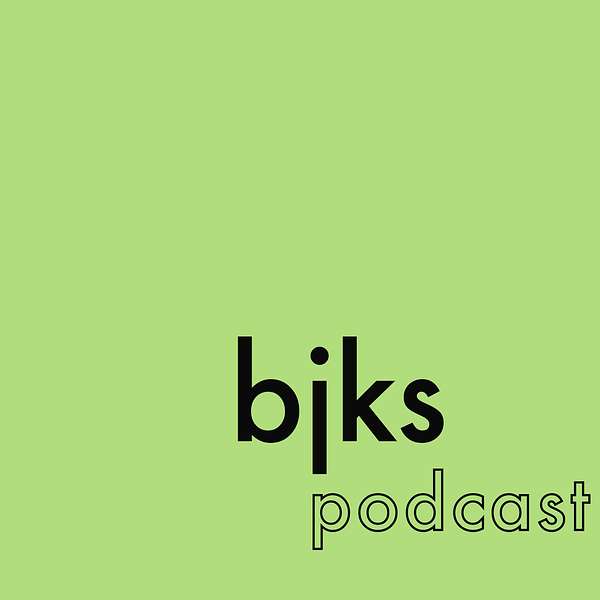
BJKS Podcast
A podcast about neuroscience, psychology, and anything vaguely related. Long-form interviews with people whose work I find interesting.
BJKS Podcast
70. Mona Garvert: Cognitive maps, fMRI adaptation, and computational psychiatry
Mona Garvert is Lead Research Scientist at Alena where she uses her background in cognitive neuroscence to advance computational psychiatry. In this episode, we talk about her academic research on the neural basis of cognitive maps, how she got into this topic, fMRI adaptation , and her recent move from academia to working at Alena.
BJKS Podcast is a podcast about neuroscience, psychology, and anything vaguely related, hosted by Benjamin James Kuper-Smith.
Support the show: https://www.patreon.com/bjks_podcast
Timestamps
00:00: How Mona started working on cognitive maps
15:28: Repetition suppression/fMRI adaptation
28:49: Start discussing Mona's paper 'A map of abstract relational knowledge in the human hippocampal-entorhinal cortex'
40:07: Are discrete and continuous maps different in the brain?
43:37: Start discussing Mona's paper ' Hippocampal spatio-predictive cognitive maps adaptively guide reward generalization'
55:50: Mona now works for Alena, doing computational psychiatry
Podcast links
- Website: https://geni.us/bjks-pod
- Twitter: https://geni.us/bjks-pod-twt
Mona's links
- Website: https://geni.us/garvert-web
- Google Scholar: https://geni.us/garvert-scholar
- Twitter: https://geni.us/garvert-twt
Ben's links
- Website: https://geni.us/bjks-web
- Google Scholar: https://geni.us/bjks-scholar
- Twitter: https://geni.us/bjks-twt
References & links
Mona's talk at TCPW: https://www.quentinhuys.com/tcpw/events/mona-garvert/
Where Mona now works: https://alena.com/
Barron, Garvert & Behrens (2016). Repetition suppression: a means to index neural representations using BOLD? Philosophical Transactions of the Royal Society B: Biological Sciences.
Clark & Wells (1995). A cognitive model of social phobia. In Heimberg, Liebowitz, Hope, & Schneier (Eds.), Social phobia: Diagnosis, assessment, and treatment.
Constantinescu, O’Reilly & Behrens (2016). Organizing conceptual knowledge in humans with a gridlike code. Science.
Doeller, Barry & Burgess (2010). Evidence for grid cells in a human memory network. Nature.
Garvert, Dolan & Behrens (2017). A map of abstract relational knowledge in the human hippocampal–entorhinal cortex. eLife.
Garvert & Gollisch (2013). Local and global contrast adaptation in retinal ganglion cells. Neuron.
Garvert, Moutoussis, Kurth-Nelson, Behrens & Dolan (2015). Learning-induced plasticity in medial prefrontal cortex predicts preference malleability. Neuron.
Garvert, Saanum, Schulz, Schuck & Doeller (2023). Hippocampal spatio-predictive cognitive maps adaptively guide reward generalization. Nature Neuroscience.
Klein-Flügge, Barron, Brodersen, Dolan & Behrens (2013). Segregated encoding of reward–identity and stimulus–reward associations in human orbitofrontal cortex. Journal of Neuroscience.
Knudsen & Wallis (2022). Taking stock of value in the orbitofrontal cortex. Nature Reviews Neuroscience.
Schapiro, Rogers, Cordova, Turk-Browne & Botvinick (2013). Neural representations of events arise from temporal community structure. Nature Neuroscience.
Stachenfeld, Botvinick & Gershman (2017). The hippocampus as a predictive map. Nature Neuroscience.
[This is an automated transcript with many errors]
Benjamin James Kuper-Smith: [00:00:00] So I, I saw in your CV that you studied molecular medicine and bioscience for your bachelor's. Um, that doesn't exactly sound like someone who wants to do neuroscience or psychology. I dunno. Maybe it is, but I was curious like, what, what, when you, you know, when you decided to do that, what, what do you think you were gonna do?
What did you want to do with it?
Mona Garvert: good question. So when I started out, I was just really interested in understanding how the human body worked. I think that was my initial motivation. So I considered studying medicine, um, but I was really interested more in the research side of things rather than in the applied, you know, treating patient side of things.
So I thought molecular medicine was a good match, but I have to admit that I discovered quite quickly that it was really very molecular and that wasn't quite the level of explanation that I had hoped to
Benjamin James Kuper-Smith: Okay.
Mona Garvert: But very luckily, pretty early on, I think in my second semester, I had a course on neuroanatomy, and then I was like, okay, this.
This is what I want to [00:01:00] do, this is what I want to study. So then I essentially, uh, tried to figure out a good way of getting there. So I did two years of molecular medicine and then went abroad for a year to France, studied essentially similar things, things biosciences and ly. So I have, I would say like grounding in biology.
So that's essentially my ultimate background. And I think that's actually been good because then I kind of oriented myself more towards cognitive neuroscience. But I think it's a good starting point if you start kind of from the basics of, from a good understanding of, of the biology and, and neurobiology in particular.
But yeah, I, I pretty quickly realized that actually what I wanted to do was neuroscience. And so I then, uh, turned away from this molecular medicine a little bit and did a master's in,
Benjamin James Kuper-Smith: But then you did psychology, right?
Mona Garvert: yeah, I did. It was a program called Neurocognitive Psychology. It was quite psychology. Heavy [00:02:00] indeed. Yeah. But I did my master's project actually in a neurobiology lab, so I didn't completely forget my roots at the time.
I did, I studied, uh, visual coding in the retina in ax lotto at the Max Funk Institute in Munich.
Benjamin James Kuper-Smith: pretty biological.
Mona Garvert: Yeah, that was pretty biological. Uh, it was really fun. I had a great time doing that, and I thought that's what I would be doing for my PhD as well. I thought I would be doing PhD in maybe systems neuroscience or something, but, uh, that turned out not to happen.
Benjamin James Kuper-Smith: Why not? I mean, so from, from what I understand, you did the, what, what I always think seems like the coolest PhD in the uk, at least for neuroscience, uh, where you have rotations in the first year, you can try out all sorts of things. Were, you're supposed to try out different things. So what, what were your rotations there?
Mona Garvert: So my first rotation was in the lab of Dimitri Coleman. And I studied, uh, I used optogenetics [00:03:00] to look at oscillations in the hippocampus. Uh, so this was when I still thought that I would be doing, you know, neurobiology, essentially neuroscience and maybe animal models studying computations in the brain.
