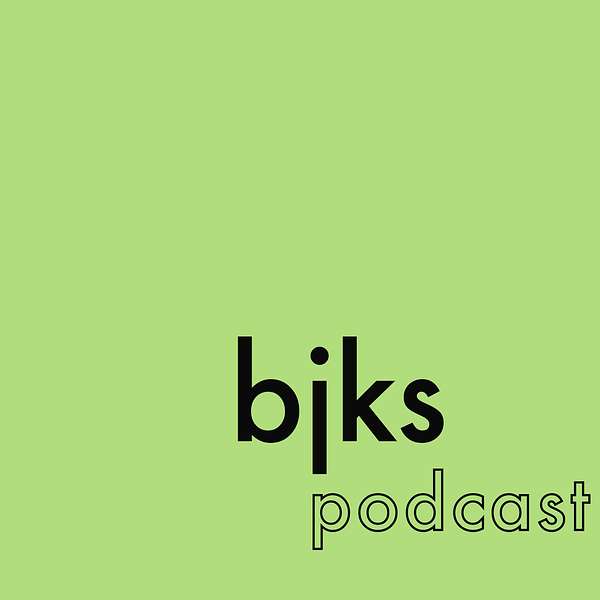
BJKS Podcast
A podcast about neuroscience, psychology, and anything vaguely related. Long-form interviews with people whose work I find interesting.
BJKS Podcast
94. David Van Essen: The Human Connectome Project, hierarchical processing, and the joys of collaboration
David Van Essen is an Alumni Endowed Professor of Neuroscience at Washington University School of Medicine in St. Louis. In this conversation, we talk about David's path to becoming a neuroscientist, the Human Connectome project, hierarhical processing in the cerebral cortex, and much more.
BJKS Podcast is a podcast about neuroscience, psychology, and anything vaguely related, hosted by Benjamin James Kuper-Smith.
Support the show: https://geni.us/bjks-patreon
Timestamps
0:00:00: David's childhood: ravens, rockets, and radios
0:05:00: From physics to neuroscience (via chemistry)
0:13:55: Quantitative and qualitative approaches to science
0:19:17: Model species in neuroscience
0:31:35: Hierarchical processing in the cortex
0:46:54: The Human Connectome Project
0:55:00: A book or paper more people should read
0:58:01: Something David wishes he'd learnt sooner
1:00:31: Advice for PhD students/postdocs
Podcast links
- Website: https://geni.us/bjks-pod
- Twitter: https://geni.us/bjks-pod-twt
David's links
- Website: https://geni.us/VanEssen-web
- Google Scholar: https://geni.us/VanEssen-scholar
Ben's links
- Website: https://geni.us/bjks-web
- Google Scholar: https://geni.us/bjks-scholar
- Twitter: https://geni.us/bjks-twt
References & links
David's autobiographical sketch for the Society for Neuroscience (in Volume 9): https://www.sfn.org/about/history-of-neuroscience/autobiographical-chapters
Felleman & Van Essen (1991). Distributed hierarchical processing in the primate cerebral cortex. Cerebral Cortex.
Glasser, Coalson, Robinson, Hacker, Harwell, Yacoub, ... & Van Essen (2016). A multi-modal parcellation of human cerebral cortex. Nature.
Hubel & Wiesel (1962). Receptive fields, binocular interaction and functional architecture in the cat's visual cortex. The Journal of physiology.
Maunsell & Van Essen (1983). The connections of the middle temporal visual area (MT) and their relationship to a cortical hierarchy in the macaque monkey. Journal of Neuroscience.
Sheldrake (2021). Entangled life: How fungi make our worlds, change our minds & shape our futures.
Van Essen & Kelly (1973). Morphological identification of simple, complex and hypercomplex cells in the visual cortex of the cat. In Intracellular Staining in Neurobiology (pp. 189-198).
Van Essen & Maunsell (1980). Two‐dimensional maps of the cerebral cortex. Journal of Comparative Neurology.
Van Essen (2012). Cortical cartography and Caret software. Neuroimage.
Van Essen, Smith, Barch, Behrens, Yacoub, Ugurbil & WU-Minn HCP Consortium. (2013). The WU-Minn human connectome project: an overview. Neuroimage.
Wooldridge (1963). The machinery of the brain.
[This is an automated transcript that contains many errors]
Benjamin James Kuper-Smith: [00:00:00] Yes, I mean, as I mentioned before we started recording, uh, you have this nice biographical sketch for the Society for Neuroscience that I will, that I read and that I'll use heavily, kind of just to structure our conversation to some extent. And I thought, uh, I'd, uh, start with some of your kind of childhood observations and in particular some of the ones that relate a little bit more to the scientific aspects.
And I'm curious maybe whether you could say, uh, Who is Herci, uh, or did you, what did you do with him?
David Van Essen: Herky is short for Hercules, who was a raven that I acquired as a, about a ten year old child. A friend of my father found this raven with a broken wing out in the wild and brought him to me. And because his wing was broken, it healed, but he wasn't able to fly at least far enough to escape our backyards.
So he lived with my, in our family and [00:01:00] ate. Everything from day old hamburger to ice cream to various and sundry items and, and spoke a little, but not, wasn't, didn't have a voluminous vocabulary, but. He was definitely an important part of my early upbringing.
