high resolution. The highest resolution I've got was 0. 39 millimeter isotropic at the Berkeley scanner, where We could look at some of the thinnest cortices in the brain.
Motor cortex is easy. It was an easy place to start because it's four millimeter thick in a visual cortex via in the range of two millimeters. And there's very hard to distinguish kind of even the different layer groups like feet forward and feet back. Feedback circuits with kind of Nyquist sampling, where you have a layer, a space in between, and then another layer and so on.
And there with 0. 4 maybe better with 0. 35 millimeter resolutions, you really start to separate the kind of, the closest units to begin with. You're right. I think nobody has ever done [00:55:00] fMRI with resolving the six layers. Don't. I'm not really sure if it would get us so far, like layer one is tiny.
It doesn't have much interesting stuff going on anyway. And then maybe layer four instead, it's much more interesting. It has different groups like, like for a, for B, for C. And then there are different MCs that have the different kind of feed forward input from the thalamus in the visual cortex. So I think the.
The highest we currently can hope for is kind of to have three different layer groups and have them Nyquist sampled, not only have them at three different boxes, but actually boxes in between that are not really dominated from either one. And I wouldn't really know what to make out of the sub layers.
Also, very often these different layer groups have huge kind of recurrent connections. So you cannot really. With FMI timescale separate them anyway. So in the motor cortex, when I talked about the output circuits of layer five, I was specifically thinking about a layer five beam and six. So if six is activated, it [00:56:00] activates layer five and so on.
So I'm not really sure if we can tease them apart. Maybe we can a bit, but yes, we cannot resolve all the six layers just yet. And I'm sure in 10 years we have higher resolution and then we will see if it's useful to, to go even further.
Benjamin James Kuper-Smith: Okay, I didn't realize there was such a clear progression. I mean, I guess it's been fewer cycles than with actual Moore's law. But if it's on the, if it's on the order of a decade. But okay, that's so cool. Yeah, I mean, one question I had in general is that It's basically like whether layer specific information in a sense is equally applicable or useful for different types of research.
I mean, for example, you know, as I said before we started recording, I did, One of my master's projects on predictive coding and, you know, so from imperception in particular and so from that it's very obvious that it's very useful and, you know, that it would be supremely useful. And especially like some of the clinical [00:57:00] applications you mentioned were also some of the things I was thinking of.
There it's built into the theories of like why these methods would be useful. Now I work more in like value based decision making and There I've, you know, read less about, I can't remember if I've read anything. I don't know, maybe I just haven't read enough.
But I'm at least not as familiar with layer specific information. And so for this, for the kind of stuff that I might be doing in the next couple of years I'm not entirely sure how useful it would be there. But so yeah, that's basically my question. I mean, presumably they're always thinking where it's more or less relevant, but yeah.
Renzo Huber: I'm not a kind of decision expert myself. At the end, if you look at circuitry, layer fMRI will be useful because you kind of know which area kind of sends feedback in one direction or the other if you kind of know your circuitry from tracer studies beforehand another angle would be that high quality data are always better than low quality data.
So having this additional. information of layers. It's always kind of more [00:58:00] useful if you even if you don't have any layer questions or the power to kind of look at play from my noisy signals and with kind of cognitive tasks like decision making. It can sometimes still be useful to do look at layers, not from the perspective of kind of your layer model, but basically from the perspective of your noise model that you know that They're on the on the cortex and is on top of the cortex above the surface.
We have a lot of vessels, like we have arteries, they pulsate, there's CSF floating around and with respect to kind of respiration and heartbeat. This introduces a lot of physiological noise. And as soon as you have big voxels and they have partial voluming, a small fraction of that voxel contains this noisy signal from CSF that has nothing to do with your task.
It kind of compromises that the signal that you care about, the bold signal in, in the deeper layers of the cortex. So some people also propose to do layer dependent fMRI specifically for denoising, basically, even at lower resolutions, maybe one millimeter or 1. 5. [00:59:00] Just don't. smoothing, not like Gaussian as some people do it, but basically only smooth within a given cortical layer.
If you, even if you don't really resolve layers at the end, you basically remove the noise that's above the cortex, that kind of superficial most layer to some degree. So there's always kind of something that layer FMI could give you, I guess.
Benjamin James Kuper-Smith: yeah, just to focus on the one of the early things you said. I guess it's also like one of the points you made earlier, but I think maybe that's worth highlighting. One of the main advantages that we kind of have a general understanding. So this is a question that we have a general understanding of.
that actually everywhere in the brain, specific layers do specific things. So for example, if we see that, you know, this area lights up then with layer specific information, we might be able to I mean, could we be able to then say more that, okay, we think we're, it's just processing this information or it's receiving or just sending it.