But then I thought maybe I should give cognitive neuroscience another chance. You know, I was in, at ucl. UCL has the fill, which is one of the, uh, most renowned institutions for neuroimaging, uh, cognitive neuroscience. And so I decided to do my second rotation there. And that really did change everything for me.
It was like such a fun environment, a good place to be, and I was like, okay, this is, this is where I want to do my PhD. So for me, this program of having rotations was really great. I think I would've ended up in a very different place and probably been less happy doing my PhD than I was. So I really recommended for people who are not a hundred percent sure, even though I guess I thought I was quite sure what I wanted to do, but it turned out that this other field was an even better [00:04:00] fit.
I did my second rotation with Ray Olan, uh, and my third rotation with Cal Friston. So I stayed at the fill for both of those rotations and also had made the decision quite quickly that I would then stay there for the rest of my PhD.
Benjamin James Kuper-Smith: I guess it helps if Ray Car try and convince you that cognitive neuroscience is a cool thing.
Mona Garvert: Yeah,
Benjamin James Kuper-Smith: I guess it worked.
Mona Garvert: exactly. It was, it was good. I mean, it's also really, I think, an important question you have to ask yourself when you decide what kind of PhD you want to do is what you want your every day. Life to look like, right? So it's really different to do cognitive neuroscience from doing neuroscience in the wet lab.
So in a wet lab, you spend your day. So the thing that I struggled with a bit is that there was a lot of kind of fixing technical issues, you know, because the recording rig has like noise in the system and things like that. Whereas in the cognitive neuroscience lab you spent, uh, you spent your time fixing bark in your code.
It's a very different, you know, it is still like solving problems every [00:05:00] day, but it's just a different type of problem. Uh, and so I would still always liked this, the level of understanding you would get by studying essentially animal models. So that's what drew me into this field initially. Uh, but I felt.
I got a bit of that also in this cognitive neuroscience lab that I joined. So I was a PhD student with RA and, and Tim Barrens. Uh, and so we really tried in my PhD and in, in Tim's lab particular to use neuroimaging methods to look at population coding. So to really try and answer more mechanistic questions, uh, with those tools in the human brain.
And so I felt like in the end I got, you know, to have this kind of mechanistic understanding that I was really looking for, uh, and not having to fix my rig. So like
Benjamin James Kuper-Smith: Yeah. Yeah,
Mona Garvert: working with code [00:06:00] more than, uh, with ethos recordings. So it was great. I had a good.
Benjamin James Kuper-Smith: yeah. Yeah. It, it sounds really cool. I mean, the, um, I mean, I think most people would try and use FM I in a mechanistic way. It's just difficult. Right. Um, but I, I guess it's just still really cool that they. I guess in Tim's lab. Really try and use it that way in a,
Mona Garvert: Yeah. I mean, Tim also has this kind of parallel strand of doing animal research in his lab or through collaborations as well. Right. So he has always really had this interest as well. Um, so that was great for me because I also really came from this more mechanistic, biological perspective. And so it was a good fit.
I.
Benjamin James Kuper-Smith: okay. I always assumed you did your PhD with Tim Barrons because the, at least the first paper of yours that I know of is the eLife paper where Tim is last author, and it sounds kind of like, you know, it's more similar to the ESCO paper and that kind of stuff, [00:07:00] but,
Mona Garvert: No, I actually, before that I did a social neuroscience study, uh, where Ray was the last author that was published in Iran. And, uh, so we used similar methods, so I kind of transferred the methods from that study into this cognitive maps, uh, work that I did later on. But yeah, I was essentially co supervised by both of them.
I was part of both labs, which is was also great because you get to see really different, uh, cultures and learn from different people. So yeah,
Benjamin James Kuper-Smith: So were there any like obvious big differences you can name or was it more like, I dunno,
Mona Garvert: one big difference is that at the time Tim's lab was still very small. So I had like really close supervision by him, which was amazing. I could learn a huge amount from him, whereas Ray's lab was really established and very big and had lots of postdocs. And so there, like, it was really fun to kind of see the interactions between [00:08:00] people and learn from other people in the lab and to see what everyone else is doing.
So it was just a very different atmosphere for that reason. Partly,
Benjamin James Kuper-Smith: Which one do you think is a better learning environment? I guess, I mean, there's always this kind of question, right? That, you know, for example, I have to start thinking about, well, I have started thinking about post applications, that kind of stuff. And there's always like, part of the question is like big lab versus small lab.
Like, do you wanna be, yeah, I don't, I don't really know what the.
Mona Garvert: yeah, I mean, I, I think it depends a lot on, on you and how you like to work and how much independence you want to have. So I feel like I got the best of both worlds for being both in a small lab and in a bi big lab at the same time. Probably like the earlier in your career, the more you benefit from being in a smaller place where you can really learn directly from the pi, a bigger place is obviously can't really offer that because there's just so many people in the lab.
And so then, I mean, you can still learn a lot, [00:09:00] um, by, because it's like such a select of people that you can essentially attach
Benjamin James Kuper-Smith: I mean, it seems like there you would have more people, you could learn specific things from
Mona Garvert: Yeah, exactly. And yeah, the fill in general is just a place where you have like such, so much expertise, uh, in like different relevant fields Yeah. In anything really. And so, uh, you just, you know, then find the people that you want to learn from. And then it's nice to be embedded in this kind of culture, uh, of, of a nice, of, of a big place where you can. Just get all these different inputs. So I, I really think, you know, it has its pros and cons that both sides have, its have their pros and cons, and it's nice if you, I guess it perhaps, usually you would have this at different stages in your career where you would maybe initially start out in a smaller lab and then move for, to a bigger lab.
For a postdoc, that's probably not a bad strategy because then you, at that stage, once you have [00:10:00] acquired a lot of skills already, then it's great to be in a place where you have a lot of resources, right, from where you can just implement the studies. So you have designed yourself. So that's, I guess, sometimes harder in a small lab because in the small lab, the pi, you know, has funding for specific projects.
And so there's perhaps not always, but perhaps more of an expectation that a postdoc or PhD student just executes those projects. Whereas in a bigger lab, there's often, you know, funding available to do, to have a bit more freedom. So,
Benjamin James Kuper-Smith: Yeah. Yeah. Okay. Okay. Yeah, I'll, I'll bear that in mind. What I'm thinking about. I don't know, I guess it's probably gonna be random anywhere where when I end up, it's not like,
Mona Garvert: Well, I think, I think this is an important consideration. This is certainly something I would keep in mind when making this choice for postdoc, but what it means to be, uh, in a different lab. But I mean, there are many factors that play a role here, right? Location and,
Benjamin James Kuper-Smith: yeah, that's, that's something like [00:11:00] these things also whether you get, get a job or not, or funding scheme for whatever, right. Vitals, it just not work out. So,
Mona Garvert: Yeah, yeah. And do you, uh, want to stay in your research field or is it maybe a good opportunity to try something else? And, you know, all these, these
Benjamin James Kuper-Smith: I mean that's in a weird way, kind of what I'm at because I want to do cognitive neuroscience or some computational neuroscience with, you know, let's say very roughly the kind of things that you do in terms of like methods and that kind of stuff. But somehow I, we never got around to doing f m I in my PhD, so I never actually learned it.
