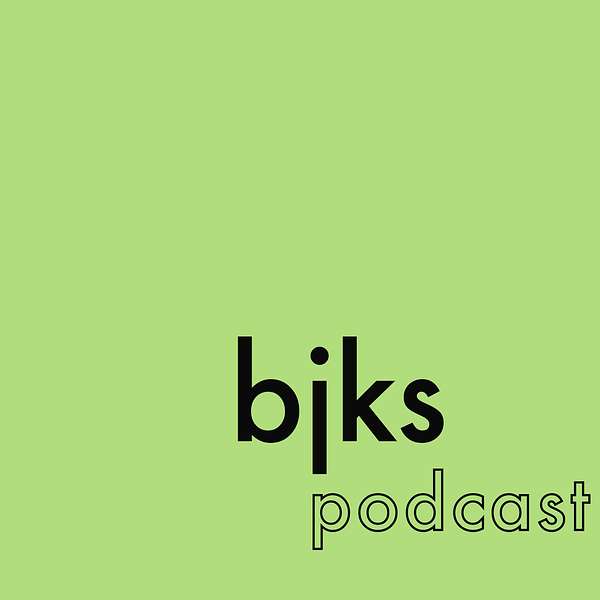
BJKS Podcast
A podcast about neuroscience, psychology, and anything vaguely related. Long-form interviews with people whose work I find interesting.
BJKS Podcast
82. Geoff Cumming: p-values, estimation, and meta-analytic thinking
Geoff Cumming is an Emeritus Professor at La Trobe University. In this conversation, we discuss his work on New Statistics: estimation instead of hypothesis testing, meta-analytic thinking, and many related topics.
Support the show: https://geni.us/bjks-patreon
Timestamps
0:00:00: A brief history of statistics, p-values, and confidence intervals
0:32:02: Meta-analytic thinking
0:42:56: Why do p-values seem so random?
0:45:59: Are p-values and estimation complementary?
0:47:09: How do I know how many participants I need (without a power calculation)?
0:50:27: Problems of the estimation approach (big data)
1:00:08: A book or paper more people should read
1:02:50: Something Geoff wishes he'd learnt sooner
1:04:52: Advice for PhD students and postdocs
Podcast links
- Website: https://geni.us/bjks-pod
- Twitter: https://geni.us/bjks-pod-twt
Geoff's links
- Website: https://geni.us/cumming-web
- Google Scholar: https://geni.us/cumming-scholar
- Mastodon: https://nerdculture.de/@thenewstats
Ben's links
- Website: https://geni.us/bjks-web
- Google Scholar: https://geni.us/bjks-scholar
- Twitter: https://geni.us/bjks-twt
References/links
Dance of the p-values: https://www.youtube.com/watch?v=5OL1RqHrZQ8
Significance roulette: https://www.youtube.com/watch?v=OcJImS16jR4
Episode with Simine Vazire (SIPS): https://geni.us/bjks-vazire
Coulson, ...(2010). Confidence intervals permit, but don't guarantee, better inference than statistical significance testing. Front in Psychol.
Cumming & Calin-Jageman (2016/2024). Introduction to the new statistics: Estimation, open science, and beyond.
Cumming (2014). The new statistics: Why and how. Psychol Sci.
Cumming & Finch (2005). Inference by eye: confidence intervals and how to read pictures of data. American Psychol.
Errington, ... (2021) Reproducibility in Cancer Biology: Challpenges for assessing replicability in preclinical cancer biology. eLife.
Errington, ... (2021) Investigating the replicability of preclinical cancer biology. eLife.
Finch & Cumming (2009). Putting research in context: Understanding confidence intervals from one or more studies. J of Pediatric Psychol.
Hedges (1987). How hard is hard science, how soft is soft science? The empirical cumulativeness of research. American Psychologist.
Hunt (1997). How science takes stock: The story of meta-analysis.
Ioannidis (2005). Why most published research findings are false. PLoS Medicine.
Loftus (1996). Psychology will be a much better science when we change the way we analyze data. Curr direct psychol sci.
Maxwell, ... (2008). Sample size planning for statistical power and accuracy in parameter estimation. Annu Rev Psychol.
Oakes (1986). Statistical inference: A commentary for the social and behavioural sciences.
Pennington (2023). A Student's Guide to Open Science: Using the Replication Crisis Reform Psychology.
Rothman (1986). Significance questing. Annals of Int Med.
Schmidt (1996). Statistical significance testing and cumulative knowledge in psychology: Implications for training of researchers. Psychol Methods.
[This is an automated transcript with many errors]
Benjamin James Kuper-Smith: [00:00:00] Anyway, we'll be discussing statistics, new statistics, confidence intervals, all that kind of stuff. And I wanted to maybe start kind of quite broad and, uh, maybe... a little vague and that's kind of the question of what's the purpose of statistics and in particularly what I was wondering is that when I had a, you know, a little bit of a look about the history of statistics, it's, you know, very recent.
I mean, Karl Pearson founded the first statistics department at UCL in 1911, Fisher, Neyman and Pearson's son were all born in the 1890s. Um, and by that point, you know, we had Galileo, Kepler, Newton, Maxwell's equations, uh, special relativity, thermodynamics, even statistical mechanics, um, even, I guess it's probabilistic mechanics is a better word.
Um, we had, uh, evolution, natural selection, the periodic table of elements. So kind of, why do we need statistics? It seemed like science [00:01:00] was going pretty well until then.
Geoff Cumming: a number of people have argued that, uh, well, look back and see what, um, uh, what Skinner and Piaget and those greats did without all this fancy p value stuff. But I think we need to, well, obviously we need to distinguish, um, descriptive statistics that basically summarize data and take the average of things.
And inferential statistics, has been the thing we agonize most about we're in any discipline where we have to deal with samples. And so you look at a couple of dozen people, and you measure a few things, and then you try and draw conclusions about the whole world, or... All people vaguely like that group of a couple of dozen.
That's inferential statistics, and it's magic. But it's only part of science, science mustn't be [00:02:00] regarded as just hinging on whether we use p values or Bayesian approaches or confidence intervals or just what. Above all, we have to follow the basic. Principles of Science of Careful Observation, and Repeated Observation, and Replication, along with Building Theories, and then Matching up theories against our data and drawing conclusions like that.
