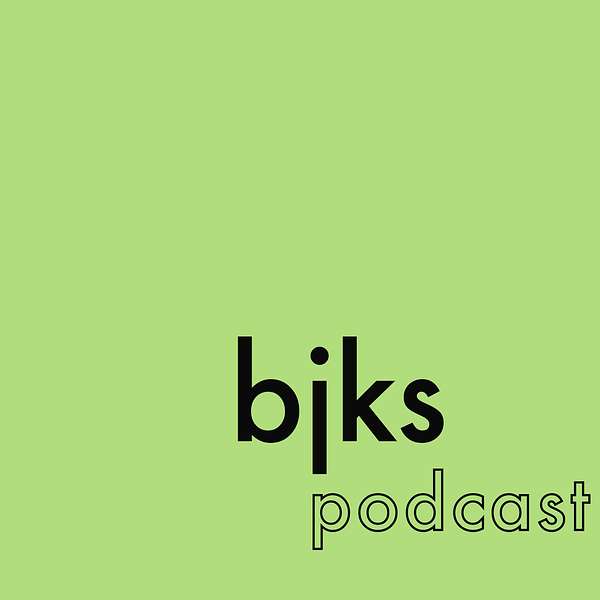
BJKS Podcast
A podcast about neuroscience, psychology, and anything vaguely related. Long-form interviews with people whose work I find interesting.
BJKS Podcast
13. Joe Hilgard: Scientific fraud, reporting errors, and effects that are too big to be true
Joe Hilgard is Assistant Professor of Social Psychology at Illinois State University. In this conversation, we discuss his work on detecting and reporting scientific fraud.
BJKS Podcast is a podcast about neuroscience, psychology, and anything vaguely related, hosted by Benjamin James Kuper-Smith. New conversations every other Friday. You can find the podcast on all podcasting platforms (e.g., Spotify, Apple/Google Podcasts, etc.).
Timestamps
0:00:05: Are we only catching the dumb fraudsters?
0:08:45: Why does Joe always sign his peer reviews?
0:11:51: Detecting errors during peer review
0:17:44: Retractions motivated by Joe's work
0:22:19: The whole Zhang affair
0:49:19: Ben found errors in a paper. Joe advises what to do next
1:04:06: How to separate negligible errors from serious errors that require action
1:11:37: When effects are too big to be true
Podcast links
- Website: https://bjks.buzzsprout.com/
- Twitter: https://twitter.com/BjksPodcast
Joe's links
- Website: http://crystalprisonzone.blogspot.com/
- Google Scholar: https://scholar.google.de/citations?user=FPOHtgQAAAAJ
- Twitter: https://twitter.com/JoeHilgard
Ben's links
- Website: www.bjks.blog/
- Google Scholar: https://scholar.google.co.uk/citations?user=-nWNfvcAAAAJ
References
Brown, N. J., & Heathers, J. A. (2017). The GRIM test: A simple technique detects numerous anomalies in the reporting of results in psychology. Social Psychological and Personality Science.
Callaway, E. (2011). Report finds massive fraud at Dutch universities. Nature News.
Friston, K. (2012). Ten ironic rules for non-statistical reviewers. Neuroimage.
Heathers, J. A., Anaya, J., van der Zee, T., & Brown, N. J. (2018). Recovering data from summary statistics: Sample parameter reconstruction via iterative techniques (SPRITE) . PeerJ Preprints.
Hilgard, Joe's blog post about the Zhang affair: http://crystalprisonzone.blogspot.com/2021/01/i-tried-to-report-scientific-misconduct.html
Hilgard, J. (2021). Maximal positive controls: A method for estimating the largest plausible effect size. Journal of Experimental Social Psychology.
Hilgard, J. (2019). Comment on Yoon and Vargas (2014): An implausibly large effect from implausibly invariant data. Psychological Science.
Lakens, Daniel: blog post on hungry judges: http://daniellakens.blogspot.com/2017/07/impossibly-hungry-judges.html
Morey, R. D., Chambers, C. D., ... & Zwaan, R. A. (2016). The Peer Reviewers' Openness Initiative. Royal Society Open Science.
O'Grady: Write up in Science Magazine about the Zhang affair: https://science.sciencemag.org/content/371/6531/767
Simmons, J. P., Nelson, L. D., & Simonsohn, U. (2013). Life after p-hacking. In Meeting of the society for personality and social psychology, New Orleans, LA.
Simmons, J. What do true findings look like: Presentation slides available at https://osf.io/93fkq/
Stapel's autobiography freely available in English: http://nick.brown.free.fr/stapel
Yong, E. (2012). The data detective. Nature News.
[inaudible]
Benjamin James Kuper-Smith:One of your recent, not that recent, but one of your last blog posts was on whether people who fake data are stupid or something like that. Um, whether all the people we catch are those people stupid. Um, and I was kind of wondering in general, along this lines. So do you think for whatever reason, let's say you want to fake some data and get away with it. Do you think you ould do it?
Joe Hilgard:Oh, absolutely. I could because I'm smart.
Benjamin James Kuper-Smith:Oh, so sorry. Um, yeah, I, but, so, uh, I'll add two clarification to that it has to be open dataset and show individual datasets in the elect data points. So the easiest thing in the world, how do you do it?
Joe Hilgard:Um, well, so we can get into this later, but we've seen that you can be very, very bad at this and essentially get caught and still nobody will do anything about it. Um, so you don't really have to worry about doing a very good job of it, but honestly,
Benjamin James Kuper-Smith:But so you don't get noticed even, right?
Joe Hilgard:So if you want to completely evade detection, basically all you need is to really understand the generative model behind the sort of data you're looking at. So if you are a cognitive psychologist who wants to gin up some Stroop data, and you are used to thinking at least a little bit about multilevel modeling, right? You can think about some subjects will be faster than other subjects. Some reaction times in some conditions will be faster than other reaction times and other conditions. Here's the amount of variability we usually see between people or between trials within a person. If you can make that model, you can make the data, right? Because that's the model people will use when analyzing the data. You can make data that looks perfectly natural given that model. Um, so to me, I think any degree of sophistication whatsoever with regard to what data on this measure, usually it looks like, or what effect sizes of these manipulations usually look like. Um, if you know those things at all, you can fake data quite convincingly on these things.
Benjamin James Kuper-Smith:So I guess one thing I think that might be that might give you a way is like, if you use some sort of function and then it's like too smooth, almost of a normal distribution, let's say, or something like that, like when it's can't you, can't you detect, you know, when, when something matches a distribution too closely,
Joe Hilgard:If you're using these functions that do polls from a normal distribution or a beta distribution or whatever, um, they will be just as noisy as any data should be from that null or normal or beta distribution. Right? So if I tell R, pull me a thousand subjects worth of data from a normal distribution, it will be as spiky or as smooth as 100 data points for that distribution should be. Um, if I'm super sophisticated, I could manually add a blemish or two to the data set. You know, the, the, the way that like a Japanese artists would deliberately inflict some sort of minor imperfection on an otherwise perfect piece of pottery or wood covering or whatever. Um, but it's, it's, it requires so much data to be able to say like, Oh, this is suspiciously smooth, or this is suspiciously. Perfect. Um, if you think about some of the guys that Uri Simonson caught guys like Sanna or Smeesters or Forster, those guys made the mistake of doing the same perfect pattern repeatedly. You can't catch somebody with these patterns unless they've done the same pattern multiple times. If you do it just once it's not a pattern, right. And if you're going to accuse somebody of cooking their data, you need to be able to say like the odds of this pattern manifesting in real data is one in a hundred thousand or one in a million or one in 10 million or something like that. Um, so if you're doing just a one-off, you know, there's no pattern, nobody can, can, can Mount that much statistical evidence against you.
Benjamin James Kuper-Smith:Yeah, it's weird that it's, I mean, I guess that's why the question was also asked on, on the tweet and on your blog post it's, like, are we only catching the people who do
Joe Hilgard:We are absolutely catching only the people who are really bad at this. Um, Joe Simmons, uh, had a, uh, talk at SPSP what was it 2020, I guess the last SPSP before the world ended. And I keep going back to it and watching it, the title of the talk is, uh, what real research findings look like. And in there he talks about, um, you know, the difference between a robust set of finance with some statistical power behind them, versus a null set of findings that, you know, look successful only thanks to publication bias and Q RPS, some combination of the two. The third thing he brings out is, um, findings that are false because they have been fabricated and Joe Simmons is a lot smarter and a lot more experienced than me. And in this talk, he says, explicitly, I'm only going to tell you what stupid fraud looks like, because I don't know how to catch smart fraud. And I am exactly the same way. Um, you have to not only make up your data, probably several to get caught, you have to be bafflingly and competent at it in order to, to get caught.