I mean, Again, like in a predictive coding sense. I understand more what [01:00:00] like the, you know, feed forward and feedback things would work. But if it's not about prediction per se, then I'm just trying to figure out like what would tell me exactly if I know that the information is received rather than sent.
Because presumably if it's received somewhere, it has to be also sent from somewhere, right? very much. I mean, okay, so then you know from where to go. Okay. Okay. Yeah, okay. Okay
Renzo Huber: But even kind of, if you don't care about layers at all, I'm going to high resolutions. Like even from a cartography perspective, you can kind of better delineate that the information processing within the two dimensional cortical sheets and going to high resolution. There's a lot of kind of kind of open ground where nobody really knows what to expect.
So, we have been quite lucky. Like even in simple things like in a motor cortex that if you don't care about layers and actually in that study we were smoothing within each column to completely get rid of the layer signal just going to high and high resolution allows you to see new things that you might not have been [01:01:00] expected and then you get more kind of hypotheses from that and then go on and so forth.
For example, in the motor cortex, we found that as you go to high and high resolution, to look at finger digit representation. For example, in the sensory cortex, everything was like we expected it, right? Neighboring fingers were next to each other in the brain. And we have this kind of homunculus model in the motor cortex.
It was completely messed up that as you go to high and high resolution, we actually see that Yes, the different spines are kind of next to each other, but as you go to the kind of microscopic mesoscopic regime of the columns, you see that within the hand knob, there are multiple representation of each fingers and the motor cortex even though in the textbooks is kind of simplified as this homunculus model, it's actually more a muscle map, not really a homunculus map, but they are kind of multiple mirrored finger representations within the hand knob that with conventional resolutions, you just didn't see.
Actually, also in the original literature from Penfield's Textbooks, of course, are always oversimplified, and there were already indications there that the same neurons are actually [01:02:00] responsible for multiple fingers. And it makes a lot of sense to kind of now then rethink the motor cortex as a more an action map.
If you use the hand rather for grasping versus retraction with those muscles for extension or flexion, they are differently represented. So even though we didn't really expect that and just wanted to see kind of the topographical representation for a task where we kind of knew what to expect, going to a higher resolution allows you to see something new and then ask new questions and then build new hypotheses based on that.
So no matter what you're doing, if you have good signal and you can do it at layer resolutions, then you might see interesting things that are worth pursuing, I believe.
Benjamin James Kuper-Smith: So, how do I do that do I have to Okay, maybe first question. I believe you already kind of mentioned it very briefly earlier do I need a seven tesla scanner or higher to do this?
Renzo Huber: 7 Tesla is helpful. There is,
Benjamin James Kuper-Smith: it's good to have one but
Renzo Huber: it's good to have like in most brain areas, let's say. Overall, the field of layer fMRI kind of is still exponentially growing so fast that it's [01:03:00] kind of outrunning the ultra high field. Again, layer papers, there are about 260 right now and they still double every 40 years or so, three to four years in the ultra high field scanners only double about every seven years or so.
So you're kind of running out of scanners. So there's kind of a pretty big drive in the field to also move this to three Tesla scanners, which not only. Makes it more accessible to continents like Africa or other countries. It also kind of makes it more diverse and allows kind of more people to use that technology.
Very so in a recent study with Martin in Salzburg, we kind of quantified the usability of layer dependent FMI, blood volume, FMI, which is what we did. I do a lot at three Tesla and it turns out, yes, you need to scan a bit longer, maybe two times, maybe three times longer, but you can do a lot at three Tesla as well.
And this is even if you have the high ambitions to see kind of voxel wise uh, Um, activity in a kind of an entire volume based [01:04:00] analysis where you want to see for each voxel, how activated it is very often neuroscientists don't really have kind of the need to look at kind of, maps, so to speak, and in the 3d volume space, but often they want to know if that lay in a given brain area.
It's acting different than the different layer in that brain area. So you can, you have the luxury to just run very high resolution FMI and then just average all the voxels in a given layer group, compare them to all the voxels in a different layer group. So even though you don't have enough signal at kind of noisier field strength of 3 Tesla, If you average a lot of voxels at these high resolutions, you still get a lot of signal back.
And very quickly you are in a noise dominated machine where you're not limited by the thermal noise of the scanner, but rather limited by inter trial noise or physiological noise and those kinds of things That seven Tesla doesn't really help with anyway.
