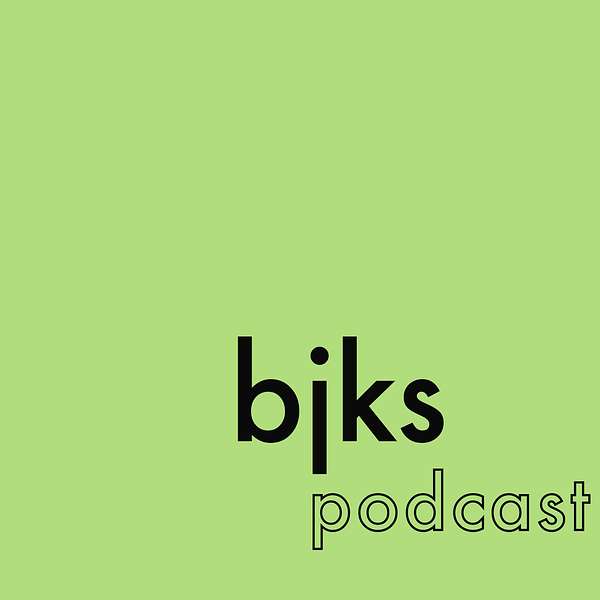
BJKS Podcast
A podcast about neuroscience, psychology, and anything vaguely related. Long-form interviews with people whose work I find interesting.
BJKS Podcast
100. Tom Chivers: Thomas Bayes, Bayesian statistics, and science journalism
Tom Chivers is a journalist who writes a lot about science and applied statistics. We talk about his new book on Bayesian statistics, the biography of Thomas Bayes, the history of probability theory, how Bayes can help with the replication crisis, how Tom became a journalist, and much more.
BJKS Podcast is a podcast about neuroscience, psychology, and anything vaguely related, hosted by Benjamin James Kuper-Smith.
Support the show: https://geni.us/bjks-patreon
Timestamps
0:00:00: Tom's book about Bayes & Bayesian statistics relates to many of my previous episodes and much of my own research
0:03:12: A brief biography of Thomas Bayes (about whom very little is known)
0:11:00: The history of probability theory
0:36:23: Bayesian songs
0:43:17: Bayes & the replication crisis
0:57:27: How Tom got into science journalism
1:08:32: A book or paper more people should read
1:10:05: Something Tom wishes he'd learnt sooner
1:14:36: Advice for PhD students/postdocs/people in a transition period
Podcast links
- Website: https://geni.us/bjks-pod
- Twitter: https://geni.us/bjks-pod-twt
Tom's links
- Website: https://geni.us/chivers-web
- Twitter: https://geni.us/chivers-twt
- Podcast: https://geni.us/chivers-pod
Ben's links
- Website: https://geni.us/bjks-web
- Google Scholar: https://geni.us/bjks-scholar
- Twitter: https://geni.us/bjks-twt
References and links
Episode with Stuart Ritchie: https://geni.us/bjks-ritchie
Scott Alexander: https://www.astralcodexten.com/
Bayes (1731). Divine benevolence, or an attempt to prove that the principal end of the divine providence and government is the happiness of his creatures. Being an answer to a pamphlet entitled Divine Rectitude or an inquiry concerning the moral perfections of the deity with a refutation of the notions therein advanced concerning beauty and order, the reason of punishment and the necessity of a state of trial antecedent to perfect happiness.
Bayes (1763). An essay towards solving a problem in the doctrine of chances. Philosophical transactions of the Royal Society of London.
Bellhouse (2004). The Reverend Thomas Bayes, FRS: a biography to celebrate the tercentenary of his birth. Project Euclid.
Bem (2011). Feeling the future: experimental evidence for anomalous retroactive influences on cognition and affect. Journal of personality and social psychology.
Chivers (2024). Everything is Predictable: How Bayesian Statistics Explain Our World.
Chivers & Chivers (2021). How to read numbers: A guide to statistics in the news (and knowing when to trust them).
Chivers (2019). The Rationalist's Guide to the Galaxy: Superintelligent AI and the Geeks Who Are Trying to Save Humanity's Future.
Clarke [not Black, as Tom said] (2020). Piranesi.
Goldacre (2009). Bad science.
Goldacre (2014). Bad pharma: how drug companies mislead doctors and harm patients.
Simmons, Nelson & Simonsohn (2011). False-positive psychology: Undisclosed flexibility in data collection and analysis allows presenting anything as significant. Psychological Science.
[This is an automated transcript that contains many errors]
Benjamin James Kuper-Smith: Yeah, I mean one, one thing I found kind of funny about your book is that, , it was published in March or something like that, right, like a few months ago
Tom Chivers: April, I think, in the UK in May. Yeah, April in the UK, May in the US.
Benjamin James Kuper-Smith: so yeah, I saw it like sometime last year then that, I think you announced said on Twitter that you had this book on base coming out or something like that.
And I was like, Oh, that's cool. Really looking forward to it. And then once I read it, I was like, Oh, wait a minute, this is actually way more relevant to like what I'm specifically doing and the research I'm doing than I thought, because I thought it was going to be more of a, What your book is in the beginning, I guess, like the first 20, 30%, like a little bit about the history, probability, Bayes, his life, the little we know about him, but then I was like, you were basically hitting through recurring themes of my podcast in the remaining.
Tom Chivers: well, the, what I think of it as is, I don't know, this is going to sound grandiose, but I I was hoping to help people sort of build a Bayesian way of looking at the world, not just, here's a fun fact about probability and here's some history of the history of probability theory and all sorts of stuff, as interesting as those things are, right?
But the point was, [00:01:00] I thought that this sort of underlying sort of structure to a lot of, to how people learn and how we make predictions in the world and how intelligence works and all these different things and how, and this sort of structure underlying it all can, to a great extent, be described by Bayes and, that was what I wanted to get across.
Not, isn't it fun that we can, that, that the 99% accurate test where will sometimes give you the wrong answer, even if, because it's about the base rates, which is like the classic, the ba, the Bayesian basic idea that everyone learns about it, breast cancer screening and all that sort of stuff.
But to do that sort of. Wide angle. Here is a kind of different way of looking at the world. And here's a thing that might explain huge amounts about how our brains work and describe would be better than explain actually, but describe loads of stuff about how our brain work or brains work and how predictions of the future work and all these other things that I just said a second ago to do that, you have to build up through.
what it is that probability [00:02:00] does, why Bayes is a different perspective to frequentist, why, I assume your readers, your listeners would be confident with these terms, but exactly. I think the beginning of the book is just like getting people who don't know about this stuff, familiar with the ideas and then familiar with what probability has been doing for the last few hundred years and how, what we want from it.
And then from there, you can widen the lens and say, look, this. This idea that seems really simple and seems niche and it's just really talking about, you know how best we work out the likelihood that you've I don't know that you've got got prostate cancer if you get a positive result on a prostate Antigen test or something like that once you've started from there You can expand it and you can start saying well actually this is also the same Structure can be applied to science.
It can be applied to super forecasting I don't know it can be applied to the fact that it applies to Formal logic is my favorite bit and even that it and then that it's how our brains seem to work I loved it But yes, it is meant to be a sort of a way of looking at the world Rather than [00:03:00] just here's some fun stuff about statistics and probability
Benjamin James Kuper-Smith: Yeah, and yeah, I'm assuming we're gonna hit on. A lot of those parts, replication crisis, decision making, the brain itself throughout the conversation. I wanted to start first by going a little bit over the history of, Thomas Bayes himself, the man. The little that we know about him, and then probability theory.
So. I mean, it's I think the Wikipedia, I think I counted the wiki, the words count on Wikipedia of his biography, I think it's something like 400 words or something like that. Um, it's, It's, well, but there's also not that much that we actually know about him. I mean, it starts with when he was born.
Right. And where I think also so can you maybe provide a brief sketch of like, you know, who is the person.
Tom Chivers: sure okay, so, I mean You're right, we don't know very much about him. We know a bit about his family history. He came from a well to do family of , his family made its, made, got rich. I think mainly selling like cutlery and stuff. Like an [00:04:00] iron, iron works and things in the um, sort of century or so before his birth.
His family was also so they were well to do middle class, upper middle class which Britain was developing at the time, in the run up to the industrial revolution, I guess. And they were also non conformist. I mean, this is crucial, right? A lot of his family were preachers in non conformist churches.
In the, England had a civil war in the early 17th century, and in that time the Religious freedom had been somewhat opened up. You didn't, you no longer had to just use the Church of England prayer books. There was a little bit more freedom of to dissent from the established religion.
But then after the Civil War and the re establishment of the monarchy, that was closed down a lot. So there were a lot of people who had tasted a bit of religious freedom, and then it was taken away from them. And these people who had, People who wanted to become these sort of preachers weren't able to study in English universities, they often weren't, their churches weren't sanctioned by the CofE, that sort of stuff.
So a lot of people, I mean, this is why people fled to the US, Puritans fleeing to the US and things, but others stuck around and just preached [00:05:00] in, They weren't massively persecuted, but they weren't, a lot of their they also weren't hugely allowed to practice what they wanted to.
So they were forced to go to Edinburgh or to the Netherlands to study and all that sort of stuff. So, what that meant was their, a lot of their lives isn't recorded, because most of the records we have from that period are from C of E, Church of England Records. So we don't actually know what year Thomas Bayes was born and we know what year he died.
And we know how old he was when he died. And I'm gonna say I think he was, I think it was 1759 and he was 58? Or maybe it was 1758. Yeah, I think it was 1758 and he was, anyway. Point is, he was He was, according to his biographer, with probability 0. 8, born in 1700. It might have been 1701, there's the, we know, he died in April.
And yeah, so that, so you can work that out, but even those sort of basic biographical details are not known. All right. We don't know much about his life. We've, there's like the old snippet here and there's I've, he's got a rather longer. Biography by a guy called David Bellhouse, U. S.
statistician, historian of statistics, and he [00:06:00] did a bit of a better job than the Wikipedia article seems to have done of digging out some things. So we know, he, we can, there are letters to his old tutor from Edinburgh, dating the time he was there, and there's letters between him and this guy, Lord Stanhope, who was a sort of very nerdy, Lord, obviously it was a rich guy who gathered a sort of coterie of scientists around him and funded and backed their research.