Benjamin James Kuper-Smith:I wonder, like what that also says about, so the people who are really, really bad at creating or faking the data, I mean, would they be also then, would you assume that they also write worse papers? So it almost doesn't matter quite as much because the papers aren't that great anyway, or is there no correlation between the ability to fake data?
Joe Hilgard:I really don't know. I mean, thinking about Stapel, he was a pretty effective author, I guess, right. You don't get 58 papers that you later have to retract by being a bad writer. Um, and some of these were in quite high profile journals that, you know, want a article that really lays out the theoretical importance and, you know, builds excitement into the reader and stuff like that. So you look at somebody like a Stapel, I would say there, uh, you have a lot more writing ability than you have data sense. Um, so I don't think that those two are necessarily correlated. Yeah.
Benjamin James Kuper-Smith:By the way. Do you know what, what actually happened with Stapel? Like, is he, what is he doing now? Do you know?
Joe Hilgard:I have no idea what he's up to,
Benjamin James Kuper-Smith:I wonder where you go from there, because is it fabricating data? Is that illegal enough to get you in prison?
Joe Hilgard:I don't believe he ever did jail time. Um,
Benjamin James Kuper-Smith:so they just kick you out of the academics,
Joe Hilgard:they kick you out. Um, yeah. I don't know what he's up to. I, I imagine, um, he's maintaining a pretty low profile. Um, I think he speaks a little bit to what he's up to in his, uh, autobiography Ontsporing, uh, translated as derailment by Nick Brown. Um, it's an interesting read it's available for free on Nick Brown's website. Um, so go ahead and check it out and tell Nick Brown. I sent ya.
Benjamin James Kuper-Smith:Okay. That's I'm going to check that out, but why does Nick Brown have it on his website? Someone else's autobiography isn't that?
Joe Hilgard:So it's Stapel's autobiography, but it's written in Dutch and Nick Brown is a bit of a, a polyglot. Um, I understand that he speaks Dutch fluently as well as English. So as kind of a public service, he translated it into English. That's my understanding of it.
Benjamin James Kuper-Smith:Huh. But wouldn't Stapel say, it's just it. Wouldn't he say like, Hey, that's my copyright don't publish it. Like, I mean,
Joe Hilgard:You know, it seems, it seems like he would have grounds for doing that, but he hasn't, he has not, he has, does not seem that he's DMC aid. Uh, the, yeah. So,
Benjamin James Kuper-Smith:Okay, well then I'm going to check it out. So as I mentioned, I'd like to talk about your blog post, but just before that, there was, um, two things, uh, two brief things that I saw on your CV that I just to ask about briefly. Um, so these are both sections or statements. I don't think I've seen on other CVS, but then again, how many CVs have I read? Um, I don't really read them that much. Uh, but so the one question is why would you always sign your reviews? So that's the last statement and it's on your CV is I always signed my reviews. So I should maybe say I'm as a, just entered the third year of my PhD now. So I've had some experience with peer review, but not a huge amount. Um, so what are some arguments for against signing your, yeah. So your overviews and why, if you'd done it,
Joe Hilgard:The reason I signed my peer reviews is, um, I put a lot of work into those peer reviews. I really try my hardest to be thorough and fair. And so I kind of want people to know I'm out here doing that work. Um, it, so it's a complicated thing. Um, because it's a lot easier for me to sign my peer reviews as a white guy. Um, than other people might have signing their reviews. Um, so far I'm not aware of anybody who has tried to retaliate against me for the things I've written in reviews. Instead, my experiences have generally been positive. I'll be at a conference or something and somebody will say, Oh, you know, your reviews really helped us out with this meta analysis or whatever. Um, so I think it helps me be accountable. It makes me kind of do, you know, find the nice things I can say about the paper at the same time I'm criticizing it. And it also, you know, kind of creates an amount of transparency so that if it's results that conflict with something that I've written, then, you know, I have to own up to that. I have to say, Hey, I'm Joe Hilgard, I don't like your results, but, um, I'll do my damndest to try to put that aside. Um, or if I am saying like, Oh, you should, you should read Hilgard, you know, at all 2018 on this, uh, that people know that I'm not like trying to be slick with it. I just think that maybe they should check out by paper. Um, so for me, it's kind of an accountability thing, but also a visibility thing. Um, I started doing it, I think either late in grad school or on my post-doc, um, I was, uh, kind of desperate for exposure, right. Uh, because I didn't know if I would have a shot at a tenure track job or not. Um, so I thought basically anything that can get the name, Joe Hilgard out there, uh, will maybe be good for me. So I started signing my reviews.
Benjamin James Kuper-Smith:Okay. So let's move, uh, uh, self-promotion sounds
Joe Hilgard:Equal parts, accountability, and self promotion. Yeah. Which works for me. They may not work for everybody
Benjamin James Kuper-Smith:Then. So when you, so when review a paper, do you then getting to slightly more about the fraud and error detection? Do you actively see whether you can find any errors in that or something, or is it an errors in terms of like, you know, whether the stats add up or
Joe Hilgard:Yeah, I mean, that stuff is always on my mind. Right. And if I can catch, if I can catch something in peer review, it is so much easier to deal with than catching something. Once it's published. Um, I have been waiting seven months for a journal to publish a retraction notice that they showed me seven months ago. Right. So it can take seven months for them just to publish the retraction notice. Whereas if you find something wrong in peer review and you squish it and peer review, it's dead within two months. So yeah, I, I, you know, I cast an eye over everything. Um, and I also always request, you know, within reason the raw data, um, I'm a signature of the peer reviewers openness initiative. Um, this is a kind of collective action thing from peer reviewers to push for greater data accessibility in journal articles. This is something we need because we all know and have known for decades, that data available on request is a lie. Um,[inaudible] and a bunch of people tried it like way back in the nineties or the early two thousands. Um, and they got data from like something like a quarter of papers that said data available upon request. So data is not available upon request. The only way that data will be available is that it is a condition of publication to put it up in a public repository, like open science framework or Github or data verse or something like that. Um, so as part of my peer reviews, I always ask people, put it up on the internet, if you can, or give me a good reason why you cannot. And when they put the data up, I tend to like to grab the data and play with it. Um, but I don't always have the time for all of that. Right. I can't give everything the complete, uh, check and complete strip down and rebuild. So sometimes it's a matter of, well, how fishy does this sound?
Benjamin James Kuper-Smith:I mean, like one thing I noticed and, you know, at the end of the conversation, I think I'd like to talk about the paper that I've had some problems with. It takes a lot of time to check for errors, because as soon as you check for errors and your, you make an error, you just look like a complete arsehole and like an idiot. And, you know, so I did a presentation for our lab just to present this paper, say like why I think there are problems with the paper in terms of just, you know, not bad methods, but just in terms of like, something's wrong with the figures and data were being presented here. Um, it took so much time. It's such a lengthy process.
Joe Hilgard:Yeah. You only get one shot, right? Um, if you are trying to bring these concerns to some sort of authority figure, everything has to be perfect. Everything has to be damning and everything on your end has to be completely flawless because if you have made any sort of mistake, now you just look like a crank and an incompetent. Um, and it's hard enough to get people to listen to you the first time, much less for you to say, oops, I made a mistake, let me come back. And, uh, maybe you'll listen to the second time. You will not get a second time. So I have experienced the sort of, uh, error checking to be very frustrating and very time consuming just because of the need to make sure everything is neat as a pin, um, before going to any sort of authority figures.
Benjamin James Kuper-Smith:Yeah. But so that's then when you review a paper, you, you, I mean, you have to, of course, like you have some basic checks that probably everyone does often even unintentionally or unconsciously, but yeah, you don't go through checking every whether all the statistics are internally consistent,
Joe Hilgard:I've got a good sense for internal consistency in statistics, right. Um, so like the relationship between a T value and a P value or an F value and a P value, um, you can shake me out of bed in the middle of the night. And I know that a T of two corresponds roughly to a P of 0.05. Um, I have some are tools that use to try to check the relationship between a T value and sample size and the effect size. So, um, some of the, kind of the R packages I use for a meta analysis, um, are designed around saying, well, if you've got this many participants and your T value is this, that implies you have an effect size of that. Um, so sometimes I'll see a paper where the T value says, one thing about the effect size and the means, and standard deviations would say something else about the effect size. And so there, I start to get like a little anxious because they're used to see maybe it's not internally consistent. Um, but there's really not like one set of checks you can do for errors or for, especially for fraud. Right. Everything is its own unique case. You don't know what the true model is behind fraudulent data.