And especially now that big data has come along where you can get millions or billions of data points. Well, p values and estimation and significance testing. really have no place at all. And we need to think about what we're measuring and how repeatable those things are. And if we see a pattern, is that going to be repeatable next time we look or in some different stream? So reasoning with data really at the basis of it [00:03:00] all. I'd rather like to go back to the way I first encountered this, because I had puzzles right at the start. I remember as about a 14 year old, my father, an engineer, off and learned psychology. And he was telling me a bit about data analysis and drawing conclusions, and he mentioned this weird thing, T. And then he said, well, from that we calculate P, and he explained a bit what P was, I think did a reasonable job, I can't remember the details, and then said, well, if it's less than 0. 5, well, we've got something, and if it isn't, we haven't, and I recall, now this might be an enhanced memory, recall thinking, hey, whoa, that's backwards. That's sort of the wrong probability. It's backwards and 0.05. Well, why not 0.01 or 0.1 or, you know, totally arbitrary. And I sort of shook my head and, and, and he said, well, that's the way it's [00:04:00] done. So I thought, no, well, that's the way it's done. And didn't think anymore about it for years later. Went to uni. Did, uh, pure maths, did a couple of years of mathematical statistics, physics, got browned off with physics, oh, it was going to, particle physics was going to save the world, and this is back in the early 60s, us free energy and solve the world's problems. But the way it was taught somehow was so tedious, there was no room for uncertainty or thinking or having to grapple with what seemed to be the heart of science, of doing experiments and not quite understanding and reading up. So I swapped to psychology, and in my third year I actually had to pay fees, and I did psychology, and it was a revelation. to go to labs and measure things, and the person on the next desk got something a bit different, and we had to figure it out. Why? And then we went to the library and read stuff and wrote essays and [00:05:00] practical reports and things.
It was amazing. This struck me as much more like what science really should be, dealing with uncertainty. Well, I hope that physics and chemistry these days has taught much more the way that was when I encountered it. And so I went on in, uh, psychology and statistics and spent a career at La Trobe University, um, Largely teaching statistics to people who didn't want to be there. And I did all the, uh, all the official things from the textbook that was about significance and p values, but I tried to do it properly. And boy, the number of furrowed brows and things just was rather depressing. But I stuck with it, and I think I did it about as well as most people trying to do it seriously. And I used to get, um, students to rate, at the start of the course, um, rate their attitude from, [00:06:00] um, fairly reasonable through to blind panic. then the blind panic group I'd meet at lunchtimes, and we'd go through very simple things. And... They were often older students, maybe had raised a family and had a career, decided to come back and psychology, first time in their life to have a big chance at university. so I spent a lot of time sort of trying to figure out how to how to examples, and I did as many things as possible pictorially as I could. So it's a bit bizarre to be, uh, having a sort of audio interview discussion like this, when I'm itching to move my hands and point to things that sketch in the air and so on. the last few decades of my life developing software and software simulations designed to make things vivid and simple [00:07:00] and visual and moving. to try and make them to grasp them. So where were we? Ah, yes, um, the origins of statistics and p values, you're quite right that, uh, Fisher and Neyman and Pearson, p values and constant syllables, Roughly a hundred years old these days, and they famously disagreed, and significance testing, or NHST, Null Hypothesis Significance Testing, that has really become deeply, deeply cemented within psychology, biomedicine, many other disciplines, to the extent really that in the last half century or a bit more, since the sort of 1950s, 60s onwards. I'd say it's become really an addiction. It's just cemented so deeply. And I, one of the things that makes me weep is that I know [00:08:00] that many students encounter the t statistic and p values and so on, late on a Friday afternoon at the end of a lab car class. a harried tutor, a graduate student maybe, suddenly realizes we need a little bit of time on to write the report, to do the results section. And so they swallow hard, and they quickly write on the whiteboard, look, trust me, this is what you do first. assume something that's a bit bizarre and you know isn't really true, that is, there's absolutely no effect, no difference at all. Second, apply this formula get this thing called p, this is a p value, a sort of related probability, but, but, um, a bit hard to follow exactly why. Then, if that p value is very small, That [00:09:00] means it's very unlikely you got the results you got if this bizarre assumption we made is actually true. Okay, repeat after me. One, two, three. One, it presumed, etc. like that. And I think the saddest thing is when The really switched on, uh, obsessive, uh, smart people come up afterwards and say, Now hang on, have I got this right? And they sort of say it back to you, and they are right. And they say, Are you serious? Is this what grown ups in universities do? Is this how scientists... Do their stuff. you say, Gulp, um, well, that's a big part of it. And that's, um, that's what you read in the, in the journals. And, um, uh, if you can get on top of that and follow it, well, you know, you'll do very well.
That's good. And you'll understand what's in the journals. And that's how we will do it. so [00:10:00] either they say, Ah, okay, we'll look. right, I get the picture. I can do that. And off they go. Or they say, oh, I think I'll change to art history, or go and take up folk dancing, or, you know, something else. And I, uh, sometimes wonder how many minds we've, we've lost to these disciplines of, uh, these bizarre things we've chosen to use. So, uh, having, uh, my experience with my father, and then some of this teaching experience. I gradually got more interested in estimation and confidence intervals.
I mean, I'd done math stats and I'd done the, uh, uh, Neyman Peirce and stuff and, uh, knew about confidence intervals and so on. And it was only really, I suppose, in the, into the 80s and 90s that, um, I [00:11:00] started reading some of the stuff really critical of Stenovicus testing. And then, one important influence was, um, Frank Schmidt, in about 1996.
He was a stern critic of NHST and always seemed to me to make sense. He's very outspoken. Fine. And, um, also mention, um, Ken Rothman, a decade earlier in the 80s. He was, he was very outspoken. And he published paper after paper in Medicine, he's a very distinguished epidemiologist in Boston. Uh, he published paper after paper about how to calculate the confidence interval when you've got, and then insert, uh, measures very often used in medicine.
Like, Relative risk, or log ratio, or correlations, or concentrations, all sorts of medical things like that. [00:12:00] he, uh, I met him a few times and had good times talking with him in Boston way back. And he would challenge people. Okay. Okay. Thank you. Give me a case you think it's essential to have a p value, and you come up with something like, Okay, I've got a four way analysis variance.