Benjamin James Kuper-Smith: You mentioned that you tried to, uh, broaden his vocabulary. How, how did you, maybe how old were you at the time? And like, how did, how did you try and teach a raven to talk a bit more? Or understand more, I'm not entirely sure which, which part of vocabulary.
David Van Essen: Well, he, he just picked up things that were said, and I was in school, and, and so I, you know, I not that I spent hours and hours with him, but I
would always go out and feed him every morning and night and, and chat with him and put him on my shoulder or my forearm and, you know, just take him around our, our yard.
But he certainly didn't speak in the way that we could have actual conversations. It was just, when he was on his own, mainly he You could listen [00:02:00] to him from our porch, for example, and hear him shattering away at times, so. It was fun, but, like I say, it wasn't a wreck on tour by any means.
Benjamin James Kuper-Smith: Um, yeah, the other thing I was kind of interested in is that you mentioned that you were very interested in designing rockets, in particular one that could transport you and your entire, I don't know, maybe not entire extended family, but, uh, quite a lot of members of your family. You were building radios, uh, to communicate via Morse code.
I'm just curious, kind of, uh, other than just being good fun, is there anything that you kind of learned from, from those hobbies or, you know, was it just a part of a fun childhood?
David Van Essen: Uh, it was definitely, as a child, I was absolutely fascinated by science, and I remember one of the best Christmas presents I ever got was an encyclopedia, and I would read it voraciously, [00:03:00] just try to go through from major alphabetically organized sections and just learn and absorb as much as I can. In broad strokes, but definitely had a focus on science and, uh, since, you know, the, uh, Sputnik, uh, first Russian satellite was orbited in, I think, 1956. And I was basically 11 years old at that time, and that, that drew my attention to, uh, The, what was happening in outer space and the struggles the U. S. had to get into orbit, as it were. And so, the context in which I was drawing rockets were based on what was happening in those early days of the so called space race.
Benjamin James Kuper-Smith: Yeah, I mean, I guess you were, it, it sounds like it, kind of a, a kid interest in this stuff at just the right time when it kind of started to go. I mean, for me, I was never that interested in it, you know, obviously it was before my time, but it, it sounds like it's, I'd [00:04:00] imagine you were, you were following that pretty excitedly and I guess, including the landing on the moon.
David Van Essen: Yes, that was a decade later, and actually my wife and I were on our honeymoon when we witnessed the moon landing. And we were traveling around in California in our little Volkswagen Beetle and went camping and then we visited her, Isabel's, an old family friend who was quite old enough that she wasn't entirely in her, in command of all her senses and she was, she believed the whole moon landing was a giant hoax. And so it's interesting in the context of today's world of tremendous amounts of disinformation. Uh, even back then, decades ago, disinformation was part of, part of the equation that one had to grapple with.
Benjamin James Kuper-Smith: Yeah, it's funny that that's still, I think there's still people who believe in that. [00:05:00] You had a, you know, as you were kind of considering where and what to study, you had this nice little paragraph in this biographical sketch that I'd like to read out and kind of have you comment a little bit about. So you wrote, In keeping with my passion for science, my first choice for college was Caltech in Pasadena, near my Southern California birthplace.
I visited Caltech in 1962 and remember being enthralled by a talk to prospective students given by Richard Feynman, a theoretical physicist and prankster who was already a legend in his time. In the spring of 1963, I was thrilled to receive a letter of admission to Caltech plus a scholarship, which was vital, given our family's modest means.
So, yeah. Do you, could you say a little, a little bit about that? I find the idea quite funny, that Feynman would spend some of his time talking to students to convince him to come to Caltech. Uh, but I'm just curious kind of what, uh, do you, do you remember that
David Van Essen: I [00:06:00] do. Feynman was a great teacher and a great communicator. And he, back in the early 60s, decided that classical physics was not being taught to Caltech undergraduates in a way that would, you know, stimulate their, their deep thinking, uh, out of the box thinking rather than just learning a bunch of physics as a bunch of equations and rules and laws.
So he was able to provide I had an intuition for how things worked and how abstract, arcane aspects of physics could be thought of in creative and informative ways and so, like I said, I was able to capture one of his lectures at Caltech where he was essentially trying to recruit bright young people to come to the different nature of Caltech as a learning institution.
And so, I got there and unfortunately, [00:07:00] Feynman no longer gave the First year physics course lectures, but we had a book that was a set of his lectures and the instructor of the course used that book as a basis for our course and that was how I learned physics and how I learned both that I It would love to become a serious theoretical physicist, but I came to realize that my brain was not wired in such a way that that. It was a great match. I just, I couldn't think deeply enough about the equations and what they signified that I would have confidence I could make a go a career of theoretical physics. So that moved me over to what do I do now? And I gravitated towards chemistry in part because I was getting excellent grades and learning [00:08:00] material, but it was not. It wasn't as fun and exciting as physics had been, and so I felt in a way that I was, I wouldn't say going through the motions, but just not as thrilled by what I was studying. And that was true also for my first two summers while I was in my undergraduate years. I was able to work in biochemistry labs, um, Dr.
Vinograd, I remember, was my, my mentor and he was a cantankerous but very engaging fellow and I enjoyed doing the experiments, but the transformative moment for my career at that stage was that summer I happened to be perusing the Caltech bookstore, which is Not a giant store by any means, but I, I saw this little paperback book [00:09:00] called The Machinery of the Brain. And I'd never really thought much about the brain before, but I said, oh, that looks like fun reading. And so I grabbed the book, read it voraciously, and made a phase transition to being fully committed to neuroscience as a career, and that had never wavered.