Benjamin James Kuper-Smith:Yeah. I mean, yeah. It could be so many things, right. Yeah. But then, so I mentioned there was two things on your severe that I was curious about. The other thing is now, I mean, what we're going basically even talk about, namely, you have a section about retractions motivated by my work. Yeah. And corrections motivated by my work. But maybe in a more general question is, I mean, I have to admit, I, I got to know you through the blog, posts, someone retweeted it or something. And then I saw the blog post read it, um, was both, uh, thought it was great and immensely frustrating. Uh, so I, I guess my question is kind of, is this, it seems like you're doing this quite a bit. Right. And I guess my question is whether it is intentional or like way that you, again, this sounds such, such negative words, like policing or something like, is that something you you want to do and try to do, or does it just kind of, do you just have a more acute eye for this?
Joe Hilgard:Oh, I love it. And I hate it. I do it more than I really ought to. I didn't choose this life. Um, I just kinda got plunged into it. So with regard to the CV, the reason I list these things on the CV, as ghoulish, as it seems, right, like literally ghoulish, like a ghoul is a monster that eats the flesh of the dead. Um, here I am sustaining my CV on the cadavers of retracted papers, um, by less these things on my CV, because I think that it is a w among my legitimate scientific contributions. Um, yeah, definitely how much more effective it is to retract a single erroneous paper than to spend 20 years. And who knows how many resources trying over and over again to replicate it before finally everybody says, okay, maybe that thing can't be replicated. Um, it's much nicer to be able to nip these things in the bud. And so when I get a paper retracted on those few occasions that I have, it's required an amount of work that is roughly commensurate with an original publication. And I think the benefit is roughly commensurate with an original publication. And thankfully my department sees it as being a legitimate intellectual contribution as well. So I listed these things on my CV. There was another question you had there that I lost in my spieling.
Benjamin James Kuper-Smith:Uh was there I can't remember. I got it.
Joe Hilgard:Did I do, do I do this? Because like, I enjoy the policing or because, and the, yeah, just like how it comes about it comes about, it's kind of like, well, like everybody else, I read my literature. Um, I do work in an area that has some, you know, some kind of, uh, wooly areas. Uh, we, we butt heads over some things and I try to make sure that my work is well situated in the existing literature. So I'm reading what's out there. Um, and sometimes in the process of reading what's out there, I'll notice something that just seems either fatally flawed or kind of straight up impossible. That results are way too good to be true, something like that. And sometimes I'll see it and I'll say, well, there's really nothing I can do about that. I know that that's probably not true, but I can't find any sort of purchase on it. There's no, there's no place for me to get leverage, um, to be able to show that this result is too good to be true, even if it's not like some obvious, it's not some obvious error. I just say to myself, there's no way that's true. It's probably some sort of mistake or worse. Um, but I'll never be able to show that. So I'm just gonna put it in my folder and try to forget about it. Um, but in the case where there's a paper where I think I can show that the result is too good to be true, or that things don't add up, there's this sort of idiotic optimism that makes me want to try to do something about it. And so it's chasing that optimism, uh, that gets me kind of perseverating and grinding on these papers over and over again, and trying to see what can be done about it. And, um, kind of see if our systems are capable of doing something about it.
Benjamin James Kuper-Smith:Shall we then maybe just talk about the, the Zhang, I guess the paper or papers, the whole Zhang affair affair, maybe that's a good term. Um, so maybe I can't remember whether this is in the blog post or not, but how did you first come across the paper and under what kind of, why were you reading it?
Joe Hilgard:Yeah. Um, so I study among other things, the relationship between violent media and aggressive behavior. Um, that's how I got interested in meta analysis is how I got interested in the study of aggressive behavior. Um, because people have been having this intense argument for decades and I thought, Oh, well, maybe I can be like a fresh face in this conversation. I can run some studies. I can do my own meta analysis and figure out what I want to believe. Um, so a lot of my substantive work is in this area. I'm now trying to do stuff with kind of just the psychometric properties of some of our aggression measurements. Um, but so Google scholar knows that I am interested in studies on the relationship between pilot media and aggressive behavior. And so when, uh, Zhang's 28, 18 2016, uh, youth and society papers came out, Google scholar helpfully delivered them to my doorstep. Um, and so I took a glance at the abstract and I thought, well, that's quite a sample size. I better read this
Benjamin James Kuper-Smith:Are the 3000 people that were there. It was 3000 children.
Joe Hilgard:Yeah. 3000 children in a randomized controlled trial. Um, this is a research area where I want to say my dissertation was the second largest experiment on this topic. And I had like maybe 300, 250 good participants at the end of it. Um, so you have a paper that has 3000 subjects and their children at that. Um, this would be basically more data than the entire field generated between 1980 and 2010. So, uh, this one study would be a real important chunk of data. So I had to read it.
Benjamin James Kuper-Smith:And then were you then already questioning, did they really collect 3000 people or was that, where were you just impressed by it?
Joe Hilgard:I was impressed by it. Um, kind of like many of the people I tried to approach as I, as I read the article, it got kind of curious about like, well, is this legit or not? Um, one of the things I was curious about was, well, how feasible is it to collect 3000 children's worth of data in a randomized controlled trial. And both me and the people I talked to kind of don't know what to expect because it's China. Um, and we don't really know how research methods go in China. We don't know how, uh, IRB review works in China. Um, we don't know how much cooperation there is between like primary school teachers and research psychologists in China. Um, so I don't know ma I mean, it, it seemed possible to me that they just had really good logistics and really good buy-in from the primary school teachers. Um, if you, you know, had enough, if you have the process set up well, enough conceivably, you could accomplish this.
Benjamin James Kuper-Smith:Yep. Okay. So impressed maybe slightly a bit, a little bit critical, but impressed in general. And then you just read and, uh, questions came immediately.
Joe Hilgard:Questions came up pretty quickly. The moment I started looking at the statistics and the tables. So table one of the paper or whatever is basically you're a Nova table, right? You have the effect of the media, the students were randomized to consume. You have the effect of, I want to say gender or age, and you have the effect of trait aggression in there, but it's not an Inova table such that you or I would recognize where they're like means at each level of the cell combinations or whatever. Um, there are just jibberish numbers there. Um, it looks like maybe the sums of squares and the mean some of the squares or something. Uh, and then there's an F value. And then there's an arbitrary number of significance asterisks after the F value that has nothing to do with the actual F value. So there's like an F value of 1.4, three, and it's got a significance asterisk after it. And there's an F value that's, you know, just the P values didn't match the F values at all.
Benjamin James Kuper-Smith:And so where'd you, I mean, I'm trying to kind of basically recreate kind of your process of going through this. So then do you just read the rest and say, okay, there's just more, or was that already enough for you to go, okay. There's just like, what were you thinking at that time? Was it just okay. They messed up some figures on some, some numbers somewhere.
Joe Hilgard:Yeah. What I thought was they messed up some numbers. Um, I thought, you know, there's something very strange with this. I'm not sure what to make of it, but I don't really want to get involved. I'll tell the editor that, you know, this table first, I told the author that the table makes no sense. Um, and the author said, Oh, I'll fix it. And I waited a while and I said, Hey, did you fix it? And he said, Oh yeah, I fixed it. And I had, didn't see any correction published. So I went to the editor, I say, editor, this author told me he was going to fix this. Has he fixed it? And the end of the sentence is the first I've heard of it. Nobody's come forward and say, they're going to fix anything. So I told the editor, maybe you should follow up with this author and get him to fix it. Then I figured at that point I was just done. Um, I thought, you know, maybe in the process of fixing it, whatever the problems were, if there were any would come to light, um, and the editor could handle it on their own. But I started reading the other papers out of, uh, Dr. Zhang, um, me and a couple other people on Twitter got kind of curious about the larger output here. And when I start,
Benjamin James Kuper-Smith:When you say people on Twitter, was this already being talked about like that none of this made sense or
Joe Hilgard:Yeah. Somebody, I don't, I don't know if it was because I had posted the table and it didn't make any sense or somebody else had posted the table. Um, but a few of us had like a small conversation about some of the weird things in the paper. Um, they also had this mediation model set up as, so the, the hypothesized model here, it shows the relationship between violent game play as causing aggression as something that's mediated by gender and age and personality. Now, of course, violent video games can't change your gender. They can't change your age. They probably don't change your personality. Very much. Those things are all we usually think of as kind of like stable preexisting factors. Right. So they would not be things that mediate the relationship. So as, as we read these papers, we said, wow, there are lots of, kind of weird things in here. Maybe sloppy. I don't know, you know, how much, how, how, just how bad we expected it to be, but we thought it looked sloppy at least.
