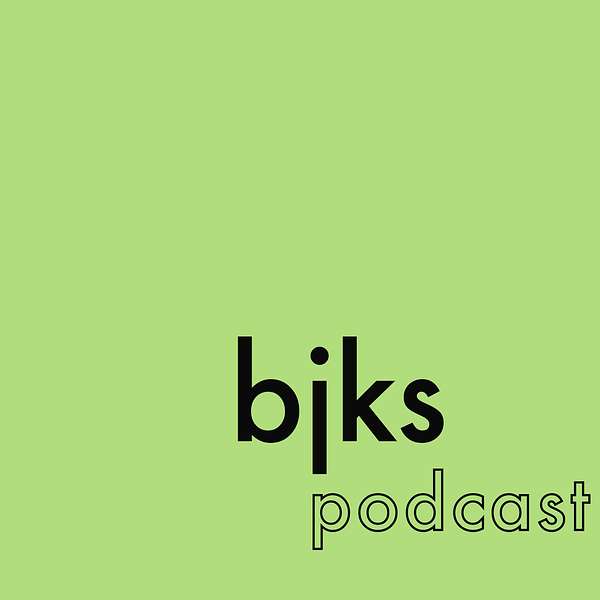
BJKS Podcast
A podcast about neuroscience, psychology, and anything vaguely related. Long-form interviews with people whose work I find interesting.
BJKS Podcast
85. Peter Bandettini: The history, present, and future of fMRI
Peter Bandettini is director of the fMRI core facility at the National Institute of Mental Health. In this episode, we talk about the history, present, and future of fMRI, alongside Peter's career.
Support the show: https://geni.us/bjks-patreon
Timestamps
0:00:00: How Peter got started working on fMRI in the early 1990s
0:05:48: What was possible in neuroimaging in the late 80s
0:18:44: Major advances in fMRI in the 1990s
0:26:39: History of structural MRI
0:29:02: Major advances in fMRI since 2000
0:40:11: The future of fMRI
0:58:19: What is Peter working on?
1:06:31: A book or paper more people should read
1:14:36: Something Peter wishes he'd learnt sooner
1:21:52: Advice for PhD students/postdocs
Podcast links
- Website: https://geni.us/bjks-pod
- Twitter: https://geni.us/bjks-pod-twt
Peter's links
- Website: https://geni.us/bandettini-web
- Google Scholar: https://geni.us/bandettini-scholar
Ben's links
- Website: https://geni.us/bjks-web
- Google Scholar: https://geni.us/bjks-scholar
References
This episode broke the character limit of show notes, couldn't include all references.
Bandettini ... (1992). Time course EPI of human brain function during task activation. Magnetic resonance in medicine.
Belliveau ... (1991). Functional mapping of the human visual cortex by magnetic resonance imaging. Science.
Biswal ... (1995). Functional connectivity in the motor cortex of resting human brain using echo‐planar MRI. Magnetic resonance in medicine.
Blamire ... (1992). Dynamic mapping of the human visual cortex by high-speed magnetic resonance imaging. PNAS.
Engel ... (1994). fMRI of human visual cortex. Nature.
Finn ... (2015). Functional connectome fingerprinting: identifying individuals using patterns of brain connectivity. Nat Neuro.
Gordon ... (2017). Precision functional mapping of individual human brains. Neuron.
Gordon ... (2023). A somato-cognitive action network alternates with effector regions in motor cortex. Nature.
Hasson ... (2004). Intersubject synchronization of cortical activity during natural vision. Science.
Huber ... (2017). High-resolution CBV-fMRI allows mapping of laminar activity and connectivity of cortical input and output in human M1. Neuron.
Huth ... (2012). A continuous semantic space describes the representation of thousands of object and action categories across the human brain. Neuron.
Kamitani ... (2005). Decoding the visual and subjective contents of the human brain. Nat Neuro.
Kwong ... (1992). Dynamic magnetic resonance imaging of human brain activity during primary sensory stimulation. PNAS.
Newbold ... (2020). Plasticity and spontaneous activity pulses in disused human brain circuits. Neuron.
Norman ... (2006). Beyond mind-reading: multi-voxel pattern analysis of fMRI data. TiCS.
Ogawa ... (1990). Brain magnetic resonance imaging with contrast dependent on blood oxygenation. PNAS.
Ogawa ... (2000). An approach to probe some neural systems interaction by functional MRI at neural time scale down to milliseconds. PNAS.
Sereno ... (1995). Borders of multiple visual areas in humans revealed by functional magnetic resonance imaging. Science.
Toi ... (2022). In vivo direct imaging of neuronal activity at high temporospatial resolution. Science.
[This is an automated transcript with many errors]
Benjamin James Kuper-Smith: [00:00:00] Yes, I mean, I guess we'll be discussing the history of fMRI, like I said, for the next hour and a half or so. And I guess, uh, the reason I, you know, wanted to talk to you about it is because your career has been very much intertwined with the history of fMRI. So I'd like to kind of start with And kind of your education and kind of what brought you into fMRI.
So maybe what do you, what did you want to do when you were like 15 or something like that, like kind of when you went into university, what were your kind of interests?
Peter Bandettini: Yeah, uh, well, thanks for having me on, on this. And, um, yeah, when I was, uh, about 15, so that's maybe even a little bit earlier. I, I was, you know, I, uh, my dad, uh, was a high school teacher and, uh, but he also, he, one of the topics he taught was psychology and he always would talk about the brain and things like that.
And, and I was completely fascinated with, with the brain, even in grade school. And I remember actually seeing, uh, I think it was in high school, uh, there was a famous [00:01:00] Scientific American article that came out that showed all the most recent brain, uh, mapping techniques. And I think that there was, uh, xenon approaches and other sort of things that just kind of showed a little bit of what activation was.
And that was super inspiring as well. That was really fascinating. But then I got into college and I. actually also liked physics, and I was thinking of pre med as well, and so I, I majored in physics, and, and I took all the pre med classes, and, or most of them, um, until I decided I didn't want to go to medical school, and then, uh, uh, and then I just, you know, I did some summer internships, uh, doing research in, uh, neuropharmacology, and that was sort of intriguing, but then what happened was, it was a, a lot of serendipity, uh, so I wanted to sort of hedge my bets, and, and sort of Major in physics, but I was still very, very interested in the brain.
I wanted to somehow think of a way, think of a graduate program that could combine physics and neuroscience in some way, but nothing really like that existed. That was about 1988, 89. But I [00:02:00] did find a program. It was just in my hometown of Milwaukee, uh, uh, the Medical College of Wisconsin. I, uh, that was biophysics and as you know, a lot of it was serendipity because there was another person in that program who was an MD PhD student, Eric Wong, who was just absolutely brilliant.
And we were in also a really nice environment that built hardware for MR scanners. Things like RF coils and gradient coils. And so, uh, we are perfectly positioned to sort of get into fMRI at the very beginning. And, and I was constantly, I was in the middle of trying to look for a thesis project. I was just struggling to try to, you know, I was reading the literature, maybe I'd do spectroscopy, maybe I would do, you know, I was trying to do, um, uh, back then, uh, Deni Libby Hahn had a technique called intravoxel incoherent motion, which was a way of having just a little bit of.
diffusion weighting, uh, that it wasn't really diffusion. It was weighted to perfusion, you know, rapidly flowing, [00:03:00] uh, spins or, or just capillary action. Uh, it didn't quite work, but it kind of got me into it. And also, so then what happened was, uh, Eric Wong actually wanted to build a, a local head grating coil.
And that was key because allowed our standard scanner to do what's called echoplanar imaging and no other centers in the world could do echoplanar imaging aside from maybe Um, MGH, uh, Bass General Hospital, uh, and a few others. And that was key to having enough, uh, stability to freeze physiologic motion to make movies, to make time series movies. so, uh, he built the grating coil and then we went to this meeting in San Francisco where in 1991, August of 91, where a person from MGH, Tom Brady, gave a plenary lecture showing the very first preliminary data of fMRI of like saying basically, oh, here's a movie. We don't use any contrast agents. We just put a person in the scanner and shine light in their eyes.