Uh, so I think I, I think I figured out what I want to do, but not exactly, you know, acquiring the skills necessary to do that. So, um,
Mona Garvert: well, I mean, it's a, it's a, it is good. If you know what you want to do, then you can try and find the place where you can best learn how to do it. But I, I guess you were in an unlucky, you did your PhD at an unlucky time, right? With Corona and everything. [00:12:00] It
Benjamin James Kuper-Smith: Yeah, that was part of it. It was, I, I could maybe officially put the blame on that, but technically it was also a, a bit of a doing too many side, basically my side projects got finished first, which is not the best idea. Um, and then, yeah, by the end it was like, okay, we don't have that much time left. We can like, you know, cram an fm I study into this, but is that really the way forward?
Mona Garvert: Yeah, these also take a long time, usually.
Benjamin James Kuper-Smith: Exactly. So it was like, probably better to just do a smaller study and do it well rather than, you know, do a half baked fm I study. But yeah, so it was, I mean, cover didn't help, let's put it that way, or that our lab moved during the pandemic didn't help either, but. There, there would've been a way. Um, it's not entirely down to that.
Yeah, no, I mean, yeah, so it's just, just a weird position where I feel like I, from my post actually, there is specific stuff I want to learn. [00:13:00] So yeah, I don't know. I'll figure something out.
Mona Garvert: much time do you have left? When do you need to make that
Benjamin James Kuper-Smith: Uh, um, I mean, so technically, uh, so like officially right now, four months until the end of my PhD, but there might be a bit of, bit more money afterwards for a few months. Uh, yeah. And I also don't really feel the, uh, need to like start my postdoc, you know, the second after I finish my PhD. I don't mind having like a bit of time in between.
So I don't know, let's say in a year or something, by the latest, I should probably have a job again.
Mona Garvert: Okay. So I guess it is something that's actively on your mind already if you're in the final
Benjamin James Kuper-Smith: Um, yeah, it kind of should be, at least, I think I'm still vaguely putting it off, but Yes, yes, it is on my mind.
Mona Garvert: cool.
Benjamin James Kuper-Smith: But yeah, but I guess in that context, like, I mean, I'm sure I'm not the only person who wanted to do F m I and then didn't or maybe realizes, [00:14:00] uh, after the PhD that FMI should have been a good thing within a smaller lab where I can, you know, or does it not matter?
I just need someone to like, to be upfront at, you know, oh, I need to learn
Mona Garvert: Yeah, exactly. Ideally you need to find someone you can learn this from. Uh, and this could be in a small lab, it could be the PI directly or in a bigger lab. It could be a post or someone who has experience that you don't have, that you just pair up with. Um, so I think there are many ways in which this can work successfully if you're embedded in an environment like the Phil or, um, I was also at Oxford for a bit after my PhD at the where again, you have like a huge amount of expertise.
It's, you know, concentration of people that have done this for many years and I've really developed some of the technologies to analyze with my data. Uh, then I think those are good places. To learn, uh, and then it matters less perhaps what size exactly the lab is, so that the size in itself is not, [00:15:00] not the only factor, at least I would say.
Benjamin James Kuper-Smith: Okay, cool. Enough career counseling. Um, although, I mean, I enjoyed it, but I think people came more to listen about your research than my. Struggles. Um, yeah, I thought before, oh, maybe I left. From what I understand, like I guess you, at some point during your PhD started working on the discrete maps paper that came out on eLife.
Um, yeah. I thought maybe actually for a few minutes, bef kind of before we talked, start talking about the papers themselves. I think it might be useful to talk about one of the methods you use in both of the papers we'll talk about today, which is repetition, suppression, or FMI adaptation. I think it might be good to get that outta the way before we kind of interrupt the actual, uh, paper talk in that sense.
Because I mean, for me it's something I'd kind of heard about before and then, you know, I know vaguely how it works, but, uh, not in any detail. So maybe, yeah, to make, [00:16:00] to make sure I, I or the listeners are not too confused. Uh, when they talk about the papers, maybe. What is repetition suppression or fm i adaptation, by the way, which term do you prefer now?
Mona Garvert: Either is fine. Uh, maybe f r adaptation.
Benjamin James Kuper-Smith: Okay. What is it and how does it.
Mona Garvert: Yeah, so. Adaptation or repetition. Suppression is essentially a method to look at the representation of similarity between stimuli. So how similarly are stimuli represented in the brain? And so it relies on the fact, or, or it exploits the fact that the rep, the repeated activation of a neuron, often leads to a suppressed response.
So, for example, in the visual cortex, if I show the same visual stimulus twice, then the second time round, the neuron often responds less strongly than it did the first time round. And so this can be observed in single cells. But, uh, intriguingly we can also see this in the bolt response. So this means that, for example, an area that [00:17:00] encodes a stimulus feature, let's say a visual feature about stimulus X, then the bold response in this box that represents this information would show a suppressed response, uh, if the proceeding stimulus was exactly the same one as the current one.
So let's say X followed by x. Now if the proceeding stimulus activates a different set of neurons within, uh, Oxo or cross voxels, then you wouldn't see this kind of suppressed response. So it allows you essentially to quantify the representational overlap between two stimuli because, so the underlying idea is that the larger the suppression is, the more overlapping the representations.
So essentially what you would do is you would show stimulus X and analyze it as a function of what the proceeding stimulus was. But yes, if the proceeding stimulus is exactly the same, then you would get the maximum amounts of suppression. If the proceeding stimulus is represented really differently, so by different population of neurons, essentially, then you would [00:18:00] expect no suppression.
So a larger response to the stimulus eggs. And if you have a stimulus that's represented somewhat similarly, then you would expect somewhat intermediate amount of suppression. So it allows you essentially to look at how similar the representations are between different stimuli. And so, um, this is great because it really goes beyond saying this area is involved in, or it, this area becomes active.
When I see stimulus acts, it's really looking at the representation of content, so to say, of the boxer or of population of neurons. So it allows you to compare the similarity of
Benjamin James Kuper-Smith: One question I had was you were saying that is kind of what counts as the same stimulus. I mean, maybe that's very much the experimental question you look at, but you know, I mean, What, what?
Mona Garvert: Yeah, I mean that is often, so, um, I guess what you can do, and what we did in this eLife paper for example, [00:19:00] is we hypothesized that we would see a certain gradient of similarity. Between different stimuli. And so then essentially you look for that, right? So you, you assume that you have more similarity between two stimuli compared to two other stimuli.
And then you try and see which areas of the brain show that particular pattern. Uh, and so obviously not in the entire brain would show it because not the entire brain would encode this particular feature of a stimulus. But in this way, you can essentially probe which parts of the brain encode which stimulus features.
For example, if we had looked instead for a perceptual similarity, then the hypothesis that we would have about similarity of, of stimulus features of of stimulus representations would be quite different. So then we would probably see more like perceptual, like visual areas showing this kind of response.
Benjamin James Kuper-Smith: The one, one question I had was, uh, I mean you, so maybe for new listeners, uh, I'll put references to [00:20:00] all the papers we discuss in the description of the podcast. Um, so whilst I was reading your review paper with this, you know, I mean you and you do go into this, um, but I kept having this question like, why does this happen?
It seems like such a random, I don't know. It, you know, often you hear about things you go like, oh yeah, that makes sense. But in this case I was like, that seems a bit arbitrary. Like, why wouldn't you wanna just fire less? Just because it seen something. Yeah. Can you, can you comment on that a little bit?
Like kind of what other, I guess there are a few different ways and why it might
Mona Garvert: Yeah. Why? Yeah. I mean, it's not, not entirely understood why exactly this happens. Um, there are different ideas. So for example, one idea is that it's related to. Prediction. So that's like an expectation essentially, uh, effect. So if you expect the same thing to happen again, you know, it's, it's related to this predictive, uh, predictive coding idea of brain function, then you would have a smaller response in a neuron compared to a situation where something novel happens that was unexpected.