I'm interested in the three way interaction. You say, Oh, no problem. I can give you a confidence interval for that estimate of that three way interaction. Sure. so you'd go on and you'd start getting multivariate and more complex. Yeah, yeah, I can do that. And he had the great achievement back in, it was about 1983, he persuaded the, now let's see, I'll get this a bit wrong, International, uh, International Council of Medical Journal Editors, something like that, bunch of editors of the top medical journals got together and they made a statement. this statement, I mean, not quite as strong perhaps as Rothman would have liked or I would have [00:13:00] liked, but a statement that basically whatever you do, however many p values you report, thou shalt report confidence intervals. And basically, since then, the middle 80s, most empirical medical papers report their confidence intervals. Now sometimes they just sit there in tables they're never referred to. Hopefully they influence the, um, uh, the discussion and at least they're there, so anyone reading them can... Seek them out and say, oh, you say this is significant, but wow, plus or minus, it's 10 plus or minus 8. I'm meant to be impressed. So confidence intervals there. So Rothman was a very early influence. And then there was Jeff Loft. Oh, and Rothman incidentally took over. Now he founded a journal, I think Epidemiology, he was the founding editor, edited it for nine years, his policy [00:14:00] was, we do not publish p values. we found one actually in a footnote to one paper, but that's not bad in a Admittedly, many people would just publish their p values and then say, since the p, since the confidence interval does not include zero, we've got a significant difference. Well, that's true, but that's throwing away most of the Useful information in the conference at all. So we had Rothman then and then we had Jeff Loftus in the middle 90s in psychology Editing a journal and spending enormous amount of editorial effort in persuading people submitting to this journal to report error bars, confidence intervals or standard error bars, and even to omit p values at all.
We did a study, we looked at hundreds of papers back then, back when you had to do it by hand or by eye, and we had this category of the full loftus. [00:15:00] This was, um, any paper where there were confidence intervals or, um, Standard error bars, there was not a single p value. And we found 8 percent from memory, I think it was either 6 or...
I think it was 8 percent of the papers published over those couple of years had gone the full loftus. And the... A proportion of papers that had confidence intervals or standard error bars rocketed up. But then when, um, Loftus's term as editor ended, the following person came in and no doubt did a very credible job, but without this, um, constant persuasion, it all slipped back and few confidence intervals were published. Schmidt came along and The journal called Psychological Methods, uh, an American Psychological Association journal. was started in 1996 with Mark Appelbaum as the first editor, and [00:16:00] Appelbaum controversially accepted a paper from Frank Schmidt that was highly critical of p values and MHST, it gave lots of reasons, had a little table, little simulation.
Now, Frank Schmidt worked in, um, a sort of industrial psychology, and, um, he worked typically with correlations. So, things like, um, the performance of some job, aptitude test for some job, and you look at the correlation between the score and performance later on. So, correlations. And he did a little simulation.
Now suppose there's a true Value of correlation of 0.3 or something, we take samples of size 50 or whatever, and then we're simulating in the computer. So situation, nice. Normally distributed populations, nice independence and so on. And we just [00:17:00] repeat this little study over and over again. Oh, look at the P values.
We get 0.03. I mean, these look. or less random. And of course, if you think about um, the whole basis of power, power is the probability that if there is an effect of a particular known size, say like the 3 correlation population, your little study will actually find statistical significance. And in psychology, having power of 0.
5 in lots of cases a bit of a luxury, and people do work and publish studies with lower power. Strange but true. In which case, suppose you had power of 0. 5, then you'd expect to do it ten times, and on average you'd get five of those experiments would be significant, and [00:18:00] the other five wouldn't. So why are we surprised if a simulation gives you a whole sequence of different p values? By the way, the, um, history proves that Frank Schmidt was right, because that paper, I think, has been by far the highest paper in that whole first volume of that journal, so Applebaum made the right decision when he accepted that paper. Anyway, being this, um, visual obsessive that I am, I thought, wow, if they're the p values you get, and I also know with confidence intervals, every book that explains confidence intervals properly has a little picture of a whole lot of confidence intervals sort of bouncing down the page.
So here we go again, here I'm trying to make a picture with words. So imagine a vertical line down the page, and that's the value in the population. Let's do it [00:19:00] in, um, not in, in, uh, correlations, but just in ordinary means. And suppose we have, um, the experimental group that was, say, given the relaxation treatment, and the control group that wasn't, and we asked them about their, um, attitude to life, or their Current well being or something like that. And suppose that the population of control people have average score of 50, but the people who've had the relaxation have an average score of 60. And we take a sample of, say, 32 each group, independent, and we give the experimental group the relaxation, and we take all the scores, suppose in the population there is a real difference of half a standard deviation. maybe the, um, standard deviation is 20, the spread is, um, Uh, that wide, and so I'm supposing there's a true difference from 50 to [00:20:00] 60, that's half a standard deviation. And that turns out two groups of 30 or so to have a power of about a half. if I simulate that in a computer, and I look at the difference between the, uh, experimental group and the control group, I'll get that difference, differences, bouncing down the screen. And if I collect them at the bottom of the screen, I'll have a sort of hump shaped heap. That's the heap, the sampling distribution of the mean. And it's heaped up around the true value, about a difference of 10. And, uh, uh, slopes off either way from there. And now I put confidence intervals on all these, uh, differences bouncing down the screen, and they bounce around from left to right, left to right, left to right. Then, against each confidence interval, I have a p value. And the astonishing thing is that [00:21:00] those p values, exactly as Frank Schmidt found and predicted, they vary enormously. Now we're not just talking about, you know, 0. 02 to 0. 08. talking about point than 0. 001 up to 0. 5 and 0. 8. around dramatically. I apologize for having to sort of do this in words, but if you go to YouTube and you search for a dance of the p values, you will find a couple of videos where that's all of them. So, when we run a simulation like that, of course, in a very privileged position. We're imagining we're in the computer and we know exactly what the true populations are, of course is ridiculous in real life as a researcher.
All you know is particular [00:22:00] set of 32 controls. 32 data points, and 32 experimental data points. you calculate that, and you get a particular difference, and you get a confidence interval, and you get a p value. So now think again about all those confidence intervals dancing down the screen. Suppose you close your eyes and grab from the screen just a single confidence interval. Now you're the true researcher. Does that confidence interval tell you anything at all about the whole dance down the screen? Answer is, it does. Because the length of the confidence interval gives you some idea about the width of the dance. those intervals bounce around, mainly, to some extent overlapping. And if we had smaller samples, of course, we'd have longer intervals and they'd bounce more widely. Much bigger samples, and of course shorter confidence intervals, and they wouldn't bounce around nearly as much. So the confidence interval us [00:23:00] very good information about the amount of uncertainty. against that confidence interval we got, you've got a single p value. Does that single p value tell you anything at all about the dance down the screen? Absolutely nothing. Virtually nothing. And so, if we report just the p value, then we're throwing away a vast amount of information. The confidence interval is much more. Much more informative. Now, I need to switch tack a bit and say that, uh, since the middle of, well, really since the start, in fact, um, Kurt, um, referred to this right at the start, some controversy, some criticism about this whole p value thing and significance testing, and, uh, certainly, um, Fisher would be horrified at the thought that it was set up as a hard and fast, um, hard barrier.