I think it was Joseph Priestley who was a discoverer of oxygen. I'm doing this off the top of my head, but I think that's right. A few others who he would like fund their research and back them up. David Bellhouse said it was a bit like rich guys might back a sports team. Stan Hope would back. Aspiring scientists to, and um,
Benjamin James Kuper-Smith: Or the way you had like court musicians and
Tom Chivers: Yeah. Yeah, exactly. Exactly that. Or it's called astronomers and things, this wasn't the sort of, it was a few hundred years after your sort of Copernicus and people and Tycho Brahe, but that was that, it's not a dissimilar idea. You're right. Hobbyist [00:07:00] scientists getting backed by very rich people. And, Baze was himself quite well off. He had an undemanding job and family money. I don't, so anyway, so they, he so we know a bit about that. Tunbridge Wells and was a preacher there. We know he was a member of the Royal society. We know that was because he'd published a.
Nothing to do with his work on Bayes theorem, it was to do with a work he'd published defending Newton's calc some work of Newton's, I think from Bishop Berkeley Bishop Berkeley, yeah, Fluxion, exactly. So which Bishop Berkeley suggested that Newton had made a divide by zero error, basically, but and Bayes tightened up Newton's ideas and tried to defend it a bit more.
He also wrote this is very dear to my heart because I did I did this sort of this at university a bit, I did, he did a work of what we'd now call theodicy. So the classic bit of theological philosophy where people say, if God is perfect, , if he's all knowing, he's all powerful and he's all loving, then how come there's pain in the world?
And Bayes did a big piece arguing that, well, these things always get incredibly bogged [00:08:00] down in sort of angels dancing on the head of a pin and stuff. But he did, he, he did one of them and that, that got a certain amount of attention and was quite controversial, I think.
Um,
Benjamin James Kuper-Smith: Title also,
Tom Chivers: yeah. Oh God, it was incredible. Yeah. I can't wait to I'll see if I can find it.
Benjamin James Kuper-Smith: Well, I've got it
Tom Chivers: Oh yeah, I've got it here. Yes. Oh yeah. Brilliant.
Benjamin James Kuper-Smith: Divine benevolence or an attempt to prove that the principal end of the divine providence and government is the happiness of his creatures.
Tom Chivers: Yes. Yeah, I've only even said half of it. Being an answer to a pamphlet entitled Divine Rectitude or an inquiry concerning the moral perfections of the deity with a refutation of the notions therein advanced concerning beauty and order, the reason of punishment and the necessity of a state of trial antecedent to perfect happiness.
I mean, like, I've got this really smart ass joke in there, because his name was not on the author page, although to be fair, there would hardly have been room. Like,
Benjamin James Kuper-Smith: Exactly.
Tom Chivers: Yeah, he's spent more time writing the title than the book itself, it sounds like. Um,
Benjamin James Kuper-Smith: an abstract than a title. Yeah.
Tom Chivers: Yeah, exactly. But Yeah, that was, those were, quite, they, I don't think they were [00:09:00] unheard of in his lifetime, the smart set passed these things off, he was a reviewer of a paper by a guy called Thomas Simpson who tried to work, do some work into how to use statistics for inference, which is obviously what we want it for now, when we do probability, we want it to do, to answer questions about it.
If we take a measurement of where a planet is in the sky from a telescope, we want to estimate where the planet is in the sky and use statistics and probability to tell us things about the world. And there was a guy, Thomas Simpson, another English mathematician who'd started making some progress towards that and Bayes reviewed his paper.
So he was obviously involved in the intellectual life of the day. But. His actual work on Bayes Theorem, which had an essay towards solving a problem in the doctrine of chances, I believe it was called. I can never remember that. I should know it off the top of my head. That was never published in his lifetime.
He, well, Bellhouse, his biographer, thinks he probably didn't think that much of it because he left his papers to um, a [00:10:00] friend called Richard Price, yep, who's a fascinating guy in his own right. He left his papers to him, and he could have, he seemed to have written a will a few months before he died, so he must have known he was not well but he did, or he likely knew that he wasn't well, and then he he never made any effort to publish it, so presumably he didn't think that much of it, but yes, it was this that we now remember him for, the Richard Price published via the Royal Society after his death.
Benjamin James Kuper-Smith: It's kind of, I found it, I mean, as you mentioned, Price is maybe you can say a little bit about him, but it's interesting to me that, I mean, I guess it was the kind of thing where he got, did he just get the papers or other stuff also? But I guess he must've gone through it and went like, hang on, this is kind of interesting.
Tom Chivers: exactly. So \ he left Richard Price a hundred pounds in his will, which I assume was quite a lot of money at the time. And he left him all his papers or at least a large chunk of his papers. I don't know. I don't actually know if it was literally all of them. And then Richard Price some a year or so later or a couple of years later, he's obviously, yeah, like you say, he's just must've been through them.
Hang on. Go Hang on. [00:11:00] This is, because degree, what Bayes paper did was what a lot of scientists, or a lot of statisticians, or I should say mathematicians, or natural philosophers, yeah, exactly, at the time um, had been wanting to do, right? Maybe I'm segwaying too early into sort of history of the history of probability here, but the history of probability before that, Going back to 16th century, Blaise Pascal, Pierre de Fermat, what probability had done up to there, it was telling you how likely you are to see some result given some, given some hypothesis.
The classic thing was you've got A gambling game to massively oversimplify. Let's go for it. So it's the first to three points. There's one person's got two points for one person's got one. It's a flipping coin thing. So it's, everyone knows that, it's equally likely to go each round is equally likely to go either way.
How, what's the fairest way to split the points. And so that, that was, that's,
Benjamin James Kuper-Smith: If you have to stop
Tom Chivers: yeah. If you have to, if you have to stop the game early, exactly. Yeah. And that's [00:12:00] interesting, and that's the probability you do at school. That's, how likely am I to see three sixes on three rolls of a fair dice?
But it is telling you how likely am I to see some result given a hypothesis about the world, that the dice are fair, that the coin is not rigged, that all that sort of stuff. And that's what probability had been, there was a huge insight by Fermat and Pascal to be able to work that out.
And then from there, people like Jacob Bernoulli in particular sort of took that on and tried to work out, I think, the law of large numbers, what can we say about these different things? But what A lot of what people wanted to do, and what people didn't realise, Bernoulli I don't think realised didn't, what he hadn't worked out was that likely you are to see some result given some state of the world is not the same as how likely some state of the world is to be true given some, result, so given that the three dice came up three sixes, you'd only see that one time in 216.
What can that tell us about how likely the [00:13:00] dice are to be loaded? It's a totally different question. And people didn't realize that for a long time.
Benjamin James Kuper-Smith: I think the best example I think you gave was of, was it what's the probability that the Pope is human or that a human is the Pope?
Tom Chivers: Yeah, exactly. Yeah. Yeah, exactly. It was a one in eight billion chance that a given human is the Pope. That is not the same as there's a one in eight billion chance that the Pope is human. Yeah, exactly. Exactly. So, I Bernoulli had been trying to do something like he wanted to, given that you draw this many balls out of an urn and three of them are white and five of them are black or whatever.
what can we say about the contents of the urn? And he didn't realize that the these two questions were different. And then people like Thomas Simpson were trying to do we've got these different measurements using a telescope of some planet in the sky and they disagree by this much.
How can we average out the the errors to make an estimate of where it truly is? That was Bayes who reviewed that paper and said, no, Be aware of measurement error, basically. But Simpson made the, Simpson had made the astute point, you should average all your results, not just using the extreme the two most extreme, which is what people did before.
But anyway, [00:14:00] these are these were all attempts to start using statistics and probability to say things about the world. And it was Bayes who had the insight that you need to have prior probabilities, that you need to bring in stuff you knew in the past. You have to use all the information available to you, basically, to do that.
And it was it's interesting to me that he didn't realize, or he seemingly didn't realize what a massive deal that was. Because he was involved in all this prob, he was so involved in the thinking about probability. He'd obviously read Abraham de Moivre's book about normal distributions, all this sort of stuff.
And he'd, he was, he'd been in conversation with Simpson, so he must have known about this effort to work out how, how to make inferential statements as opposed to just ones about what we'd expect to see. But it didn't, he didn't make the leap and it was Price who, like we, we, like we hinted at, he's fascinating.
He's another non conformist preacher who was, like, friends with like half of the founding fathers of the American Revolution. Like, it's such a cool, it's amazing sort of insight into how small the intellectual world was of the day, I think. [00:15:00] But he's just, he's exchanging letters with Benjamin Franklin and Thomas Jefferson.
He wrote a pamphlet which was credited with inspiring a lot of the work of, inspiring the American Declaration of Independence. And it's funny, you don't really hear his name that much these days. He's, or at least I didn't much before, apart from as the guy who published Bayes paper.
Benjamin James Kuper-Smith: I didn't know about them either.
Tom Chivers: No, exactly. It's I found that really interesting. Oh, yeah, so so it's fascinating to me how Bayes didn't make this connection that this oh, wow that i've answered Such a huge question here or if he didn't make a big song and dance of it And then he just died and it was left to price to work it out
Benjamin James Kuper-Smith: Two interesting things I find there. One is that, I mean, I think in general, it's often not entirely clear to people creating something how important what they're doing is going to be. I think, that stuff often only, takes a few decades or sometimes centuries for even the world to recognize actually what's going on there, how useful something is.
But, I mean, what I also found kind of interesting about, The history of probability theory is that I mean, so I don't I think I've, [00:16:00] I might have read something a little bit about it, but pretty much most of what I know is from your book. And it seems to me that people weren't thinking about probability, at least in a formal way, Until really late.
I mean like for some stuff like calculus, you can kind of understand why it might take a little bit but probability I guess is something that we use so much in everyday life today the way we talk about events and that kind of stuff and I want I don't know whether do you know Were people just were they thinking about it, but just they hadn't formalized it or was it? I don't know
Tom Chivers: They had they were thinking about it, before Fermat and Pascal, people have been trying to work out things like, how come, Oh, what was the classic thing? It was, how How come the chance of seeing 1 6 on a 1 6 on four rolls of a dice is, or four rolls of a die, I should say, on four dice, is different from seeing a double six on 24 rolls of, a pair of dice, like that could people say, well, that's the same sort of relations to each other.
Surely it should be the same thing, but actually [00:17:00] they're very different and people were puzzled about why this was different. That was it's called I can't remember. I don't think it was a particular name for it. But yeah, there was this guy Cardano who was trying to work out those particular problems years before and people were getting What seem to us now like comically stupid errors.