And so this could be [00:21:00] one explanation, could also just be like adaptation effects. So this is actually what we observed very much in, in the first study, the first neuroscience study that I ever did on like neuro recording and the retina. You, you see it in the retina, right? So if you re, if you stimulate the retina again and again with the same kind of visual stimulus, then you see a suppressed response.
It's just like adaptation effects. Partly, you know, I guess in the, in the restaurants, the reasons may be a little bit different. You want to adapt to the kind of range of, of contrast, for example, that you. That you see. But, um, it's, it's a very common phenomenon. Another explanation potentially is that, uh, response just changes slightly.
So you have like an accelerated response, for example, which then leads to a change in the bold response so that the neural response essentially peaks a little bit earlier, the second time round. Uh, and therefore you have a reduced bold defect. So there are various kind of mechanistic explanations for why you see this.
Um, we don't really a hundred percent [00:22:00] understand what, what the underlying mechanism is, but we can still exploit the phenomenon for looking at representational similarities, which is what we did here.
Benjamin James Kuper-Smith: Is the, I mean, so you mentioned that the, in this case there's, you know, fairly clear, there's fairly clear evidence that this works at a single cell level and that kind of thing is the mechanism. I'm just curious, like is the mechanism at the single cell level the same? Like is it's basically the, if we see suppression at the cellular level, like individual cell level, is that the same mechanism that explains what happens at the kind of larger scale level with thousands of neurons in an F MRI study or
Mona Garvert: Yeah, I mean, so there have been studies looking at the bold response, single cell activity, uh, and uh, local field potentials in parallel. And so they've found that, actually, you know, what explains the bold response best is not necessarily the single cell activity, but it's more like the local field potentials, the, [00:23:00] the Paris synaptic activity.
Benjamin James Kuper-Smith: PERS synaptic activity, meaning?
Mona Garvert: So, um, essentially like things that are changes in the field, potential that happen around the synapse. So not, not necessarily this kind of action potential that you have within a neuron, but, uh, yeah, changes in the field potential that are essentially the sum of multiple neuro activities. Uh, and so this seems to be more closely related to the VUL response.
So in that sense, there is not a hundred percent direct translation between this reduced response you see in the neuron itself in terms of a reduced kinda action potential. And the change in the bul response that we pick up was fmri. It seems to be more about the combined activity of many neurons that are kind of locally within a particular voxel.
Um, but again, like we do see signatures in the bold response that are similar to what we see in the neurons. And so therefore we, we assume that there's some correspondence between the processes.[00:24:00]
Benjamin James Kuper-Smith: Yeah, so one that kind of big question I had. When after kind of finish reading your paper is that it seems to me like there's a, I mean, to me, to me it felted almost a little bit like an elephant in the room kind of question that you kind of addressed, but not exactly. And I'm just curious like what you think about this is that you mentioned that the time scale at which, uh, this suppression happens can vary greatly and sometimes it can vary, you said up to a day or something for some sort of, I dunno exactly what, but the, the, the span is quite big.
So the kind of question I had then, it's like how do I know, or how do I have any basis for believing that the phenomenon I'm interested in has a time delay that I can study with F M R I? And there was this big moment of like, like every time you run a study like this, is there just a possibility that you'll get no results and it's just completely uninterpretable And, uh, I don't know, like when I, when I read that the, the time delay can vary so greatly and.
Yeah, there was just part of me that [00:25:00] thought like, I'm not sure this, it's a really risky method to use almost. It seemed to me.
Mona Garvert: Well, I mean, I think the, the usual time scales are more like, well, it depends on the part of the brain you're looking at really. And that's very consistent. Of these kind of hierarchies of timescales have been found, uh, for example, in the monkey brain by, uh, people, uh, who've looked at essentially, uh, you know, how long information is, is maintained in different parts of the brain.
And so what we can see there is that in visual corsis for example, the response goes down really quickly. So you have short time scales, whereas the higher you go up along the hierarchy of the. Towards the frontal cortex, for example, the longer the timescales are. And so this is consistent also with this idea that, you know, like working memory needs to maintain information with working memory, maintain information, uh, in the brain.
And so this is something that we can see with F [00:26:00] R I adaptation. So Miam, client Fluger and Helen Barron did the study published in General Neuroscience a while ago where they looked at this and they found like adaptation effects, uh, in the visual cortex to de decay quite quickly. So there they would see adaptation, you know, on really short time scales of like a few hundred milliseconds.
Whereas in the frontal cortex you would still see adaptation effects, uh, on a much larger time scale of a few seconds. And so, um, the studies that I have run have essentially kind of assumed that this is the timescale that we're working with a few seconds. Uh, and that seems to have worked reasonably well.
But I mean, of course there's always a risk that it won't work.
Benjamin James Kuper-Smith: Yeah, I mean, so to maybe specify, the concern I had was it was more like, you know, so you said like you, you present stimulus A and then stimulus B, and then for example, there would be no suppression, but if it's a, and then a, then there would be suppression. Now if that suppression lasts enough seconds, doesn't [00:27:00] it just, you know, adds suppression for the next,
Mona Garvert: Yeah. So that is obviously,
Benjamin James Kuper-Smith: suppression effect, right.
Mona Garvert: yeah, I mean, that's a valid concern and in order to prevent that, you need to really make sure that you randomize your stimuli properly. So in case there is such an effect, but it kind of disappears because it's, um, there's no systematic relation between what, what comes next as a stimulus that thereafter because Yes, and you, you would assume that it kind of carries over to the next stimulus and potentially even further down.
So you want to avoid that by randomizing properly.
Benjamin James Kuper-Smith: Okay, so just design your experiment properly, then
Mona Garvert: Yeah. That's really important. Yeah.
Benjamin James Kuper-Smith: Okay. Just, just for this kind of study. Yeah. Yeah. I don't, I mean, this isn't in a way that much of a question, but I somehow I was just like so surprised that it works. So it seemed vaguely miraculous that you'd have like this complex functions and then.[00:28:00]
It just seemed to me like there would always be some, because I mean overlap here, right? We're not talking about, I mean, in the visual system we could talk about overlap between, you know, use the same color and something, or you use the same shapes or whatever, right? I mean, sure you can vary that, but it seemed to me at some point, like if you use like complex tasks and people have decide between options, there's always gonna be all sorts of overlap between things and I don't know, I was just, I was just like, like, this works
Mona Garvert: Yeah. I mean,
Benjamin James Kuper-Smith: you get boxes with thousands of neurons and then somehow, I don't know, like that you can, that that it measures that, that precisely just always really surprised me when I Yeah.
Read these
Mona Garvert: it? I'm also still really glad that it worked, to be honest, and that it's so specific, right In this eLife
Benjamin James Kuper-Smith: Yeah, exactly.
Mona Garvert: found it specifically in that region in the Wales. So maybe I should give a little bit of background information. Uh, so what we were looking at in that paper is essentially we were interested in how the brain represents relationships between [00:29:00] objects, pretty much.
So we know that the brain forms maps for things that can be mapped. Onto continuous dimensions like space or time, especially for space. This was very well known from like the seminar work and play cells and grid cells and so on. Uh, and then Alexandra Cinco did a study around the time when I was in Tim's lab as well, where she essentially demonstrated that the same organizing principle seems to also organize other types of relational information.
And in her case, she essentially designed stimuli, um, bird stimuli that could vary in terms of the length of the neck and the length of the legs. So this way she, she spanned like a two-dimensional space and she found that the same region that encodes distances in physical space also seem to form a map of this two-dimensional birth space.