This is, so, What we're going to decide significant or not. [00:24:00] No, no, he said got to aim in science for a situation so you can repeat an experiment and regularly get significance. Well, he didn't realize quite how stringent a criterion that was. Anyway, so what developed in science was bizarre for a number of reasons. The backward probability jumping to certainty from just getting P that happened to be less than 0. 5, the misperception of the amount of bouncing around, and many other problems as well. These were described in compelling arguments by scholars across a whole lot of disciplines from the middle of the last century. Even as early as 1980, there was, um, uh, one famous, uh, Michael Oakes wrote a beautifully scathing critique, it was the Imbian Statistic, and he's saying, having gone through all these criticisms, [00:25:00] but we've known this for 40 years or more. have been willfully stupid at ignoring it. And there were similar very sweeping statements, not some nitpicking statistician's tiny correction needed, but the whole foundation is crazy.
We're just not making cumulative science. And then came along the replication crisis. Oh, perhaps most famously 20 years ago, 15 years ago. Where even in medicine, one famous case of a wanting to choose a promising area to investigate, to invest a whole lot of money in drug development, so they looked at number of studies right at the forefront of cancer research. And these were studies highly significant, in very good journals, done by reputable people. they said, right, well, before we sink the money in, we need to replicate them. [00:26:00] So they did. A bit of difficulty doing it, but they did. And they found, I forget exactly, but definitely smaller than 50 percent of the studies.
It was 15 or 20 or something, a very dismal number. It was eight out of 50 or something replicated properly. And this became known, this was in medicine, life and death, and in psychology, similar sorts of studies. Things that were out there, in very good journals, highly significant. Just couldn't be replicated.
What was going on? then John Ioannidis came along. I think it was, what, about 2015, is that right? And published his paper, Most Published Research is False in a Medical
Benjamin James Kuper-Smith: 2005, I think, right?
Geoff Cumming: I think you're right. Yes, 15 was much too late. Yes, yes, 2005. Well, that's a bit of a bold sort of statement. [00:27:00] Why Most research findings are wrong. And then he had, unfortunately, a quite complicated sort of argument, but what it boiled down to, my take on that paper, was that first, select publication. If journals will only select, only publish, on the whole, results that are significant, the five out of ten, or whatever it is, that happen not to be significant, never see the light of day. So the stuff that is in the journals is biased towards those that happen to find a slightly larger effect, and so get a slightly smaller p value, more likely to be significant. And then, uh, second, there's immense pressure on us all, of course, to publish. That's what our deans insist on, that's what we have to claim, have to show to get research funding.
So, well, we... have our data and we're convinced there's something in there. We just have to look a bit harder. And so, of [00:28:00] course, there are these outliers we really need to exclude. And, oh, look, we really should take logs because this is the measure it is. And, uh, we do all sorts of things like that that are not really wrong. But we don't realize how many degrees of freedom, uh, statisticians have this, um, this saying that, uh, if you torture the data sufficiently, they will confess. And, uh, I've had some most upsetting experiences giving talks about all this and afterwards a graduate student or postdoc will come up and case in tears and say, look, I get what you're saying.
I agree. Yes. But I, I, I take my results and analyses to my professor or my supervisor and they say, away. There's something there. Find it. So, uh, young students in many cases get the picture and it's the old fogies look who's talking [00:29:00] the old fogies who edit the journals and dish out the research money and become deans They're the ones who are, perhaps in most cases, many cases, most deeply addicted to this whole. So A& E's first is the circulated publication. Second is the pressure to do p hacking, and p hacking is really jumping through rather questionable hoops until we've got significance. Then the third is, if we put on such a pedestal, then we don't bother to replicate. for centuries, The whole history and philosophy of science is full of, well, what's one of the basic tenets of science?
It's got to be repeatable. It's got to be objective. Somebody else has got to be able to go and look in the same place in the sky and see the same thing. Or if you do the same procedure in the, in the lab, you should get the same sorts of results. no, uh, funding body in the past has been interested in [00:30:00] funding replications. Less interested. Journals less interested. They want the new and the sexy and the innovative. to, uh, boost their ratings and their advertising so they're interested in publishing replications. Anyway, so that led to the rise of Open Science about 10 years ago, and, uh, the rise and development of Science practices aimed at improving research procedures and All the way through from publication to everything to do with conducting research and statistics and, uh, evaluation of research.
Try to make it more open and trustworthy and, uh, replicable. Now you'd think that that's the very... basis of science, why do we have to have these enormous arguments and conniptions just to, to do what we should have been doing all along? [00:31:00] And in fact, there's a nice, um, term, um, it's not so much the replication crisis as the methodological reawakening. perhaps scales from our eyes and we're now beginning to do, at last, what we should have been doing all along. And, uh, so that's the, um, uh, one more way to put it is, okay, open science above all demands replication. Now if we've got replication, a number of people have tried doing this and got all their results, we have to have some way to Combine these results. How do we do that? analysis. What does meta analysis need? Estimates, point and interval estimates, confidence intervals. P values and significance testing need play no part at all in meta analysis. And so that's another major reason for wanting estimation[00:32:00]
Benjamin James Kuper-Smith: Yeah, so, I mean, in the new statistics paper, the beginning of the final paragraph is the key is meta analytic thinking, appreciate any study as part of a future meta analysis, um, so I was hoping you could expand a little bit on that.