And look, there's visual [00:04:00] cortex lights up. And, and we don't really know why this happens. He literally said that. I'm like, my jaw just dropped. looked at Eric, his jaw was dropped, uh, he was sitting next to me, lots of excitement in the hall, uh, with all the players, uh, early on, like, you know, Bob Turner and a few other people. And I went back and within a month, uh, you know, we programmed up, he programmed up, uh, the echo planar sequence for doing fMRI. I talked to at MGH saying, okay, what were the parameters that you used? We did it. I, I put myself in the scanner, tapped my fingers. And so, uh, it took a while.
I mean, it took a while to get the RF coil, right. And everything, but it worked. And, we were lucky enough to, you know, get one of the first, you know, be one of the first people to, to do this work. And yeah, uh, ever since then I've been. Working in method development in fMRI, it's been, was unbelievably exciting. It was especially because I was, I went from graduate [00:05:00] student, not having a thesis project, just, you know, thrashing about trying to figure out what to do to producing this. You know, once in a generation field, you know, uh, field changing results and, you know, having this wide landscape of, of just developing it and exploring it.
And so was amazing. That was, that was, and that was a lot of serendipity, obviously being in the right place, working with exactly the right people at the right time. And, know, I, I like to think I sort of. Brought that together, but, uh, or not really. I didn't bring, I mean, I sort of, uh, was able to engage Eric and other people to do this, but I, I was lucky.
Um, I was very lucky.
Benjamin James Kuper-Smith: Yeah. Um, maybe to set the scene a little bit in terms of. late 80s, early 90s, what you could do, kind of what were people doing to get ad brain activity, what was some of [00:06:00] the benefits and disadvantages, and maybe why it was, you know, why was fMRI exciting at the time?
Peter Bandettini: So the way people were doing brain imaging then was, was mostly the state of the art was, was pet imaging, positron emission tomography, uh, in which they would inject radio tracers that were either in the blood or, bound of various ligands that, you know, deposit themselves based on brain activity. And, you know, it worked.
The temporal resolution was, you know, extremely low. Sensitivity was pretty good, but, um, but the spatial resolution was extremely low and it was invasive and it was very, um, And one thing nice about fMRI is that. Uh, you know, there were already about, you know, 000 MR scanners in the world, uh, that were, uh, used clinically and you could just jump on those scanners and, and, you know, obviously with some adjustments, uh, uh, start doing it.
So PAT was there, that was a state of the art. There was also, you know, obviously people had, uh, EEG, uh, which, um, you know, obviously had [00:07:00] temporal, high temporal resolution, but low spatial resolution and low spatial certainty. MEG was also out, but all of these were, were very, very expensive because the market was very small for these. Uh, so, so, you know, not many PET scanners in the world. And also you needed a cyclotron for PET scanning typically which was also very expensive. So it was a very big operation. Uh, only a few, a few centers that were very lucky could do that. And so now, uh, what was so exciting about fMRI, I mean, so just before fMRI with endogenous contrast with, uh, blood oxygenation level dependent contrast, and also I was in contact with Sejio Ogawa in the Minnesota group.
He was at Bell Labs. We collaborated with Minnesota. Uh, he sort of pioneered this idea that you could use blood, that it changed susceptibility, magnetic susceptibility when it was oxygenated or not. And so that was an endogenous contrast. But it's interesting. And there. In the literature about 10 years before people said, Oh, you blood is sure it changes, but you can't really see much well, you know, [00:08:00] physiology, nobody knew that oxidation actually changed in that direction actually went up, uh, with activation.
Nobody that the field wasn't fully aware of that. Peter Fox had a pet study that sort of suggested that, but anyway, I'm, I'm digressing before and Dodge's contrasts. Was technique that came out, and it was this iconic cover of science magazine of November of 1991, uh, where Jack Beliveau also at MGH used a contrast agent, wasn't radioactive, it was just a susceptibility contrast agent, to map blood volume changes, uh, with activation and that, that got a lot of people excited, but literally what I like to say is before, you know, the ink of that science article dried, uh, Kwong came out with these results. uh, and everyone sort of that as a technique for brain mapping. It was, it's a really, it's a used, using, uh, gadolinium as a contrast agent is very common clinically for mapping perfusion and, [00:09:00] and blood volume, but, and also other things, but not for brain activation. So, so Ken Kwong came out with this and yeah, the field was extremely excited.
And there was, you know, as you can expect, there was a lot of buzz about, well, is it real? You know, what are we looking at? And it became pretty clear that we were looking at something highly correlated with brain activity.
Benjamin James Kuper-Smith: Yeah, it's funny, it never occurred to me that, I mean, it's kind of obvious once you say it, but it didn't occur to me that a big advantage was that the scanners already existed in, you know, in a form that needed to be modified, but Still, some of it just hadn't occurred to me. I mean, obviously, they had their purposes, it's not like they were just standing around waiting to be used, but still, yeah, that's, I mean, that's pretty lucky coincidence that these scanners that are everywhere can actually be used for something you want to use.
Peter Bandettini: Yeah. And so we just jumped right on the back of the whole clinical industry of all these scanners and they were, they were cheaper. And, and usually most hospitals had, [00:10:00] I mean, obviously you could just suddenly a lot of neuroscientists were knocking on the doors of the radiology departments and, uh, um, But it was hard because those scanners back then, if you tried to do echoplator imaging at that resolution, you would, you'd break the scanner.
It would, it would blow up the amplifiers. And so you had needed, that's why we needed this small grading coil had very low inductance. So it could be switched very rapidly. Um, and since then, since the scanners have. You know, I would say about 96, all the scanners became equipped with echo planar imaging. Uh, they beefed up their great amplifiers and made their gradients a little bit higher performance. But, but what's interesting is that even to this day, you know, it's a, it's a sort of a love hate relationship with the, with the vendors, because we all. And, and they produce these sequences that we use, but still no clinical market for fMRI.
So the vendors don't really care about fMRI. And so there could be a lot, [00:11:00] you know, I'm just, that's one thing I, you know, maybe I'll get to, uh, later on, but you know, if we just have one killer fMRI, the vendors would take. Notice, and they would start putting more man hours into developing things for fMRI. and there's so many more things that could be done with that. Right now, we're just doing what they can on their stock sequences and then writing. Some people are writing their pulse sequences on the side and trying to do what they can, but there's so much more to be done.
Benjamin James Kuper-Smith: One thing I find kind of interesting is that It sounds to me that you all realized that in, like, when this was developing, how important this was going to be. And I'm a little bit surprised by that because I have your, what is it, 1992 paper in front of me. Time course EPI of human brain functioning during task activation.
And those are some blurry images.
Peter Bandettini: Yeah,
Benjamin James Kuper-Smith: those images to me, it's not like evident that this is going to be one of the driving forces in neuroimaging or like, did you know that the resolution was just something that was gonna like catch [00:12:00] up at some point or how,
Peter Bandettini: yeah. So those images, um, I mean, echo plater imaging was really a challenging technique and, and, and on top of that, we could only collect one slice at the time. And that was just because. was a programming thing, and it was just hard to get more than one slice, and so, but we knew that we were going to do that, and we knew that it was just an engineering challenge to make better images than high resolution, and, and also, but the thing is, those images, I mean, just the fact that they were, they already, like, at the first shot, I mean, at the first try, like, those images, And they were particularly bad because I picked in that particular paper, I picked like a super thick slice, like I didn't quite know where the motor cortex was.