So that was really interesting, uh, because it showed that this organizing principle, like places and grid cells might have a much more general [00:30:00] role and also map other types of nons spatial relation information. But, so then what we were thinking is that, um, there's other types of relational information as well that cannot just be mapped onto two dimensions, right?
Like length of things and, or distances instead, you know, we might have associations between objects that we regularly experience in our environment, for example. So these are like discrete associations between things. And we just wondered, uh, if indeed this is such a general organizing principle, would it then even go beyond these continuous dimensions and also map relationships between discreet relations such as.
Objects. And so to test this, we essentially train people on object sequences that were generated according to a random walk along a graph structure. Uh, and then we use this method that we've now discussed at length to look at how afterwards these objects are represented in the brain. And we hypothesize that if indeed this [00:31:00] organiz principle is so general that it even maps these discre discrete relationships between things, then maybe we should see that objects that are closer to each other on this graph structure should also have a more similar representation and objects that are really far away from each other.
On this graph structure, we should have a more dissimilar information, uh, representation. So in other words, do we see a map of the SPR structure in the brain? Uh, and yeah, we looked for it and we found it in exactly, uh, that like hippocampal brain region that also is known to map space. And so this to us means that this organizing principle is even more general than we thought after Alexa study was published.
So it's not even just about things that can be mapped onto continuous dimensions. It really is about mapping relationships between things very broadly. Uh, and yeah, I mean, it was a bit risky to be honest at the time to study this because we didn't necessarily have, like other than this, this the theoretical [00:32:00] idea that it should work because, uh, if indeed, uh, it's like a, such a general principle, then we should be able to find this.
Um, yeah, that we just essentially set up to test if we would. We did. So I was quite lucky, I guess.
Benjamin James Kuper-Smith: there's lots of theoretical things that should
Mona Garvert: Yeah, exactly.
Benjamin James Kuper-Smith: I was like this one did. Um, yeah. Maybe to make that task a bit more. Graspable or understandable. Um, can you maybe briefly describe kind of what the, as, so you mentioned this like discreet sequence of, of items or events or, um, yeah. Can you maybe ex explain a little bit what the task was, just just so I have a bit of a better idea of like what people were doing and then what you were, how you were analyzing that basically.
Mona Garvert: Sure. So essentially people saw just one object after the other on the screen. And they had to do a cover tasks. That means there was a task that was completely unrelated to what we actually [00:33:00] wanted to study. So they had just had to press one button if the object faced in one direction and the other button if the object faced in the other direction.
And so the, this mapping essentially had to be learned over time. And so this is what people thought they were doing. They thought they kind of, their task was to just be as quick and accurate as possible in responding which direction the object faced. Now, what they didn't know, and no one figured out is that the sequence in which the objects appeared was not random.
It followed this underlying graph structure. And so our idea was that hopefully the brain, even if the person don't consciously realize, uh, that, that this is what's happening, that the brain would extract this underlying structure. Uh, and so we on the next day put people, uh, back into the scanner and again presented them with object sequences.
This time the sequence was not, did not follow the structure. It was completely random. Perfectly counterbalanced every object followed every other object equally, often. And so this then allowed us to use this, this idea [00:34:00] that, you know, the response to an object should really depend on what other object was shown just before.
So if the other object shown just before is close on this underlying graft structure, then we should see a suppression. And if the other object that was seen just before is really far away on the graph structure, we should see less suppression. And so this is essentially then the signal that we were looking for.
And in the scanner, again, people just did a completely independent task. The objects just had little patches on there sometimes, and occasionally people had to report if an object had this patch or not. So again, completely unrelated to the thing we actually wanted to study.
Benjamin James Kuper-Smith: Yeah. Why did, so one, one question I had about this task is kind of why did people have to learn this implicitly? Like, why was it important that they didn't know what the structure was?
Mona Garvert: Um, I am not sure that's necessarily important. So in the follow up study that we're gonna talk about, I guess as well, um, it was not implicit, people have quite explicitly learned the relationships [00:35:00] between objects. We just thought it would be even cooler if we can see this in the absence of any explicit awareness, right?
Because the spatial knowledge that you acquire tends to be mostly explicit and you know, you're consciously aware
Benjamin James Kuper-Smith: Like, you know, this object is next to that
Mona Garvert: Yeah, exactly. You know, if I want to get from here to the supermarket, then I need to go left, right, left, uh, then I'll be there. So that's like explicitly accessible, this kind of knowledge.
Uh, and. I guess maybe that was a bit risky looking back, but like we were saying, or we were thinking like, if this is such a general mechanism that just organizes any type of relation or knowledge, then it should also work if you're not even consciously aware of these relationships. Uh, and that's what we found.
Benjamin James Kuper-Smith: I mean, Just a brief aside here, what's your thought on risky versus safe experiments? Because yeah, I mean, in hindsight it's, yeah, because you could have also, you know, said, okay, let's first figure out whether it works when people explicitly know this [00:36:00] and then, you know, take a step by step. But instead, it seems like you went, um, you know, for, for the, one of the most, one of the harder tests of the theory straight away.
Is that, is, was that a good strategy? I mean, because it could have not worked right? Like,
Mona Garvert: have not worked. I mean, and there were quite a few pilot experiments that we did before. Right. We didn't go straight for the imaging study, so we did find a behavior signature of this. Map like representation first. Uh, and only then did we move to the imaging study. So essentially we, um, did like a priming task in the scanner where we wanted to see if the response times are modulated by distances on the graph.
And so I think, I mean, it wasn't completely out of the blue, right? So we were very much inspired, for example, by a paper by Anna Shapiro who had looked at the representation of community structures in the brain. And she was used to task a little bit like this. And so kind of we came, [00:37:00] kind of came from there and thought, okay, well maybe we can more directly test if this is even takes the shape of, of a map and.
Yeah, so the thing that, that didn't work, for example, one of the things that we tried in our pilot study is that we used fractals, like really abstract stimuli. And there we didn't see any signature in the response times and the behavior that people actually managed to learn this. And that's, it was probably just too hard.
People couldn't separate the different stimuli, whereas everyday objects, you know, you have so much familiarity with them and turned out that that was a bit easier. But yeah, in general, I mean, I just really like, you know, answering, uh, new questions and so that always involves taking some risks. I've always found that more appealing than kind of just making smaller, incremental changes to a paradigm or something like that.
It's, it's just, but yeah, it's, it's certainly risky.
Benjamin James Kuper-Smith: But I guess by that point you also, I mean, I'm thinking, I mean, you know, I guess the annoying thing [00:38:00] about science is that you have to do it in the context of academia and that there's these like, you know, jobs and stuff to think about. But I guess by that point, for example, you're already. That was after you already did the paper that the study that appeared in neuron afterwards.
Uh, right. So like, I guess you already, did you already know that there was like, okay, I have like, if this doesn't work out, I always have this other study or,
Mona Garvert: Yeah, I mean, I was, at that point I was quite lucky. So I had published my master's, uh, project, uh, in neuron as well. Then I had, uh, a smaller neuro image project from one of the rotations I did, and then this other, uh, neuron paper. So I was okay, you know, even if this hadn't worked out, I would've done an okay PhD, I think.
And so I thought I might as well
Benjamin James Kuper-Smith: Okay. Yeah, yeah, yeah. Okay. And I guess your co-authors, Ray Olan and Tim Barrens, they're also okay. They,
Mona Garvert: I mean,
Benjamin James Kuper-Smith: if this thing wouldn't work out, they were doing all right.