Benjamin James Kuper-Smith: I've, I've never heard of the machine with the brain, uh, by Dean Woolridge. So maybe, uh, what was. Uh, was it was a popular science book? Was it a textbook? I mean, kind of what, what was it about? What did you like about it?
David Van Essen: It was meant for, you know, the intelligent lay public, and Dean Woolridge was not a neuroscientist, he was a An engineer, a founder of, co founder of TRW, uh, Thompson Raymo Woolridge, a defense oriented, uh, company. But he got fascinated by the brain and he timed [00:10:00] to go around and talk to people, learn about the brain and present some of the exciting fundamentals and discoveries that were being made. Now in the mid 60s, for example, he explained the pioneering work of, uh, David Hubel and Horson Weasel. And, Who later got the Nobel Prize for their work at Johns Hopkins University, then later at Harvard. And, uh, really transformed our, uh, collective understanding of how information is encoded in the visual cortex of, of mammals and in particular the cat, the visual cortex of what they studied. And. That captured my fancy, to put it mildly, and years later I had the good portion of working with Hubel and Wiesel, and we might return to that in our conversation.
Benjamin James Kuper-Smith: Yeah, definitely. Uh, I'd have a brief question just to go back a little bit. Um, you mentioned that you, while studying physics, no, you know, noticed that you didn't, as I said, uh, [00:11:00] have, have kind of the brain for physics for that kind of thing. I'm just curious, how do you notice that kind of thing? Is it just, you know, it was just really hard and your grades were terrible and you spent All your time trying to figure out basic things or, uh, was it that you didn't like the kind of thinking you had to do or, you know, I'm just curious how do you, because that's, you know, it's, it's, it's often, I don't know, I guess it depends on the person, but I'm interested in many things and for me, it's always really nice if I can exclude something from the things I'm, I might consider spending lots of time on.
Um, so I'm just curious how you make that realization that this or that kind of thing isn't for you.
David Van Essen: It certainly wasn't the case that I was terrible at physics. I was getting A's in physics course in physics lab, and enjoying it, so I was not by any means lost in the field. I just, I remember, for example, there was a, there were opportunities to go to lectures and talks, and I heard a [00:12:00] presentation by Murray Gilman, uh, who was. At that time developing his, again, later Nobel Prize winning work on quarks. In the course of that and other scenarios where I was trying to Latch on to these very abstract and deep thoughts. I can say I found it fascinating, but I, I had the feeling that it just wasn't the right fit for me. And it's possible I was wrong, that I could have made that leap.
But honestly, I don't think so, because Years later, when I got into computational neuroscience, I had a colleague, a collaborator, Charlie Anderson, who was a physicist and engineer first, and became interested in neuroscience. And our collaboration over more than two decades worked because Charlie was master of the [00:13:00] equations, and I could understand what he was getting at and help put it together. into a neurobiological context, but I just couldn't, then couldn't, as my, going back to my undergraduate career and, and cannot now grasp and manage the, the complexities of many mathematical formulations of what's going on in the brain or how one analyzes it. I understand how important they are and I get the gist of it, but I need to work with others who are. really facile with the math, because that's not my forte, much as I respect and am fascinated by all types of math.
Benjamin James Kuper-Smith: Yeah, it's funny. You had, I thought I'd mention it later, but I guess I can ask about it now [00:14:00] is about your postdoctoral training. Um, kind of relates a little bit to the, the quantitative qualitative difference. It's not exactly what you just mentioned, but I, I, I thought it might kind of fit in this thematically.
So you, you wrote my postdoctoral training in visual neurophysiology had emphasized qualitative rather than quantitative approaches to analyzing and reporting receptive fuel properties. This bias was most colorfully articulated by David Hubel, who famously declared that, quote, If you need to do statistics on your data to know whether the results are significant, then the results are probably not very interesting, end quote.
However, my perspective, yeah, changed once at Caltech. Um, this kind of contrast between qualitative and quantitative science. I was a little bit surprised when I read that quote, because I guess to Someone like me who comes from psychology, biology seems like, you know, the, the, the quote unquote harder science.
There's this sense that, I don't know, I guess [00:15:00] a vague heuristic is that it would be more mathematical, that kind of stuff. But I'm just curious, just because you mentioned the kind of different abilities and how you are very good at collaborating with the people who are maybe more mathematical. Yeah, maybe the advantages and disadvantages of the two approaches or kind of just how you think about those two different approaches to doing science.
David Van Essen: Sure, so, as I said, Hubel and Wiesel were able to explore the properties of neurons in the visual cortex using just very qualitative listening to the sounds of neurons firing away and telling the difference. At a glance, whether a neuron was screaming away because the stimulus was very effective at one orientation, for example, and when the orientation was changed, just a little, the response was audibly, listening to the loudspeaker, much weaker, and moving the orientation [00:16:00] to an orthogonal, uh, to the great, the best stimulus, the response would be absolutely silent.