Benjamin James Kuper-Smith: And so do I need to You know as a broad question Do I need to do anything differently? From let's say when I collect the data is it do I get layer specific information by analyzing the same data [01:05:00] differently? Do I need to set up the whole thing differently from the beginning? Yeah
Renzo Huber: Yes. This is a yes. Also getting already to the disadvantages of the fields. Yes, there's a lot different. You need to acquire them differently. Specifically. There's one big problem and challenge in lay dependent FMI aside of the fact that it's noisier as you go to smaller voxels. But the other thing is that.
With conventional FMI methods like gradient echo bold. you are more sensitive to large straining veins. Like most of the oxygenation changes that we see with great neck and bold are happen to accumulate in a large big vessels that are not closely aligned to the laminar neural structures. So, one trick that I often do is to use a different FMI contrast, which is called vaso to be more locally specific to the blood volume change in the micro vessels that are closely aligned with the laminar neural structures.
So you use a different sequence. You use a different readout. Usually in the analysis, I think that's a big question. Also there, you, there are more and more kind of streamlined layer dependent fMRI analysis, specifically [01:06:00] there's a software tool, Laney, that I'm writing with from Google that tries to help there, but overall, it's not like conventional fMRI in conventional fMRI.
It's really standardized. There's a conventional way of doing it and you can run things like fsec prep and it just works In lay fmi, that's not the case. You kind of need to fine tune more specifically also with respect to tissue Segmentation you need to have higher quality standards like in lay dependent fmi.
We need to know kind of at which layer a given voxel centroid is located. And in order to estimate that we need to have kind of geometrical models of the layers and given the cortical curvature. So, to start with that, we need good tissue type segmentation. We need to know where the, Gray matter ends and where it starts between gray matter, white matter and CSF.
And there are a lot of automatic tools out there, good tools, FreeSurfer, Brain Voyager, and so on that work quite well for conventional FMI. In layer dependent FMI, often we need kind of higher quality standards. So very often you need to go manually in and correct things. And [01:07:00] that's a problem. The first problem is that it's not really scalable.
So if you just want to do it in your decision making paradigm where you might have 60 participants, it's just a lot of work to go in there for every data set and manually correct it. So I think ultimately this will be solved with better analysis methods. Maybe machine learning is a solution. I don't know.
The second big problem also is maybe a more conceptual difference compared to fMRI where. FMRI and neuroimaging, they had this reproducibility crisis and I guess their solution to that is to use large ends and to use kind of user independent analysis, which is different than other disciplines.
Like in, in kind of cellular neuroscience, when you put your electrode, you have the animal on your surgery table. You need to put the electrode in, listen to the neuron, pick a different one, and then until you find it. And there's a lot of manual skill. There's a trait to it. So in lay FMRI, we are kind of, as we are in the different spatial scales, we are kind of in, in the.
in the regime between standardized fMRI, kind of [01:08:00] large scale, big data sets with standard analysis compared to the manual labor of having a person on your surgery table. So in lay fMRI, there's a lot of optimization and manual work needed. Maybe to pick another metaphor here that There's beer and wine, right?
Beer is standardized. There are these multinational brands of beer corporation. You just pump it through Henry Ford assembly line production and it just works. And it always tastes the same and it's reproducible. And by reproducible, I mean homogeneous. You always get the same thing. In wine, there's no international brand of wine corporation, right?
You need to tweak it a bit more, right? You need to consider which country you're from, if that season was very rainy, if you're, you know, Getting your grapes from a North Hill or South side or whatever. And then you get the best wine by, by tweaking it on every case basis. And so, layer fMRI is kind of more the wine of fMRI.
You, you cannot simply run it through standardized pipelines. And sometimes we are kind of branded of saying that. And we are not doing the true science because it's more user dependent But at the end we get kind of consistently higher [01:09:00] reproducibly higher quality by doing that So maybe that's one thing in layer dependent fmi.
Yes, you need to tweak your analysis Maybe even on a given individual person You see that the four and the motor cortex is folded in that person on that specific way So you tweak it slightly different, optimize it different for that person. And then in the analysis, you see, Oh, I have this artifact. So maybe I need to use this additional step which is not kind of tweaking it kind of, so you get, you can get any result that you want.
It's just kind of a craft of getting the most high quality data, which is something fundamentally different that you would need to do both on the acquisition and analysis, which also requires kind of more skill and training. Which is maybe one thing that's still holding us back that layer fMRI is still hard and kind of We are trying our best to kind of teach people how to use it on the scanner online tutorials click along kind of Example data sets on how to run the analysis, but it's more involved for sure
Benjamin James Kuper-Smith: yeah, I mean, it's always going to be difficult to compete with an automated process that you can just, [01:10:00] I mean, this is also always a problem that you can just click a couple of buttons and get your results, but I don't know, maybe you're going to get them all. You know, the people actually want to think more about what they do.
Maybe.