So I picked an axial slice. That was about, think, a centimeter or two thick, uh, just to make sure. Cause the problem was, is that we couldn't see the images come up on the screen right away. We had to like, get the scanner prepared, put it in the grading coil. Scan, download the data, walk a quarter mile to our center, [00:13:00] upload, reconstruct.
And so we want to make sure we got it right. Um, so yeah, but those images were still even then comparable to PET scanning. And, and so it was, it was already good. And the fact that it was non invasive was just like magic. Um, it was, it was, yeah.
Benjamin James Kuper-Smith: yeah, I haven't,
yeah, I haven't read many neuroimaging papers from the like 80s and early 90s. So I guess maybe I'm As someone who grew up, you know, studying, you know, psychology and neuroscience in the 2010s, uh, maybe I was, I was not quite aware of how, how blurry things were 20 years before that.
Peter Bandettini: Yeah. And also just to, just to be fair, the Minnesota group actually came out, they had a slightly different technique, which involved multiple Like echoplaners, like with one RF pulse, you get a whole plane. That's called, that's why it's called echoplaner. The Minnesota group had a sort of a multi shot technique that was less stable, but because they were scanning at four Tesla, a little bit higher field [00:14:00] strength gives you a little bit more signal to noise for susceptibility as well. had high, they had high resolution images. If you look at their first paper, so our paper came out and then a week later, their paper came out in PNAS, um, along with the MGH group's paper and, uh, their paper had the highest resolution,
Benjamin James Kuper-Smith: Okay, okay.
Peter Bandettini: yeah,
Benjamin James Kuper-Smith: Yeah, by the way, I'll link all the papers we discuss in the description. Um, yeah. Okay. So, so that happened. Um, maybe, uh, in your, in terms of your career, I'm just, uh, was it obvious very quickly that, that you were onto something and people should definitely give you some good faculty jobs or what were you doing some really weird stuff that no one really appreciated or in between
Peter Bandettini: yeah, I don't even know, you know, it's funny because I'd never even, it's, it's a different environment in graduate school now. I, I actually was, just, wanted to get my PhD. I wasn't even thinking beyond that, but I didn't even necessarily, I didn't feel, I mean, [00:15:00] I, I stayed on, it took me about five years, um, maybe three years after that to, to finish up.
And I, I could have finished earlier and, but I really wanted to make my thesis right. And so it ended up being almost 400 pages and it
Benjamin James Kuper-Smith: that's like,
Peter Bandettini: you know, um,
Benjamin James Kuper-Smith: Yeah,
Peter Bandettini: um, you know, but I think actually what I felt was that it wasn't like, Oh, you know, cause I think it was almost preliminary. People weren't getting, giving faculty jobs for fMRI because had scanners really.
And, uh, you know, they didn't access yet. There, you know, it wasn't, it wasn't a common thing to modify the scanners yet. So I wasn't even thinking about that, to be honest. So towards the end of my. My graduate career, uh, Bruce Rosen at MGH and Jack Beliveau, you know, I met up with them at a meeting and they said, Hey, do you want to, want to postdoc?
And, um, I think I looked at with Gary Glover and Peter Fox. Uh, there are only a few groups. Um, Gary Glover was at Stanford. Peter Fox is at Texas at San Antonio. And they both had scanners, but I, I picked [00:16:00] MGH just because, know, that's sort of MGH has, has been and still is sort of like the epicenter of functional MRI and, and it was uh, stimulating experience going there.
Uh, I was only there for a couple of years, but it felt like 10 in a, in a good way.
Benjamin James Kuper-Smith: yeah.
Peter Bandettini: uh, it was so intense. There's so many brilliant people there and it was such a, such a great environment. I mean, where I came from as well was, was amazingly nurturing and perfect for getting going, but MGH was great for kind of expanding it.
It was like perfect postdoc as far as just stuff and expanding out. And then after that, what was interesting is that before I left. Uh, M-C-W-I-I was very kind of naive, not I, I was naive. Um, I guess I still am naive to some degree, uh, which is a good thing sometimes. But, um, MCW had written a program, project grant, and so they, they got this big grant, one of the first, uh, from NIMH [00:17:00] to, to do functional MRI method development.
And so, but one of the CRI criteria, I think as, as it's the story's told, is that, uh, that I had to come back for them to. Uh, you know, uh, that was one of the agreements, I guess, and for the grant, I don't really think was the case, but that's what they told me. And I believed it. Um, so I felt obligated to go back.
Otherwise, I probably would have stayed at MGH and I would probably would have still been there today, but I went back and, uh, did the best I could, uh, as a faculty, a new faculty at MCW. And, uh, And then, uh, during one of the program project grant site visits, Leslie Unglater from NIH, uh, was one of the site visit people.
She took me aside in the hallway during the site visit for MCW and said, Hey, we got a opportunity for you at the NIH. And I said, okay, I'm going. Um, and that was awesome as well, because I mean, so much, there's so much serendipity with at [00:18:00] least my career and probably many people's career early on in fMRI.
Um, so she offered me this position and, uh, you know, running the core facility at the NIH, growing it, and then having my own group. Uh, it's nice being at the NIH. You don't have to apply for grants. You get sort of a budget and, are free to do, um, focus on the research and, uh, and it was great. So right now we have. At the NIH, we have two, seven Tesla scanners, uh, three, three Tesla scanners, about 30 PIs use the facilities. I have my group. I just, I just got a machine learning group, uh, and a data sharing group as well that I sort of started. And so, yeah, things are going well, um, uh, they're still thriving.
Benjamin James Kuper-Smith: Yeah, um. So going back to the history of MRI then, kind of what were, I guess kind of what I want to do like in the next hour or so, is kind of go through some of the like, kind of the big changes that happened in MRI and kind of how this changed, what you could do with it, how people thought about it and that kind of stuff.
So early [00:19:00] 90s or 92 let's say, we know it works. It can, or some people would believe it works and it can do some cool stuff. What was kind of the next big shift in this?
Peter Bandettini: You know, it's funny. Uh, you know, there, there were a lot of conceptual advances. And, uh, so the first one. Was, uh, as far as I remember it. So just to, just to couch all of this is that, you know, most of FBRI as it's done today is still pretty much more or less the same. We use bold contrast for almost everything. We use echo planar imaging, it's just higher resolution, more sophisticated, but, but there's a lot of, uh, really, there are a lot of smart people in the field developing things. And one of the first conceptual advances was the ability to do, everyone, like when they first did fMRI, they did what's called block design studies, where they, 20 seconds on, 20 seconds off, because everyone was thinking. Still in terms of PET scanning, and then for the very first time, people said, you know, let's do event related. So in 92, actually, one of the very first papers, uh, by [00:20:00] Andrew Blanmayer at Yale, he did event related. He didn't call it anything. So he didn't, people forget that he, uh, did event related fMRI. He, it was like a two, two second or three second, you know, a brief event and you could see it really nice
Benjamin James Kuper-Smith: Yeah.
Peter Bandettini: Um. In 96, Randy Buckner did the first cognitive study and also, uh, the people at Yale as well, uh, with that related fMRI. So that was sort of a, shift. and around that time, and just after, you know, the whole idea that you can do. Deconvolution. You can actually do events like really rapidly, even though fMRI was slow, like the hemodynamic response was slow, that you could deconvolve, uh, that response and, you know, to go very rapidly and still get the response.
So that was, uh, that was probably the first, uh, conceptual advance. In about 94, another conceptual advance was more in the area of post processing or paradigm design. And that was Steve Engel and, uh, and also Marty Serino. They made these amazing maps of [00:21:00] retinotopy where they just continuously varied the stimulus and then mapped the phase of how it felt.