Like A guy who wrote some groundbreaking mathematical textbooks. Giacomo Cardano, I think his name was. Girolamo, sorry, Cardano. And he, he was asking about what's the odds of rolling a six on a, on four rolls of a die.
And he thought it would be four times the chance of rolling a six on one die. So it'd be four and six. But that's, even if I find that baffling because even thinking about it for a second, you, well, hang on, wouldn't that mean it would be certain to see it on six rolls of a die? But that's obviously not true.
That, that can't be true. So,
Benjamin James Kuper-Smith: And from experience he must have known that.
Tom Chivers: Yeah, it's fascinating to me. But yeah, it does suggest that there wasn't just like some [00:18:00] basic breakthroughs, some sort of real sort of perspective shifts needed to happen before people could make this work. And the fact that it just wasn't, there in people's heads.
And Pascal and Fermat did manage those breakthroughs. then, yeah, there was, It was still a long way to go. There was still and that, that, that was only what I was saying a minute ago, that was only working things out that would help you if you're gambling or, like it's the sort of insight that lets you work out the chance of seeing like a full house in poker, given that you've just drawn an ace and a jack, or you're just drawn a pair or something like that, stuff that's interesting if you're in a casino, but It's not inherently useful if you want to do inferential sort of science about the world, which I'm sure from a fairly early point.
Must have been what people wanted, you know that There are people at that point just you know, it's the renaissance People are just getting interested in science. They're starting to do these measurements of the world They're seeing This particular light in the sky has shifted this far.
Oh, well, and we're just coming to grips with I guess, you know the just [00:19:00] Heliocentric model of the solar system. We're trying to work out all these things, and You know At that point it must have been really useful to start doing statistics and probability on how likely some, where things are to be in different places and all that.
But it just, the conceptual sort of shifts, the conceptual sort of insights hadn't happened by then and it was really being pieced together for over, over centuries, you know. Mm
Benjamin James Kuper-Smith: is that so I, yeah, this is also one of those things that I've read somewhere once and it was a while ago, so I don't quite want this, but that I think it took pretty long. I mean, I think like some aspects of mathematics were well developed, like geometry and that kind of stuff.
I think that a lot of that was developed fairly, well Euclidean geometry at least, was developed fairly early, but that I once saw something about the history of mathematics where they showed how mathematics was done in the middle ages and they hadn't figured out the whole way of having equations for stuff like they were writing everything out in sentences and so you I wonder like whether maybe like you know I guess [00:20:00] Newton was a bit before that I don't know exactly when this happened but I mean that must have had something to do with them getting a lot better a lot of these kind of abstract quantitative
Tom Chivers: It is. I mean, you can see it in the, I looked at some of the original paper, the original papers, I found scans of them online, and they are they write things out longhand an awful lot, which might, you know, and there's old Pascal and Furman people saying things like God, I would say the.
He goes, if I win, I shall gain all, that is 64. If I lose, 48 will legitimately belong to me. He's writing out, where is write whole paragraphs of text to explain something that a modern mathematician would do in probably eight symbols. Just I'll do a larger than and then a little dot over some number or something.
We now all understand what this means, can we move on? It's actually really useful for me, as a non mathematician, Because I can follow the concepts when they're written out, but I don't feel like I see a Sigma, I see like a Sigma sign and my brain freezes up, Oh God. But I can
Benjamin James Kuper-Smith: that's going to be a new application for AI translating current mathematics [00:21:00] into middle aged writing.
Tom Chivers: Yeah, yes, exactly. Make, write this out. Blaise Pascal would not be able to understand it, but not in French not in like early modern French.
Benjamin James Kuper-Smith: was about to say, it's not even French. Yeah. Um, so maybe to go back to continuing the history. So Price publishes this. I think, if I remember correctly, you also said with a lot of additional stuff that Price added of examples, I believe, or
Tom Chivers: Yeah, absolutely. So. Bayes original stuff was pure theoretical maths. It was just, here's, he imagined someone the specific example was he was someone rolls a ball, a white ball on a table, and he doesn't say a billiards table or a pool table because he's a, very serious and godly man a non conformist preacher, but he obviously means a billiards table, the ball bounces up and down and lands somewhere at random. You take the ball off, but draw a line on the table somewhere at the point where the ball ended up, across the table. And then you continue, then you throw more balls onto the table, rolling them up and down. And the person you, the, or rather someone does this, and they tell you about it.
They [00:22:00] only tell you whether the balls land left or right of the line. And your job is to do your best guess with the information given to you where the line is, and therefore the probability that the next ball will land Either left or right of it. And that was, that's the fundamental.
But Bayes big insight was if they throw three, five balls and three of them end up left and two of them end up right, your best guess is not, the white line is three fifths of the line at the table, but because you have to take into account your prior, prior decisions.
Probability, which is that the ball could be anywhere. You have no prior information about where it could be, so you have to estimate, assume that it could be randomly anywhere. And so then, the way he did that was, you say, imagine, basically, that there's an extra ball on either side of the line.
So, instead of saying your chance that the next one will land right there. Left is three out of five. You say it's four out of seven. So the number that you, the number that you had plus one, the number left plus one divided by the total number plus two, don't worry too much if the people aren't following that.
But it's just saying you have to add the, you have to add a sort of bit of information to say that I that I have some prior [00:23:00] information about this. And it was, you know, it's an interesting theoretical result, It was Price, who one historian of statistics.
I think it describes him as the first Bayesian. So the first person to use Bayes insight on practical applications. And he, doing the same sort of thing like, can we use this to estimate how likely we would say it is that the sun will come up tomorrow, given that we've seen it X times.
And it's the same thing. I've seen the sun come up a million times. But I can never be certain it'll come the next day. So we have to do the same thing of saying, adding one to the didn't happen stage and adding one to the did happen stage. So instead of saying the next chance that the sun will come up tomorrow is, a million and a million, absolute certainty, instead it's a million and one in a million and two.
So it's near certainty, but you never quite get to actual certainty. You keep pushing it further, pushing it closer and closer. But he also wanted to do it to show that exact point that you never get to certainty. He wanted to use it as a sort of a hedge against or like a defense of of God against David Hume.
[00:24:00] And I really enjoy this. See him. David Hume, obviously a great atheist philosopher of the era and by all accounts, really lovely, affable, charming guy, but he'd written this thing saying, which is in itself, when you think about it, it's quite Bayesian, right? The, he said I can't remember the quote, but it was the people tell you that if someone says to you that someone came back to life after dead, after dying, you have to select, okay, what's more likely that, that actually happened or that this person is lying or mistaken.
And then he's saying, evidence of miracles, accounts of miracles you hear, to believe them you have to say it'd be even more unlikely that the person telling about them is wrong than that the miracle occurred. So it's actually, so he's saying, my priors, of these things not happening is so strong that almost no amount of evidence will overturn them.
Price's point was you can never be certain. You will, the fact that you've seen people not coming back to life a million times doesn't mean the million first time they won't. And these are both true facts about when we look at the world through a Bayesian [00:25:00] lens, it is true you never reach certainty, but it is also true that The very confident predictions, such as people don't come back from the dead, need a lot more evidence to overturn them.
So both men are just angling, taking different angles of the same, of Bayes theorem, I guess, to look at it. But it was it's quite nice because they had this sort of, exchange, but Price was quite uncharitable about about Hume and said all this like it's specious and all sort of stuff. And then Hume wrote to him and said, Oh, I see your point and all sort of stuff.
And then you can see Price going, Oh, I actually feel a bit bad about this. I've been rude and changing it. And so he said, you've been um, you've been really polite and thank you. And they had a really lovely exchange on the whole thing, which I always quite like, you know, people disagreeing about something really important and major, like it does God exist and still able to have a civil discussion about it.
Oh, that was great.
Benjamin James Kuper-Smith: Yeah. This is something great about someone being kind of vicious and the other person being really kind, and then the first person to feel like, oh shit,
Tom Chivers: Yeah, exactly completely undercutting them. Yeah. Oh, I feel like a right bastard
Benjamin James Kuper-Smith: shouldn't have done that.
Tom Chivers: [00:26:00] Yeah, Yeah, but yeah, but that's really but that's the first, it was the first half of the paper. A An essay towards solving a problem in the doctrine of chances. I'm pretty sure that's what it's called And he the first half of the paper is all bayes.
It's all Theoretical it's all a very sort of Ivory tower stuff and the second half is all price and it's all the applied things and basically him trying to Say no actually miracles could happen You david hume, and so so it starts out from beginning of having quite lofty applications.
I think it's fair to say
Benjamin James Kuper-Smith: Yeah. Yeah, definitely. There's a, yeah, the application is a lot more important than many other stuff. Um, So this was then, I guess, what would've this been like late 18th century or
Tom Chivers: Yeah, mid 1765, something
Benjamin James Kuper-Smith: like that. And then, so this is what happens then for the next, I know the, Pearson of these people were like 1900 or something like that.
Tom Chivers: Well, so what happened? I mean, a lot of things happened, obviously. Firstly,
Benjamin James Kuper-Smith: the world of statistics and probability
Tom Chivers: yeah, yeah,
yeah, [00:27:00] exactly. And then there was the Napoleonic Wars, no, the well, for a start the idea of this sort of, this inverse probability, as it came to be known, the idea of.
probability that tells you how likely something is to be true given some data rather than just how likely you are to see some data given that something is true. Um, that was independently discovered by, Laplace a decade or so later and it would have had his name on it had by I think, had luckily Price not gone to Paris and met uh, Laplace's uh, I want to say PhD supervisor, but that's obviously not right.
That's massively anachronistic, you know, sort of equivalent, some sort of his sort of,
Benjamin James Kuper-Smith: his mentor
Tom Chivers: yeah, mentor. Yeah. And he, and I think it was LeCondorcet or someone like that. He said he said, Oh, actually, Oh God. Yeah, this is exactly what Laplace has said. And so therefore by law of sort of priority, this should be Bayes theorem, not Laplace's theorem.
Laplace, very gentlemanly fashion went, yep, no, you're right. Even though I think by all accounts, Laplace is the one who's done the better treatment of it, gone into more detail, not just dashed it off as an afterthought and then died. Um, But uh, yeah.