And so, that's what I mean by qualitative descriptions. You don't need a quantitative numerical account to discern that degree of specificity. But as it turns out, neurons are, in the visual cortex, are tremendously diverse and Responsive to varying degrees to many different kinds of stimuli, and sometimes the subtle difference between one stimulus and another must be characterized quantitatively to know whether you can believe it, whether it's real signal or just noise. And then one also needs to appreciate the fundamental multidimensionality of response characteristics in the brain in general and in the visual cortex in particular. So rather than just thinking that because a neuron in the visual [00:17:00] cortex responds best to vertical and not at all to horizontal stimuli, many people in the field had gravitated towards saying, okay, let's just call this neuron an orientation detector because It's, it's job is to detect vertical stimuli, but in the larger context I was alluding to, if one finds also that this cell is modestly selective for the color of a stimulus and moderately selective to the direction in which it's moving, and also is influenced by the what we call binocular disparity, the depth in the visual field and the distance from the eyes, one can. see that many neurons in the visual cortex are selected to some degree to many, possibly dozens of different stimulus parameters. And so to capture that and to grapple with the implications of what we [00:18:00] might now call multi dimensional or multi modal tuning response characteristics. It really becomes essential to have a quantitative description of how neurons respond across many different features and stimulus types.
Benjamin James Kuper-Smith: Is that difference an indication for, it just occurred to me that something similar, I, um, I was recently talking to, um, Nachum Ulanowski, um, who does spatial navigation in, uh, bats and, um, we were talking about all the different kinds of cells that, cell types, there are place cells, grid cells, and How, you know, these are just, they're more and more types and that kind of stuff and just kind of seeing that analogy, I'm wondering, is that maybe a sign of, you know, when the field is very new and you can make these like big fundamental findings and qualitative approaches are fine, but once kind of the field matures a bit to get at the nuances, uh, you need this more quantitative thing, or is [00:19:00] that, uh, just a coincidence that it wasn't this field?
David Van Essen: That's a fair general observation that, uh, works well for many fields, many sub fields of neuroscience.
Benjamin James Kuper-Smith: Just returning briefly back to your pre PhD days. Uh, I'm just curious, you mentioned that you took a course with Max Delbrück. It's funny, I, I, so I grew up in Germany and so the name Max Delbrück is pretty common here and they tend to name lots of things after him. Uh, but I don't actually know what he, what he did in any detail.
I'm, I'm just curious kind of what, what courses did you take with him? What was he like as a lecturer? And yeah, is there maybe something you learned from him?
David Van Essen: So he, like many biophysicists and people coming from other domains, got interested in the brain relatively late in his career. That was through Seymour Benzer, who was also at [00:20:00] Caltech, and many others. Delbruck, in his case, got interested in thinking about a, um, I'm trying to remember the name of the species, I think it was a small fungus that was photoresponsive, so it would grow up and point its head towards the light and follow, follow that. And Delbruck thought this might be a good bondal system to, to study, but it's an example actually of intuitions that aren't always successful because, uh, Delbruck never got that really going strong in his lab. It sticks in my mind only because I took a course that he taught, and this was one thing that he focused on in the course. But actually I would contrast that with Seymour Benzer, who I mentioned previously. Benzer [00:21:00] was also a molecular biologist, molecular geneticist, who had established a strong claim to fame from work decades earlier, but he came to Caltech in the mid 60s. I guess it maybe was late 60s. It was right around the time that I was an undergrad there. Benzer made his bet on fruit fly Drosophila as a model system for neuroscience. Drosophila had been intensively studied at Caltech as well as other institutions for decades for many different aspects of fly genetics and studying development and various characteristics of the fruit fly's functional organization. But Benzer was the first one to really Focus on the nervous system, and that has turned out spectacularly well, but to circle back to [00:22:00] David Hubel, I remember being in Cold Spring Harbor in 1990, there was a meeting on the nervous system, and Hubel was there and Benzer was there and Benzer gave his lecture on why he thought Drosophila was a great model organism and Hubel in an informal chat I had with him soon after that talk basically expressed his skepticism that genetics was going to be all that critical, all that valuable for studying. The nervous system, so I think it's clear now that Benzer was on the right track, wildly successful, uh, in the studies of Drosophila, including circling back to computational neuroscience. I think Drosophila is one of the spectacular success stories of computational neuroscience in the [00:23:00] modern era because it's possible to actually model in elegant detail.
structure, function, and actual neural computations with a granularity that can't be matched anywhere else in the brains of any other creatures studied to date.
Benjamin James Kuper-Smith: It's interesting thinking back to that because, you know, to me, someone who. You know, basically in the last 10 years, let's say has learned a little bit of neuroscience, you know, all of this is standard and it's, you kind of take it for granted. And like, you know, there's these different, uh, model species you have, and it's easy to assume that, you know, it was always that way, but I guess it had to be established at some point, of course, what were the kind of, uh, model organisms used in the, in the sixties?
Um, I mean, when I just go through some of the stuff you worked in, it's, it's, Yeah. A lot of different species, and I don't know how representative those are, but I'm curious kind of what were the, what kind [00:24:00] of species were people using back then, and why, or for what kind of purpose.
David Van Essen: It certainly was a much narrower spectrum than we currently have, but when I got to Harvard as a graduate student in 1967, I was strongly inclined towards studying a so called simple nervous system. Based on, in part, the, this Machiner of the Brain book that I'd read, but also the neuroscience courses that I'd taken while at Caltech in my junior and senior year, uh, as an undergraduate there. And so I became enamored of the so called simple system approach, and when I got to Harvard, uh, I found that there wasn't any lab there that was a great, match to my interests until, by good fortune, in the midway through my first year there, John Nichols joined the faculty. John had previously [00:25:00] worked with the Harvard Department Chair, Steve Kufler, but Nichols had gone down to Yale and I set up a laboratory studying the leech nervous system and, and John brought that model system back to Harvard and, and I immediately contacted, uh, Nichols and, and found it was a good fit in terms of both his model system and the opportunity to be mentored by him was, was a great experience, uh, for me.