Geoff Cumming: with enormous pleasure. So, uh, meta analysis, uh, Gene Glass is one of the founding people in. Psychology and Social Science, 1976. of he and his wife conducted a laborious meta analysis with billions of office cards, stacks of them. And he was constantly frustrated because these studies he was reading, didn't report what he needed. um, he has a famous quote that we've got at the, uh, at the beginning of Chapter 9, our meta analysis chapter in our introductory book, which is that, um, Statistical [00:33:00] significance is the least interesting thing about the results. You should describe the results in terms of measures of magnitude. What we now call effect sizes. much different was it? Not just does a treatment affect people, but how much does it affect them? That's what we need to know. Now surely that's the statement of the obvious, but that's what he found he had to do. So he went on and meta analysed this, and I was fascinated to read this. It struck me as just... basic and simple and straightforward. And I started teaching meta analysis to my beginning class more than 20 years ago, and I used the forest plot, which is a fancy name for a very simple picture. So suppose you've got a dozen studies on more or less the same question. All you do is put one above the other. the graph, the mean and the confidence interval from each of those dozen studies. And so of course the [00:34:00] means vary a bit from left to right a bit down the screen. And the confidence intervals are longer or shorter depending on whether it was a small study or a large study. And then at the bottom, you have a sort of, the outcome of the meta analysis, which is really just a souped up, fancy, weighted average of all those results. And weighted because if you got a study with a very small confidence interval, that means probably a big study, lots of participants, then it should earn a heavier weight, and it does. And that's the basis of meta analysis, and I've had, um, probably in, in, in that teaching about meta analysis from a forest plot, very simple diagram, the thing that would teach you just. Just would die for. Students coming up afterwards, instead of the expression, say, Oh, that was good. That made sense. Oh, gold. Can I [00:35:00] bottle that? Wow. And that's one of the great pleasures of teaching, uh, confidence intervals. meta analysis from the very start, before people have been exposed to this addiction of seeing living as testing and all that ritual. Now, I have to mention our introductory book, Introduction to the New Statistics. Bob Kalin Jaggeman is my co author, a brilliant young guy in Chicago who's doing all the software for the Uh, second edition, done a fabulous job with that. Our second edition, uh, should be out in, uh, March next year. And, uh, uh, we'll be starting work on the page proofs. boy, I'm enjoying reading, and I reckon that's good stuff. Anyway, that's just a, uh, objective arm's length. Uh, uh, but, he and I agree that it is such a pleasure starting off psychology with confidence intervals because they simply made [00:36:00] sense, and they're visual. And you could talk to people in the street about, say, Okay, look, there was an opinion poll the other day, proportion of people who, um, I don't know, uh, support the Prime Minister on some particular issue.
And it showed that, um, 46 percent of people, in a poll with an error margin of 2 percent supported. And I suspect most people will get the idea, OK, 46, that's from a survey, so if I did it again tomorrow with a different number of people, I might get 47, not 42, or 45, or something. And the error margin of 2, well, that's better than an error margin of 5, not as good as an error margin of 1, means probably the true value amongst all people is within 46, plus or minus 2. Pretty likely. And that understanding of a confidence interval, the plus or minus two, [00:37:00] not too bad. And if people have that understanding, then they're well on their way. And the trouble in psychology, of course, is that, particularly in the past, we haven't reported confidence intervals. One reason we haven't is that they're so embarrassingly long! And if I do all this work, and I get a difference of 20, it's significant, that's wonderful. But if it's actually 20 plus or minus 16, You impressed? I could have told you that about doing the study. yet, 20 plus or minus 16 is probably about 02, or something like that. Oh no, it's pretty close to P.
01. Oh, significant! Doing estimation. I've grown up, of course, with this p value thing, and the trouble is dichotomous thinking. It encourages you to think there is an effect or there's not an effect. It's significant or it's [00:38:00] not. But if you estimate, then, following Jean Glass, to what extent, how big is it? so this has led me to think that the very basis for this change in thinking to the way we should be doing science, open science and estimation, is way you ask the first question. So I try and school myself, and I never say, I wonder whether it would make a difference. Because of course it'll make a difference, it just might be trivial. You always ask, oh, I wonder to what extent it would make a difference. So if you put to what extent in front of every question, will steer you towards, uh, thinking in terms of estimation, confidence intervals. similarly, meta analytic thinking means, as in that quote, always having in mind that very rarely does a single study [00:39:00] answer any question in science definitively. And we have to always think that, um, in most cases there will be other studies out there we should know about so we can combine the results. Or we're thinking of our next study, or your next study, or can we find someone else to replicate it so we can compare notes and combine, combine our insights. And so that's what I mean by meta analytic thinking. And it's not only in psychology and medicine, you know, um, one of the, the, um, founders of, uh, uh, in psychology and education, Larry Hedges, one of the pioneers in, in, uh, meta analysis, he published a paper, uh, a while back, uh, 1987, possibly, there might be a bit wrong, on, um, hard is hard science?
How soft is soft science? And he said, well, okay, we know in physics, you know, Newton's laws and, and things are, um, Einstein [00:40:00] thinks are cut and dry. You measure things to how many decimal places? let's go out to the frontiers. Let's go out to, then it was particle physics. Say the lifetime of the mu meson.
Do you lose sleep about that? Well, he, well, the first thing is he discovered that in physics, there were a lot of similarities to the case in social sciences, that different labs would get different results. And so they'd visit each other and they'd double check their, their calibrations and their procedures and their measures and so on. And they still got different results. So they developed techniques to combine results. They didn't call it meta analysis, but the formulas were actually very similar, Larry Hedges failed. So, I'll make this short, um, he... He chose a dozen, 15 examples from particle physics, a dozen, 15 examples from, and social science, meta analysis in every case. And he reckoned the amount of [00:41:00] variation study to study was very similar in physics and psychology, at least back then. And so of course you can quibble in various ways about that, but at least he's saying It's not just a sloppy old medicine, sloppy old psychology problem, this is a problem for science. one more thing, Bayes, Bayesian statistics, a totally different approach to things. In fact, all of us in real life are probably Bayesian, meaning we if not consciously, know and take into account the likelihood that things will happen one way or the other. we're always much more open to the expected and the unexpected takes us by surprise. And Bayesian statistics is another whole approach, and um, I first took some courses in that in 1966, And it's Uh, terrific and it's growing and I have, um, many [00:42:00] close colleagues who, are very strong advocates and lead it and do very well and we have very productive conversations backwards and forwards. And I think the most important thing is dichotomous versus estimation. And if you do that in, uh, Bayesian statistics, well, you use credible intervals instead of confidence intervals. A whole different philosophical basis. are numerically very similar, and I'd be delighted if you use credible, uh, intervals. I think were that many Bayesian's still focus on, um, on using the Bayes factor to make hypothesis tests in yes no decisions. All I say, oh no, no, no, All in favor of estimation, yeah, that's what they do. if you estimate, then I'm totally with you. If you make um, Bayesian analyses and Bayesian modeling, I'm totally with you. fantastic. I'll mention one more thing. Significance [00:43:00] roulette. If you're tantalized by that, um, that phrase, well, go to YouTube and search for significance roulette. And see if that dramatizes to some extent, there's an explanation as well, dramatizes the variation in p values. Calculating a p value is a spin of the roulette wheel.
Benjamin James Kuper-Smith: Maybe one question I had is why, why is the kind of p value, as you say, such a roulette? I mean, it seems like, shouldn't there be... It should be some sort of, it should be somatic in some sense, or why is it kind of so almost random what you get out of it.