It's changed to, to do these, make these stunning maps of, of visual cortex, uh, organization. And I like to think that is a conceptual advance because, you know, it's sort of like people realizing the potential, the flexibility that they had in the time series. But the big, so to me, the biggest conceptual advance, uh, even bigger than everything else that's followed occurred in 95 or 94 was when it started. Barat Biswal, also at the Medical College of Wisconsin. was playing around with the data and, you know, looking at the noise and just trying to just characterize the noise for his thesis. But then he, he just took a region of interest in one motor, you know, while the subject was lying in there doing nothing, he had them doing nothing because he was just studying the noise.
He just, he wanted to characterize the noise. And he just took one voxel from one motor cortex and said, okay, how does this noise correlate with the rest of the brain? And he saw. Oh, wait a second, the other motor cortex is showing the highest [00:22:00] correlation, uh, with this one motor cortex region of interest, uh, just in the noise.
This is crazy. when resting state connectivity, or rest, they didn't call it connectivity, but they, resting state correlation started. I love the stories where it's sort of, there was no, like, I mean, I think that the field is truly ratcheted forward with hypothesis driven studies, but I think that people underestimate how important it is to sort of try things and look carefully at your data. And that's an example of discovery. He was doing something else altogether. He was looking at the data and he's like, Oh, wait a second. This is something significant. so that was, that was huge.
Benjamin James Kuper-Smith: Okay, I didn't realize that that was that early. I mean, I've, I've never really, I mean, maybe for context, I haven't done any FMRI yet. I'll probably do it during my postdoc. Um, I've, you know, taken some like. Stuff about neuroimaging, but I've never done it. I didn't, and for somehow, I don't know why, but the resting state stuff never seemed all that interesting to me.[00:23:00]
Um, I don't know whether it's because I, because I come from like a cognitive perspective or something, I don't know why. But I also didn't realize that it was that early. I thought it was, was like five years later or something like that. Um, so maybe, maybe to, to, to get me out of this slight neglect of resting state stuff, what's, what's so interesting about it?
Peter Bandettini: Well, well, just to, just to mention, um, your, your perception of resting state is, is correct because it actually took an entire decade, before anyone else, almost anyone else started looking at it again. Only like maybe three groups looked at it and no one cared,
Benjamin James Kuper-Smith: Okay.
Peter Bandettini: uh, because they're all so busy. I mean, we were all, the whole field was like, Oh my gosh, you know, let's just do the, all these tasks.
Let's try everything. You know, let's just, you know, they want to do tasks. They would, it would seems like a waste of time at that time to like, just put a person in a scanner doing nothing. But what's interesting about resting state. Well, to me, there's two things. One's practical and one's something that we're still trying to figure out is. the fact that when [00:24:00] the brain is in sort of a quiescent state, it's never really quiescent, and that the areas that are functionally related constantly of, I wouldn't say talking to each other, but sort of, you know, exchanging some sort of information or being somehow driven in a way that's spontaneously. So you have this spontaneous, you can imagine neural firing, uh, that's occurring that Is suggestive of the entire functional organization of the brain. And so that's in itself kind of a mystery. First of all, why it does that, what's driving it? What's the purpose? And secondly, it's exciting practically because everyone realized and it took about another 15 years before people started doing it.
Well, is mapping the entire functional organization, at least. From areas that are correlated with each other, you know, mapping out the entire. [00:25:00] Connectivity of the brain without any tasks at all showing and it looks a lot like, you know, Broadman's map, you know, you have and so that's what people do now is they the state of the art techniques for parcellating the brain.
So people with the way they analyze the data. Now, a lot of ways is is they first put a person scanner or and and there's many different ways. But one way is putting person scanner doing lots of resting state. Parcellating the brain based on the resting state connectivity and then taking each of those parcels as a region of interest and putting that in a connectivity matrix, then to do analysis and, uh, and what people found is that, you know, people's resting state connectivity varies depending on whether they have disorders, uh, and other sort of things that were still in that exploratory stage of, of understanding the significance of resting state and it. And the practical use of it, but, yeah, I mean, it's still a mystery. And actually, what, you know, one of my favorite papers, uh, it was recently where Marito Corbetta, you know, wrote this article about what, what the resting [00:26:00] state could be. And it was a very thoughtful, uh, interesting piece about how it could be, you know, the network sort of testing priors, uh, or priming itself, you know, based on what it's done during the day or what's done the past week, you know, just sort of, you know, so we don't know. Um, but anyway, it's a really, uh, People are still probing to find a really and it would be and I think actually as far as clinical applications are concerned being able to put a person in the scanner and not forcing them to do anything might you to do patients that can't do tasks and things like that. So it's it was exciting for a number of reasons Um,
Benjamin James Kuper-Smith: Okay. Um, brief question. We've only, you know, talked about fMRI, just a brief question about structural MRI as that, how did that develop in parallel? Was that much earlier? Or what's kind of the, um, relationship there?
Peter Bandettini: So structural MRI, you know, conceptually was kind of came about in the 70s. And, uh, the first practical, the first [00:27:00] clinical scanners were sold in like the early 80s, very early 80s. so, uh, yeah, when I came along, whenever I came along and structural MRI was around for maybe a decade, if that. And so since then, it's, it's right.
I mean, it certainly has been, you know, Developing, uh, in terms of, you know, once again with the, with the clinical scanners and with the vendors with basically they want to get higher fidelity images that are, know, there's many things you can do to improve an image quality and, you know, to have enhanced contrast, high fidelity, high resolution, but all, uh, in, in as short a time as possible. They've been optimizing things continuously for the last 30 years. So the structural, structural scanning is, is just amazing as far as, uh, what they can, what they can do. And, there's other types of structural imaging. There's diffusion imaging, which is used clinically to look at, you know, [00:28:00] Inflammation and things like that, but also for functional MRI.
It's used for natural functional for neuroscientists. They like to make fractional anisotropy maps, which or or even using those maps to create, um, do track tracing like Trace all the white matter tracks all the connectivity in the brain and that's been developing ever since as well Uh that that sort of came about with Denise Libby Hahn and Peter Basser uh in the late 90s and uh, and that was a A big advance in terms of what we can do with fMRI or with MRI. Um,
Benjamin James Kuper-Smith: Yeah. Um, so a
Peter Bandettini: also just to mention quickly, there's some, there's places, there's also baseline perfusion imaging using arterial spin labeling people, Ken Kwong, even in his first paper had mentioned that can look at. independent of bold contrast, and um, uh, there's ways of non invasively looking at blood volume contrast too.
So there's a couple of other
Benjamin James Kuper-Smith: lot of things you can do [00:29:00] with With one machine. Uh, a brief question about kind of, you know, when we, when we talk about some of like the major advances in fMRI and that kind of stuff. For example, you haven't mentioned any of the things I almost thought you were going to mention, something like, I don't know.
Combining it with computational modeling or decoding or, uh, any of those kind of more advanced analyses or kind of, uh, yeah, how, how do you think about those or kind of fMRI adaptation or that kind of stuff? Um, I, I thought this was what you were going to say. Um,
Peter Bandettini: Yeah, well, well all those came along. I just started with
Benjamin James Kuper-Smith: okay. It's just a bit later. Okay. Yeah. Okay.
Peter Bandettini: without a doubt, um, another conceptual advance was, right, as you mentioned, uh, multivoxel pattern analysis. First, sort of shown by Jim Haxby, sort of taken forward by Nico Krageskorde. I was lucky enough to have him as a postdoc in my group as he was doing it, but he was kind of working as a PI, even though he was a postdoc in my
Benjamin James Kuper-Smith: Okay.
Peter Bandettini: very driven.
Benjamin James Kuper-Smith: So,
Peter Bandettini: exactly what he [00:30:00] was doing. as you mentioned, the paradigm design also, right, one of the things that I, is fMRI adaptation, which is a very powerful technique for pulling out, uh, pools of neurons within a voxel that might show adaptation to a, a task. Uh, there's other things too. The whole idea of using naturalistic stimuli. So it's somewhere between resting stage and task, but you just show like people movies and people would always figure out, try to wonder, how do you process this data? there were two, I think, breakthroughs in terms of how to process it. And one is Yuri Hassan in about 2004 came up with like a, uh, uh, intersubject correlation, like seeing what the correlation was looking, watching the same exact movie then seeing how the subjects correlated with each other.