Benjamin James Kuper-Smith: to [00:28:00] be very generous. And I mean, I guess maybe, I mean, yeah, that's just, I guess his view he was, was first, but I was a little bit surprised. Like when you, in your book when you get to that point and he's like, yeah, he just accepted it. He's like, Oh,
Tom Chivers: Yeah, no, I mean, that's the rule, right? And you can, oh, yeah, I suppose, oh shit. Oh bollocks. But
Benjamin James Kuper-Smith: I mean, people always have fears of being scooped in science, and man, that must have,
Tom Chivers: that must've really
Benjamin James Kuper-Smith: that one must have stung.
I guess Laplace has his, has his name in the history of mathematics. He's fine.
Tom Chivers: yeah, it's not as if we don't remember who Laplace is. But the, yeah, so, so it was rediscovered and it, then that it became, I think it was on its way to becoming quite a big deal, but. And I'm slightly cribbing from the history of a guy called Aubrey Clayton here, who is nakedly a Bayesian partisan.
Right. He had this his line in his own book, which was, there are lots of people trying to end the war. stats wars between Bayesians and Frequentists. I am not trying to do that. I'm trying to win the war. So he got some brilliant line like, consider this book [00:29:00] propaganda to be dropped from planes over enemy territory.
At least you're sort of really open about this. I quite, I found it quite refreshing, but he said like a lot of what happened after that was that people didn't, I suppose the big thing, which we haven't said really about Bayes theorem, is that the implication of it is the probability is subjective.
You know what I mean? Probability is, it's not some true fact about the world. It's not like we can say, if this coin were flipped a million times, an infinite number of times, it would come up heads half the time, whatever. We are saying, my best guess about how this coin will come up is 50 50, because I haven't got enough information to do better.
That, and all probability estimates are to at least some degree subjective, Best guesses of how likely things are to be how likely things are to happen. And that's really uncomfortable that a lot of people don't like that at all. They still don't they didn't at the time. And I think a lot of people wanted to say that no science is objective.
There is a true number, which is this [00:30:00] is how likely this thing this scientific hypothesis is to be true or whatever. And that. It's just not within the gift of Bayes theorem. It's not within the gift of anything, to be clear. You can't do that. But There's something that feels more sort of rock solid if we're saying these are actually the chance of seeing this result, given, given the null hypothesis, and therefore we can say, we can just say this is objective truth. I mean, of course, even that's not true. It's not completely true either, but you know, it has this sort of veneer of objectivity, which Bayes theorem doesn't.
So I think that, a lot of people got uncomfortable with that in the and the people kept trying to, , apply these sort of measurement techniques and statistical techniques to social science in the years afterwards. And I think a lot of for very, for various reasons. I mean, Clayton's claim is that it's a lot to do with people wanted it to back up racist claims about which different races are better and all that sort of stuff.
And they can just say, oh, we've got, the numbers are objective and say this. I don't buy that. I [00:31:00] really like Clayton's book. I think it's really interesting. That particular, it felt very inflammatory. And I bet if the history of science had gone the other way I'm sure there'd have been lots of Bayesians who would, people, you could justify the finding of the claim that Bayesians were more racist because it's just, people were racist then.
Right. You know, and, And the, the establishment was,
Benjamin James Kuper-Smith: and they were trying to find stuff out,
Tom Chivers: yeah, exactly. Yeah. So, I mean, I was speaking to a Bayesian statistician after the book was published and he was saying, well, there's a lot of people Firstly, you can't judge which framework of statistics is best by who had the most unpleasant adherence.
And secondly, it's not as if Bruno de Funetti, who Was a great I, he's dead. So I think I can say this without libel but he was I think he was a member of the Italian fascists, and he was one of the great pioneers of Bayes theorem and all this sort of stuff. So, there's just, I didn't feel it was worth, I don't think there's any real sort of mileage in there and that it was frequentism is designed as a cover for for scientific racism.
But. [00:32:00] And it is true that a lot of the people who followed it did and then it's just and people but also people did want to be able to say This is just objectively true. This is what we have seen and we don't there's something squishy and unpleasant about saying I'm not going to publish this scientific result because I didn't believe it was very likely in the first place, you know
Benjamin James Kuper-Smith: Yeah.
Tom Chivers: the problem right?
That's where it gets squishy. And I think people didn't like that then they don't like it now
Benjamin James Kuper-Smith: Yeah, I mean, it's still that in science, I think you still kind of have the sense of trying to find out how things actually are. That's, I feel like that's inbuilt into the whole proceeding that you don't go, okay, I'm trying to create, I mean, in a way, you know, you're creating models that predict some data or you're collecting data to test the model or both.
But it does seem to me that you are trying to find some sort of objective truth That's out there in the world to explore And I can understand that. I mean, especially maybe we'll get into a little bit later the whole problem of having over the prior invasions and how you select that, but [00:33:00] Yeah, I mean, I guess I guess the whole thing is that like I would imagine It's just very uncomfortable if changing the prior can potentially change quite a bit about the
Tom Chivers: Exactly. I mean, that is definitely uncomfortable. I mean, to be clear, right, subjective doesn't mean made up. It doesn't mean equally good. You can, and you can, the example I often give is if I, if we were gambling on the roll of a dice and I said, well, my prior is that this dice will is five out of, five times out of six, this dice will come out of six, and your prior was one time in six.
And then both of us would be rational to bet at evens on. It not coming up, on you saying it won't and me saying it will, but Under most circumstances your prior is better than my prior You will make more money than I will on that bet and I am And so a these models are better and worse insofar as they better predict the world it's the old george box another great bayesian george box.
Who said All models are wrong, but some are useful and
All these models of the world. I mean, even down, even down to quantum mechanics or [00:34:00] relativity, to some extent, they are just mathematical predictions of the world. Which, you and I would probably say, are getting closer and closer to describing the underlying reality.
But, they're just, they're still, they're not, they are not the underlying reality. The map is not the territory, and.
Whether or not we'll ever say, yes, we have now perfectly mapped out what reality is I'd be a little surprised if there's ever just a point where we stop like that.
Maybe there will be, but even so it would be, we have just met, we've made a model that we can't improve on and. It perfectly predicts all our data. So therefore we can say this is as good a model as we're going to get, I think that would probably be where I imagine would end up. And then I would also be happy to say, and I bet it's extremely close to a fundamental underlying reality, but I won't be able to approve that.
Yeah,
Benjamin James Kuper-Smith: Doing your science and suddenly you're like, wait a minute, I think we're finished.
Tom Chivers: exactly.
Benjamin James Kuper-Smith: I think I did it.
Tom Chivers: Pack it up guys.
Benjamin James Kuper-Smith: Okay, that was, oh my god, gotta find a new job now.
Tom Chivers: Yeah, exactly. It's been a good, it's been a good 2000 years guys, but we can all go home now.
Benjamin James Kuper-Smith: Yeah, but as you said, I [00:35:00] mean, as you said, but to add to that, I think I'm assuming Newton's model was thought of as being perfect for centuries and then Einstein came along and said, well, not always, but Newton's model is still very useful.
Tom Chivers: Yeah, I mean,
Benjamin James Kuper-Smith: it's not like it's thrown away,
Tom Chivers: no, exactly, well, it still tells you when the next eclipse will be on the continental United States or whatever, you can still say, I can predict a solar eclipse in Minnesota in the year 6000 AD or something, and it's still, I mean, I think I'm right in saying they don't bother with relativistic predictions when you're, slingshotting a solar probe around Venus, you just say the Newtonian physics does you perfectly well for that.
And again, to, for very high speeds and very long way out, it's only when you get up at not far off the the speed of light that really starts to matter. It does matter when you're doing GPS. Satellites, doesn't it? Cause they actually need to be down to a frac, tiny fractions of a second to do their syncing with other satellites and with your position.
Otherwise you end up slightly drifting off your position and people start driving into the North sea when they use Google maps and things. But
Benjamin James Kuper-Smith: Well, I think [00:36:00] they can also use perfect GPSs to do that.
Tom Chivers: yeah, well, that's also true. Yeah. But like actually the model that is Newtonian physics is astonishingly accurate and remains so even if there are slightly more. Tiny bit more accurate ones that work better at very high speeds and incredible temperatures or very large masses, whatever the different things are.
Benjamin James Kuper-Smith: Yeah. And what is useful depends, read on the context
Tom Chivers: what you want it to do. Yeah.
Benjamin James Kuper-Smith: yeah. I had a just because I found this amusing. You had when you talk a little bit about the, I guess the when Bayesianism or Bayesian statistics at least became more popular again, at least in some circles in the 20th century you talked you mentioned quite a few Bayesian songs.
Tom Chivers: Oh yeah
Benjamin James Kuper-Smith: Uh,
so if you want, feel free to sing a few otherwise you can describe what was going on
Tom Chivers: yeah, I'm not sure anyone wants to hear me singing. There's no business. There's no theorem like, like Bayes theorem. It was, there were some great ones. The um, do I dare, but mine eyes have seen the glory of the [00:37:00] Reverend Thomas Bayes and all that sort of stuff. Um, uh, that's, That's as much as I'm going to do.
Cause my wife ever hears me, my kids, they're
Benjamin James Kuper-Smith: That's nice.
Tom Chivers: Thank you. Yeah.
Benjamin James Kuper-Smith: I didn't, I didn't think you were going to sing any of
That was more than I hoped
Tom Chivers: yeah. Yeah. But it's brilliant. They had, they, the bayesians, get accused of being a cult, and I do sometimes think that maybe if they didn't have a literal song book about min Eyes have seen the Glory uh, that would've probably helped them, if the, but it was uh, but yeah, that was great.
There were these, so in the. 1970s, late 70s. Bayesianism basically got sidelined in the late 19th, early 20th century. The sort of giants of statistics were Francis Galton and then well, Pearson, Kyle Pearson the guy, Naaman, whose first name escapes me,
Benjamin James Kuper-Smith: Yeah, Jerzy or
Tom Chivers: yeah, exactly. Um, Yeah, it's, you know, you're right. Yeah, gee, something like that.
And and Ronald Fisher and they. hated Bayes. They just, they really, they Fisher described it as the greatest [00:38:00] mistake that science has ever committed itself to or something like that, Fisher and Pearson had a lifelong falling out, longer than lifelong falling out when Pearson misunderstood.
So Fisher came up with the, what we now know as the likelihood estimate. Which is of half of the Bayes equation, basically. It's working out how likely, how much more likely you'll be to see some result under one hypothesis than the other. Which is what you need to do to work out Bayes, but you need to, you also need to do full Bayes and need the prior as well.
Pearson misunderstood what he had done. Thought that he was doing, thought that he was assuming they were including a prior and that he was assuming everything would be equally likely and he's just saying that this is an error.