Benjamin James Kuper-Smith:And then, so you'd already contacted the authors and the journal. I mean, was it, then I'm trying, I'm trying to like, imagine just what the, basically what it was like for you to go through that whole thing. Was it just you sending an email and then you wait two months and then you go, wait, what happened with that thing? And then you sent an email again, or
Joe Hilgard:So here's one of the things that w not to jump ahead here, but, um, I think that there are very serious problems with all of the papers Dr. Zhang has published. Um, that's why I wrote to his institution to say, Hey, would you please check on this guy? And that's why I wrote to all these other journals saying, Hey, would you please look at this paper you published and consider retracting it for these reasons. Um, so I think that there are in general serious issues with Zhang's work, um, uh, uh, losing my train of thought here, shoot. I started trying to set the, the, the, the, the background. And then I get,
Benjamin James Kuper-Smith:Yeah. I mean, uh, I was asking like, whether you send an email every few months.
Joe Hilgard:Yes. So, so issues with Zhang's work in general, they're kind of obvious problems, right? Um, you can just look at the F value and say that doesn't, that's not P less than point of five of that F value. One of the remarkable things about Dr. Zhang that has made this easier still to pursue, um, is that at least at the time, he was very Swift and replying to emails. So it would send them the question and I would go to bed and the next morning I would get up and I'd have a response from him. Um, that is not how it's been with other people. I have had problems with more commonly. When you write to somebody saying, Hey, can you explain to me, what's the deal with this thing in your paper? You can wait two months and then you can nudge them again. And then you can wait another two months. And by then, you know, somebody has changed institutions, or you have a new set of responsibilities, and it's basically a waiting game. Um, people will just wait you out. So, um, Zhang's Quick replies to email made this easier for me. Um, but most people don't reply to emails nearly so quickly as, as he does.
Benjamin James Kuper-Smith:So he, he, he made a for all data fraudsters out there, he made a crucial error there in responding to your emails.
Joe Hilgard:I've got a, I've got something in my drafts folder of, you know, how to make up data and get away with it. And rule number one is don't reply to emails, make somebody re email you 20 times over two months before you reply and, um, make it like their problem that they're emailing you so much. It's inappropriate, it's harassment for them to email you so often you're just over here trying to be a normal person.
Benjamin James Kuper-Smith:Yeah. I'm just trying to do, I'm just
Joe Hilgard:Trying to do research and you have some sort of weird chip on your shoulder about me. I don't understand.
Benjamin James Kuper-Smith:I mean, that's basically what, there's this briefly after you published your blog post, there was this brief, this short write-up in science about the whole thing. And didn't he basically say like, well, if this, uh, Hilgard guy just exactly what his phrasing was, I can't remember, but something like he did,
Joe Hilgard:I'm trying to make a name for myself by tearing down other people's research. Yeah, exactly. Which, you know, if it can be destroyed by the truth, it deserves to be destroyed by the truth. Um, Zhang is not the only person I have had problems with. He is not the only author whom I have criticized. Um, he's not the only author where I have some subjective probability that something is seriously wrong. So this not a personal thing between me and Dr. Zhang. Um, and if I seem to have made a habit of this, it's just because now that I can see it, I can't stop seeing it. And I feel kind of a personal responsibility to try to deal with these things because not everybody can see it. Not everybody can do something about it. I can see it. I can do something about it. I'm the only one hard-headed enough to do something about it. Um, so I'm going to try no matter how much it sucks.
Benjamin James Kuper-Smith:Yeah. Have you, have you had any case where you, um, I don't, I mean, I've, I've, I've got the pay, like the, on your CV. I can see the papers that, uh, you know, were corrected or retracted. Many of them,
Joe Hilgard:Those corrections by the way, are like the most honest and simple of mistakes. Things like calculating the effect size by dividing by the standard error rather than the standard deviation. Um, so just because something's in the corrected column, there doesn't mean that there's something fishy about it. Um, just want to make that super clear. Yeah. Yep. Yep.
Benjamin James Kuper-Smith:So I've seen, I see some papers that you've got here, but, um, is there anything that you thought, Oh, this is too too hot to handle, or you're slightly afraid of touching that paper or that author or something like that?
Joe Hilgard:No, no. Um, I am fortunate to work so I can imagine that things could be too hot to handle. Um, if they touched on some sort of, some sort of scientific issue with real stakes. Um, fortunately, unfortunately I work in a scientific area that has no stakes whatsoever. Um, in 2010, the Supreme court ruled that violent video games were protected speech under the first amendment. Um, so the stakes here are whether little Johnny gets to play fortnight for an hour or not. Uh it's it's, it's, it's not like people's lives are the balance here. It's not like we're trying to, um, end racism or, uh, you know, figure out the correct risk assessment related to the Corona virus. Um, none of this stuff matters. Um, and the responses of certain editors have really hammered home that none of this matters. Um, so if I were working in an area where things did matter, and there were kind of like social and cultural stakes attached to it, I would try maybe a little bit more like lightly. Um, but this, this stuff is all just parlor tricks with undergraduates. It, it has no real-world applications. Um, nobody's going to try to, you know, portray me as a right-wing nut or a bleeding heart liberal over the work I do here. So I can, I can do whatever I need to for the science,
Benjamin James Kuper-Smith:But with the whole to handle, it also meant in terms of off some very influential or powerful peopl. I mean, I don't know about the dude. It but with Zhang, it seems like, I mean, I don't know, you know, your research field or anything, but it seems like he's far away in China. Pissing him off isn't as much of a problem as if I don't know. Uh, again, I don't certainly your, your, your head of department, right?
Joe Hilgard:Yeah. Well, so fortunately, none of this is unfolding within my department. My department again, has been very supportive of me. Um, Zhang does have some, uh, American co-authors, um, and they have reacted with varying levels of concern when I've tried to express to them my concerns. Um, so some have been relatively proactive. Others have said like, Hey, leave me out of this. Or even like, what are you doing this for? Don't be a Dick. Um, and, um, I've definitely made some editors frustrated with me. Um, but again, those editors, I don't think were very fond of me to begin with because of my substantive research. Um, some of the findings I've got from, um, either studies where I couldn't replicate an effect or meta analysis where I say, Hmm, this effect seems to be badly overestimated by publication bias. Um, so I really didn't have much to lose here. Um, the, the, the, the, the powerful old heads, um, didn't already like me. So, um, you know, that kind of reduces my leverage with them. I can't, I CA I don't have much pull with them, but, you know, it's not like I can sour our relationship further.
Benjamin James Kuper-Smith:So you're protected by prior. Yeah.
Joe Hilgard:I, I'm just, I'm just a big wallowing in the mud.
Benjamin James Kuper-Smith:Okay. Well, as long as you're having fun,
Joe Hilgard:It is fun. Sometimes it is thrilling in a way that, uh, my mundane research sometimes fails to be thrilling, right. This has an element of drama and mystery and Sherlock Holmes elements to it. Uh, that, that my, my day to day work, doesn't always,
Benjamin James Kuper-Smith:Yeah, definitely from the, from the one case that, that I've had in the last few months, I definitely had the sense of like, yeah, you really feel like, uh, I mean, you know, you're just trying to, like, as I said earlier, make sure that you don't say there's an error in something when there isn't and you're just being stupid. Um, so you're basically just trying to cover your tracks until that time, but there is a sense of adventure almost where you go, like, if being like, like a detective and going, like, what if this is the case? And that is the case, then, you know, this could also be the case or something like that.
Joe Hilgard:We talk about, should we talk about the thing that you're curious about?