And so that was how I got into, My thesis were, and did focus on, on the leech, and thought for a while that was going to be the, the, the direction of my long term scientific career. But, Again, by happenstance, uh, John Nichols lab was right next to the Hubel Wiesel lab, uh, and they were [00:26:00] doing the pioneering visual cortex explanations, explorations that I talked about a few moments ago. Then the opportunity arose to jump from leeches to mammalian visual cortex, basically because of a, a new methodology that I was able to use in my thesis work on the leach, and that is studying the morphology of individual functionally identified neurons using a fluorescent dye called Procyon Yellow that had been shown by Ed Kravitz in the Harvard Neurobiology Department. Just a couple of years earlier, that, that fluorescent dye could be used as a cellular marker. And so, I used protean yellow dye in my studies of sensory processing in, in leech mechanosensory cells. And [00:27:00] that was very helpful for those studies, but it also offered a window of opportunity to Explore visual cortex in mammals using the same general approach, what had become by that time known as the stick and stain method.
And so I teamed up with Jim Kelly, who was a graduate student, had been a graduate student of Max Cowan at Washington University. We came together and spent a couple of years as postdoctoral fellows in the Hebel Wiesel lab. injecting cortical neurons with this fluorescent dye and trying to test the hypothesis that the hierarchy of visual processing within cat visual cortex had an anatomical morphological correlate. So in a bit more detail, Hebel and Wiesel had identified what they called as simple cells, whose properties could best be explained or could be readily explained in [00:28:00] terms of getting direct inputs from the Incoming sensory fibers from the lateral geniculate nucleus, whereas the second cell type that Hubel and Wiesel found in, in the cat were so called complex cells where it was made more sense to think of the complex cell receptive fields emerging from, not from the direct geniculate inputs, but rather from the first stage simple cell neurons in the visual cortex. And so We obtained enough data to make a story out of that, which was, in essence, in a qualitative way, the simple cells had typically a so called stellate cell architecture, and were located in the middle layer four of the visual cortex, where the geniculate inputs are most dense, [00:29:00] and the complex cells were more concentrated. external layers, uh, and had a pyramidal cell architecture. So that was qualitatively appealing and, you know, fit the, the mindset of the day that the visual cortex had it. within just the primary visual cortex, a hierarchical organization. In retrospect, it gradually became more and more clear to me as well as many others that that was a serious oversimplification and that the way that visual cortical neurons obtain their orientation selectivity and obtain these characteristics that we call complex receptive fields is fundamentally more complex by, in terms of the number and nature of the interactions between different cell types.
So It wasn't that [00:30:00] our original story was completely wrong, but it was very important in my thinking about the brain to realize that a deep understanding of how the visual cortex actually works requires a much more sophisticated characterization of a highly complex network that has Forward going and so called feedback and lateral interactions amongst this network of neurons that is needed to explain how the cortex works, which is not simply detecting neurons of a given orientation, but rather inferring orientation and many other characteristics. In the context of a natural world, a visual world that is not dominated by just lines and sharp contrasts, but rather by rich [00:31:00] textures and colors and motion and dynamic interactions, and that to decipher how the cortex works in a natural, rich environment requires a much more sophisticated characterization. and modeling of the visual network at the level of an individual area, let alone thinking about the interactions between cortical areas, which is where my recent career has much more focused.
Benjamin James Kuper-Smith: Yeah, I mean, I wanted to talk a little bit about hierarchies in the cortical structure, because that's also how I, and probably many people, first heard about your work with, was it von Essen and Fellermann, 1991 or something like that, your, I think, most cited article. Was kind of this work with Hubel and Wiesel the first time you started thinking about, kind of, cortical hierarchies, and kind of how did that interest [00:32:00] develop, kind of, over the next decades?