But he basically accused him of Bayesianism. Fisher hated being accused of Bayes so much that he basically, they fell out. Never talked again. And Fisher maintained a feud with not only Pearson, but also his son for essentially, for years afterwards, despite being, them both being given the those, sharing Pearson's chair of, they were the chair of the professor of eugenics at [00:39:00] um, UCL.
Interesting little detail. UCL used to have a chair of eugenics, but anyway, there we go.
Benjamin James Kuper-Smith: Also, just for context, Pearson's son was also a statistician. He wasn't just randomly attacking Pearson's son.
Tom Chivers: yeah, yeah, yeah, Yeah. He was also, but that was, yeah, so, because Fisher and Pearson's son ended up sharing that, that UCL chair. But yes, that is an important detail, I agree. So, yeah, I don't think it's overstating the case to say that Fisher and Naiman and Pearson invented what we think of as modern statistics.
There's a the Fisher in School of Frequent Statistics and the Naman Pearson School, and they all just utterly rejected bayesianism and, all the things people use to do statistics now, like P values, statistical significance, likelihood estimates, Ava L, that sort of stuff. It's almost all Fisher really.
And that it just squeezed Bayes out completely. But for the sort of 50 years or more between Fisher doing all this in the early 1900s and Bayes becoming more mainstream in the [00:40:00] sort of set, start the revival of Bayesianism again in the sort of 70s, people kept reinventing it sort of because it was useful.
It's actually, if you want to do an inferential probability, you need Bayes. So what's his face, Alan Turing invented something very Bayesian to do to do inference of how likely some, when they're working out the signals from the Enigma machines, you had to use some sort of, assume that some string of letters was more likely to read E I N in German rather than some random, J Q X, whatever.
And so you needed, he needed that. So he did, he reinvented Bayesianism. People kept using it for other, I remember speaking to one statistician. He said, like he went to Silicon Valley and met some friends who said, and who worked out a way of estimating of what the exactly what the software he was doing was.
But he's just he's just reinvented basis and Bayesianism. And tech, a lot of people are doing this a lot. And other areas as well. And then, Oh, what was his name? Jeffries, Harold Jeffries was. Involved in a big argument in the sort of 30s, I guess, [00:41:00] with um, Fisher about the different, but, you know, he was a geologist and it was useful to him to use prior data, cause he didn't have that much data when you're studying how waves propagate through the earth and you've only got occasional earthquakes to try and, measure them and not very good.
So it was useful to use Bayes where you're allowed to use pre existing data. Whereas under frequentist systems, you have to throw away all previous data and pretend each test is on its own. So Jeffries was a big fan of that and he had a lengthy debate with Fisher about it in the paper, in the pages of some scientific journal or other.
The debate was inconclusive by all accounts, but Fi Fisher won. As in we still use Fisher's stuff but then in the seventies, and this brings us back into the songbook , someone had basic I spoke to this um, former president of the Royal Fiscal Society, guy called Andy Grieve, and he said that whenever I used to turn up at statistical conferences. They'd like give the bayesians the joke slot, like the af the um, the before lunch slot is a, here you,
Benjamin James Kuper-Smith: Mm.
Tom Chivers: Andy to talk about bays. Eh, way everyone then um, and then they did a [00:42:00] conference in I can't remember. It was, I think in France somewhere. And it was just bayesians and everyone.
Oh God, I, it's quite nice this, I don't have to like defend bayesianism against everyone. Like I don't have to I don't have to give, but end up being treated like a comedy afterthought. So this was fun. And they, and so they started setting up more in in Valencia quite regularly. And then a few other ones, a few other places as well.
And it sounded like carnage, like they just got hammered and they were like having, they're saying like they all have a siesta in the afternoon and start working again at 6 PM. And then at 10 o'clock, they'd all go to these parties in the evening where they all like karaoke. And they were singing Bayes inspired song like a Bayesian to the tune of Like a Virgin, you know, um, that one I just sang you Bayesians in the night to the tune of Strangers in the Night, so David Spiegelhalter who, Professor Sir David Spiegelhalter, another former president of the Royal Statistical Society, he tweeted at me.
He's a good lad. He tweeted at me saying, our performance of the full Monte Carlo was before the smartphone era, so no recordings [00:43:00] exist. And they uh, so there was sort of six male professors of Bayesian statistics taking their clothes off in front of a screaming crowd in a Spanish nightclub. It sounds brilliant, anyway yeah, it was
Benjamin James Kuper-Smith: that's what, That's what happens once the Bajans are freed from having to justify themselves.
Tom Chivers: yeah. .
Benjamin James Kuper-Smith: I guess we've kind of gone through like the first, what is it, like two, three chapters in your book now,
Tom Chivers: Yeah.
Benjamin James Kuper-Smith: that. Um, And the, the, the remainder are specific applications.
And I guess to pick one out the one that actually surprised me was the most, I mean, not really surprised me per se, but, well, yeah, it did, so it did surprise me a little bit to see that, as the first application you mentioned was with the replication crisis, because, I mean, to me, like decision theory in Bayesian brain is like super obvious that Yeah, it's just all over it.
Um, And one is, it's in the name. Replication crisis for me and the stuff I've done on my podcast so far about it has usually been stuff about you know trying to replicate results and pre registration and all these kind of things. But I hadn't really done actually that much about [00:44:00] this aspect that you mentioned and how You How the replication crisis could be improved.
So maybe if you could kind of briefly start with a topic actually I've already discussed with, Your friend Stewart Ritchie I initially talked about, well, I think the first minute of my interview with him is me mentioning that I knew I talked to, yeah, it's too long. Anyway yeah, it's too long, but it's exactly about the BEM stuff.
Um, It's basically the first, the first minute of our podcast. So maybe if you could just describe kind of briefly 2011 stuff that was happening in that year and how kind of the Bayesian Bayesian statistics kind of then. Offer some sort of help or solution. Yeah, exactly.
Tom Chivers: 2011 was a big year in science, although, you know, I was a science journalist at the time, or at least writing a lot about science. And I didn't, I didn't know for three, two or three years afterwards, but it was There were various things. There was a Diedrich Staple fraud case in which it turned out the sort of up and coming star of social [00:45:00] science, psychology, I think social psychology, he he'd basically been making up his results.
I mean, completely out of whole cloth, that he, his, and lots of these quite famous results of his just were based on Um, and that
Benjamin James Kuper-Smith: Not just like tampering with data, but
Tom Chivers: they're just literally making it up. Yeah sitting at home with a glass of wine, apparently, filling in the
Benjamin James Kuper-Smith: Typing it in. Yeah.
Tom Chivers: um, And that was bad, but I guess, you know, fraud happens, right?
You know, it's bad because you don't want people to be fraudsters, but it's not as, it's sort of, uh, it's priced into an extent, people sometimes are bad actors. They are, they, and you hope you weed them out, but basically some people are just cheats, right?
Benjamin James Kuper-Smith: There's always going to be crimes
Tom Chivers: Yeah, exactly. But what was worse, in a way, was the same year there were two papers released which couldn't be true, really, unless something was totally different about how the world worked. Couldn't, the result they found couldn't be true, couldn't be [00:46:00] real, unless everything we understood about the world was wrong. And yet they were both proved, they both were true. They showed to the level of statistical sort of satisfaction that there's enough to get most things published, and they seem to use perfectly normal statistical techniques.
So the one with Daryl Bem who I, who you discussed with Stuart, I gather. And he did a study called feeling the future, which. I mean, without going into too much detail, seemed to show that people were psychic, and it found with very low p values, very and to an extraordinary level, to the same levels of statistical significance that we would be used, happy to use in other situations, and using the same statistical techniques, it showed apparently that people were psychic, so that was one problem.
The other one was, and that was an honest, serious paper, the other one was a sort of satirical joke paper. but serious point paper called False Positive Psychology. And it found, I mean, among various other things, that um, people who listened to When I'm 64 by the Beatles became younger. Not like they felt younger, but their [00:47:00] birthday became more recent than people who listened to a control song, which for 90s music fans was Kalimba by Mr.
Scruff. And obviously that can't, that logically cannot be true. I suppose it is logically possible that psychic powers might be real, but it's logically impossible that people's birthday could get near. I think that is a fair thing to say. So that really threw people and people were sort of like, well, what's going on?
And it turns out, that people have been using very small sample sizes. They hadn't been pre registering. You could, they were One crucial thing was there was, you could stop, you could test, do multiple tests and then pick the one that finds something , you could stop your data collection whenever you see that it's dropped below P value uh, given P value and then say, okay I'm, I'm uh, I'll stop there.
And these things were easy ways of getting low P values, apparently surprising results out of basically noise. And yeah you'll have discussed, like you said, you'll have discussed a lot of this in other situations. I'm a big fan of things like registered reports and pre registration for ways of limiting this.
But there, there are ways in which not being Bayesian in this situation can be harmful. I [00:48:00] think, I really want to be careful about overstating it as some sort of panacea, because I don't think that's true. But I mean, for a start, on the very specific problem of optional stopping where you can, you're gathering data and you can just decide to say, I'll stop now.
And look, Oh, look it's my P values dropped to zero point 0. 049 stop immediately. That doesn't matter in Bayes theorem because each new bit of data is added to the data you already have. And, you, so it actually optional stopping is a good thing in Bayes theorem. But that's quite a
Benjamin James Kuper-Smith: Good because it's more data efficient, right?
Tom Chivers: Yeah, it's more data efficient. You can, yeah, you, if you've got to a point where you're confident that your cancer drug or your vaccine works, you don't have to keep plugging away. To the end of the trial, you can get it in get it into publication, get it into production faster.
So that is a good thing. There's also a point that because like we've discussed under frequentist models frequentist will probably tell me there's more to it than this, but you do kind of have to throw away all your previous data. Unless you're doing a meta analysis, you know, if you're saying I.
This new study I've done, [00:49:00] we ignore everything that's gone before. And if it gets it under below, below 0. 05 P value or whatever is your threshold, we will treat it as though it's real, but that privileges in combination with the problems that we have with scientific publishing, people want novelty.
And that scientists are driven to get things published because otherwise they won't get, they won't progress in their career. The publish or perish model of academic careers and also the demand for novelty in scientific publications combined to like people are incentivized to try and get papers into into journals, , even if the findings aren't real.