Renzo Huber: It has its advantages for sure. I mean, maybe another metaphor. Let's think of McDonald's burgers, right? They are very homogeneous. They're very reproducible. They always give you the same taste, but if you go to a gourmet chef, then he can kind of know that, okay, we are in fall now, so maybe the tomatoes are not as good, so maybe I use this different spice and to go to high quality stuff you need to have more manual tweaking.
It's not as scalable to, to that degree. Maybe also another aspect is for kind of discovery science. You cannot, like bold is not being invented by running standardized analysis, right? That the big leaps in science are very often kind of not so hypothesis driven. We need to have this balance of exploring and then see what we see and then see how stable it is and so on.
So, sometimes I'm being. Called uh, like when I [01:11:00] um, this is not a
Benjamin James Kuper-Smith: what are you being called? What do they call you?
Renzo Huber: let's say when I'm on the scanner and I say, okay, and this person, I have a more artifact and it just can't get it better. So I'm changing my echo time and changing my temporal resolution slightly bit. And then this person has a thick motor cortex.
I can do it perpendicular. So I optimizing everything for that person that
Benjamin James Kuper-Smith: Sorry, during data collection.
Renzo Huber: exactly in the data collection. So then, and then I'm just. Trying to see what I see I want to tweak the hell out of it for my scanner for my Participant where I know how it's folded for my sequence and for my analysis I'm just seeing how much I can get out of it And then I'm basically describing what I see and some of my high impact papers are coming from that basically, I'm just seeing what I see And this is not really generalizable because it's really optimized for that person.
And if I give those tools to another person it might not work as well. Right. It's however, it allows me to see things that nobody could see before. So I've been called Yeah, you're just on a fishing expedition, right? And then you yes I [01:12:00] am. Right. And I'm the fisherman with the smallest mesh size I can catch fish.
I said, nobody else can. And it's exciting. And then time will tell how reproducible it is. And it's this kind of double sided sword. So I have this advantage of not being automatized. So I'm in this unique seat to see something and to discover stuff, right? And then at the same time, one, one reason why we are privileged in method development is that we always can kind of switch gears between the visionary researcher towards the janitor, like the scanner janitor, the enabler, right? It's really important that you not only show the highest resolution image where you see a new feature and you describe it like the Mirror fingers or like sampling layers or something that makes it to high impact publication, but then we also need to do the dirty work of Kind of making those tools robust making them available Writing manuals doing tutorials doing the teaching so everybody else can use it and that's not really rewarded in there Kind of scientific hiring procedures and so on.
And [01:13:00] however, it's also nice to have this kind of support role often as a technical and person that it's easier to find jobs. For example, you don't need to compete for grants as much because they're always kind of the support jobs as well. Yeah. So it's nice to, to.
Benjamin James Kuper-Smith: If I understand your metaphors correctly, you are both a high end gourmet chef and you also clean your toilets. Is this that roughly? The
Renzo Huber: Exactly. Yes. I think I'm yeah, to some degree I'm, I need to be the garbage man. And so it's a kind of useful job for the community. Imagine there's no garbage man. And. And, but it's a kind of a fine balance to to live in both worlds. And it's I'm a staff scientist here and it's also a kind of it kind of, it's it's hard to bridge the gap.
Yes. Sorry.
Benjamin James Kuper-Smith: Yeah, no, I mean, uh, I think this is a really interesting kind of topic or question because the Yeah, I mean, as you said the breakthroughs don't happen by just, you know, running the thing that everyone's running with the same, you know, You're not going to have a breakthrough study by using, I don't know, psychology questionnaires that [01:14:00] someone else developed and then another questionnaire that you correlate the two with a standard, you know, Like that's not really going to lead to anything particularly insightful, probably. So I completely understand what you're trying, what you're trying to say with the, trying to tweak it, but at the same time, I mean, how do you manage to make it reproducible? Is it just doing it with lots and lots of people and, do you try and separate the exploration from the, you know, basically how do you separate the exploration from the confirmation in this context, if it's, if a lot of the work is so involved, so much of you actually looking at what you're doing and yeah.
Renzo Huber: not sure if I have a solution. It's just kind of a lot of work. Like you, you do something, you do it again, and then. Like for, if you see something new, then you just invite the same person and do it again, test, retest, and see how stable those patterns are. I think there's a lot of non science work in making it kind of usable kind of the janitor work of the scanner.
Like you need to work with the vendors and to work with [01:15:00] them is kind of difficult. They care about different things. And also. Kind of the knowledge on knowing how the scanner works internally is not research. You don't learn about this in academic journals, right? It's kind of inside knowledge and depends on kind of industrial decisions that the vendors made commercially.