David Van Essen: When I left Harvard after this postdoc with Hugh and Weasel, I was actually in a, an interesting trajectory, uh, because I had decided to work as a postdoctoral fellow, uh, with a Norwegian colleague, Jan Jansson, working on simple systems, uh, because of the mindset and perspective I'd gotten in the Nichols lab. And so I had a very productive and interesting time at working in Norway with Jan Jansson. But, as I said, I had also become fascinated by the visual cortex and I decided to jump over to continue to work on the visual cortex but in macaque monkeys, not cats. And for that I spent a year as a postdoctoral fellow with Senor Zeki in England and then moved on to my faculty career at Caltech. [00:33:00] But as part of that Entry into monkey visual cortex, I was committed to moving past just the primary visual cortex and explore the collection of visual areas in the so called extrasparate cortex, which is in monkeys, is much more extended than the primary visual cortex. And moving in that direction, I guess the first really important facet of how I So, what I mean by that is if one looks at the cerebral cortex and recognizes that it's a sheet of tissue actually. two independent sheets for the left and right hemispheres. And to fit inside the skull, the human cortex, you can think of as a 13 inch or [00:34:00] 30 centimeter size pizza sheet of tissue that has to get highly crumpled to fit inside the human skull. And the macaque monkey, which I was studying in the Zecchi lab, is essentially a chocolate chip cookie size sheet of tissue that gets crumpled to fit inside the monkey skull. And that folding, or convolutions, is critical in terms of making maps of the brain, because In order to study the anatomy, one has to, particularly in those days, physically remove the brain and slice it up and get a complex set of histological sections where the sheet like nature of the cortex is much harder to remove. to track. And so I use the analogy of the Earth's surface, which is a sheet of the interface between the [00:35:00] Earth's core and the external world. And, and you can think of the Earth's surface as a convoluted set of mountains, hills, and valleys into which billions of People are dispersed in patterns of organization that we call political subdivisions, countries, districts, and other political or cultural domains. And by analogy to the The cerebral cortex, the billions of neurons in the cerebral cortex are organized into distinct cortical areas based on the different characteristics of the many neurons that populate the cortical surface. To study the Earth, we make maps, flat maps of the Earth that were quaint at the time of 16th and 17th century explorers and cartographers, now vastly more sophisticated with Google Maps and [00:36:00] Google Earth and the like. For the cerebral cortex, what I realized was that we were missing a way to make maps of the convoluted macaque cortex. And instinctively, I knew that this would someday become a good job for a computer, but computer programming and technology was not up to snuff, basically. So I instead developed a manual pencil and tracing paper method of making flat maps of the monkey's cerebral cortex. And that helped to set a, an important framework or representational approach to showing the layout of many different cortical areas that turned out to be dozens rather than just a handful, as people had originally speculated. It also provided a framework for analyzing the wiring, the connectivity between different [00:37:00] cortical areas, which my lab and a number of others spent much of our effort in the 80s and 90s, exploring using anatomical tracer methods. And that led into this situation, the Fellman and Van Essen hierarchical approach that you alluded to. It actually started with my graduate student, John Mansell, who did his thesis work studying the functional organization of one of these visual areas known as MT, the middle temporal area. And John was able to characterize quantitatively many different features of MT functional organization, but also to make tracer injections into MT and characterize the rich, collection of inputs and outputs to MT, which he realized did not fit the simple serial hierarchy going from one [00:38:00] area to another, V1 to V2 to V3 to MT and so on, in a linear sequence, but rather the Thank you. The richness of connections and the distinctive layering pattern of inputs and outputs led John to hypothesize that there was a cortical hierarchy that had many levels, but multiple areas existing at each level and the distinction between levels based not just on who is connected to whom, but how they're connected in terms of this, the layering patterns I alluded to before. So Dan Fellman and I took that and ran much further with it by characterizing not just from our own laboratory, but from the relevant literature of hundreds of studies over decades of analysis. And we developed this framework that we called Distributed Hierarchical Processing that had 30 different areas interlinked by several hundred [00:39:00] connections. And a hierarchical framework of 10 distinct levels that isn't the final story by any means, but it was a, a kind of end point in terms of how my lab attacked and wrestled with these issues, because We were using tediously generated manual flat maps to characterize the lay of the land and using tedious anatomical tracer counting and mapping methods that didn't scale well, especially if one thought about applying similar approaches to the human. So I became convinced that For my own efforts, I wanted to move into what one can think of as computerized cortical cartography that is still focused on making maps of the cerebral cortex, but doing it with computerized methods. And so, Our lab moved in that direction, other labs were also very [00:40:00] successful in generating the toolkits that can capture the rich three dimensional structural organization of human as well as a monkey's cerebral cortex and to capitalize on the spectacular advances made possible by magnetic resonance imaging, including structural imaging that allows one to capture the detailed shape of the cortical fold or convolutions and to represent them in accurate three dimensional models that can then be Turned into surface maps that can be inflated and flattened, uh, to make modern representations. Still in the spirit of the flat maps I pioneered decades earlier, but now with a much more detailed and, and rich infrastructure for exploring structure function and connectivity [00:41:00] in the cerebral cortex.
Benjamin James Kuper-Smith: Maybe just briefly about hierarchical processing. I mean, what does the hierarchy exactly buy you? Like what's, what can you do with it that you can't do without it? And maybe related to that, then how kind of evenly distributed is this kind of hierarchy across the brain? Or is it more prevalent in some compared to other areas?
David Van Essen: So, the notion of hierarchical organization, I think it is, in many ways, become more complex at the systems level that we're now discussing just as the intercore, the within area hierarchy that I talked about, Hebel and Wiesel model, becoming richer and more complex as we better understand the internal circuitry of individual cortical areas, uh, thinking of this network of many cortical areas and also subcortical structures that they're interfaced with [00:42:00] thalamic and basal ganglia structures that are also a hugely important part of this equation. The nature of signaling and interactions and computations in the, Larger cortical network really cries out for essentially a new generation of computational model that is being approached by many investigators. Some coming from the kind of network domain of studying networks from airports to populations in the human domain that can be modeled by network science methodologies. This to me is an intriguing opportunity and an exciting area of exploration, but I think it's also tremendously challenging because we, for all the advances that are emerging, from the domains of human [00:43:00] neuroimaging, there are fairly serious limitations with even the best experimental data that one can get at this stage.
So, for example, conictomics has become a very major enterprise in human and non human primate circuitry. But the imaging based methods that have developed from the Human Connectome Project and other enterprises involve using two very indirect methods. One of them often called structural connectivity and it's based on diffusion imaging which It relies on the preferential diffusion of water molecules along the length of axons rather than trying to escape across tiny axonal membrane of the axon ultrastructure.