And they are, and in order to do that, they will have to find novel, exciting results. And the novel, exciting results. If you are if any more, less than one in 20 coincidences enough to count as a novel, exciting result, then you are incentivized to go and look at really unlikely things and hope that you get a freak result.
You know? So like,
Benjamin James Kuper-Smith: of thing that's really surprising,
Tom Chivers: yeah, I, yeah. If you did like a study into do hammers fall [00:50:00] faster than helium balloons, then you get a, then you just drop a hammer and a helium balloon at the same time you do that. six times, I think you get a P less than 0. 05 result. If the hammers crashed the ground each time and the balloon floats up, then you say, look, I've got this, but don't go, I don't care.
Obviously that happens, right? But if you got someone to guess six coin flips in a row and say that would only happen one time in less than one time in 20, that's statistically significant. Therefore, this guy's psychic, that's much more exciting and impressive. And that would be In theory, obviously it's not really how it works.
That would, but
Benjamin James Kuper-Smith: Yeah, I mean it's obviously a cartoonish like depiction of it, but I think it still makes the point that most, usually it's not that obvious that it's a silly question and there's obviously more to it, but
Tom Chivers: yeah. You're, it privileges silly questions and. A virtuous frequentist would say, I'm only going to research things I believe to be true because I, they're, they use prior information about what they know about the world to guide them to [00:51:00] research questions that they think are likely to be true, but if you're unscrupulous or you're desperate to get it public, then you might, then you are incentivized to go and fish for out, for outlying results, and then you get all of the priming studies of like people saying.
Putting them in a room with a box of, a room that smells fishy and see if they act more suspicious when they're in a room because it smells fishy and you get people getting those results and that is not. I don't think that's very likely to be true to be like a real underlying replicable finding, but it, it ticks the counterintuitive box and Oh, this is exciting.
And it, you can, if you do, if you did 20 studies like that, on average, you get one freak result and then you take it to nature and say, look, I've, you know, so, so I think there is an element of frequentist model that does over privilege these freak results or silly questions. On the other hand, a frequentist would say, and a frequentist did say Daniel Larkins who's a very strident frequentist, but I think, a very bright guy, and obviously understands that you need Bayesianism for, to do estimates [00:52:00] of, How likely something is to be true, but he just he says I don't want dogma in science.
He says Sure, you publish you should do your research. Well, you should pre register your results You should do all these things But if a surprising result comes in you shouldn't say well, I don't think that's very likely I'm just going to sit on it, so I it's not it is not a clear cut i'm not coming down as pure bayesianism as the answer for everything, but It would solve certain problems while You And I think it's also just aesthetically neater and includes a lot more, includes a lot more of the system that you don't have to like keep bolting on new methods to do stuff.
It includes the whole data analysis by itself. It is its own meta analysis for a start a lot of the time. So I think it's a lot neater and more elegant. But it wouldn't be without its own problems. And one of them is you have to be careful about, as I'm sure we'll discuss, where you get your priors from.
Are your priors going to influence your result? Do you say, yeah, I think this is like 99. 999 percent likely to be true and therefore whatever data I brought in doesn't really matter. That, you need to be scru you need to be careful and guarded against that sort of problem.
Benjamin James Kuper-Smith: Yeah, I mean, [00:53:00] I've not yet done a lot of Bayesian statistics myself, or almost none, but to me, that always seems like just the big questions, what is, especially if you have, let's say you combine a few studies, often papers are, multiple experiments that, aren't exactly the same.
They're not perfect replication of each other. So for me, it's really, as someone who's still on the outside of that and hasn't done it practically himself, it always seems like that's just such a big somewhat arbitrary plucking a number out of thin air, of whether it's, 0.
1 or 0. 001 or 0. 5, like I don't, yeah.
Tom Chivers: Yeah, I mean, it's a problem. It's not a silly thing to worry about. Speaking to the Bayesians who did this, they were like, if you're doing it in pharma, depending on why you're doing it, you use preexisting, there's usually is data from earlier studies, and that's why, but that's why I was saying like, there's the meta analytic prior is, that's a, you can actually just you gather up the data from previous studies. You put them into you put them into a, like a, into your, into a meta analysis basically. And then you, and you say, this is our starting prior for the new ones.
And you [00:54:00] use, and that is a good point and a good way of doing it. There's, I mean, there's also, I speak to one guy who's working in I was I can't remember his exact, he's looking at Fishing techniques and developing world or something. I can't remember exactly. I don't know how you describe what he did, but anyway, he was saying you can look at that, you just use data, but then if someone comes up to you and he says I've no one in this In this part of the world would ever do that thing you're talking about there.
So it's not data, but it feels mad not to use that information in your to not use it somewhat to downweight your prior. So yes, it is arbitrary and subjective, but it is, it does need to be done. The other thing is that someone else pointed out Jan, Eric Jan Wagenmachers. He said you can. You can crowdsource bait, you know, like get people's like, does, does this prior seem reasonable to you?
And if someone says, no, you just, you put it as 99. 999 percent likely that psychic powers exist, I don't buy it, you can crowdsource and double check, but also you can check the robustness of your results. Well, what if I'd used a different level of prior? And if you've got reasonably good data, then. To some [00:55:00] extent you're, it should wash out your priors effectively anyway. If otherwise you're very, it sounds like your data is pretty rubbish, so but then you might reasonably say, well, in which case, what's the point of having a prize in the first place? So, you know, that,
Benjamin James Kuper-Smith: Yeah. No, I mean, it does. I think in part, it's also, I think just good modeling practice where you try and test your results over, if you, range of parameter values, basically to see how it affects it. I mean, it's, I think that is actually a very good point. That yeah, just see what would happen.
Is basically not like to see that basically how dependent are your final results on the initial prior you had.
Tom Chivers: Just a robustness check. Right. If I does, and if it completely changes everything, Oh, maybe my data was a bit rubbish, maybe we should be less confident in this and maybe I'm over relying on my priors, but there are ways, there are principled ways of getting priors.
I mean, there's a whole thing called that Jeffrey's tried to set up called objective Bayesianism, which was trying to do this in a more, objective way, obviously. They didn't really manage objectiveness, obviously, because none of us can. Objectivity, I should say. But [00:56:00] they made a more principled way of putting uh, putting systems in place for getting priors that aren't just, I pulled this one out of my arse, what are you going to do about it?
You know, um,
Benjamin James Kuper-Smith: yeah, Yeah. I think the general problem is just that there's so many, should I put it, so many things that are basically impossible to quantify when doing science, that it almost feels pointless. Even if you do a meta analysis, it's well, this study is probably going to be, has maybe an obviously better, you know, methodology than another study, but like how much better than you, would you want to quantify that into it?
And there's
Tom Chivers: Yeah. How much do you weight your studies? That's
Benjamin James Kuper-Smith: Yeah, I mean you can do it by number of people, but if one study is way better than the other, then is that even important that there's lots of people in it? And yeah, so I mean, yeah I probably will at some point start looking into this myself and actually doing it and using it, so we'll see how I,
Tom Chivers: I think it strikes me that there are definite uses for it. And I think in some places it improves [00:57:00] inference. And I also, I think it has a neatness to it, but you know. I'm just some guy who writes. I'm just, I'm, I'm a journalist. In the end, don't take statistical advice off me. And if you're in any way someone who actually works with these things for a living, you'll be able to read the equations in a way that I usually can't.
So if you find things work fine with frequentist methods and you're finding out true things, then great, more power to you,
Benjamin James Kuper-Smith: yeah, this is, we should maybe highlight, this is not a textbook.
Tom Chivers: Yes, Yes, exactly.
Benjamin James Kuper-Smith: Um, Yeah, before we get to the recurring questions , I just had a few questions basically about You, how you got to do this.
I mean, it seems to me you spent, you studied uh, philosophy, I believe. I found it somewhere on some website. It was a bit hidden, but I got, I got it. And something about medical law and ethics.
Tom Chivers: Yeah. So I did philosophy undergraduate at Liverpool university, which I strongly recommend. Northern campus universities to anyone who wants to northern it because it's just a lot of fun Anyway, there was I had a great time, but then went to um, [00:58:00] king's college london to do a master's degree at what was what is called their center for medical law and ethics, but It was more philosophy.
It was the it was under yeah, and it Then after my master's I tried to do a phd to Complete lack of success, had no idea what I was doing, but it would have been a PhD in the ethics of science journalism, but I hadn't worked a single day as a journalist, I didn't know what I was talking about, it was like being a, I don't know, a lepidopterist who's never seen a butterfly, it was just a lot of me trying to prove that my prejudices were ethical, you know, um, but it was, it was uh, uh, many such cases,
Benjamin James Kuper-Smith: Yeah, I was about to say that as well, but sorry, was this, so you started a PhD at the same center or what, and then just realized it wasn't working
Tom Chivers: Well, I realized it wasn't working when they said you can't have any funding after a year of self funding and then not getting any money off that. So
Benjamin James Kuper-Smith: Oh,
Tom Chivers: yeah, but it was, I mean, it was good. I learned I turned my. Work into some interesting articles later on. I also, I came out of it like, Oh crap, I've got to get a real job. So, and luckily managed to get a couple of weeks work [00:59:00] experience at the Telegraph and then Daily Telegraph UK newspaper. And managed to not cock that up basically. And uh, thank you very much. One of my proudest achievements.
And then uh, you know, over. The next several years tried to slowly steer my career towards writing about science y things and have successfully done that more and more, right, more I can write opinion y, feature y things about science, the happier I've been generally. And as for writing about Bayes in particular, I mean, I don't know if you ever used to read Ben Goldacre's columns back in the day Bad Science.
Benjamin James Kuper-Smith: Not the columns, that was, I grew up in Germany, so that was uh, that one, but then when I, I started my, my bachelor's in 2010. One of my flatmates really loved Ben Goldacre. So then I read Bad Science, Bad Pharma. We even went to one of his like talks or something
Tom Chivers: Yeah. Yeah. So, so yeah. So I think that was probably where I first became aware of Bayes theorem, him writing about exactly stuff we were talking about medical tests, this not applying in the same way you think they do. And people are getting confused by that. And that was [01:00:00] that was super interesting.
And then. And just by nature of being somewhat more mathematically inclined than most journalists, i. e. being able to multiply one number by another, you know, um, yeah, well, yeah, I mean, yes, the um, uh, that made me the guy who understood stats a bit, And it just, I just was interested in it.