Mona Garvert: I think Tim is known for doing risky things and ambitious things, right? So it's like, [00:39:00] uh, and. For me, that was always very appealing. I wanted to fund new things and um, was happy to take the risk. But yeah, it is certainly risky. These things also then often take longer, right?
Because you need to have this extensive pilot period before you even go into the scanner. This is very different if you take a paradigm that has already been developed.
Benjamin James Kuper-Smith: Yeah. I mean, I know people who spent the entire PhDs trying to get this paradigm to work, and then it doesn't. Right. I mean, yeah. But I guess having the backdrop of stuff that already worked makes
Mona Garvert: yeah. And I mean, uh, it was also really lucky, I think, to work with someone like Tim, who just kind of stretched so much optimism and excitement,
Benjamin James Kuper-Smith: Okay.
Mona Garvert: you know, so that I, I was never really worried about this, to be honest. It was never, we just tried to do the best work and this is what it took.
Benjamin James Kuper-Smith: Okay.
Mona Garvert: And he was always [00:40:00] convinced that it would work out, and so that somehow calmed me down as well, I guess.
Benjamin James Kuper-Smith: Okay. Um, I'd like to talk a bit, bit, a little bit more about kind of cognitive maps and in particular, I mean, what's, what's kind of maybe the, as you mentioned, the, this kind of whole research area started in physical space and then, yeah. I guess over the last, let's say like seven, eight years or something now, um, has moved into increasingly more abstract spaces, um, and that kinda stuff.
I'm just curious, like what's the, in a way, this feels like a really slightly weird question to ask, but like, what's the difference between a discreet map and a continuous map? I mean, obviously what it maps to, right. But, um, I don't know. Is there like any fundamental, like neural difference or, yeah, maybe Like what's the relationship between these two?
Mona Garvert: Yeah, I mean, so the way I think about it, Is that there isn't actually a [00:41:00] fundamental difference.
Benjamin James Kuper-Smith: Mm-hmm.
Mona Garvert: So one thing that we found in this eLife paper and that I also then, um, that we confirmed in a follow up paper that is coming out soon now, is that actually what describes those relationships best.
It's not necessarily like a mapping into a Euclid space, but it's more something that's called the successor representation. So essentially the idea that you don't represent like the current state, even in space, it's like the location where you currently are, but more as like the likely future states you're gonna find yourself and given where you currently are.
And so if you think about it in this way, then you can really kind of compute it for any type. Relational structure. It doesn't matter if it's a continuous space or if it's a discrete space. As long as you know how things are related to one another, you can compute these kind of likely future states. Uh, and there's some good evidence [00:42:00] that this is perhaps also what the hippocampal, antiviral system is doing, but it's not so much about like telling you where you are right now, but telling you where you are likely gonna be next given where you are right now.
And so if you think about it in this way, then really continues and, and discrete spaces are like not fundamentally different from each other.
Benjamin James Kuper-Smith: Yeah, I guess it's just that, yeah, they, they might just seem a little bit different because in continuous space, the next likely state is basically where you are, just slightly around
Mona Garvert: Yeah.
Benjamin James Kuper-Smith: Um, so it seems like, yeah, I guess the next step is Okay. Yeah.
Mona Garvert: I mean, you would essentially define states, right? So transitions between states is what we're looking at. And so in, in our eLife paper, one state was like an object. And so you would transition from one object to the next. And so that you can kind of conceptualize this transitions between different states in continuous space.
You know, a state is a particular location [00:43:00] in space and then you move to the right. And so that's the next stage. So if you think about it in this way, then again, um, you can kinda formalize it very similarly.
Benjamin James Kuper-Smith: Okay. Yeah, that's, that's a good answer because yeah, I wasn't quite sure like what the, you know, sometimes you have a question and you, you, you, you expect, it's like, is it this answer or that answer? Right. Um, you have like certain, um, few options you think the person might say, but in this case, I wasn't entirely sure like what direction the answer would
Mona Garvert: Right.
Benjamin James Kuper-Smith: Okay. Well that was, that was interesting. Yeah. Do, yeah. I guess you, you already mentioned the other study that, uh, I mean, I've read as a preprint, but you said it's gonna come out soon or I guess the time that this is published. Um, so maybe, uh, yeah, do you want to briefly intro, I mean, you already mentioned briefly kind of what that was about, but Yeah.
Uh, a bit, a bit more formally kind of what, what was that paper and, uh, what did you find?
Mona Garvert: Yeah. [00:44:00] Um, so after this eLife paper was published, essentially we knew that the brain forms maps of things, right? And so one follow up question for me was, Why, like why is this such a, why is this a universally good way of representing things? And I think one answer to this question is because it's really useful to know how similar things are to each other.
Uh, and it's useful for like generalization and inference. If you find yourself in a new situation, for example, that you've never been in before, like how do you know how to behave well, you know how to behave because you've usually been in somewhat similar situations previously. Right? So I've never been on your podcast.
I've never been on any podcast to be honest. And so, uh, like how do I know what to talk about and like, what's appropriate here? Um, well, so I guess what I'm doing right now is I'm trying to think about similar other situations I've been in before. Like I've given talks before, I've talked to [00:45:00] other people about my work and, uh, and my motivation for doing things before and so on.
And so I'm essentially transferring the rules that I've learned in those other situations to the current context. And so for me, that seemed like one likely reason for why you might want to form maps in the first place. And so we then went on to study this more explicitly and try and understand like how this works in the brain.
How do we actually use maps to inform our choices, in particular, in situations that we have no direct experience with. To set this up, uh, what we did is we had people learn a map of things. In this case, it was, uh, a spatial map. So people navigated around the virtual arena, uh, and every time they approached a particular location, a certain monster would pop up.
So over time, as they navigated around the space, they would realize or they would hopefully, or they were instructed to learn where each monster lives, so to say, so they were explicit. In this case, as I said, this [00:46:00] was very explicit. We explicitly instructed them to learn the monster locations. And we then put people in the scanner to look at the representation of those monsters in the brain using the same technology that I described before.
And then we put them in the scanner again on the next day and gave them a decision making task where they could win rewards. And they were explicitly told that how many points they would receive for selecting a particular monster would depend on its location in space. And so what this means is that if you're clever, you're gonna use your spatial knowledge to inform your choices.
Right? So if I've
Benjamin James Kuper-Smith: you didn't tell them, like, you didn't tell them explicitly though, right? Like this monster gives you this many points.
Mona Garvert: no, this, they had to figure
Benjamin James Kuper-Smith: out.
Mona Garvert: Yeah. But so if they just selected a monster that gave them a high reward, and then on the next trial they were presented with a monster that's either near this one or far away from this one, then if they're clever, they would select the monster that's nearby to [00:47:00] maximize the rewards.
Right? And so this is a way of essentially using this. Map, like knowledge about distances between things to inform new choices. So explicitly actually had two monsters that were never presented during this choice task. So at the very end, we asked people to tell us how likely or what reward they believed they would receive when they selected this monster, which they never explicitly could select.
Uh, and we could, we, we found that people could actually do this quite well, so they could really use their knowledge about the relationships to inform their choice. So what's interesting here, I think, is that we found that there's not only the euc ukranian distances between the monsters that were represented in the brain.
But there's also something that's really very similar to this kind of predictive state relationships that I mentioned previously that were also represented in the brain. So just think about [00:48:00] the fact how, like how people learned these monster relations, right? So people could freely navigate around the arena to learn the monster relations.