So that. Pulled out that you wouldn't be able to just model in the time series from the movies. Uh, or so we thought until Jack Galant and Alex Huth came along and they did the same sort of paradigm. [00:31:00] They didn't do intersubject correlation because they felt you couldn't really interpret that that deeply.
And so what they did is they. broke down the naturalistic stimuli into all these regressors that represented features of that stimulus. And then they did voxel wise modeling of all those regressors to weight each voxel as to what it was sensitive to. I thought that was one of the most stunning papers I've seen. One of my favorite papers is Alex Huth paper in which he maps the semantic space of subjects listening to, um, the moth podcast and and also, um, or the moth radio and or or watching videos. And basically, I still think that the field is catching up to this because it's sort of turning. Brain mapping out its head in a sense that say that there are these semantic features like everywhere in the brain and it's, it's really interesting.
So I think that's brand new. So, and of course, in 2005 about Comitani, uh, developed decoding, which is sort of, and, and John Dylan [00:32:00] Haynes as well. Um, that was Sort of related to the fMRI multi voxel pattern analysis, but it didn't have to be. Um, but it would, it, it sort of used the multi voxel pattern for which is really cool and, and very, very useful.
And, and sort of gave an indication that, hey, there's, it's not just blobs of activation that we're looking at. It's these little multi, just voxel wise patterns that looks like salt and pepper, that's actually contains information. And I actually credit Jim Haxby for, for really that realization that, you know, once again, it was a serendipitous thing, I think, where he was trying to look at faces, the face area, and it was a big blob, but then he looked at houses, and that wasn't a blob, and then he looked at other objects, they weren't blobs, but then he's like, okay, let's just see how these maps correlate, you know, with themselves versus, you know, split, splitting the data up versus others, and he found, wait a second, there's a higher correlation with the same Object and there's patterns then so so that was huge, uh, [00:33:00] understanding that there's patterns in the data.
It's not just blobs of activation. So that was a big thing. Uh, dynamic resting state. So now Katie chain came along in about 2010 showing for the first time that not only is resting state interesting, but it changes the correlations change over time. so that was sort of a advance that we've been. picking up on ever since. And, and let me just end, let me just, uh, say, mention the last couple ones that are just, that are going on now. Um, the ones that are, that are actually going on right now that I think are, are, well, that are really interesting in terms of processing, at least, are the whole concept of deep sampling. of fMRI data. And it's a little bit more nuanced, but the idea is that, you know, about 2000, though, the idea of having big pooled data sets looks like, okay, so we can't pull out that much of our data with one subject. We can't even do it with 100 subjects. Let's just, [00:34:00] let's get tens of thousands of subjects or, you know, maybe not that many, but the biobank, the UK biobank has that, but, um, and, and see what we can, we see what we can do.
And, and people are working on that and deriving interest, interesting information. But it's limited and, and it, and so the group, I like to think that we, we did this once, but this other, this group at Wash U has sort of pioneered this idea that, okay, let's take one subject and let's say, just take a set of 10 subjects.
They had something called the midnight scan club where they scanned all the volunteers for like, you know, 10 hours. And do you get anything more? It's truly stunning how different people are in terms of, you know, the, the nuances of their anatomy and their functional organization. To me, after seeing their data, I realized it's a big, it's a huge problem trying to pool subjects together, uh, spatially, at least to average them to make inferences about other than just some sort of data. You know, small effect [00:35:00] size of the mean that comes out. But if you look at individual subjects deeply, uh, I think right now we're sort of like in that stage of like, okay, this is interesting. Um, on top of that, so a post, uh, staff scientist of mine, Javier Gonzalez Castillo, scanned a subject for 10 hours and realized even if you lighten up your models, if you don't say, okay, the hemodynamic response has to look like this, and it was a very simple task. We found that After averaging for 10 hours, we found that literally the, with a very simple task of visual stimulation and pressing a button when you see, uh, something within that visual stimulation, that literally the whole brain showed time locked activity that wouldn't have been caught if you just use a simple canonical model of the hemodynamic response, that, that there's something going on everywhere in some way with everything. So that's, I think deep imaging is a big thing right now.
Benjamin James Kuper-Smith: so what does that tell you if, if all of the brain is to some extent involved in the simple task?
Peter Bandettini: Yeah, [00:36:00] uh, well, that, well, right. When I tell statisticians this, they don't like it because there's no, no, suddenly there's no, no model, you know, it's like, you can't, um, you can't assume that there's, you know, uh, if the whole brain is active, it's the question is how do you, how do you, is that other stuff that you pick up? important is that? And, and certainly I think it's probably a lot of stuff that's just transient where the brain is sort of like shifting, um, know, perspective or shifting attention or, or something, uh, so a lot of it, you know, a lot of it was subcortical where you would see like a transient response or something like that, but you also see a lot of decreases in activation that are time locked that, that you might not, uh, see otherwise that are very subtle, but that everything kind of other things go down.
So I wouldn't say activation, but the brain does the whole brain does something, uh, with, with these responses. So we're still in the, in the stages of. Trying to work exactly that question out. It's sort of like we made this observation and we're trying to, you know, [00:37:00] make sense of it. uh, so yeah, so anyway, so there's a lot of work that. That needs to be done as far as deep imaging is concerned, and, and you need to scan, you know, at least five hours, uh, a single subject, and it's truly amazing the amount of new information you can get out of scanning one subject, and also it's really nice because the subject, you can take, take them out of the scanner, put them back in, but the registration problem is, you know. Not a problem because everything is is the same. Two recent things. Uh, the concept of brain fingerprinting and it's sort of along the lines of, uh, the shift in fMRI right now where you're trying to look as opposed to drawing inferences about groups, you're trying to draw inferences about a single individual. And, uh, Emily Finn pioneered this, and, she wrote a paper in Nature, I think it was Nature or Science. Either way, uh, in 2015, talking about brain fingerprinting. And, and now people are [00:38:00] doing, uh, you know, either using resting state or watching a movie or whatever, trying to pull out subtle differences in connectivity between individuals, as opposed to trying to draw, like, inferences from groups.
And so that's, I think that's a, A large conceptual advance. The latest one is, uh, super high resolution, high field MRI allows you to image at cortical layer resolution, that will, that's not only a benefit, you know, where you get to higher resolution, but also you can then start to make connections with all the other neuroscience work in electrophysiology or whatever, trying to look at input or feed forward and feedback connections based on where they're located in the cortical, uh, in, across layers. So if you have like, you know, And we're still trying to work this out where if you have models where, you know, upper and lower layers are active, you can infer that it's sort of a feedback sort of connection and where [00:39:00] if it's a layer four in certain areas, you can infer that they received input from somewhere lower in a hierarchy.
It's like input, so there's lots of fun to be had with that requires not, uh, bold works, but, uh, it turns out that blood volume imaging using a technique called vaso is more specific. to the localization. So you can get high resolution, but you, but it's hard to get the functional resolution unless you get rid of all the vein effects and things like that. So the draining vein effects that are downstream. Um, but so that, so that's also a recent, uh, sort of conceptual advance and, uh, Renzo Huber has been developing that. And there was just a paper out last week, I think, in Science with David Feinberg in Berkeley, who combined the use of now we're going back to the day one where we use the head grating coil, combining the use of a grating coil along with their high power gradient amplifiers and 7 Tesla to get at 0.
[00:40:00] 3 millimeter resolution in fMRI, and so that will open up a lot of new questions that you could ask with fMRI.