So but like doing things, asking questions what is the denominator here puts you above a lot of journalists in terms of asking these sorts of questions and then, but then a lot of where I got really interested in the base side of things. I don't know if you were aware of a guy called Scott Alexander, who's um, uh,
Benjamin James Kuper-Smith: quote him in your book,
Tom Chivers: I do.
Yes. He's actually a psychiatrist in the US, but he's he's just a really fascinating blogger and part of the sort of wider community of nerds called the Rationalist Community who who my first book was about which was originally called The AI Does Not Hate You, but is now The Rationalist Guide to the Galaxy, for the paperback, and that was all, and they are very much about the Bayesian worldview and when you want to think about people thinking [01:01:00] Bayesianism is a cult, there's a good place to start.
They're not. I would say they're not, but you can see why people think they are sometimes. Um, um, But they they're very much, you know, he, the idea like the Bayes can describe the brain, Bayes decision theory, Bayes, E. T. Jaynes and all that sort of business. That was all, I was picking up all that stuff.
Now, God, this is amazing. This one simple equation that describes so much. And that was when I got interested. And then the reason I ended up writing this book I've, I've, I've written now three books, all three of them have at least a chapter on Bayes in them. Because of this obsession I've developed.
But the third one, the second book had, it came out during COVID. Very good timing. Um, But it had a chapter on Bayes, which was, yes, exactly. Really clever of me to start that pandemic. To leak that, to cause that leak in that Wuhan virology lab. Um, But yeah, the chapter on Bayes, the observer, British newspaper asked me to turn that into a.
A feature for them. I did the the editor who wrote, who put it together just completely like reasonably to my, you know, without really thinking about it said, because [01:02:00] it was just sort of how this one equation that you never heard of or something, how the simple equation explains, so much for the world or whatever, and he said, he called it this obscure theorem in the subhead and people went mental, they were absolutely mental.
Days and days people do obscure like I'm a professor of biostatistics, and I use Bayes all the time. Of course you do Like but you go out on the street and ask 90 percent of people they won't know you know They won't know who it is what anything about it, and yeah, I suppose it is a bit You know as theorems go it's not that obscure, but nonetheless.
I didn't think it was anyway either way I thought it was a bit of a crazy storm that people got incredibly upset about it, so I got
Benjamin James Kuper-Smith: guess it's like one of those famous, non famous things, right? There's some things that are like huge within a community, but somehow just haven't gone beyond it.
Tom Chivers: Exactly. Exactly that. Anyway, so I can see the point. I just got annoyed by the incredible hyperventilating about it. So I went to my agent and literally within two days, I looked back at the emails, within two days of this kicking off, I'd pitched a book about Bayes to my editor and to the agent and [01:03:00] editor.
And so that's why, but this book is born completely of spite. That was, that's where it's come from.
Benjamin James Kuper-Smith: I think that's the best kind of motivation.
Tom Chivers: exactly. Destroying your enemies. What's that? You know, To hear the
Benjamin James Kuper-Smith: But I mean, it's not really destroying you though, right? It's just, I mean, in a way you're helping them. You're like, Hey, I wrote this entire book about this thing. You're like,
Tom Chivers: Yeah, exactly. No, you're right. You're right. In fact, so yes, comforting your enemies and comfort. Yeah. Yeah. But yeah, so, and you're right. It makes it even less obscure. Hopefully there'll be people saying, I know all about, I now know all about Bayes. So yeah, so that, that's how I ended up where I am writing books about Bayes.
Benjamin James Kuper-Smith: Yeah. Why did you, one thing I found kind of interesting when I saw just the timeline kind of your career is that it seemed like you worked as a journalist for, so where was it that, was it the Daily Telegraph
Tom Chivers: Daily Telegraph, then BuzzFeed, and
Benjamin James Kuper-Smith: so it seemed to me that was like until like 15 and then it started, you started working for something like that, like more like online kind of publications and you started publishing books and that kind of stuff.
I'm just curious, was that like an intentional thing or why was it just kind of just coincidence that at some point you decided, I think I want to write books now or?
Tom Chivers: [01:04:00] So it was a bit of a coincidence. So what happened is um, 2015 Buzzfeed asked me to join them. So I went and joined them and that was just a Buzzfeed was very much the up and coming thing at the time. And it was cool and interesting and Telegraph was having a bit of a bad time and to be honest, still is.
So I was happy to get out of there, but then in 2018 BuzzFeed had massively overextended itself and started to do some redundancies. And I'd just been offered this book contract for the first book and I realized there was no way in hell I was going to be able to write it on a full time job.
So I said, I will take your undisclosed sum of money and I will go away and I'll write this book and it worked out. And then I'll it's a bit unnerving to go freelance, but when you've got an actual project and, lump of money to go away and do it. It was less Unnerving so I did that and that was
Benjamin James Kuper-Smith: But I mean, you, I mean, you mentioned earlier you have children was that, did you already have children
Tom Chivers: I did. Yeah, that was so 2018. So I had um
Benjamin James Kuper-Smith: increases the pressure a bit,
Tom Chivers: It does It does but then also by then i'd been a journalist for 10 11 years, so I knew enough people in the [01:05:00] industry with People to write for so it wasn't as bad as it could have been, you know there were people that I could write like my For a while I wrote for UnHerd and still occasionally pop up for them.
And that was easy because I knew the editor of it who I'd worked with before. And I say, look, I'm quite good at this. Would you like a piece? And they
Benjamin James Kuper-Smith: I see. So you're like, you basically had enough experience and,
Tom Chivers: and
Benjamin James Kuper-Smith: Contacts and skills,
Tom Chivers: Yeah. Yeah. Yeah.
Benjamin James Kuper-Smith: that you knew like the worst cases was not that low.
Tom Chivers: Yeah, exactly. It was very demonstrable that I could do the thing I wanted to do, that I was claiming to be able to do, which is why it's really hard at the beginning.
Cause you say you might be the best writer in the world, but no one knows, and they've got especially for like a daily newspaper or something like that, they're like, I've got a big hole in my paper tomorrow. If you don't file on time or if it's rubbish, I don't like you. So, so if you know that, so if you've worked with someone and you know that they like, they will get me clean copy, making a good point, Too length, you ask for 800 words, they file about 800 words, and they'll get it to you by deadline, and deadline at 5pm.
I remember getting an email from my [01:06:00] editor at the time, just, because someone had let her down, and she was like, I don't know, she said, she emailed saying, can you write me this piece? And I was like, yeah, what time do you want it? like, That's what a proper fucking journalist says, what time do you want it, not what day do you want it!
Like,
Benjamin James Kuper-Smith: Right.
Tom Chivers: yeah. Um, Uh, Yeah, so I still I, I've quite tempted to find that email and print it off, I remember that really fondly. So yeah, so, so that was less scary. It was scary, but it was less scary than it would have been for an and I'd found myself in struggling for work early on in the career and that had been difficult, but this was, I'll be fine.
I knew I'd be fine.
Benjamin James Kuper-Smith: yeah. Okay. Okay. And what's the uh, so now, so what is it now? Like you mainly write the books
Tom Chivers: so I, I, I should say I've got a, I've got a day job for a US media outlet called Semaphore, which um, set up by my old boss at BuzzFeed. And um, so he was New York times uh, he's got called Ben Smith. He was, he went from BuzzFeed to being New York times media columnist, but now he set up a new.
Website's, very much news focused called [01:07:00] Semafo, S-E-M-A-F-O-R with a guy with another guy called Smith, Justin Smith in a relation who used to work at, I think, he's going to be annoyed if I get this wrong, but it would be like he was, he worked at The Economist and Bloomberg and ran their sort of financial operations on that side of stuff.
So these sort of two big ish hitters, quite big hitters of US journalism kind of set up this thing. And they asked me to come and write their daily newsletter. That's my day job. That is that keeps me taking over. And then I write, I wrote the book in the background of that. And I've got this podcast with my, well.
Stuart Ritchie, our mutual acquaintance Stuart Ritchie, we do a podcast together called The Studies Show. So that's plenty. I'm thinking about pitching another book, but it's a lot of work, and I don't know
Benjamin James Kuper-Smith: Yeah. Okay. I was just, it's just because I think your book came out like in a two, every two years or something, the three books, I think. So, so it seemed almost like it was like, oh, this is what you do now,
Tom Chivers: Yeah, I should, but, and the second one was written with my cousin, and we do think, we have got an idea for another one together. But, I don't know when I'll find the time. So, so I'm keen to do it, but I'm there. Oh God, I [01:08:00] should. But you know, evenings are pretty full, that sort of
Benjamin James Kuper-Smith: Yeah, yeah, Yeah, no, okay but that was kind of what I was curious about because like for me I obviously checked out kind of what you're doing, but it was never quite like from an outside perspective, it was never quite clear what is this like a full time thing you're doing?
Or is this just like a small thing you're doing in the books are the main thing when I kind of
Tom Chivers: No the main day job is a classic journalism job. And then the books, if there are any more and the podcast fitted into time around it.
Benjamin James Kuper-Smith: I see. Okay. Uh, Should I do the recurring questions? Okay. So, at the end of each episode, I'll ask my guest the same three recurring questions. The first is what's a book or paper you think more people should read? This can be famous or completely unknown. It can be old or new. Just something you think more people should read.
Tom Chivers: So not necessarily nonfiction or um, whatever you
Benjamin James Kuper-Smith: Just a reading recommendation.
Tom Chivers: Ooh. I've managed to find some novels recently that I've really just enjoyed and I'm going to recommend a novel. I think the one I'm going to recommend is Piranesi by Susanna Black. Um, If you've, do you ever [01:09:00] heard of Jonathan Strange and Mr.
Norell? No. Okay. So Susanna Black wrote this book about Jonathan Strange, Mr. Norell, which is it's big and chunky and it's this strange otherworldly book about set it's set in England of the early 19th century, but they, England, it's just established that magicians or wizards are real in that world, but they sort of haven't happened for anyway.
So it's wonderful, eerie strange, somewhat unsettling book, but it's very long, but she also wrote this other much shorter book that I read recently called Piranesi, which P I R A N
Benjamin James Kuper-Smith: Yeah I'll, I'll put in the description the proper title and everything.
Tom Chivers: And again, it's just, it's essentially about this guy who. In elaborate. I, I, I can't begin to do it justice.
But again it's, it's strange and unworldly and there's something going on. You dunno what it is. And slowly that's all pieced together and you learn this anyway. So, so I, that, that was I loved, it was one of those books that I ate, I just ate up too quickly and I was really, [01:10:00] I, I wanted it to carry on.