Benjamin James Kuper-Smith:Yeah, let's do that the, so, okay. I've as a, uh, I think I mentioned to you before we started recording, but the, um, so I'm not gonna the name of the paper or anything, because I mean, I've thought about it and there's obviously something wrong, but I'm not entirely sure yet, um, how much I'm going to say is wrong with it and that kind of stuff, because this is also very new, but the, the gist of it is that I've, I've read a paper in one of the, uh, many areas and that vaguely related to my research, and there were some obvious errors in it. Some of them well was just, like they said, like one value in the text and another value in the figure. Like, you could just see that, like, not text and figure can't be both correct. Um, stuff like that kind of stuff, but the, um, let me see. Yeah. So this is, there's sort of basic internal inconsistencies then based base. So I knew there was one and there was, so this is the thing, some of these things can be easily identified by a lay person outside of science. Some of these errors require knowledge of some modeling techniques that the author is probably aren't familiar with. Uh, and I, yeah, I mean, based on the analysis they've done, I can pretty much guarantee that they don't know how to run this stuff. And it's, it's also kind of started off for me as a kind of like, huh, I wonder how that, and that figure can both be correct. And kind of trying to figure like, okay, can, I don't think both could be correct, and I have this modeling way of finding out kind of. And so it almost started, it was a puzzle. And then I kind of started looking serious into the thing and just found errors all over. Um, the thing is most of these, so this is maybe the thing I should say at the beginning, from what I can tell this is just negligence. Um, so from, I've also looked into two other papers by the same authors written at similar time, and they all kind of had some, um, should we say, uh, like typos executive, but those kinds of areas where you go like, okay, they made a small error here, but it's not, it's not going to change your interpretation of the results or anything. Um, but somehow the thing is, so it seems like basically someone just didn't take enough time to write the papers. Let's imagine that. Um, yeah, exactly. Yeah. It's, I mean, it's not even, uh, yeah, but my point is like, I don't think I found any fraud or anything, but there is at some point when you found lots, like how many small errors can you find until we just start distrusting the entire yeah. And yeah, so that's kind of a rough day. And so what I I'd like to, so things I'm setting aside like the small errors that are obvious, um, and that might just be, you know, some typos, not, not a big deal in the grand scheme of things. Uh, I am still kind of interested in this modeling thing that I mentioned where I'm not entirely sure with, uh, my modeling approach of it actually shows that the Arizona, that what they did is wrong or not. It's just kind of something I'm also actually interested from a like conceptual perspective. And so I'd like to have their data and to analyze it and see whether what I did was correct or not. Now there's two, a few things here. So the first is that, you know, as you can tell, it's not an open dataset. And the second thing is the research area is clinical, and these are, this is data from patients, even though what I'm interested in, isn't clinically relevant. So let's put this way if I record. So the most for the data, they could easily hand me the data without having any kind of ethical concerns or anything like that, but they could obviously hide behind the clinical thing if they wanted to and say, well, it's clinical data. We don't want to give it out. You know, that kind of thing. Um, further, it seems that by now this was a few years ago, but by now all authors are fairly senior and they probably have better stuff to do, respond to and dig up some data that I could imagine isn't particularly well digitized and that they might just say like, ah, dude, just leave me alone. I've got stuff to do. Um, yeah. So that's kind of the overall picture and yeah. Presented in some lab and everyone, I think kind of agreed. And when it seems like there's definitely something fishy going on here, but we're also not entirely sure what to do now. I mean, you know, do I mail them and say, Hey, you know, I found multiple areas in your paper. Would you like to give me your data? Um, or do I say, you know, Hey, I'm interested in this modeling technique. I was wondering how both figures would be correct. Yeah. So that's kind of also what I'd like to have some sort of advice on, if you, from someone who just has some experience with it, because none of us have ever written an email like that.
Joe Hilgard:Oh Lord. Um, so my advice to you is, um, how far into your PhD are you
Benjamin James Kuper-Smith:A third year and, well, I've basically got two more years of funding.
Joe Hilgard:Okay. My advice to you is don't contact them at all. Don't just, uh, because here's the thing, nobody is going to come out and give you a metal for detecting the problems with this paper. And I mean, so best, best case scenario, you send them a nice note saying, Hey, I noticed these issues in these papers. They say, Oh, you're right, we'll fix it right away. They publish our core agenda. They give you a warm and hearty handshake for, you know, noticing the mistakes. And everybody just kind of gives each other a little thumbs up. That's the best case scenario. It involves the least amount of future work from you involves the most cooperation from the authors. And even in this case, um, it's not like this is going to help you with your career, right? Uh, mm,
Benjamin James Kuper-Smith:No. I mean, yeah, it's already wasted like a week of my life.
Joe Hilgard:Ed, you've already spent a week of research on this. So the worst case scenario is you continue to grind on this. You continue to dump more of your research time and energies into this, and you earn three or four senior people who think that you're some sort of annoying fly that keeps biting them in the hunches. So, um, and so, you know, you could earn yourself a, uh, an enemy who was going to put negative reviews on your papers or your grant applications. Um,
Benjamin James Kuper-Smith:And just a slight caveat here. It's not really from my own research field. It's kind of an adjacent thing. It's sure. I'm not sure these people would ever review my papers.
Joe Hilgard:Okay. So the distance makes you safer. It also makes you easier to ignore, right? Because they could say, who is this guy coming over into our field from that field who cares about him? Um, so, you know, you're not going to receive any material benefits from this. Um, nobody is going to recognize your efforts and applaud you, um, for, for identifying these mistakes at best, you might be able to relatively painlessly correct the scientific literature, um, by getting these authors to correct whatever mistakes they made, if they were indeed mistakes. Yeah. But that's the best case scenario. And so rationally speaking, I would say don't do it.
Benjamin James Kuper-Smith:So, okay. Yeah. I mean, I, I, that I should probably also add, I'm not sure even with the errors that, uh, it necessarily disproves that main findings. Yeah. Um, even when I did my modeling and seals team, like it pretty much came out the way they said it would. Yeah. Uh, it's just, yeah, it's just a very messy paper in that sense. Um, but, okay. So if, if you advise me against doing it, why are you doing it then?
Joe Hilgard:Because I'm stupid no, it's because I have no ability to, uh, let things go. Uh, I am so stupid and so obstinate and it really just gets my blood up when, um, somebody tries to blow me off or brush me off or refuse me data or whatever, um, that I will just grind and grind and grind and grind on this. I probably should talk to a therapist and instead of doing this sort of work, um, but I can't not do it. Um, and there's also kind of a research interest here for me, you know, as kind of, uh, I'm not an out and out metascientist, but I have metascience research interests, right? So something like the Zhang affair is to me an interesting data point as a stress test of our scientific self-correction. One of the things we tell the public for why they're supposed to believe us is because we have other scientists who are treating each other's work, uh, skeptically and critically. And so if there are mistakes, one of us should find it and report it to the system and the system should work. And I am the scientist, who's finding problems and reporting problems. And I am various very curious to see whether or not the system works again, given how bafflingly crude, the issues are in Zhang's papers. Things like straight up recycling a table from another article, right at the same table appears in three different articles, um, or, or subgroup averages of six and 10 somehow average together to yield 20, the average of six and 10 can never be 20. Um, it really suggests to me that like, this is the lowest possible bar. And so the fact that some editors have brushed me off, um, the fact that it has taken so long to get some of these papers retracted really suggest to me that they're broader problems. Um, so for me, it's been kind of this, this metascience stress test, and that's been, for me, that's been worth the cost of admission. Um, but I'm, I'm on a tenure track. Um, my department is kind to me. Um, you are at a much earlier and more vulnerable stage of your career. Um, so I would recommend against it.
Benjamin James Kuper-Smith:Oh, all that work for nothing.
Joe Hilgard:Welcome to graduate school, all that work for nothing.
Benjamin James Kuper-Smith:Yeah. To be fair. I've gotten used to that point. Um, but, uh, but part of me then is, you know, it's this weird combination of motives that goes into asking yourself what to do about it, because, you know, part of me, as you kind of said, like earlier, like this is effort, like I put effort into this, I found this thing and you know, this was work. Um, and I I'd like to have some, something almost to show for it. Another part is the, like, it's not like, like the paper isn't that complicated. Like just please correct it. Like, just get it right, please. Um, and then this is kind of also idealistic thing about like, you know, as you said, as you kind of mentioned, like, it should be a self-correcting thing where you, as soon as you mentioned an error, people go, Oh, I'm sorry.
Joe Hilgard:Well, so I was a little flip when giving you my cynical advice, um, of just don't, um, we can be a little more optimistic and say you, and a more senior person to gather, could draft a letter, outlining the issues and requesting a check and correction. Um, if you can shield yourself with a senior figure and you can use a senior figure for some leverage, um, so that you're taken seriously, then I think there's a greater chance that the authors will take your concerns seriously, and they will do something about it. There's still a good chance that they'll say, Oh yeah, we understand that there some issues, but they don't substantively change the conclusions of the paper. Uh, we're very busy. That paper was XYZ years ago. Um, so we'd prefer to just let it lay, you know, let it lay as it is. Um, and I don't think that's the most thrilling outcome to this, but I think that that is a as satisfying an outcome, as you can hope for. Um, because everybody has stuff they're up to and every paper kind of has a statute of limitations on it. I don't know how old these papers are.