And still you need to keep up with that a lot and that it's not kind of open. They need to build your, Kind of, networks with people from the vendors and so on, ultimately, they, they have something like an app store for sequences where I can kind of upload my sequence. And now the kind of delay for my basic sequence that I'm providing is installed in like more than half of the seven Tesla scanners out there, certainly more than 50 or so.
So, and then people do it again, and then it's iterative, right? You're bombarded with a lot of questions. If I get the same question three times, I put it on the blog. Which then they have a huge FAQ for how to do that. And the same for analysis, like it's a lot of effort to kind of do like screen recordings, click through your example dataset, teach people how to do [01:16:00] it.
And then it slowly gets reproducible and other people do the same thing and see the same features across layers. And then usually time will tell. And yeah it's a hard balance and maybe it's something for, The kind of closing questions that you usually have in your podcast where you ask what people wish that I, they would have learned like earlier.
And I think that balance is something I haven't really learned yet. And I wish I would have learned earlier to think more big picture, like in the sense that sometimes I feel I'm investing years and years for building a software for other people to use because they're very thankful for it and use it.
But it, it doesn't um, allows me to be seen as a kind of. Innovative researcher myself. I'm most judged as an enabler or kind of a service person in grant reviews or something, if it just provides software because the incentive structure just doesn't work like that. And we, as scientists, we have a incredible luxury that we Can kind of, I mean, we get government funding, grant funding, taxpayers money [01:17:00] usually.
And you can pick the projects that kind of fit us, what I like to do right now, what I find fun, what fits my skillset. And if it doesn't work out, like in science, sometimes some things doesn't work out, right? We are even encouraged to do risks. And sometimes I wish I would. I would have learned sooner to kind of to, to think big picture and early on to not only be the janitor but sometimes kind of to, to explore more and then rather kind of push the field and show them where there's interesting information to harvest to some degree.
So I think very often the scientific industry and. It's frustrating. It's brutal out there, right? You need to cope with rejections from grants. You need to cope with kind of hateful, unnecessary and destructive reviews. And so very often that's in our kind of mind, like in my emotions are kind of tuned to a way that I want to do studies that are kind of easy to do and that I can defend in front of a reviewer, [01:18:00] which is not the right approach.
I think so. And to answer your question on how you balance, like sometimes I, Pick projects that are kind of useful for the community. They're easy to do, write a software that helps everybody, be the garbage man and kind of forgetting kind of the big picture question, where I actually want to end up in 10 years, where, how I, I have the biggest impact for the field.
So at the end, I think I just need to do both in my discipline And we shouldn't be guided by kind of the frustration of mental energy being put in kind of the low hanging fruits and easy projects which are sometimes kind of the janitor works to be useful to people in your institution or something.
We need to kind of have our own questions to it on the side or do the garbage work on the side. We need to do both.
Benjamin James Kuper-Smith: Yeah. The, I mean, that's the fun thing, right? If it if it kind of works out then in 10 years people will just be running this routinely and you'll be sitting there going like, you have no idea how much [01:19:00] work I put into making this easy to use. People won't even know that they'd have to be grateful for it.
I guess the same way that now you can just use all these fMRI, the standard things. And if you have a kind of new paradigm, a new task, then you're not even aware of, like, how much work went into making it so that you can do it fairly easily. Um, , so in Philae, fMRI, and you in general, what are kind of your some plans?
I mean, what do you think is going to happen? What are, I mean, I guess, resolutions going to get higher in the coming years? That's but yeah, I'm just curious kind of, what do you think I mean, maybe two things one, kind of, what are some fairly obvious things that you think are probably going to happen in the next couple of years?
And then secondly, also what is something you'd really like to see, be like, well, I'm not sure that's, I mean maybe some of the things you'd really like to see will happen, but yeah, basically what will happen and what would you like to happen.
Renzo Huber: Resolution is going to get better. It will have kind of compromises. You will be slower. Hardware is going to [01:20:00] be better. There's this renaissance of new hardware. Like we had multi channel RF coils since 20 years. We had seven Tesla since 20 years and there was little development now with the new gradients and with the higher field strength beyond seven Tesla, there's a new push.
So, things just will be kind of the resolution will grow. Clearly, the layer fMRI will be easier simply because it has to there are more and more people using it and which means there's a lot of mental energy from all different labs with all different expertise and there's no way around it, it will just be easier.
And one very obvious thing that I'm actively working on is kind of whole brain layer fMRI that's kind of robust there are few people trying. to get to what's kind of whole brain. Usually, most people still focus on a given brain area and then look at the different layer connections, feed forward, feedback, input, output, and so on.