And diffusion imaging gives us strong clues about the direction of major fiber bundles in the white matter, [00:44:00] but the actual complexities of the wiring in the white matter is Much greater than can be accurately inferred by even the best, uh, uh, diffusion imaging methods to date. So, diffusion imaging and structural connectivity give us good starting estimates of connectivity patterns, but it's It's inadequate to map the richness and precision with which actual connections are made. Another parallel method is called functional connectivity, which relies on the functional MRI response that is strongly correlated with neural activity in individual domains and has had its most obvious successes in identifying activation patches and patterns of neural responsiveness to various [00:45:00] tasks that have been successfully explored and exploited.
But there's a different realm called resting state functional connectivity that involves acquiring these signals even when an individual is not doing it. particular instructed task, but it just is at rest in the scanner. And the brain is very busy in that context with fluctuating signals that are often correlated between nearby and also very distant regions, and those correlations are often lumped under the rubric of functional cognitivity because they are similar to the patterns one might expect from anatomical connectivity, as observed in the monkey tracer injections that I described earlier. But functional connectivity is also fraught with biases and artifacts that one [00:46:00] has to be very careful to avoid over interpreting. So we're in the realm of incredibly rich, detailed, quantitative information about these structural and functional connectivity measures, but it is very challenging to put these into a modeling framework that captures the actual richness of human neural function and how it relates to human behavior. So I think the field is at an exciting stage, but I think one has to be very critical and cautious and not over interpreting simple inferences that are appealing but unlikely to reflect the full richness of what's going on inside the skull.
Benjamin James Kuper-Smith: Uh, you mentioned the Connector. Uh, so I guess we might as well move to [00:47:00] stuff you've been doing. Well, I guess we have to some extent moved to the Stuff you've been doing more recently with the Human Connection Project. Maybe whilst you kind of ended on the, you know, difficulty interpreting different tasks, uh, and all that kind of stuff.
I'm curious whether, I mean, maybe just first a brief introduction to what the Human Connection Project is. And then, uh, kind of, how do you With this kind of large scale effort to decide what kind of, like what measurements are you going to use, what, you know, tasks that you're going to use, which modalities, et cetera, you know, since you have to think so carefully about, uh, interpreting different, uh, results, you know, at some point you have to make decision, which one of these are we going to acquire now on mass.
Uh, so kind of, how do you make that decision?
David Van Essen: So the Human Connectome Project was announced as an endeavor that the NIH was going to invest in back in 2010. And that, for me, was a very serendipitous and fortuitous time, in part [00:48:00] because the tools that we had been developing in our lab for computerized cortical cartography had come along nicely to the level that they could be applied to an ambitious endeavor such as mapping the human connectome. And also, I had been I was fortunate enough to recruit an exceptionally talented MDPH student, Matt Glasser, whose interests and expertise were complementary and very synergistic with my own and fit very well into the domain of what the Human Connectome Project was focused on. So, Matt and I and a number of faculty at Washington University put our heads together when this opportunity came onto the radio screen in 2009 as an opportunity, and We realized we had the core of [00:49:00] much of the expertise needed to tackle this exciting endeavor, but we needed to combine forces with other teams, in particular the team of Camille Louverville and colleagues at the University of Minnesota who had great expertise in MR, Magnetic Resonance Imaging, physics and hardware development, which was not a strength at Wash U, but, but, It fit very well with what the Minnesota team had their expertise in. And we also teamed up with Steve Smith and others at Oxford University, who had great strength in analysis of both functional MRI and diffusion imaging, uh, that I touched on earlier in our conversation. So we put together, for us, it was a very exciting project, but in the competition amongst you. many proposals submitted to NIH, uh, ours was judged to be a [00:50:00] winner, but there was also a parallel investment in a MGH consortium that was dedicated toward developing a new cutting edge diffusion imaging scanner.
So, in the end, there were two parallel Connectome projects, but for our WashU Minnesota Oxford consortium, one of the major targets of our efforts was to generate a better map of human cortical parcellation. So I mentioned previously that the cortex is this convoluted sheet of very high surface area and in humans and the question of how that sheet is Organized into distinct areas and maps, I had touched on previously in terms of how we approach this in the macaque monkey. But for the human, uh, one doesn't have the opportunity to use, uh, [00:51:00] uh, tracer methods that was vital for our, uh, macaque studies. And there was also a history that over a period of, uh, since early in the 20th century, anatomists had explored the architecture of human cortex. and had made estimates as to how many distinct cortical areas there are.
So two sets of German neuroanatomists kind of pioneered and framed the issues. Uh, Corbinian Brodmann used cytoarchitecture of human cortex to estimate there were about 50 cortical areas in humans. Uh, whereas his colleague Cecil and Otto Vogt looked using myelin stains and estimated there were about 200 distinct on Milo architecture. And over the ensuing century, there were ongoing debates and analyses, but no clear cut resolution [00:52:00] of what the actual count of cortical areas is in humans. And that was our aspiration for the Human Connectome Project, and I think that's what we're It's fair to say that the biggest success story from this project was the effort spearheaded by Matt Glesser to use multiple modalities, the fMRI, the structural imaging, and Combine those in a very carefully balanced set of analyses to identify each area based on sharp transitions or spatial gradients in not just one, but four major types of information related to function and architecture and connectivity and what we call topography, the maps of sensory surfaces laid out in, individual early sensory areas. And using this approach, Matt et [00:53:00] al., were able to identify 180 distinct cortical areas in each hemisphere and to show that these are strikingly bilaterally symmetric, even though there's a lot of literature talking about human cortical asymmetries. To first order, we would say that there's symmetry is the dominant theme on which there are modest but very interesting left right asymmetries.