Benjamin James Kuper-Smith: Yeah, I wanted to ask a little bit about what we can gain from just increasing the size of the magnet, because I guess since, you know, since the 90s, uh, there has been generally a bit of an increase in the, in the scanner. Uh, sizes, and I, I did my, I mean, I did a master with two masters projects, but one was in at Neurospin and outside of Paris where they had this something like 15 Tesla whole body scanner, something ridiculous.
They got, it's just like the size of the entire building, right? I'm curious, kind of what you think about, you know, I mean, yeah, we have seven Tesla and some do like 9. 4, whatever the biggest size after that is. Are we going to gain anything conceptually new by that? I mean, you already alluded to laminar activity.
Or is it just more precision of the same stuff?
Peter Bandettini: Yeah, uh, well that's a really good question. I, yeah, the, the Neurospin, right, [00:41:00] Leviathan was, uh, leading the way. I, I think it was, yeah, 11. 7 Tesla human scanner. Um, and they're just got it up and running, I think, uh, recently. So it's now
Benjamin James Kuper-Smith: Okay.
Peter Bandettini: You know, there was, there was a lot of doubt, uh, in the field.
They're like, Oh my gosh, you're not even using a standard vendor. It's, I think they were using the same company that made their cyclotrons. So, so we're worried about that. Uh, but we're happy that they got it working. Um, you're right. At about, um, 2000 or so the year 2000 that everyone was most ever that's when three Tesla started becoming standard because it was a clinical, uh, also they're told to clinically. 2005 or so, 7 Tesla caught on. Now there's about 150, uh, maybe a little bit less than that, 7 Tesla scanners. And everyone wants higher field strength. I mean, certainly it allows you, uh, it gives you higher sensitivity. And there's always trade offs with everything. I mean, it's harder to make good images.
There's signal dropout and things, but people are solving those problems. But, um, higher sensitivity. What does that buy you? That [00:42:00] buys you resolution. else it buys you, uh, you're able to the same resolution and go a little bit faster because you don't have to average as much, um, that could open up certain types of questions.
And it gives you the ability to see more subtle variations in the time course. Whereas before, if you were like, What's called thermal noise dominated. It's just white noise or Gaussian noise. Whereas if you're physiologic noise dominated, you can filter out the physiologic noise some degree. Then you can start to get at these, you know, subtle time course variations that, that might be related to transient neural activity that we haven't seen before.
And that could contain interesting information. But as. you mentioned, as I was talking about, I think that the big thing now, and you're right, going to just high resolution is exciting. You might be able to draw, you know, more detailed maps, especially at the subcortical And that's one of the areas that hasn't been explored that much with fMRI [00:43:00] is the subcortical structures and how they communicate with the cortex.
It turns that, you know, maybe most of our, you know, I'm in National Institute of Mental Health. most of our. originate in subcortical cortical disruptions, potentially. And so to be able to do fMRI on those areas, you need high field to, to really have enough signal to noise to resolve those structures and then to resolve their activation.
But certainly layer fMRI, as I mentioned, is, is, and, and column fMRI, which is important. And you know, the brain, ultimately that might be. Columns and layers, even though there's many, many millions of neurons within each voxel, even at that resolution, unit of, the most relevant, you know, I'll stick my neck out here, you know, potentially the most relevant unit of functional organization of the brain might be the columns and layers.
I mean, it might be at that scale, [00:44:00] that meso scale. Individual neurons are certainly interesting, but they all, you know, they're similar neurons that do similar things at certain, at layers or columns. So if we get there, we could start to do much more sophisticated modeling. You know, the whole goal is to sort of feed this fMRI data into computational models to try to figure out how, you know, instead of just mapping what's going on, to actually figure out how the brain is computing and the mechanisms of, of mechanisms rather than just mapping.
Benjamin James Kuper-Smith: Yeah, I mean, I guess part of the, to, to use the metaphor of the map. I mean, it's, it's good to have a detailed map, often only to a certain degree, right? Depending on what your purpose is. But also you need to have a map of the right thing. Like, you know, having a highly detailed map of something that doesn't actually tell you anything useful.
Yeah. Isn't going to help you.
Peter Bandettini: Right. I mean, I think, yeah, we're all, you know,
Benjamin James Kuper-Smith: So I guess my question is kind of like whether you think we're drawing the correct, the useful, useful maps in that sense. If for example, there hasn't been any [00:45:00] clinical application yet, uh, or any, yeah,
Peter Bandettini: Well, I mean, that's, uh, right. I mean, there's many reasons why there's not a good clinical application yet. But, but I agree. Uh, and this is, is sort of what I'd lament a little bit. I mean, all of fMRI is kind of, you know, just cartography, uh, some degree. I mean, certainly you're looking at dynamic changes, and you're looking at how things are modulated with activation, which can give you some inference about how the brain is computing to some degree. Yeah, so going to higher and higher resolution, and you might in the one of the holy grails of fMRI is to sort of come up with biomarkers. You know, if you had a map, let's say you had a map. I mean, you know, the initial hope was that Oh, if you had a subject with schizophrenia, you put them in the scanner and give them a set of tasks or resting stage and the pattern would look different. even though we don't know exactly what's going on with the pattern, it's something that would help our [00:46:00] diagnosis or help our diagnosis treatment. And that was the hope. And it turns out that there's just, people are too similar in many ways and too different, uh, even among normals.
Benjamin James Kuper-Smith: yeah.
Peter Bandettini: so there's too much variation.
It's not a question of sensitivity of fMRI. It's more a question of how similar and different normal populations and those with schizophrenia are and how it manifests. And unfortunately, hasn't manifested robustly enough our maps to
Benjamin James Kuper-Smith: like general problems with, you know, psychiatry in itself. You know, obviously if you have these categories that have so much overlap, then that makes a lot things a lot harder. And I don't think, you know, we need to use clinical applications as the, the, the kind of final.
Score of whether fMRI works or not, but yeah, I just kind of want to use it a little bit as a kind of to see like, you know, is the field going the right direction or is it just more, more resolution of the, [00:47:00] how far is that going to take us? Maybe, maybe that's the question more.
Peter Bandettini: Yeah, that's a really good question. Um, I don't know how far it will take. I mean, I think that, uh, going to higher field and getting and, you know, because there's so few experiments so far, uh, that are done well at that resolution. And what we've always found is that the higher resolution you go, you do get more insights as to, for instance, there was a paper, uh, by Renzo Huber at, you know, looking at, um, digit representation. you know, with the standard fMRI maps, you, you, you would just map along the homunculus, but go to high resolution, like really high resolution. You could actually see that there's like about three different maps of digit representation in the motor cortex that are kind of mirror images of each other.
That was sort of new. I don't know if it gives us any more insight into what's actually going on mechanistically, but those are all things that sort of open up. So I think the idea of going to high resolution is that it opens up, [00:48:00] opens up new questions and new areas where you could explore. And very specifically, I mean, layer fMRI is unique in the sense that only does it go to high resolution where you can resolve layers, but from that resolution of the layers, you can start, yeah, as I mentioned, you can draw inferences about, uh, not only where areas are connected, but where they are, are in the hierarchy of connection, uh, whether they feed into an area or whether they get feedback from an area.
And so then the exciting prospect is if you think of the entire brain is organized as sort of a inference machine, you know, you make models of the world. And then you compare that model with your sensory input. That's the unit at which it's done, potentially, uh, where at the cortical column. layers where you have your prefrontal cortex giving feedback that feed back into that area and then the sensory areas feeding and then comparing it, uh, so that there's a lot of potentially really, really interesting work to be done with that [00:49:00] one extra piece of knowledge as far as that's concerned.
So, and that's where I think jump could occur beyond just simply delineating things more accurately.
Benjamin James Kuper-Smith: Yeah, that's really interesting to me because, I mean, I don't work on that now, but I did my, I said my master projects, my master two projects, and the first one was, um, at University College London, uh, in Coffrey Stinson's group using kind of predictive coding. So like that stuff is, uh, it's a bit, it's been a while, but it's very familiar to me.