It's just kind of ironic that layer FMI is inherently a connectivity tool and You don't really always see, kind of, if there's output, where this output is going to, or [01:21:00] if there's input, where this input is coming from. So ultimately, layer fMRI really will fully develop its promise if we have whole brain.
And to have 200 slices at TRs that neuroscientists like, of very few seconds only, maybe three, That's still hard and so I think in the next few years, maybe four years, we have a nice connectome whole brain functional connectivity across the layers as a kind of baseline data sets available.
I think that's something that, that I'm working on and that, that is just going to happen. Other things like it can extrapolate a bit from the changes that I currently see on my blog layer for my. com slash papers. I track all the papers out there and then some trends. So it in the early days there was, it was really.
Kind of acquisition hardware focus. Then there was this golden age of understanding the contrast, a lot of stuff in animal models. Then it moved towards kind of more acquisition methods, sequences, and until kind of the neuroscientists really jumped on. And since then, it's exponentially growing.
So I think the field will [01:22:00] shift more. Away from people like me, methods developers more to application focused people, which sometimes I see kind of weird quality papers out there. So I hope that we're going to do it right. And this shift towards applications and these applications are moving away from the early sensory areas, more to a visual auditory to more kind of higher cognitive areas like the DLPFC or.
Or more network kind of ideas, hippocampus, I think it's just a thing that's going to happen. Maybe also kind of working on it right now with Khasar Ahmadi and visiting from Bochum, looking at kind of memory. So that kind of inevitable, a change will be kind of to apply it on the conventional tasks that people do in cognitive neuroimaging, maybe decision making, they'll just do the same old task with now layer dependent fMRI.
Other things where that's, I'm still frustrated that it hasn't really happened is kind of more clinical applications because there are maybe a handful of review papers that talk about the promise of layer dependent fMRI for neurodegenerative diseases that their [01:23:00] specific motor neurons are like affected in a motor system that are.
degenerated. And then you can look at that with layer dependent fMRI, psychosis, schizophrenia also Alzheimer's with respect to the different layers in the endorhinal cortex. There are a few studies that kind of going there, but it's still hard. We did some study here at NIH with focal hand dystonia patient, which was a huge learning curve to work with patients and they move and they have different needs and they're not as strained.
And it's still, it was Like not as hard as I expected, I guess, to look at focal hand dystonia, which are patients. It's a quite common neurological disorder where people kind of use their fingers in everyday life, but for some tasks they just cramp like writers cramp or musicians cramp. And then there are hypotheses about that with respect to microcircuitry, namely that it's a lack of surrounding inhibition of the individual finger, which seems to be what we are seeing as well.
So I think more clinical applications will come in. As more cognitive neuroscientists will use it it will be more scalable. There will be larger ends and those kind of [01:24:00] things. And then I'm not really sure how we will Merge in with the neuroimaging community of kind of user independent, large and scalable data sets where you're not allowed to touch the data.
And so, so, so there will be something that I hope that the needs of layer dependent fMRI will be met somehow to have remaining flexibility in acquisition and analysis without being, you know, Branded as a being on a fishing expedition or something like that. And then I guess I see a bit of the open science movement in layer dependent fMRI since 2019 I think the more and more people at least share the data because in the field of method development people are really protective of their intellectual properties because maybe the Closeness with the vendors and they want to have patterns and become rich.
If they develop their sequence, it's not standard that you will share your data and still for code sharing of scanner programming code, there's nothing because the vendors, they don't want you to, right? You need to sign something that if you want to develop something with a vendor that you're not going to [01:25:00] post that code.
And then there are kind of new developments that, that I hope are happening. So hopefully the biggest thing that I want to see is that it's getting easier specifically also with respect to. Acquisition methods like, like blood volume sensitive methods that are not the kind of standard bold methods that are mostly sensitive to large training veins.
And maybe the biggest challenge in the near future will be that. LayerFMRI is still a method of opportunity, a method with potential. It hasn't really lost its innocence yet, that it needs to be useful. It needs to give grant money and fund scanners and so on. So as soon as it's really established, I think then people want to use it and have it turnkey.
So I think there will be a lot of criticism and then kind of pointing fingers, oh, what's this artifact? I can work with a method that sometimes works and I have a dropout rate of 10 percent or something. Right now, we are still in that. domain that, that I don't care if I have a dropout rate of 10 percent and if there's too much motion, which sometimes happens, [01:26:00] it's a problem more for layer dependent FMI.
And I can just ignore it so far. But at some point I will not be able to, because then people will complain and say, well, I have my patient flown in now this needs to work, you know, and we are not at that kind of robust level just yet.