Geoff Cumming: Well, suppose you do a study and you happen to get exactly p05. And then you replicate. Well, you've got a 50 50 chance that next time it'll be a bit more or a bit less. And it might be quite a bit more or quite a bit less. Suppose you get, do a study and you get 0. [00:44:00] 01. About a 50 50 chance that replicate and you'll get the next p value. than 0. 01 or more than 0. 01. So, of course, replicating after 0. 01, on average, you'll get a slightly smaller p value than after 0. 05, but the point is, both cases, there's dramatic variation, and I think the extent of that variation is the thing that hasn't been brought out by that half a century of logical, careful, cogent argument about the problems of the basis of significance testing, of misunderstanding p values, of interpreting whether it's just one or the other side of the significance boundary. And so my aim was to not go for the, um, just endlessly banging on the same arguments trying to persuade people, but go for the gut. Try and have [00:45:00] a dramatic demonstration that answered the p values. or even perhaps more, significance roulette. This is the sampling distribution of the p value mathematically laid out.
I mean there's no doubt about these things used in the normal very simple assumptions, the extent of that variability, but it hasn't really been grasped by people. We have empirical results from statistical cognition experiments that researchers tend to drastically underestimate the extent that p values do vary. So they do have some information. If you have a very small p value next time you're likely to get on average slightly smaller. But there's enormous variation and that's the important thing. Because the p value doesn't tell you how much uncertainty there is. Confidence interval does. It waves it in your face.
It might be shower, so wide, but it's truth. You've got to take account of it.
Benjamin James Kuper-Smith: Okay, [00:46:00] um, I kind of, oh, I know the answer to this one, but because I read your paper, but for those who haven't, uh, so are these complementary? Should we use both? Some, some significance testing and confidence interval?
Geoff Cumming: Wash your mouth out. Um, uh, look, uh, there are those who, and journal, a few journal editors have tried to ban the p value. I'm not of that, not of that school. hope it'll just wither and die as we realize it's, it's not adding much. If you want to add a p value as well, fine, but I urge you to base your interpretation on the point interval, sorry, the point estimate, the mean or whatever, and the confidence interval, so that your is based on saying, well, we found a 70% improvement, um, with a, uh, confidence interval from plus or minus 5 or plus or [00:47:00] minus whatever it is. And if that's the basis of your conclusion, then, uh, I'm, um, I'm very happy.
Benjamin James Kuper-Smith: One question I had is, uh, you mentioned it earlier already a little bit, Statistical power. So basically the kind of question is if when, you know, which comes from the, within the significance testing framework, if we don't use that how do we know how many people we need for a study?
Geoff Cumming: Yep. Good question. And, um, the answer is we use precision for planning, called AIPE, in Parameter Estimation. And basically we say, look, I've done this, um, survey and I've got a. Margin of error of, um, two. Um, but look, I'd really like a margin of error of one. Okay, I need a big sample. Now, how much bigger? So, if you say, uh, look, I'm doing a, uh, a [00:48:00] study with two independent groups. Say an experimental and a control. And I want to estimate the difference um, or minus, um, 0. 2. This is a Cohen's d of 2, or a 0. 2 of a standard deviation of the population, plus or minus 0. 2. Then, uh, look up the book and, uh, look up our book in, uh, chapter 10 with the precision for planning. Table, picture, or use our software and... Uh, just move the, uh, slider to 0.2 and you can read off that you need a sample of or 82 or whatever. It's, you might say, oh, whoa, I can't afford, that's far big. Alright. Um, let's suppose you say, um, instead of plus or minus 0.2, let's make it plus or minus 0.3. Ah, well, I can get away with only 40. And so just as with power, people traditionally will say. [00:49:00] Okay, to get power of 0. 9 I need so and so, 0. 8 I need so and so. So, the same with precision for planning. the advantage of this is that you're thinking in terms of effect sizes, of the extent of the difference from the very beginning. Your planning, your analysis, and your interpretation, and your meta analysis, all focused on the That length of the confidence interval. And so, you don't need power at all. And, uh, that, we argue, is a far better way to do things. One more little reason, oh yes, I haven't even mentioned the new statistics. Why did I call it the new statistics? Well, in a way, it's a bit of a cheek, because the techniques themselves, intervals, they're approaching 100 years old, and meta analysis, getting on for, years old. And of course, they're not new, but it would be new for [00:50:00] most researchers in many disciplines, particularly psychology and other social sciences, to use estimation and meta analysis as the basis for their work. So in that case, in that sense, it is new, and it would be a great step towards building more quantitative disciplines, more quantitative models, quantitative assessment of goodness of fit, and so on.
And that would be, that would be a great step forward. Um,
Benjamin James Kuper-Smith: Nothing's perfect. So I'm using, let's say I'm, I'm doing a new study now. Um, I've got my, my point estimate. I've got my confidence intervals. Um, well, I mean, basically what are some problems with confidence intervals or maybe what's, I mean, this is of course very difficult to answer in the, in the generic case.
Um, but what are some things that are maybe missing from it or that, yeah.
Geoff Cumming: to go back from the very beginning, um. mainly talking about statistical inference. We start our book saying that this is [00:51:00] primarily about statistical inference, is, drawing conclusions from a sample of limited size to, and trying to apply them to a population. are many logical steps, and there are many things we need to be interested in. The measures we're using, and the reliability and validity of those, and the justification for the assumptions we're making about independence of sampling and so on and so on. So they all need thought. If we're dealing with very small samples like four or five or six, like quite often in biology and drug development, things like that, where things, where effect sizes are enormous, well then the confidence interval, um, doesn't tell you very much because as you go from sample to sample, it bounces around in length enormously because you're estimating, estimating that from the data.
So imagine if you've got a distribution and you take a sample of say four data points, And then another sample [00:52:00] of four, and then another sample of four. Those four in some cases are going to be closely lumped together, in other cases very much spread out. So when you estimate a confidence, calculate a confidence interval in those two cases, you'll get very different lengths. Much easier to have a picture, but symbols for very small samples can be misleading. At the other end of the scale, I was mentioning before, if you've got millions of data points, well then, P is essentially 0, 20 decimal places, and a quantum symbol is invisible, infinitely small. And so, That's irrelevant. We're estimating that point, um, precisely, but you still need to have all your critical faculties at the alert. Are you measuring the right thing? Are the assumptions about independence or whatever justified? To what other situation or other population is it justifiable to draw conclusions? so on. So all [00:53:00] that reasoning about data, all those additional things that we introduce in, even in chapter one, when we're talking about a very simple sample survey, uh, and introduce confidence intervals and meta analysis in that, uh, chapter one, those other issues come in. so there are many situations where the calculation of a confidence interval is not the most important thing, but we still need that logic of estimating, um, from our data.