And, but yeah, we, but we didn't do that with FMRI. I mean. I was, you know, I had a certain data set and, you know, that was intracranial EEG. But yeah, it didn't occur to me that that kind of work with fMRI might be possible once you have just higher resolution.
Peter Bandettini: Yeah. There's a long way to go for it to be super robust. I think it'll be another, you know, five or 10 years before like everyone can, or a lot of people can do this and actually really do penetrating [00:50:00] experiments, uh, with it maybe earlier, but, it's, it's coming. And, and I think things are starting to converge, right?
I mean, I think Carl, you know, probably is taking interest in this, uh, as a, as a way of further refining his His constructs of the brain's inference engines and things like that.
Benjamin James Kuper-Smith: Um, one thing that's kind of, you know, going towards, I mean, I guess we're already doing that kind of discussing like what might happen in the future and what, maybe what you would like to happen in the future and that kind of stuff, one kind of overall impression I'm getting that from, from hearing you talk about it, that I didn't get before, uh, we started the conversation is just how many.
basic questions are still there to be answered in fMRI methodology. Um, I guess maybe when you come it from it from the outside, it's like there's all these different methods you can use to analyze the data, all these pre processing steps you have to, you know, abide by, and etc, etc, etc. And it feels like this huge amount of stuff that's, you know, this large corpus of [00:51:00] work and knowledge that we already have, but it's funny hearing some of the stuff you said when it's just like, Yeah, but if you scan someone like 10 hours in a row, I mean, not consecutively, I'm assuming, but, um,
Peter Bandettini: Yeah,
Benjamin James Kuper-Smith: that would be a bit, yeah.
Peter Bandettini: that'd be torture.
Benjamin James Kuper-Smith: some people love it, but, uh, yeah. Um, yeah, just how many basic things are still there to be done. And that's something I didn't quite realize. That's kind of interesting.
Peter Bandettini: Yeah. There's a lot of, I mean, and usually these are internal debates of, you know, I mean, certainly there's been FRI has had its share of Internal debates that have sort of spilled out into the public media, like the, the whole, uh, you know, cluster clustering, you know, how do you cluster this? You know, some paper said that, you know, if you don't cluster, right, then things are off or else, you know, the most famous 1 was the, the, the dead salmon study were basically was saying what everyone kind of knew that, you know, if you have, if you have Too many voxels that are observing, you know, by chance, you'll get [00:52:00] positive results and you have to control for that, you know, basic Bonferroni correction, but there's many, many other things like, like, even, resting state still people don't really know.
I mean, there's a lot of things in resting state there, you know, respiration, ongoing thoughts, other sort of. People are still pulling it apart. A lot of people don't even believe in the utility of dynamic resting state. There's a lot of debates on that. And there's even debates on, you know, the whole problem that has been around for 30 years is it's an indirect measure of neural activity.
And so you're very much dependent upon having that neurovascular coupling mechanism, the same throughout the brain, which it seems like it mostly is. But you still have the problem of like these large vein effects, like, you know, ideally you would want the whole brain to be this mesh of fine capillaries where you have activation and just the area of activation shows the change, whereas in fact, it drains into these veins and it shows up with [00:53:00] bold at least, um, another problem that's debated that's, there's been some nice papers sort of making some headway into this is differentiating excitation versus inhibition, uh, neuronally, you know, people say, Oh, you can't really do that with fMRI, but I think there's signatures in the fMRI signal that might be able to be pulled out to indicate, you know, how much inhibition is going on.
So that's another thing. You know, there's all kinds of unknowns like that. And even, even the question, it's, there's certain things that. That haven't, you know, it's funny, there's certain things that are blind spots that I think that haven't been debated enough and now are becoming standard practice, like this whole concept parcellating the brain of taking a standard parcellation template, putting a whole bunch of brains together, putting them in a parcellation and then say, okay, this is, this is how the average parcellation and I, I sort of cringe when I see that because after seeing some of these deep scanning studies, the brains, each brain doesn't really parcellate the same.
And [00:54:00] sometimes there's. Okay. a huge amount of difference. And so, you're throwing away a lot of data with that. Um, and even something as basic as picking a threshold, people pick thresholds, uh, of activation, but there's so much significant, I would say, you know, just if you average a little bit more significant activation all over the brain. If you pick a threshold, what, what does that mean? How can you interpret that? So all these things are still actively debated by, you know, the 200 or so methodologists who, you know, think about these things,
Benjamin James Kuper-Smith: I mean, like, yeah, it sounds like a good time still, you know, it might as a from, for me from the outset, I mean, I'm not of the engineering type, let's put it that way maybe. So maybe it's more obvious once you're more interested in these things. Um, but yeah, it seems like it's lots of stuff to be done, uh, for people who, who want to do this methods development,
Peter Bandettini: only towards the, the, and there's 2 other sort of [00:55:00] cultural differences. I think there's the statisticians who are great, but who want to make sure you're doing whatever you're doing correctly. But there's also these, the methodologists who are trying to open up possibilities of doing things Uh, and I think it's, there's a tension there. You don't want to have standards. You want to do things correctly, but you want to also be able to maybe look at things a little differently sometimes.
Benjamin James Kuper-Smith: kind of a slightly offset. No, what's it called? There's a saying I can't think of right now, a question that's, uh, maybe slightly, slightly random. Um, so I talked to Gareth Barnes about the new MEG systems, the OPM MEGs that, you know, don't require the cooling and are much smaller, et cetera, et cetera. Is anything like that ever like theoretically possible with fMRI that somehow you can have these?
I don't know, like magnets can be much smaller, like for example that you don't need a huge room, but you can have something you can wear around your head. I don't know, is anything like that theoretically possible? Um, it's obviously always [00:56:00] difficult to say X or Y is never going to happen, because then, you know, wait 10 years and someone's going to show you.
But I'm just curious, like, um, right now fMRI machines are these big, heavy, expensive machines. Is any change of that theoretically possible?
Peter Bandettini: Well, the one thing that I think people in the magnet industry are waiting for, um, and I think it will happen, who knows, is room temperature superconducting. And if you can get room temperature superconducting, if you, you know, you can afford it. And then you don't, you know, most of those scanners, uh, what they are is this big doers of, of liquid helium.
And that creates a cool enough environment so that you can have superconducting, uh,
Benjamin James Kuper-Smith: Right, so it's mainly a fridge with a magnet attached to it.
Peter Bandettini: Right. And so if you have that, you can get. to something smaller, uh, and, and maybe in some very well engineered isolated situations, you might be able to have something wearable, but that's, that's a long time in the future, [00:57:00] and it's a big, a lot of big ifs, but I think that that would be the best hope, uh, room, uh, you know, at least not even room temperature, like something where you don't need it that cool to just to be
Benjamin James Kuper-Smith: What, were you following the recent debates? Uh, I didn't really follow it about the superconductor or whatever it was. Were you following that closely or?
Peter Bandettini: Uh, I, I, I pay attention to it. Uh, it's interesting. Um, it's what's, what's really interesting about the whole field of superconducting. There's, there's an example where it's just sort of like this black art. You know, it's like people, you know, they think they have theories about how superconducting works and it's, they're probably, you know, I don't know enough about it to really say much, but it seems to me that they're just trying stuff. And everyone's just trying stuff, you know, it's, and they, and some people try things that are a little bit, you know, more couched in theory, but it's still just trying stuff and we're still trying to figure out what magic combination of materials will, will allow superconductivity at higher temperatures.
And think there's a, there's [00:58:00] definitely a well developed science, but yeah, I've been following it a little bit. Um, it seems like, yeah, maybe a year ago or so, there were some advances. At least in the theory as well, that might allow us to make more progress. But, um, I don't know how far that went.