Benjamin James Kuper-Smith: Yeah, I guess if you, I mean, hypothetical example, but if, yeah, he's had somewhat psychosis and you, I don't know, wanted to test whether it was this or that kind of psychosis and that could be done with it. Yeah, they, I mean, I guess if there's no alternative, maybe they'll still try. That might not work, but uh, Okay.
Yeah. So it's a lot of streamlining seems to be right. Like a lot of taking the thing that already kind of exists in some contexts and just like making it work more, more. easily more as I said like with fewer Errors or problems or whatever? Okay I yeah, so at the end of each interview I asked my guests the same three questions. I guess one of them we've kind of [01:27:00] already answered. But to start with the first one, what's a book or paper you think what people should read?
Can be old, new famous, unknown. Yeah, just some sort of reading recommendation.
Renzo Huber: Yeah, it depends. There are a lot of papers, as I said, on the block. All 60 are listed there. I gave a recent.
Benjamin James Kuper-Smith: Sorry, just as a, I mean, people usually often recommend more than one, but I'm gonna limit it to less than 250.
Renzo Huber: So, There are a lot of metrics on the website where I'm kind of summarizing the most cited paper, maybe good papers for people that want to start in the field.
And recently for an education workshop, we asked all the faculty of all the layers from my leaders in the field what they recommend. And I think one of the introductory papers for acquisition methods, not so much brain focus, but for acquisition and the scanners. And I guess the first half of this podcast, there's a paper from Saskia Bollmann.
in the progress in your biology journal [01:28:00] 2020, which is called new acquisition techniques and their prospects for the achievable resolution in fMRI. And I think that's really written for the, without, for the lay audience, without too many kind of specific terms. The most cited paper is one from Jonathan Polimini with the title.
Laminar analysis of seven Tesla bold using an imposed spatial activation pattern in human V1, which is kind of old. It's from 2010 and it's just kind of lifting the curtain of kind of what layer from I could do. I basically showed that you can do it and their signal, but it didn't really tell you a lot on kind of, kind of the entire landscape that would evolve in the decades to come.
I think that there are Kind of there's a YouTube channel layer fMRI, which has a lot of kind of the overarching kind of keynote lectures of people that kind of give an all good overview of fMRI. Actually one from Peter Panettini that I would recommend. that I found kind of [01:29:00] inspiring also for finding new questions in layer dependent fMRI.
But I think there's not a real one paper that I think everybody would read. The book that I almost give every student that I found that it was very inspiring for me was Surely You Are Choking, Mr. Feynman, that made me study physics. And it's just about the joy of finding things out about scientific integrity.
And it's kind of entertaining. It's still now when sometimes I'm dealing with rejections, I read it and remember kind of. And that I'm in the field where I want to be, even though it's kind of old and outdated. And he has some controversial opinions about kind of gender and questions where he might have been progressive in those days, but it's really outdated nowadays, but there's a lot of cool stuff in there that makes you thrive for science.
Benjamin James Kuper-Smith: Okay, yeah, it's funny, the It's funny, I had a very brief period in which I wanted to be a physicist. I mean, I was, I mean, basically I wanted to do music for most of my life, and then at some point I was like, I actually don't know whether I actually want to do that [01:30:00] as a job. And anyway, so I randomly, you know, in a bookstore came across That book by Feynman.
Obviously in the slightly more awkward phrasing in the German translation. Which is just a terrible way to write it. But yeah, I was like, oh, this is so cool. This is what I want to do. And then I just had the worst physics teachers next year. And that was kind of the end of that. And since then, I've never been interested in physics again.
Um, But yeah, it's Haven't read it since then though. So yeah, no idea, but yeah, I guess it's, it seems to be a book that gets people into physics. Yeah. Yeah. I mean, something you wish you'd learned sooner. Do you want to expand on something said earlier or should I just link back to it or?
Renzo Huber: No, I think like the balance of being the janitor and the visionary scientist is It's something I wish I would have learned a bit sooner. I'm still kind of circling back and forth. One aspect that I definitely wish I would have learned sooner is kind of how many skills it involves to be a [01:31:00] kind of a scientist in general, a researcher.
Like I studied physics because it's. It's contained, you have your ground truth and actually I failed English in school, right? I dropped out for a year and I was very bad at reading and writing and so I did physics because I didn't need English and I didn't need reading and writing and it's ironic that now I spend most of my day reading and writing English.
So, something that I wish I would have learned sooner is that kind of as a scientist you just you cannot have this attitude that, well, I'm focusing on the science. I'm not publishing you at the end, need to learn how to advertise yourself, how to build your brand and those kinds of things specifically for incoming postdocs, I think that's something you will need to rely on and maybe it's awkward and I kind of, I did physics because I can be in the basement, socially awkward and do my stuff and do it well, but at the end.