Benjamin James Kuper-Smith: Wait, so what do you do when you have, uh, I mean I never do or read about science that has millions of participants, uh, I don't think. So you, you can't use p values, you can't, confidence intervals don't tell you anything. What do you do in that case?
Geoff Cumming: Well, um, just think of the data scientists laboring away at Google and at Amazon and at X and at, I don't know, all those other places, uh, collecting basically unlimited, unlimitedly large data sets then [00:54:00] asking questions of it. And so... I hesitate to think what sort of questions they are, but, um, they are, um, ones that they can answer without any concern about, um, confidence intervals or p values. can answer in percentages or whatever they like, the things they need to think about are the things I mentioned, that is, have they got the right measures to answer the questions they want to ask, and so on. And, of course, if you've got a million data points, you can ask, oh, Sort of combination questions and so you go data trawling and then you're wondering well I found a patent here, but is it real or is it just cherry picking?
Is it just seeing faces in the clouds? And that's a different sort of question and a thing we discuss in even the intro book because even with quite small sorts of designs you can have There are several affects you might want to look at and you need to consider those problems of cherry [00:55:00] picking, but they become more in the spotlight, much more of concern in very big datasets. So it's a bit different and certainly estimation is not the important thing, but all the other aspects of open science having all your materials and data and procedures of things out there publicly to the extent you can and encouraging replication and encouraging, um, combination, meta analysis, cross studies, all those logical things still still apply.
Open science is just as important. Actually, could I add just one brief story about that, that big science it did a different way. of the Higgs boson. Now I don't know the details, I might get some of this wrong, but of course that was a monumental advance in fundamental physics on Equipment, course, there's only one such device, it [00:56:00] cost billions and many years to develop. Enormous teams of hundreds of scientists working on it. So how do we analyze it? We've got this just gigantic terabytes of data. So being very sensitive to the problems of cherry picking and so on. we can't just search around and say, Oh, look, here's an interesting pattern. Uh, I understand that they, uh, the sort of guardians of the data the only ones who sort of looked at the whole set for a start. They had two teams of highly skilled and committed, uh, statistical analysts. And operating totally independently, the of umpires, the monitors, gave each just a selected subset of the data. The same subset, random, but selected to be as similar as possible to the whole set. And the, each team was allowed to explore this as they like, they can think of let's try this analysis, that [00:57:00] analysis fitting this, um, uh, fitting this model, et cetera, et cetera. Then they had to decide how they were going to analyze the data, and had to write that down in detail, sealed envelopes, submit it to the umpires. When they'd committed to this analysis, then they were, each team was given the whole data set. They had to apply exactly the analysis that they had specified. And then we get the results. And of course, they could then do further exploration if they'd like, but that was a much lower status. Could have been, um, uh, cherry picking. And happily, their results very largely agreed, so we were convinced. Now, I'm sure I've got details of that wrong, but the point is that even in physics, that we think of as being precise.
Those same issues of cherry picking and big data and are we measuring the right thing and how do we draw conclusions and so on, that we see in tiny miniature scale in [00:58:00] psychology and medicine. They still apply. And so the open science sorts of principles really are very important and apply very broadly.
And it's um, this development of open science the last decade is probably the most development in the way science is done a long, long time. And there's now a discipline called meta science, which studies science itself in a quantitative sort of way evaluates the open science practices and the open science innovations with a view to refining them and improving them. So this is a, a beautiful way in which science is sort of delivering on its promise to be ever self improving. And we can feel good about that, and we can feel good that so many young people, early career researchers, are getting into this in the, um, oh, Society for Improvement of, SIPS, S I [00:59:00] P S, Society for the Improvement of Psychological Science.
So many young people, so much energy. Unlike that poor woman who came to me in tears because a professor wouldn't Allow this or that. They're just picking up the ball and going with it. And there's some SIPs, for example, and workshopping stuff and running hackathons and developing tools and developing new ways to do things to make it easier. And that's a fabulous development.
Benjamin James Kuper-Smith: Yeah, I mean, if anyone interested, I talked to Zemin Vazir a few weeks ago, so that's episode 80, I think. So we talked a little bit about SIPs.
Geoff Cumming: Yeah, yeah, yeah, she was a co founder. Yeah, she's right on the ball, no doubt about it. And she's the new incoming editor of Editor in Chief of Psychological Science, which is a, just a totally fabulous development. We're all quaking in our boots at, at, at what, at what changes she'll, uh, she'll make.
Benjamin James Kuper-Smith: Well, I mean, I asked her what changes she wants to make, so, uh, there's some answers to that question in our episode. Uh, I'll, by the way, I mean, for those who don't know, I put it, uh, I [01:00:00] put it in the description, including references of stuff we talked about, papers we mentioned, your book, uh, second edition, that kind of stuff.
Um, uh, yeah, so now we can, uh, Move on to the recurring questions section. So first one is always a book or paper that you think more people should read. Uh, I mean obviously now is your, the new edition of your book, but uh, maybe one that you didn't write yourself, a book or paper more people should read.
Um,
Geoff Cumming: the book, the international, well, the newstatistics. com. If you go there, uh, and read the first two sentences, you get a link, and then you can go and read chapter one of the first edition, second edition, more or less the same. And they're very simply, no formulas, few pictures. You get the whole of open science and new statistics laid out.
An on the ball 14 year old would understand it with no trouble at all, I bet. Okay, uh, I want to mention one old book and one new book. The old book is How [01:01:00] Science Takes Stock, of meta analysis by Morton Hunt. And that's from about, um, when is it? 1997. So what, 25 years ago. And this is very chatty and very informal and really it's the story starting off with Jean Glass of meta analysis and even how the U.
S. back in the 80s a meta analysis to help them decide whether some particular that supported single mothers. should have its funding continued. Just astonishing. So that's the story of meta analysis and I thought it did a great job in, um, telling a bit of the I won't say salacious, but a bit of the sort of, uh, reads a bit like a slightly racy novel at times about the early meta analysts. no, don't get your hopes up. A [01:02:00] little bit of stuff. that's the first book. the second book, uh, A Student's Guide to Open Science by Charlotte Pettigan, very new, a few months old, a quick read, a hundred pages or so, telling a very personal story about how she started off, had her hopes dashed, was terribly disappointed, uh, depressed, then sort of Discovered there was this whole movement going on, so she leapt on board and has become passionate persuade everyone to learn about it, use it, read it, contribute to it, and so improve their, um, improve their own research. And, uh, so they're the two I mentioned. Of course, they're, uh, exactly on the topics I've been talking about, but... But there you go. I think they're both, uh, for rather different reasons, um, extremely good reads.