Benjamin James Kuper-Smith:It's not super old. I don't think that statute would apply. Um, it's also, this is, so this is the next thing. It's also one of very few papers about this particular topic. So it's almost, if you're interested in a kind of question, this is the paper you read, so in a way, but thereby kind of, you know, clinical stuff is always harder to collect right. So thereby it also gains a higher input. It's not some random paper. It also came out in a fairly decent journal, um, or actually I think very good journal. Um, so it, there's the slight complication here that it's more, you know, within a somewhat smaller research area, fairly influential. So when we want that paper to be, you know, not filled with area,
Joe Hilgard:So maybe, maybe you should try, but, um, again, you'll need to, you'll need to express yourself concisely so that people will read the darn thing. You'll need to express yourself pleasantly so that people don't get defensive and say, no, you're wrong, go die. Um, and again, you'll probably need to have some sort of senior figure, put their name on it as well, so that you're taken seriously. And, um, people know, you know, the, the office in question though, that your concerns have been vetted by somebody, if roughly they're saying power level. And if it's a good journal, I roughly feel like some of the editors at some of the better journals do take things more seriously. So, you know, I would go to the authors first, but if you need to alert the editor to issues, you could try it, the editors may ignore it, or they may have other stuff they gotta do, but at least they probably won't bite your head off for, you know, reporting concerns, errors. Yeah.
Benjamin James Kuper-Smith:It's a tricky, yeah. It really is a weird trade off where I kind of have to calculate these things about, you know, like how much time to have a PhD. Isn't really that important. Um, how much time does it take to actually write that letter concisely? And yeah,
Joe Hilgard:It takes surprisingly long to write the letter concisely.
Benjamin James Kuper-Smith:I mean, it takes me ages to invite someone to the podcast. So like criticizing someone is going to take even longer to write that email. Um,
Joe Hilgard:It's a good exercise if you, if you can afford it.
Benjamin James Kuper-Smith:Yeah. In a way I feel like I probably can in terms of time, just also I think maybe like you, um, sometimes I know I'm doing dumb stuff, but I was doing anyway. Um, I mean that, those were your words. I think I, on those words. Good, good. Um, but yeah, and then this is the thing like, yeah. Okay. So maybe they, you know, made some slight type of, and then that bar's slightly higher than it should be, but who cares? Right. I mean, right.
Joe Hilgard:Yeah. And I appreciate your use of, um, Hanlon's razor here of who's the rest of, what am I using? So, so many of us are filming the familiar with Occam's razor, which is, um, to use the simplest most parsimonious explanation possible.
Benjamin James Kuper-Smith:Are you referring to the one that, what is it, uh, assume malice rather, sorry. Ignorance, rather than
Joe Hilgard:I assume ignorance rather than malice. Right. Um, that's, that's where I think we have to start when we see errors in the paper is assume ignorance rather than malice that said, you know, in some cases I've been involved at, I eventually have to give up that, that assumption, but for your case, it sounds like as you say, probably just ignorance, not malice.
Benjamin James Kuper-Smith:Okay. I that's the way I've said, so far, I still think this is the case, but it's part of me that goes, I mean, I guess this question, like how much ignorance, maybe that's more of the question, but like, so I had this thing where, so, you know, the, uh, Nick Brown and James Heathers have done, they've done these toolboxes on, um, whether certain values can be correct. Given your sample size, like whether there's certain means of sending deviations,
Joe Hilgard:The GRIM test, the SPRITE tool. Yeah,
Benjamin James Kuper-Smith:Exactly. Those things. Okay. Yes. I actually, somehow couldn't quite, I wasn't entirely sure how to use it for this one, but I could use the same logic to figure out that the mean they provided context list. And so in principle, I'd say, okay, maybe they excluded some data, they didn't report it. You know, like again, that can be some very benign reasons for that, but they never report on excluding anyone or any trials. There's no like anything about exclusion and the, um, uh, um, the interesting thing is that the, the, the, the, the, the mean that they get and the input you put in, it really doesn't match up. Like, it's kind of like, it's, it's a really odd value to get once you actually think about it and put it like, once I like, basically laid out what are the values that can go into this to make this happen? Either they have way more trials than the report, which makes no, all that excluded and rounded weirdly. Yeah. And, um, so there's,
Joe Hilgard:Or there's a data entry error, right? Something typed in 99 instead of nine. It could be, it could be anything It's such, I always come back to Tolstoy. Right. Uh, all happy families are alike. Each screwed up dataset is screwed up in each their own way.
Benjamin James Kuper-Smith:I think that's exactly what Tolstoy said with data science, data science guy, Leonard. Yeah. Yeah. But, um, yeah. It's yeah. I guess I'll just have to see what I do, because part of me is just pretty curious to know, like, okay, how did they mess up? Because I don't know how you can. Yeah. I'm really curious how you mess up a data set in such multiple ways.
Joe Hilgard:Um, uh, if it's anything like my graduate school training or my early graduate school training, it's, uh, the way you get all those things screwed up is you have a grad student manually cleaning the data in Excel at two in the morning, uh, when they have to present this stuff at lab meeting at 10:00 AM the following day. Right. Um, I think that in many areas of psychology, uh, where open code is not common, our data cleaning processes are idiosyncratic and irreproducible, um, boiling down to manual scrubbing in Excel, uh, by a grad student, sometime between happy hour and 3:00 AM. Um, so, you know, I feel like anything's possible. Sometimes it papers of us. I could actually see like the code that does the cleaning.
Benjamin James Kuper-Smith:Yeah, yeah, yeah. I never even thought of something like, like[inaudible], but yeah, I, I studied psychology. I, I know how you, how the training is. Um, yeah, I guess I'm not going to be idealistic then.
Joe Hilgard:Well, and so I think this comes back to, um, why I think some of the systematic changes are so important, right. Um, we can't have a perfectly decentralized system of reproducibility checks and, uh, data cleaning guidelines and open data being, you know, what you feel like doing for that particular project for that particular day, things would be so much easier if we could get institutions and journals kind of on these transparency and openness guidelines, things like the top system. Um, I mean, again, when I review I'm a signature, I'm a signatory of the peer reviewers openness initiative. I just asked for the data on everything I review as a matter of course. And I say that like, I'm, you know, I'm oath-bound to ask for the data. It's just the thing that I have to do. It's not personal. I didn't have to sit down and think about whether I wanted your data or not. This is just what it is to do business with me as your reviewer. Um, and so I think to the extent that we can make some of this transparency stuff, just business as usual, it takes a lot of the hemming and hawing and, you know, stomach knots out of it. And we can just post the GD data and, uh, people can look at it and figure out what's going on with it. And so people don't have to spend a week trying to figure out whether they need to ask for the data and then another two weeks drafting a letter and, you know, uh, it's just so inefficient.
Benjamin James Kuper-Smith:Yeah, exactly. I mean, this is the, this is kind of the, I mean, in a way for me, you know, if I just take this as a one-off, it's been an interesting intellectual exercise at worst, right? Like, it's been interesting to also just for me to see, like how can I take a paper apart? And I learned a lot of stuff about my own research because you know, it is related some sense. Uh, yeah. I learned some stuff for that and it's, it's not like this has been completely waste of time or anything. Um, um, Jesus, again, I'm losing my thoughts today. Sorry. Um, I'm a bit all over the place, right?
Joe Hilgard:Open enough, a fresh, a fresh can of worms. Is there another, another, uh, now there's something else we should talk about or,
Benjamin James Kuper-Smith:Um, okay. So one question I had earlier then is I feel like in some sense you do have a, it seems to be, in some cases you do have a kind of obligation to say stuff though, right? I mean, so let's say my case is, okay, it's a bit of a border case. We go, okay. Probably some stupid error somewhere, and the main results are set the same and it's just correcting it. That would be a lot of effort for anyone involved for basically very little output. But then it seems to me, you do also have some cases where, you know, for example, I think what was also mentioned, like in this, in the science write-up of your, of your blog post or the situation that this, I can't remember who it was, but some other guy was doing a meta analysis included, like had to include it basically because it's not retracted. So it seems to be, sometimes you do have an obligation to actually speak out and say, I think this date, there's something really wrong with the stage. So how do we, where do we draw this boundary between Whoa, it's probably just a small error that doesn't matter. And this is something that has to be done if we want science to work as an enterprise. Yeah.