So Piran by Susanna Black, that'd be my one.
Benjamin James Kuper-Smith: Okay, good. Second question is something you wish you'd learned sooner. This can be personal, private, whatever you want, but something you think, you know, if I'd learned that a little bit sooner, then I probably would have helped. And if you want or can also like maybe how you learned it or what you did about it or, yeah.
Tom Chivers: Yeah. That's a big and difficult question. So, I'm trying not to be too glib, there's also stuff I, dance like nobody's watching type things. Um,
Benjamin James Kuper-Smith: Believe in
Tom Chivers: yeah, yeah, Yeah exactly, exactly. Um,
Benjamin James Kuper-Smith: I mean if you, you know, whatever, I mean.
Tom Chivers: no, no, no, No, I'm trying, I'm trying to, I'm trying to think of what the, I think that there are lots of things that would be appropriate answers to this.
So give me 30 seconds and I'll
Benjamin James Kuper-Smith: Yeah, okay. Shorten the pause to 25 seconds.
Tom Chivers: yeah, very sensible. . Okay, so, the big skill that journalists need to learn, and this is going to sound so basic, right, pick up the phone.
Like phone people, like actually it's really, and it is now, it is such a basic thing. I like, I just [01:11:00] I, Find some academics number online. I'm just a pest to this guy. If they don't want to answer or they're busy, that's fine. Cause with academia in particular and journalism, the timescales are so different.
I used to want like joke about it being like like mayflies trying to understand continental drift, like I, I would email in an academic and say, can I speak to you for this piece I'm writing? And three months later, I get someone get in touch and say, Oh, I'm actually a bit busy this year But I can speak to you in 2026, you know And I wrote that I wrote that piece.
I finished that piece later that afternoon like well, I've actually forgotten what we were talking about so but I saw it a lot with young journalists when I was getting later in my career and as and for me when I was Yeah for me when I was um out just even just phoning press offices was unnerving.
I But it's actually it's not scary and it's important learn to be good at just like phoning people up asking questions That's so crucial to a journalist Also, I never had shorthand But I can type really fast and be make sure that you can take down these notes quickly that was something really useful, but I think [01:12:00] Don't be afraid to just phone people up I it took me a few years because of my strange route into journalism to be able to get really comfortable with it And now it is it feels like a superpower
Benjamin James Kuper-Smith: Yeah, just so before I forget it, one thing I found funny when you mentioned the different timelines between academia and journalism. I think when I mailed you, I think you responded two days later apologizing that you weren't quicker. And I was like, you were quicker than most people responded to me.
Um,
Tom Chivers: mean, Journalism has hours timeline, most of the time. There's always this magazine, it's different.
Benjamin James Kuper-Smith: yeah,
Tom Chivers: go, it seems like things happen over months and years, it's
Benjamin James Kuper-Smith: I mean, it takes, you know, up to years to write a paper, right? I mean, to actually do all the whole thing. Um, yeah, Yeah. Yeah, phoning is a weird one where. Yeah, I it's, it's, yeah, I understand how it can seem scary. And, especially because I guess you just don't know what's, yeah. Or what state they're in and what's going on or
Tom Chivers: well, I think also nowadays people I am such an old man shouts at clouds thing this but But I do I [01:13:00] get the impression now Just phoning someone out of the blue is almost a bit rude normally like people text to say is it a right if I call and stuff which is obviously
Benjamin James Kuper-Smith: one thing, one thing I find interesting is that my, so my sister's six years younger than me and she basically started studying again, basically when she was a little bit older not like super old, but yeah, like mid 20s or whatever, right?
Anyway, so her friends are then often 20 or 21 or something like that and she said every time they want to order food or something they have to call for whatever reason, she always has to do it.
Tom Chivers: Hmm.
Benjamin James Kuper-Smith: And she's, because just somehow even that five year difference or something seems to yeah, because I guess just not used to it at all, whereas she had some sort of use of it.
Tom Chivers: I do remember a young journalist when I was at Buzzfeed. So, I mean, she'd probably be 30 odd now, but the landline rang on her desk and she just like, stared at it. You know, You're supposed to, that might be something important. There might be someone with a story. You should probably answer that, like it just completely, I don't know what to do with this, but it was fascinating.
It was really fascinating.
Benjamin James Kuper-Smith: Yeah. [01:14:00] Final phone comment from me. Here's that. So I just started a postdoc in Zurich and we don't actually have phones in our office.
Tom Chivers: Really?
Benjamin James Kuper-Smith: Like There's, and it's annoying because I need to call someone recently and I was like, well, like there's no phone in our office. Like, where is it? It's like, oh yeah, we don't know.
We got rid of them. It's just, there's one phone on the floor, like in the middle of the hallway or something.
Tom Chivers: weird. That's a, we used to have them on every desk and it was
Benjamin James Kuper-Smith: Yeah, Yeah, exactly. That's what I'm used to too, but it's probably just because now people have mobile phones anyway or whatever.
Tom Chivers: yeah, it makes sense, but if I want to phone the US, I don't want to do it on my own personal mobile, you know,
Benjamin James Kuper-Smith: Yeah. Yeah. Anyway. Um, Final question. Yeah. So usually this question is, like advice for PhD students or postdocs, people in that kind of transitory period I don't know how, you know, most of the people I interview are in the academic system.
So I don't know how much you can comment to that specific one, but you can take it a bit more metaphorically if
Tom Chivers: Okay. Well, I would say I did Start a PhD. And my one advice would be make sure you want to do it. I did, I just, the reason I started the PhD was because I [01:15:00] was scared of the wider world, like I was good at university and I was good at my master's and I didn't know what I wanted to do with my career really beyond vaguely be a journalist, but I didn't know how you did that.
And I didn't know what I was supposed to do. And it's just staying in academia seemed like the easy course, you know, like,
Benjamin James Kuper-Smith: think that's a pretty common, yeah.
Tom Chivers: Yeah, exactly. I actually don't Make sure you've got some question you want to answer. There are too many people with phds and not enough places for people to get To this is my impression Anyway, there are a lot of people with phds these days and not that many places for them to work afterwards Getting a place on tenure track is tricky.
So if there's some question you really want to answer Brilliant. Go and do the PhD. Actually, I think the world will be better if, or I will be better, be happier if I've answered this question. The world will gain from it. Brilliant. If it's just I don't know what else to do, and a PhD is there, and it's I'm comfortable at university, be wary of that.
Because, It might not be that, it might be that at some point you're probably going to have to take the bold step out into the [01:16:00] world anyway because it might well be there aren't enough postdocs or tenure track positions in universities going around. There's a lot and so that's my bit of advice.
Make damn sure you want to do the PhD before you start the PhD. I think that because I didn't and I, I don't regret it, but I, I had to get out of it.
Benjamin James Kuper-Smith: I mean, that's basically my question. Would you still recommend people start, try it anyway and see whether it works or is that, because I guess you did it for a year or what?
Tom Chivers: did it for a year. It was fine. But basically I was being bankrolled by my parents for that year. Right. So not most people couldn't do that. It wasn't particularly a wise use of family funds anyway, but, and
Benjamin James Kuper-Smith: okay. Yeah. But like, let's assume like you have a scholarship or a position or something like that and you, you know, to try it out. Would you still think or, or would you say basically like,
Tom Chivers: I, I'd
be careful of it. I'd be wary of it because, I mean, and I imagine most of your listeners will be science in science, whereas I was philosophy, maybe not all, but, you know, um,
Benjamin James Kuper-Smith: yeah, no, that's true. Yeah,
Tom Chivers: yeah, [01:17:00] and my, it was very lonely, actually. It was just me sitting around in a library. I'm not, I don't wanna put people off, but that was, and this, it was 20.
It'll be 20 years ago this year, so things may have changed, but I think the fundamental reality of a lot of philosophy PhDs is that they are a guy sitting in a library or reading on the internet, you know, and that
Benjamin James Kuper-Smith: that is very different. Yeah,
Tom Chivers: Yeah, that was quite lonely. Whereas I think science PhDs are much more, you go out and you're on a team, you're titrating things together or whatever, you know, um,
Benjamin James Kuper-Smith: I mean it can still be I think a lot more lonely than people think when they enter it, but yeah, okay. That's definitely a big
Tom Chivers: Yeah, exactly. So basically the only people I saw were my housemates. Some other people on a related PhD course would go for drinks sometimes, but we wouldn't wouldn't be working together and my, my, I see my supervisor once every two weeks or how many, every so often, and it was a bit like, yeah, I'm just, I'm basically, it was the mid 2000s.
Um, YouTube would just become a thing. And it was very difficult, very, you just [01:18:00] end up sitting sadly watching early YouTube videos of plane crashes and not, and I should be doing some work and I'm not doing any. And and I just, I'm just a bit lonely, so, so that I would say that is a risk.
I don't want to put people off academia because I think academia is important and good, but I do think there's a, there is a certain sort of person. And I was that sort of person for whom it was a sort of comfort blanket. And you just, you don't. actually know what it is you want and you're I'll just, I'll keep doing that because I'm bright.
And university keeps telling me that I'm good at university. So I guess I'll just stick with university. But actually you could use the same skills that you've just developed in your however long at university to do things in the world, which firstly earn you more money and secondly, build more skills and thirdly, probably have a clearer career path.
So, Be very confident. Just try and be confident that academia and a PhD is the actual thing you want and it's not just sticking on the route that you were already on and inertia is carrying you forward. I think that'd [01:19:00] be my, that'd be my advice.
Benjamin James Kuper-Smith: Okay. Yeah, and I guess to just add to that is that If if people try something else um, Try something else, like journalism, whatever, and then realize it's not for them. The PhD program is still going to be there.
Tom Chivers: Yeah, you can always come back. I do think sometimes I'd like to go back and, having now done a bit of work as a journalist, over the last, 15 years, I could go back and do that Ethics of Science Journalism PhD and it would be a bit more informed. I'd know what I was talking about instead of just making stuff up and, so, I I, that would be, yeah, don't be afraid to go out into the real world for a bit if you're not sure that the PhD is exactly the thing.
Yeah. No.
Benjamin James Kuper-Smith: Don't be afraid to go out occasionally.
Tom Chivers: exactly. It's cold and scary and there are wolves, but you'll learn things, you know.
Benjamin James Kuper-Smith: okay, thank you very much.
Tom Chivers: That's alright.