And what this meant is that different individuals chose really different navigational trajectories. So some actually had paths that looked pretty random, but others had really stereotyped behaviors. So they would, for example, mostly walk around like the, the border of the arena, or they would kind of really systematically scan from top to bottom, things like that.
So that means that even though the spatial layout, like the distances between the mon was the same, the way in which or the order in which they were experienced was really different from one individual to the next. And so what we found interestingly is that. The degree to which, uh, people generalized the, the values later in the choice task was not only influenced by the spatial relationships between the, but also by these kind of temporal or predictive relationships that they had kind of experienced over the course of, of learning.
Uh, [00:49:00] and we found in addition that both of those types of relations were represented in the hippocampus simultaneously, but the representation of the spatial map over time became stronger. Which is interesting because it's a spatial map that was relevant for guiding choice, right? And also in the behavior, we could see that over time people like relied more and more on the, the spatial relationships as opposed to these, these other predictive relationships.
And we could see that mirror, uh, in the hippocampal map representations as well. And so maybe this goes into a bit too much detail now, but we found that this was essentially driven by the orbital frontal cortex. That seems to really compare the outcome that someone observed to the predictions based on the two maps.
Uh, and so probably, you know, the more consistent the outcome is with one of the maps, the stronger kind of the, the signal to the hippocampus was to kind of boost this representation as opposed to the other [00:50:00] one. And so, yeah, overall, um, this shows, I think that, uh, first of all, that we can have multiple maps represented in the hippocampus simultaneously, and that we can then rely on that flexibly, depending on the test demands to kind of generalize and inform our choices and situations that we haven't, that we have no direct experience with.
So this is like a mechanistic explanation hopefully, of how this might work in the brain. Uh, yeah. And so this was a project that I did, uh, together with. Christian Duller at the Max Frank Institute in Lae. Uh, but it was a really important contribution also by, uh, tan Sank, who is a PhD student in Eric Schultz's lab, uh, at the Max Frank Institute in Tubing.
Uh, and Niko Schultz was also critically involved. He's, uh, also at the Max Frank Institute in Berlin. So it's like a cross,
Benjamin James Kuper-Smith: Yeah,
Mona Garvert: plank collaboration and really a project that that relied heavily on collaboration because there's a strong [00:51:00] modeling component. And that was very much led by Eric.
Benjamin James Kuper-Smith: yeah, yeah. The, I mean, yeah, we don't have the, uh, time here to like, go properly into the modeling, but it, it, I mean, that, that was kind of, to me, the, I mean, that's the kind of what really makes that paper, right? That, that you can really, um, uh, yeah, figure out the past that people took when exploring the thing, whether the arena and then using that information, um, to create these temporal maps.
And I mean, you, I, I found this talk you gave, um, I mean, Quentin Hoy, he has this like talk series on his website, right? And you, uh, and you gave a talk there a few months ago or something, and this was like, one of the favorite plots I've seen was just, yeah, this like one screen where you showed all the exploration paths
Mona Garvert: Right. Yeah,
Benjamin James Kuper-Smith: screen.
I was like, that's so cool. Like, just seeing how the people moved through this. And
Mona Garvert: yeah, yeah. It's really, it's fascinating, like, like I'm really interested in inter individual differences as well, [00:52:00] and like that's like a prime example I think, of how
Benjamin James Kuper-Smith: yeah. And then yeah, being able to like, quantify that and use it as un super cool. And I mean, what I really liked about that is also that it, it occurred to me, and I mean correct me if I'm wrong, but it occurred to me whilst doing that is that, uh, In a way, the, the, the temporal map that you have, um, let's say, let's say you just do like a, a spatial map task or something.
The, the way that people explored it and the temporal map could have been seen as noise in the other experiment because it kind of wasn't really related to the task, but it still influenced how people behaved. And I th yeah, I guess, I mean, that's kind of what science is, right? You have a measurement, you have noise in it, and you try and figure out where the noise comes from.
And in this case, it, it's a nice principle of showing like that the exploration explained part of what could be considered noise.
Mona Garvert: And so the thing that occurred to me only later, to be honest. Is that obviously the spatial map is only [00:53:00] constructed based on the experiences they actually have in the environment, right? Whereas this, this, this transition structure that people learn through the exploration itself, that's like actual subjective experience.
And then you use your knowledge about space combined with those subjective experiences to essentially construct this Euclid representation. That's actually something that really occurred to me only in response to one of the reviewer's comments who, who raised this. And I, I think it's a really interesting thought that, that in this sense, those two maps are qualitatively somewhat different.
Uh, and yet they both influence choice to some degree. Degree, I guess partly because we had this embedded in space and people ignore the rules of space very well, so they can transform the subjective in experience into a subject, uh, into an objective map of space. It's the same for everyone.
Benjamin James Kuper-Smith: Yeah. But I guess that is also part of the explanation of why the temporal thing has an effect. Cause yeah. You that's the basis for
Mona Garvert: Exactly.[00:54:00]
Benjamin James Kuper-Smith: Yeah. Yeah. Yeah.
Mona Garvert: Yeah. And it was really cool that we could see both of those then represented in the brain and then this adaptive nature was also interesting. Right. And so this is something that we obviously didn't explore that would be interesting to see. Like what happens if instead of the spatial relations, the temple ones are relevant for the reward distribution, what we then see, you know, a shift more like a stronger representation of, of these subjective, predictive or temporal relations between objects, uh, as opposed to this patient one.
Things like that.
Benjamin James Kuper-Smith: Yeah, I mean, I also really like that you, I, I didn't, I, I thought that you just mentioned the, the RFC connection. I thought that was a good context because I mean, I've just, uh, like a few weeks ago I read this review by Joni, uh, Wallace, and. Someone else and in nature of is neuroscience that talks about kind of like two views of ooc as like map and value as those kind of being like the two main hypotheses of what ooc [00:55:00] does.
So it's really cool kind of to see that that came out in your study, which kind of combines both of them.
Mona Garvert: Yeah. Yeah, it really, yeah, it seems to, in our case, just almost some kind of evidence integration, right? So what's the evidence that the outcome I see here is, uh, generated based on the spatial layout or based on the temporal layout? So it really seems to integrate this information about the maps with information about rewards.
Benjamin James Kuper-Smith: Yeah, it's really, yeah, it's really interesting just because in that review they, uh, they kind of say like there's, you know, there's these two views of what FC does. And it's not really clear which one or how or why, but I guess that might be one suggestion
Mona Garvert: Yeah, exactly.
Benjamin James Kuper-Smith: in fc. Um, yeah. One thing that I guess is, I dunno how new it is for you, but at least I found out about it fairly recently, is that you, uh, I dunno actually whether this is [00:56:00] exclusive your job or it's part, part, or exactly the relationship is here, but that you are involved or working for a company now called Alina.
Um, so yeah, I'm just curious like kind of what the, what are you, maybe first what does the company do and then kind of what's your involvement with that and, and are you still working in academia or are you, have you gone completely to industry or, yeah.
Mona Garvert: So I work as a lead research scientist at Arena, which means that I'm leading a team of cognitive and computational neuroscientists mostly. Uh, and what we're trying to do is really essentially do computational psychiatry. So this idea that we can use cognitive neuroscience, cognitive computation, neuroscience to inform psychiatric treatment.
And so this is something that I've always been really interested in. So my research was much more basic in nature, in in many ways if we've, if we've discussed at length now. But I often felt like it would be really [00:57:00] nice if there was a more direct impact of what I'm doing somehow, right? If it's not just for the sake of gaining knowledge, but like, can this inform, um, for example, treatment.