Joe Hilgard:That's a good question. I'm not sure. Gosh, how would you draw that boundary? Um,
Benjamin James Kuper-Smith:Well what are some good questions maybe to ask to differentiate one from the other or
Joe Hilgard:Yeah, well, in the case of Zhang's stuff, um, the sample sizes make it really kind of important to make sure that those numbers are right. He had, I want to say two different papers with a sample size of 3000 another with a sample size of 2000. And so if you were to put all these together in a meta analysis, like 80% of the weight of the meta analysis will be these results. So if those are wrong, any meta-analysis including these data are wrong. And it's, it's, it's very difficult as a meta analyst to say, I'm going to leave all this data on the table because I don't trust it because then it becomes, you know, you're being subjective, you're being unreasonable. You're, you know, excluding data, you're you, it makes you look like you're putting your thumb on the, on the scales. So I think we can kind of assess the relative weight and importance of some studies. Um, and we can roughly tell the difference between like a minor error versus a load bearing error. Um, which sounds like that's kind of the thing you're struggling with.
Benjamin James Kuper-Smith:Um, yeah, like basically I think my problem here is an accumulation of small errors at some point should become a load bearing error. Yeah.
Joe Hilgard:It does make, I mean, it sounds like you're kind of questioning the entire quality of the work given all the superficial.
Benjamin James Kuper-Smith:Yeah. Yeah. It's the base. Yeah. It's based like if in the final product, the thing that's been signed off by several authors gone through peer review, if there are so many small errors in this thing, how many went into all the stuff that isn't even documented, like the way they tested the people. Right. And you know, all this kind of stuff,
Joe Hilgard:It's like the Brown M and M's thing. Right. If they can't get the M and M's right. It means they didn't read the whole thing correctly, which means that there's probably something else that's also wrong.
Benjamin James Kuper-Smith:Yeah. So yeah, it just makes me distrust the, yeah. Yeah. Um,
Joe Hilgard:You know, I wish I knew how to tell small errors from serious errors. Right. Um, the thing again is that unless we can actually see the data and the code, we have no idea how deep the analytic errors run and in just the same way that unless we can see like the actual process that generated that data set, we don't know if that data set was generated by people typing in real data or Diederik Stapel staying up late at night, typing numbers into Excel on his own. Right. Um, there's just, it's, it's this whole, uh, mysteries zone that without at least open data and open code, you can't penetrate that first zone. And I'm not sure we'll ever be able to penetrate the second mystery zone of where the state actually came from. Um, I've had some ideas about what would be either for that, but I can't imagine anybody signing off on any of it because it involved a new layer of bureaucracy and audits to make sure that the data are what you say they are. And I just can't imagine anybody going for that politically.
Benjamin James Kuper-Smith:Yeah. I have to admit. I'm also not a fan of more beaurocracy.
Joe Hilgard:Yeah, no, I mean, I'm, I'm, I couldn't do it. I am terrible at paperwork and getting forms filed on time. It's my least favorite part of any job, especially this one. Um, one time as a grad student, I got audited by the IRB, just a random audit, not because I had done something wrong. Um, but it took the wind out of me. Like it just flattened me for a week having to deal with this IRB audit. So yeah. I, I mean, I say like, these are the things that we would need to be able to tell whether data sets are real or not. Um, but I don't think we, I don't think that's actually practical. Um, I think it would, it would be just a lot of burden and everybody would hate it. Uh, th th the psychologists would revolt.
Benjamin James Kuper-Smith:Yeah. Um, but so is the long-term solution is the more, we just adopt more open science practices. So at least some of the errors can be, um, you know, the kind of stuff that I have can be easy to check if I have the data. So that kind of stuff could just, I mean, that's in a way always already changing, right? Yeah.
Joe Hilgard:Yeah. I think that's the low hanging fruit, right. Is people post their data, people post their materials, people post their code. Um, so that in cases like the one you're dealing with, you can see what the numbers are supposed to be and how deeply the problems run as far as misconduct though. I think posting the data makes it a little bit easier to see the, to see the issues, right. When I got data from Zhang, it definitely revealed some issues that were not easily apparent, um, just by reading the reading of the articles, but even then editors have been reluctant to deal with some of those things. And so I think that if it's misconduct, you're concerned about, we also have to be asking how we keep journals and journal editors and institutions accountable, um, because as best I can tell editors and chief do whatever the hell they want, and nobody can say boo to them and institutions handle, um, investigations for scientific misconduct about as well as they handle any sort of internal investigation, which is that, uh, the purpose of the investigation is really just to make the thing go away. So I have not yet been impressed by the rigor of the university's internal investigation. So how do we change that? What are the things that we do to increase the accountability for editors and institutions? I don't know that yet.
Benjamin James Kuper-Smith:Okay. Yeah. I, okay. I think in general also, uh, I think we've run through my questions, uh, that I had. Um, I remember the, uh, before we started recording, ask you whether he wants to mention anything and, uh, you said two papers that I said we were going to bring them in smoothly. Um, I, that's not what happened. Um, do you just want to mention them briefly now, uh, and maybe have briefly introduce what, what yeah. What they are.
Joe Hilgard:Oh, sure. So one other nice thing is that our tool set,
Benjamin James Kuper-Smith:Uh, just one comment, I'll put the references for this and the description as for the other papers we've been discussing minus the one I mentioned, I'm not going to put the reference for that.
Joe Hilgard:Yeah. Um, so our, our, our tool set has been improving. Right. We've gotten new things, like, as you mentioned, GRIM and SPRITE from Nick Brown and James Heathers and folks like them. And in my own small way, I've tried to add to that tool set. Um, one of the things that suggests stupid fraud, um, is in fact, that's just way, way, way, way, way too big if you've been, so I'm in social psychology. If you've been in social psychology, you know, that our effects are not like massive, even a kind of big, obvious effect. Like conservatives say that social equality is less important to them than liberals do. Um, like that's kind of, you know, uh, a clear political difference. There's liberals say they want this more than conservatives do that difference is about seven tenths of a standard deviation. It's a Cohen's D of 0.7. That's kind of obvious effect. Um, in social psychology, most effects are going to be smaller than that. They're not going to be bigger and more obvious than that. So if I'm reading a paper and it says, um, we whispered the word equality into the room five minutes before subjects entered so that they would be subconsciously activated by the echo of this word. Um, and that effect is like one and a half standard deviations. I know that something is very seriously wrong, right? Either this is an extreme case of publication bias or, uh, somebody misplaced the decimal place, or, uh, maybe possibly there's a chance that somebody jammed up the data. So I have this paper out in JESP. Now that tries to estimate, okay, given this dependent variable, given this measurement that you have, what is the biggest possible effect you would see on it in a realistic sort of lab question? Would it be one standard deviation would be two standard deviations? Would it be three standard deviations? Um, because if you get that biggest possible effect and you see a paper in the literature that has a subtler manipulation, that yields an even bigger effect, something might be wrong. So if people are pouring hot sauce with greater reliability and precision and consistency than when you ask them to pour exactly the same amount, right? Suggests that there's something unusual about the consistency of those hot sauce pores. If you tell people, tell me what a mass murderer would do in this situation versus telling me what the world's nicest dad would do in this situation. And that gets you a smaller difference than people describing what a generic person would do after being primed by a violent or nonviolent movie. That again, suggests that something is off the effects are too good to be true. Um, so there must be some sort of mistake. So I, I think when we start thinking about effect sizes, we're going to have an easier time detecting some obvious mistakes, but as far as more sophisticated frauds or more subtle mistakes, um, I still have no idea what we'll ever do about that.
Benjamin James Kuper-Smith:Yeah. Uh, one point on the effect size thing, it's interesting, this is something I never really like, what would be too big and effects as not something I've really ever thought about too much. And then again, I never really had effects as were huge or saw them, but, uh, there was a, I think it's a blog post, but Daniel Lakens the hungry judges thing exactly. The hunger judges yet, where he says like this, if this isn't true, the effect size is too large, like if this was true, then we'd organize our entire lives around it. Yeah. Um, and I think the, the thing that I found interesting that as he mentioned, the, the effect size, um, of other things that are very large, for example, the difference in height between men and women. And I think they're the D the, the effect size is almost like 1.7 or something.