But the 180 areas are matched pairs in each hemisphere. And they can be identified based on these transitions in a, what we call a group average, uh, or typical brain representation. But remarkably, Matt was able to use what we call an aerial classifier to identify. Nearly all of these 180 areas in nearly all subjects and giving us a tool for approaching the functional [00:54:00] organization at the level of individual subjects. This sets the stage for another part of your question is how is it that we should be exploring the relationship between brain structure function and circuitry in accounting for the tremendous differences between each of us as individuals. We have our own intellectual strengths, our cognitive and emotion related characteristics that are tremendously diverse across individuals.
And one of the strategies that I think holds the greatest promise for characterizing these differences and getting a handle on what underlies them is to use this new cortical parcellation that I've talked about that is really the closest to what Essentially the ground truth mapping of cortical functional organization and to [00:55:00] relate at an area by area level, uh, the relationship between different Brain, aspects of brain circuitry and connectivity to the behavioral characteristics, not just one at a time, but in terms of a spectrum of behavioral phenotypes that can be related to the spectrum of brain features at the level of individual cortical areas.
So this is going to be an interesting domain for exploration in, in the coming. Decade or two, especially, and I think will offer promising opportunities for deciphering functional organization.
Benjamin James Kuper-Smith: At the end of each episode I ask three recurring questions to each of my guests. The first one is, what's a book or paper you think more people should read? You know, it doesn't matter whether this is a new book or paper or an old one. Doesn't matter [00:56:00] whether it's complete, like everyone's read it, but they should read it again or no one's ever heard of it.
I'm just curious for a kind of, you know, some Uh, recommendation about, uh, you know, just a personal favorite maybe of yours that you think like this is a great book or paper, uh, and I think more people should read it.
David Van Essen: Well, I enjoy reading about history, biography of famous people, various aspects of science. And one particular book that absolutely fascinated me was one I picked up off the shelf at a bookstore called Entangled Life by Merlin Sheldrake. And it's a book entirely about fungi and how they are extraordinary aspects of the interface between plant and animal life that are hugely underappreciated and underexplored but involve networks of [00:57:00] interactions not just within the human genome. individual plants, but at the interface between a community of organisms linked by these very sophisticated and exceedingly complex networks of fungi that are richly interacting within our our own bodies, and in the external world in terms of forests and under, underwater domains. And I was absolutely fascinated by this as something that's intellectually intriguing and extremely important in a practical sense of how we're going to cope with the changing environment.
Benjamin James Kuper-Smith: It's, yeah, I've heard of the book, and that's supposed to be good, but I didn't, I never really heard what it was about, um, that much, other than something with, you know, fungi. But yeah, that's, that's another reason to read it now. Um, second [00:58:00] question is, uh, what's something you wish you'd learned sooner? Uh, this can be from your private life, from your work life, whatever you wish, just something that you think, you know, if I'd learned that a bit sooner, my, my life, you know, it might have been a little bit better.
David Van Essen: Ugh. I wish I had learned mathematics better, but as I said, that's, that's something that was an intrinsic limitation, and I don't think my brain was well suited towards Grappling with the tremendous utility of mathematical analysis, but I rely on collaborations to achieve that end.
Benjamin James Kuper-Smith: It seems like collaborations is quite, uh, you know, recurring and important theme of your career. Yeah, I'm just curious. Is there anything about collaboration that, yeah, just anything that sticks out, something you learned that, [00:59:00] uh, maybe how to be a good collaborator, how to find good collaborators. Um, I'm just curious.
It seems like it's, it's, you know, it's something you've mentioned a few times and, um, yeah.
David Van Essen: Yeah, I think it's, to me, one of the biggest joys of science, besides the thrill of discovery, is the opportunity to interact with individuals. Where there's enough common interest and, and shared expertise that one can get on the same page. And yet, the differences in skill sets and, and knowledge and, and expertise that makes the collaboration synergistic and much. Essentially more than the sum of the parts and I think the nature of modern neuroscience is going more and more towards the importance of multidisciplinary attacks on major questions and the need for collaboration that we It [01:00:00] respects the differences in not only knowledge, but, but perspective about thinking on hard problems.
And we don't have, to my knowledge, much in the way of courses on how to collaborate, but I think part of training and part of mentoring in the modern neuroscience world relies on informal, but very important, uh, appreciation of and guidance as to how to collaborate effectively.
Benjamin James Kuper-Smith: Final question is, any advice for PhD students, postdocs, you know, people on that kind of, in that kind of transitory period, anything you'd like to tell us? Because I guess I'm very much part of that group.
David Van Essen: Well, I'd say one thing is follow your passions, uh, you know, there's so much exciting work going on about what really grabs your attention and makes you wake up in the morning and say I [01:01:00] really want to continue working on, on this, that's, that's hugely important, uh, being a successful neuroscientist. I think it's critical not just to have a job, but to have something that you're really fascinated by and willing to devote your efforts towards making significant advances through hard work and reading and, and collaborating to bend the needle in terms of the progress of modern neuroscience.
Benjamin James Kuper-Smith: Those were all my questions, so unless you have anything else to add, uh, thank you very much.
David Van Essen: Okay, thank you.