Uh, psychiatric disorders in, in some way. And so Alina has made this its mission and the idea is that, uh, essentially to, uh, address the treatment assignment issue that we have in psychiatry. So one problem in psychiatry is that many people, That potentially actually have, uh, slightly different symptoms even are still classified under the same umbrella term of a particular disorder.
And so one problem with that is of course, that it's likely that this group of patients that has depression, let's say, is actually really quite heterogeneous in the sense that the underlying problem in the brain, the cognitive mechanisms that are 40, are really different from one patient to the next.
And therefore the [00:58:00] treatment that works for one patient doesn't necessarily work for the next patient. And that's in indeed what we see, right? So the treatment of psychiatric disorder is in many ways, very much like trial and error based. And so the idea here is that what we really need is not necessarily so much like a symptom-based classifi.
But we need a better way of characterizing the cognitive processes and then use that to assign treatments specifically, uh, and in, in a personalized way depending on the cognitive impairments that someone shows or the, the particular cognitive profile that, that someone has. And so that's what we're trying to do, and we're doing this specifically at the moment for social anxiety.
There's a very well established model for treating social anxiety. So it's a cognitive behavioral therapy model that was developed by Clark and Wells, and uh, it essentially addresses different cognitive functions. So one issue that people with social anxiety tend to have, for example, is that they focus their attention very much on themselves and in that way cannot learn about the environment as well as I should.[00:59:00]
Uh, and another issue is that there's like post-event processing. So there's like replay again and again of particularly negative social encounters and things like that. Uh, and so two individuals that are both diagnosed with social anxiety might have one or the other to a varying degree. So what we're trying to do is essentially develop cognitive.
That, uh, capture these kinds of processes to then based on performance and, and, and parameters that we extract from those tasks, assign the treatment more specifically. And the hope is that in this way, the treat the treatment will be more effective. Uh, and, uh, yeah, we, we kind of get around this issue of just having to do trial and error.
Right. Really precisely characterizing what exactly the problem is. Um,
Benjamin James Kuper-Smith: just briefly, why, um, if I can interrupt, like why is this a company then? Because it seems to me that this is, you know, this is what computational psychiatry
Mona Garvert: yeah.
Benjamin James Kuper-Smith: this is what lots of people do university. So [01:00:00] what's the added benefit of, or like, Yeah, because I guess if you're a company, you have to make money from it in a specific way and all these kind of things, like, yeah, why?
Why is it set up this way?
Mona Garvert: I mean, um, You have to make money, but to some degree there is also sometimes a larger availability of money. And I think some of these things are actually really not cheap, right? So we are now designing all these different tasks and in the longer run, what we want to do is to set up these really big studies into these treatment assignment, uh, problems, for example.
Uh, and so that. Can actually be quite expensive. So it can be good to have kind of extra funding. But the other thing really is that, uh, one goal of Alina is to really bring together expertise from different fields to kind of make this work well. Uh, and so this is something that I think often in, in academia is a little bit, you know, people tend to work in, in, in silos to a larger degree sometimes, but always, of course.
Uh, but so here we're really kind [01:01:00] of trying to get expertise, you know, from the technical team that, that implements these things. And then the cognitive neuroscientist computation neuroscientists, but also people like user researchers who've, who spend their time really trying to understand what the exact problem is that people with social anxiety, for example, face.
So how can we incorporate that? Um, and then we are also, uh, hoping to offer. A solution, like a product that can help people with social anxiety directly address this problem. And so this is like a, a digital men mental health app that essentially integrates these, these task-based characterizations of cognitive function with really targeted treatment options.
Uh, and so yeah, the idea is that by bringing kind of this expertise together, we can kind of move fast and build something maybe quicker that really addresses this, this very specific problem.
Benjamin James Kuper-Smith: Yeah. Yeah.
Mona Garvert: And yeah, we work very closely with academics though, so this is really not [01:02:00] an like, not a, not an usual industry in many ways.
I think, for
Benjamin James Kuper-Smith: I, I looked at the, at the team and I think half the people are recognized from ucl,
Mona Garvert: Yeah, exactly. Exactly. Yeah. So Quentin, who is for example, or Peter Latham and Vanessa Brown, uh, these are all
Benjamin James Kuper-Smith: I mean, as Zine Maya, I, I talked to Gareth Barns, uh, for my last episode. Yeah. She, she was with the FlexWare cast,
Mona Garvert: Yeah, we have a very strong UCL bias.
Benjamin James Kuper-Smith: exactly.
Mona Garvert: Yeah. And so it's really, it's a like very science driven endeavor, right? So really the idea is to, to do new science. We're developing novel tasks that really targeting those particular functions, but then feed that directly into something like a product that can kind of be used by people and hopefully help people.
Benjamin James Kuper-Smith: Are, are you still doing basic research? Are you still publishing papers or is
Mona Garvert: I mean, yeah, I, I'm still,
Benjamin James Kuper-Smith: you and
Mona Garvert: I still have an affiliation with [01:03:00] the Max Bank Institute in United Say, and they're like, uh, a few projects that I'm still working on as well in parallel. So I haven't cut all my ties with academia, I guess. Um, but yeah, I'm mostly focusing on that at the.
Benjamin James Kuper-Smith: Yeah. And I mean, I guess you, you started fairly recently, right? So, uh, or can you already say much about like what the work there's like, or is it still the excitement of starting and, uh,
Mona Garvert: Uh, I started half a year ago now, so yeah, I mean, I, I think it's, I think actually to be honest, that it is, would be really good for, it would've been good for me when I was an academic to know some of the procedures and processes in industry because I think that a lot more thought often goes into how you can optimize things for them to be more efficient and for people to be happier and motivated, and how [01:04:00] to essentially best achieve this particular goal that you have set yourselves.
Uh, I think this is really something that I would've benefited. When I was in academia. So I feel like I'm learning quite a lot, which is really nice. And I think the science we do is really interesting. I mean, there's no neuroscience in the sense, there's like no imaging at least directly, uh, through collaborations in the future potentially.
But at the moment we, we essentially develop behavioral tasks, but we're doing, like, we are not just applying tasks that existed out there. We're really trying to develop novel things so that very much targeted to these questions that we have. So that makes it like very interesting from a science perspective.
And yeah, it's kind of nice to work on something that has a tangible outcome at the end, you know, beyond a paper. We're also planning to publish papers and go to conferences and so on, but we are also actually building something that hopefully people will find helpful. [01:05:00] So that's, that's kind of rewarding.
Uh, it's nice to,
Benjamin James Kuper-Smith: you have an answer to this old existential question, academic style, like, why am I doing
Mona Garvert: Yeah, exactly.
Benjamin James Kuper-Smith: it, especially when it goes bad, it's like, why am I doing this? But now you have a, now you have a final answer.
Mona Garvert: Yeah, exactly. It's, um, that one is, I, I certainly had that question before where it sometimes felt almost like a bit of an indulgence to answer these questions that I was super interested in, but, you know, you see all these crisis around you in the world, you're like, what? How am I adding to, to solving any of
Benjamin James Kuper-Smith: People have maps in their heads. Isn't that cool?
Mona Garvert: Yeah. So I, I've always had this kind of, that was always an issue that I had. And so it's nice to kind of contribute to, to one of the things that I think are really, um, critical in our society, mental health problems affect so many people, and if we can help them, You [01:06:00] know, people might have build resilience to face all the other crisis crisis that at the moment they might not necessarily have, uh, and maybe also have the capacity to develop solutions for, for other problems.
So that's quite satisfying. And in general, the, the pace is quite fast. So we're essentially piloting again and again. We use platforms that academics also use, like prolific and yeah, just try to do new things.