Joe Hilgard:1.7, 1.8 standard deviations. Those numbers come from, um, Simmons, Simonson, and Nelson, um, uh, talk they had called, um, uh, I think it's like beyond P hacking or life app life after P hacking, where they just try to get some obvious benchmark effect sizes for how much power you would need to detect some obvious things. So to detect men are taller than women. You can use your eyeballs. Uh, you could also use samples of about, I don't know, like eight or 10 per, um, and the point they were making was if you're studying something that is subtler than men are taller than women, on average, you're going to need more than eight or 10 subjects per condition. You're probably going to need a hundred, 150 per condition. Um, but this has been useful to me when I see a paper that says, Oh, uh, you know, we whispered the word activate into the work, into the room five minutes before subjects walked in and they showed a three standard deviation difference in behavior. You can say, no, you didn't. Because just as surely as we have men's size clothing and women's size clothing, we would have structured society around this massive effect of, you know, subconscious activation. Yeah.
Benjamin James Kuper-Smith:Yeah. And I've had it really interesting to just have like some real-world examples as yeah. As a benchmark to go like, Oh, okay. Like, yeah, if you have a effects, that's bigger than that. I mean, we actually, I had one thing that we did where we had pretty large effect sizes and then all that big, but it was kind of actually, that was one that was actually as big. And I thought this doesn't seem right now. Yeah. I had a coding error. I compared two completely different questions with each other.
Joe Hilgard:Yeah, absolutely. Yeah. So this is something that I think we're really lacking in psychology is we don't have good horse sense about how big an effect we should expect. One, our expectations are probably way too big, um, because publication bias and P hacking have led us to believe that all effects are big. Right. I th the joke around grad school was everything correlates at 0.4. And, uh, the, the horrible truth of that is it's not the case that everything correlates with 0.4 is that everything, when you filter for a statistically significant correlations at a sample size of about 40 or 50, the only things that reached significance are a correlation of 0.4. So our expectations are too high for what an effect size looks like in our fields. Um, but two, we really don't have a lot of experience thinking about plausible effect, sizes. Everything is so contextually sensitive to the population or the measurement that we're using or whatever, um, that sometimes people just say, Hey, look, I have literally no idea what effect is plausible here. Um, it could be an effect of 0.01 standard deviations, or it could be an effective 10 standard deviations. I have no clue whatsoever. And I have had editors make that argument explicitly to me, when I say, Hey, Stroop data, doesn't act like this. They say, well, but maybe Stroop data acts like this in this population and this age range under these circumstances, you really don't know. Um, I find that implausible, but I can't prove it right, because I don't have access to that population at that age, in these circumstances. Um, so we really don't have a lot of experience thinking about what sort of data is even likely, uh, we were, were acting like we were born yesterday. Each time we come to a new research question
Benjamin James Kuper-Smith:Yeah. Is, um, to bring it back to t he, y our paper, then what exactly is that the paper then? Is it a, u h, discussion about the topic?
Joe Hilgard:Oh, it's, it's a demonstration of just the kind of idea of saying, if you have a measure and you want to know how big, the biggest possible effect size could be on it, hit it with a sledgehammer and see just how much of an effect you get out of it. Um, and if you are, let's say running a meta analysis or a systematic review, and you see an author or a paper that reports effects that are routinely in excess of that, maybe you should be concerned about that author's output. Um, because if the biggest possible effect you could plausibly get on this measure is 1.8 and there's somebody out there reporting to two and a half, three, four. Maybe you should check on that guy.
Benjamin James Kuper-Smith:Yeah. Once I heard it, the example with height difference in men and women effects as a full, I wondering what that would even be.
Joe Hilgard:Yeah. You need like two per cell.
Benjamin James Kuper-Smith:Yeah. I mean, like the difference between like grown-ups and infants,
Joe Hilgard:James Heathers has found another example. That's like the effect of, um, opium on analgesia, right. So like, is this effect of being primed by this word or playing this video game or reading this persuasive essay as potent as, um, you know, getting a big dose of an opioid painkiller? Um, probably not.
Benjamin James Kuper-Smith:Yeah, probably not. Okay. Uh, that's one of them, the other paper.
Joe Hilgard:Oh, the other papers, just on the same, same general idea. Um, they're just two papers, both kind of exploring ways to think about effects that are too big and maybe take a poke at them and see if they are indeed too big or if the data are indeed too consistent, too reliable.
Benjamin James Kuper-Smith:Okay. And that's, uh, just so I can, for the references, uh, where did that happen?
Joe Hilgard:Uh, that was in psych science. That was comment on Yoon and Vargas.
Benjamin James Kuper-Smith:Okay, cool. Yeah, I'll put that in the description of the podcast. Um, yeah, I think, I don't know whether you still have anything to add. I think,
Joe Hilgard:No, I can't go through my stuff. I feel like that's, that's been about it. Um, yeah. Uh, thanks for, thanks for listening to me. Go on about this. Um, it's, it's, it's, it's cathartic, as you can see, I, I could talk about this for days. Uh, I've got a lot trapped at me right now. Um,
Benjamin James Kuper-Smith:Yeah, I mean, no, it's, uh, it's also been really interesting to me, for me, uh, you know, specifically about the, the example that I had. I don't know why somehow I expected you to say, okay, here's what you do. You contact the person, you use say like, not necessarily like you tell me exactly what to do, but I somehow assumed it would be more in line of like, yeah, yeah. Contact them. Of course.
Joe Hilgard:Great. If we had a consistent and clear flow chart for how to handle things like this. Um, but we don't. And even at the journal level, uh, there's the committee on publication, ethics has flow charts for what editors are supposed to do. When somebody writes in to say, Hey, I'm peer reviewing this thing and it looks fake or, Hey, you publish this thing and it looks fake. Um, and those are only so helpful, um, in part, because they tell the editor, you're not supposed to investigate, tell the university to investigate. And so if the universities are only doing like these, um, half-assed show investigations, um, then the editor is not going to be able to do anything about it.
Benjamin James Kuper-Smith:Yeah. It's just a circle of people asking other
Joe Hilgard:A circle of people ignoring each other's emails for eternity it's academia,
Benjamin James Kuper-Smith:And then you just writing. Well, yeah. I mean, I guess, yeah, it's really weird. Like, it seems to me like in part, I'm kind of optimistic about the whole thing, just because, you know, with more open data sets, a lot of these problems can be, um, you know, even like, as you mentioned, like in peer review, right. And a lot of this stuff can be found out much quicker. Um, and I think that's something that's changing fairly quickly also. And I mean, we even have this once that, so my, my supervisor was reviewing a paper and I kind of helped out. And, uh, one of the comments we had was, you know, like for the experiments you're doing, you could easily put like individual data points into, uh, the, the figures, right? Like there's no reason not to like, it's, it's, you can see everything clearly. And it would be useful to kind of know what what's going on. And then like a bit later we saw a talk by the, by the people who, uh, had submitted this paper. And, you know, now there were data points and all the figures. Yeah. And, you know, it's, it's weird how, like, just, I mean, it's almost weird, like seeing your actions actually have an effect, uh, quickly. Um, but so I think like that kind of stuff I think is changing fairly quickly, but, you know, as we said, right in the beginning, um, the actual fraud is, uh, more or less if you're halfway intelligent about it. Yeah. Undetectable. Yeah. So that's not optimistic then.
Joe Hilgard:No, no. Um, I, again, I, I, I, I do the things that I do because I think it's helpful in the long run to bring a little bit of attention to this. Um, I've got in my drafts folder, basically a how to guide on committing fraud and never getting caught. It's a little bit satirical. Um, I don't know if I should submit it or not, uh, because I don't know if it would actually, you know, help people address it or if it would just become the, the, how to guide for how to actually commit fraud. Um, I don't think anybody would ever actually publish it, but I do think, um, I don't know, I'd be interested in reading. I think there's value in getting everybody thinking about this problem, um, because it is so hard to clean up. Um, and the more we think about it, I think the better, our chances at reaching some sort of institutional change that makes it a little bit easier to deal with. Yeah. I mean, I'd be happy to read, I think other people would be too. And so I think there's also like there's a paper by Karl Friston about it's called something like statistical device for non-statistical reviewers or something. And it's like how to give advice if you don't know statistics. Um, and it's, you know, it's, it's a sarcastic or ironic kind of thing where like, one of those, like always ask for more samples, larger sample sizes like that. Um, and kind of just, uh, I CA I mean, I read this like my master's, so a few years ago, so I can't remember exactly what it's about anymore, but I think when I read it, I was like, Oh yeah, these awesome fairly, generic, like criticism that people have that don't really mean that much. Um, so I think, you know, in a way, this is also an article that's kind of a tongue in cheek, but fulfills a purpose. So I think, okay. Going back to that one and I'll, I'll see if maybe that can give me a little guidance
Speaker 1:[inaudible].