You need to be learned to be a communicator and you need to learn that science is done by people. There, there might be a ground truth down there, but you need to advertise it and you need to invest in this skill. And like in the first three years of my PhD, I didn't [01:32:00] publish, I didn't see the point despite people recommending it to me because I, I built my sequences, people used it and that was my reward, right?
I was, I saw that I was useful and to the community, but at the end, publishing is just something you need to do. It's like paying your taxes, even though you have other plans. In your life and your long term, you just publish and you publish whatever and you, it's just something you do and then kind of, there's all different aspects in being a researcher and there's no way around it.
That's something I, I recommend people kind of not to do. Neglect and it's something I wish I would have learned sooner. And I'm saying this also because I see it in other people that say, yeah, I get my talks at conferences. I get my recognitions. I don't need to publish this. Do I? No, you do. And you need to kind of build your network and to have the future boss.
And you probably know your future boss already. So be kind to people and be a people person, even though it's not your strength. And maybe that's even the reason why you went into a nerd field.
Benjamin James Kuper-Smith: So I'm just trying to imagine that conversation [01:33:00] between you and your supervisor. They're saying, you should publish this, and you just sit like, but why? Because that's how the system works. Yeah yeah. Yeah, I guess again you've, I guess, well, I was gonna, you know, final question is advice for PhD students, postdocs, people in that kind of roughly that area.
Is it kind of linked to what you just said, or do you have some completely other advice?
Renzo Huber: No, I think it's. Yeah. It's that you need to build your things. Yeah. Maybe one thing I also learned, and maybe I'm overdoing it, is to accept that you cannot be perfect especially for PhD theses, as you, and you can write your thesis for the rest of your life. At some point, you just need to make a cut and say, this is it.
And you can always kind of optimize it further and further. And maybe I'm a very bad writer and I just accepted it. And if you read my blog, it's full of spelling mistakes and whatever. And I'm fine with it. And if in my papers, sometimes there are things that are kind of weird and I don't really have a perfect answer.
I [01:34:00] still make it into a paper and I acknowledge that this is unsure and I don't know. But ultimately I think. That the acknowledgement not to be perfect or mind the gap or whatever, like the UK Metro says, is this fine? Acknowledge that you have this gap and that's something I learned and maybe overdoing it right now, but that's something I would recommend because that's something I think that people are really literally self sabotaging themselves, like themselves, that they are trying to be perfect.
And there's still this question. I'm not sure about this. It's fine. As long as you're transparent, kind of. It's okay not to be perfect.
Benjamin James Kuper-Smith: Yeah, it's funny. I remember when I started my PhD, I wanted to really have my thesis be like this thing. And then as I was going through my PhD, when I started writing, I was like, oh, this just doesn't matter You know because I guess the metric were counted by his papers and these kind of things and so I was like Yeah, genuinely.
No one's gonna I mean it has to be like a certain standard obviously, so I don't fail [01:35:00] and uh, so I get like a decent grade for this but I mean, I think actually one thing that's quite nice for us also You is that and I'm assuming it's similar in the field you worked in, is that the thesis in itself, there's very little you can actually do, unless you like actually make it basically into, I mean, because the unit of measurement in a way is a paper, right?
And through your PhD, you kind of made those. And surely there's some unproper stuff and whatever, but like at the end of the day, you know, this isn't I don't know. Well it's like in other fields but you know I imagine maybe if in the humanities or whatever then that's, the writing of the thesis itself can be a lot more of a thing but then it seemed to be someone's like oh this is like I've done the work now it's just summarizing it basically for people to understand.
Renzo Huber: I struggled with kind of how much you include, right? You always have this other experiment that didn't make it to a paper. Do you include it now? And how do you packet it? Actually, I would like to finish acquire a few more people. And then I also have this other [01:36:00] open issue solved. But yeah, no, at some point you need to kind of, yeah, but it's just something you do.
And yeah, along those lines as I said, it's more and more important to kind of build your friends, build your networks, because at the end, science is brutal and frustrating, especially in the science industry, how it works. So surround yourself. with nice people that, that you like because science is done by people.
Benjamin James Kuper-Smith: Okay, well that's a nice final word. So, thank you very much. And we managed to do this, the audience doesn't know this, I mention now, without the drilling starting again in your building. We managed to get through the entire episode. Apart from the first five minutes or whatever. Don't know whether that will be audible, but we made it.
Renzo Huber: Yeah. Thanks for having me. And then I really like your podcast. It's a nice service that you do for the community and congratulations on kind of more than a hundred episodes already. It's really a lot of work and I appreciate it. Thanks.