Benjamin James Kuper-Smith: Mm hmm. Uh, second question, something you wish you'd learned sooner. Um,
Geoff Cumming: well, where do you want to start? Now [01:03:00] I think the, um, the absolutely core thing is And I had some outstanding scholars, uh, and I, Gained an incredible amount, uh, this was at Oxford in, oh, many, many years ago. Um, gained an incredible amount and lots of people came through and gave talks and I always buttonholed them and, pestered them and so on and so on and some came to stay and being a non Brit, uh, being an Australian there, uh, they would sort of almost take me into their conferences as they, um, were sounding off about how terrible the English were and things. Some of those were very influential, but I think finding, perhaps even deliberately, perhaps even negotiating with people, are you prepared to mentor me, perhaps more than one person, and really trying to build up that personal relationship with somebody you really respect. [01:04:00] Whether you're working in a very close field or close topic, whether it's a supervisor or, or just what, or a couple of different people, two or three people for different aspects of what you're doing, finding that person to be a sort of support and advisor and just to hear you discuss through the sort of issues, should I do this or put more time into that?
Or am I going up a dead end here? So I think that can be immensely valuable. Thank you. And I've certainly, I think I should have done more of that, uh, particularly starting off in a lecturing career earlier. And I certainly try my best to that, that sort of role with people, um, who have been working with me and colleagues and so on and so on. mentors.
Benjamin James Kuper-Smith: Mm hmm. And any advice for PhD students or postdocs? Uh, let's say, people on that kind of [01:05:00] transitionary period, as I am right now.
Geoff Cumming: Well, every case is different, isn't it? I mean, I. Yeah. Yeah. I recall having discussions with other experienced supervisors and people talking about the issue of, well, you know, some students, really have to. them, wish them well, do what they choose, give them lots of rope and off they go. They might stuff up a few times, but gee, you just keep an eye and they go really well. Other people, well, you need to sit down and really map out experiment by experiment what they have to do and keep their nose to the grindstone. So, it's all different. Richard Feynman, bongo drummer, amongst other notorious things. Oh, extraordinarily brilliant physicist, was all for encouraging people, uh, they'd get advice from their professor, well look, just choose some nice good experiments, the next ones in the series, do two or three of them, stick to [01:06:00] this little plan, staple them together, there's your PhD. do you want to be like that?
No, no, no. So, this is a chance to out and follow your, follow your, um, Hunches. And of course that's tricky. We all have to try and decide. We've got limited time, limited resources, limited space. Where do we invest it? being a bit bold just might pay off, even if we've got a bit of safe stuff there as a backup. that's, that's, and I'm a bit in favor of the, having at least a slab of the Feynman boldness there. Collaborations are immensely valuable, and if you can leverage off some, um, skills you've got, it might be in experimental design, it might be in lab techniques, it be in particular skills in editing or doing meta analysis, or whatever. If you [01:07:00] can, use that to build some, some collaborations, then that would mean you've got a broader and you saw just a percentage of your time doing those broader things. Then hopefully you'll have some names on papers collaboratively with those different groups. learn a wider range of skills, meeting a wider range of people. And you're less dependent on your sort of core research, working out brilliantly the first time, as if it would, um, because you've, you've got that spread. I think too, uh, I've seen lots of students, or a number of students, I should say, really, who say, come into it, they're, they're a bit doubtful about statistics and things, but we get into what now we'd call meta science, studying how things are done. And so, really consider that, even if you're primarily a, Social Psychologist, or Cognitive, or Neuro, or whatever, developing an interest in how science is done and keeping a bit of an eye on SIPs and an eye [01:08:00] on Meta science might help improve what you're doing also even possibly lead to some collaborations or some chances to contribute. And one of the interesting things about open science is that there are several systems and several practices emerging of sort of global collaborations. And so somebody will nominate a project and look for collaborative groups, perhaps around the world. And so, if you're, say, a cognitive psych, there might be, primarily interested in cognitive, there might be some cognitive projects, and you could do the, uh, Australian, or the Swiss, or the Argentinian, or the Brazilian, or whatever version of it. And that would be interesting in its own right, it would contribute possibly to a paper, perhaps a replication report with 20 or 50 or something contributors. And so that's another way of sort of thinking laterally as to how you [01:09:00] might develop some breadth in your research. Also, don't be limited to the college, to the university. Particularly these days, colleges and universities are poor, many academics are really terribly, terribly exploited and under recognized, and recognize that in industry and public service, in business, are all sorts of opportunities for research, and might come by slightly different labels. But think of the skills you've got in, not just data analysis, but in conceptualization of variables, in the basic idea of how you evaluate things. it's very sobering, I guess, if you have any sort of role as a statistical or methods consultant, even perhaps some distinguished researchers will come to you, and you find yourself going through very basic [01:10:00] questions. We need to solve this. What question were you really asking? But don't I do analysis of variance on this? but question, what answers were you seeking? Now, I caricature a little bit, but you get the idea. And, um, once you've had a bit of that experience, then you're in a situation, you've perhaps been in a, uh, a department in, in a situation as a student where all these immensely bright people around and you perhaps don't realize how, how good you are, what skills you have, what What you do have to offer and maybe if you can find some work experience or find some people to talk to out in Fields that might interest you whether it's in government or public sector or nonprofits or whatever just might find that the skills you've got And you'll have to adjust a bit and use a bit of a different language and things. In fact, you've got [01:11:00] really strong skills that, that can do a lot of good in the world. And when, uh, students, whenever, I think, most people in universities have this experience, they go and survey where their students went, and they finished up in places you'd never think of. But the sort of general skills of, um, Thinking clearly about data and presenting things and trying to design studies that didn't have biases and confounds and try to combine results with different things and present them in graphical ways that made sense to ordinary people. I mean, they're immensely valuable and they'll be valued if you can find the right, right sorts of, um, settings to do it. And you'll improve the quality of, um, decision making and administration and understanding in those organizations. So, um, are lots of possibilities.
Benjamin James Kuper-Smith: Okay, great. Um, those are my questions. I don't know if you have anything else to add. Um... Yeah. [01:12:00] Yeah.
Geoff Cumming: really, uh, novel and interesting version of, uh, one of the things I suggest just towards the end, that is, uh, use your skills to do something a little bit different and, uh, you never know who you'll finish up talking to, what sort of things you'll, uh, be reflecting on, and, um, I bet there aren't many CVs with, um, bizarre podcasts with odd people from around the world, uh, uh, uh, attached to it.
And, um, maybe one of these days you can write a, a racy thriller where you pick bits out of all the best podcasts and put it together, but anyway, good on
Benjamin James Kuper-Smith: Well, thank you very much and thank you for your time.