Benjamin James Kuper-Smith: Yeah, yeah. Uh, kind of, as we're moving towards the end, uh, kind of what are, what are you working on? Like, what do you want to, like, personally make happen in the next five years or ten years or whatever? I don't know, something that just, you know, you've mentioned lots, we've talked about lots of different things that are happening.
What are you trying to make happen and why those problems in particular?
Peter Bandettini: you know, I'm a little bit biased in terms of what I said was really important. Uh, I'm, I'm actually, you know, we have two seven Tesla scanners and I think I'm lucky enough to have probably the world expert in layer fMRI in high field is, is Renzo Huber. We're working really hard to make whole brain layer fMRI at. 0. 5 millimeters or less or higher, [00:59:00] sort of a robust pipeline for people to ask these new questions, uh, that you could ask with layer fMRI. So that's one thing that we're putting a lot of energy into. I'm putting a lot of energy into trying to pull, generally speaking, pulling more out of the time series. So, so, you know, dimensionality reduction techniques of. Extracting doing dynamic, either connectivity or tasks to be able to the ultimate goal would be to kind of read out every state in the brain as it's changing moment to moment as you go over in time as you go in time. And so we're working on various techniques for for doing that.
One recent one is, um, I have a postdoc who's pioneering, which, which is called. Uh, edge analysis. Basically, two time series, you can correlate, calculate the correlation, but if you essentially multiply each point, you get an edge, and so that kind of gives you a snapshot into how well they're correlated from moment to moment, and so I think there's
Benjamin James Kuper-Smith: So what do you mean by an edge? Like graph theoretic, or edge, [01:00:00] or
Peter Bandettini: Yeah, I think it, I think it links to that. Uh, it's essentially, let's say you have two voxels or two regions. They have a time series and, uh, uh, you could calculate the correlation between the time series, which just is one number you can calculate. What's called like a windowed correlation. If you average like, you know, 10 points and see what the correlation is. That's people do that, which is slow still. But what we're trying to do is the edge is. If you make a separate time series the, the point by point type multiplication of those two time series, and that's what we call an edge. So that's an edge time series, and it's noisy, but it's, it contains a. unique information that's potentially useful that's different than dynamic time series and, uh, and, and just looking at the correlation.
So, so we're doing that, uh, so we're, we're working on many different ways of sort of pulling apart the ongoing signal, [01:01:00] uh, and separating that from. respiration or whatever. Um, so there's one other kind of cool project that's sort of fun that I've been thinking about for 20 years. Uh, and that's going beyond, uh, hemodynamic contrast, uh, trying to look at direct neural imaging. People, and it's funny because I always, whenever a postdoc or graduate student comes into my lab, I always say, Hey, you know, I have this, I, you know, these thoughts, you know, we could try these things and none of them want to touch it with a 10 foot pole because it's, you know, it's super risky and it probably won't work.
And so they don't want something that just doesn't work. And that will, you know, that, and I'm not, I don't push too hard because I, I care about their career. Um, and,
Benjamin James Kuper-Smith: You don't want your lab to be like the place where hopeful postdocs just go and tank their career.
Peter Bandettini: Right, right. Exactly. I, I, I'd like to, I usually like to ask them what they want to do and I throw out ideas, but, but one, this one postdoc as a result. So there's a recent paper that came out. You might have [01:02:00] been aware of it. It's called, uh, Diana imaging, which is. this group in Korea, uh,
Benjamin James Kuper-Smith: Ah, yeah.
Peter Bandettini: fast.
Yeah, and they were able to say that, okay, we can see the signal at 20 milliseconds after, you know, that represents spiking. Everyone was very excited. I actually worked with somebody else in Korea who ran the center, uh, Sanji Kim. Not really, I didn't really work with him, but I had talked with him a lot. And, uh, he tried to reproduce the results. He couldn't. And so we tried to reproduce the results. We couldn't really. Uh, at all. And there's some, you know, and that became interesting because we're trying to reproduce it all. So we're, we're kind of excited and I still think there's probably something there.
What we, what we did, and this is where, this is the one thing that's sort of exciting is, is that. Instead of imaging, we thought, okay, let's make the MR scanner into a big EEG system.
Benjamin James Kuper-Smith: Okay.
Peter Bandettini: Uh, it, we just turn off the gradients, let's turn off the gradients. So if you have the gradients on that makes the image, if you turn them off, you just have RF coils all around the head and they're just [01:03:00] detecting signals.
So it's super high signal to noise. we did these event related responses and we detected signals that were. Pretty rapid that looked like they could be electrical or magnetic. So it's kind of that we see that we see something that we think we believe, but we have to be super careful about, you know, we have to do a lot of control experiments, a lot of tests, but could be fun.
So if we, if you could actually latch onto that signal, then that's fMRI could go.
Benjamin James Kuper-Smith: Yeah, I mean that sounds like the definition of a paradigm shift, right? If, if that were to work and become adopted. Yeah, well,
Peter Bandettini: yeah.
Benjamin James Kuper-Smith: let's see what happens.
Peter Bandettini: the Diana results, I mean, even though it's catalyzed the field to do a lot of things. I mean, Unfortunately, those results, to the best of my knowledge right now, have not been robustly reproduced. So one thing I just do want to add really quickly, there's a lot of other future directions [01:04:00] of, like, clinical fBRI, but, you know, we didn't even mention things like simple application clinically is Is mapping areas of activation or connectivity for the use of neuromodulation techniques that then can localize them better? Uh, that's a potential clinical application. There's a lot of physiology in the fMRI signal. And so a lot of potential clinical applications of neurophys neurovascular Physiology, uh, that are just waiting to be done. There's a lot of great people doing that. Um, so anyway, a lot of other methodology techniques like multi echo and things like that that are great that more people should use. Another thing that's sort of out there that just kind of works. That's really cool is. Is real time feedback, uh, where a person gets fed back, you know, you get to collect their functional images in real time and it gets fed back a cool twist on this, where I think it actually will work is work done by Mikkel Ramat, in which she did feedback below a person's awareness.
So the brain is, the, the FRI scans are [01:05:00] monitoring, they're trying to like. Increase the strength of a connection of, you know, functional connection in the brain and with autism. And what happens is, is that here's how they do it, though they whenever. The scanner, whenever you detect an increase, like spontaneously over time, in the connection between those areas, it gives a stimulus that is like a reward, is like a dopamine.
Benjamin James Kuper-Smith: Well, like a, like a drop of juice with, like with monkeys. So
Peter Bandettini: exactly, well, and here it was sort of a paradigm in which they were trying to, they were trying to somehow mentally fill in a picture. And so they would get a new part of the picture every single time this connection was stronger. So it was like a dopamine. So, and dopamine is an amazing reinforcer, So the idea is that it worked. Is that, uh, with enough of this positive reinforcement, that they didn't even know why it was happening, but it would come from time to time. Their connection, that connection would be reinforced. [01:06:00] And so, that's kind of cool. Um, that could, there's a
Benjamin James Kuper-Smith: I haven't heard about that. Yeah. I mean, I also didn't think that was possible because I assumed the analysis would take too long to make it real time
Peter Bandettini: The complete analysis. Yes, but if you're set up
Benjamin James Kuper-Smith: for one specific. Okay.
Peter Bandettini: Yeah,
Benjamin James Kuper-Smith: Okay. Okay.
Peter Bandettini: Yeah, that's about it. Yeah. Yeah. There's all
Benjamin James Kuper-Smith: That's the entire history of fMRI, yeah, nothing left to cover, cover, um, yeah. So recurring questions, uh, first question is always a book or paper that you think more people should read.
Peter Bandettini: Yeah. Um, that that's sort of a tough question. because I mean, at least lately, I've been seeing so many papers that are just amazing that I would see. I would pass it on my lab. It's like, you got to read this. Um, and then I would sort of like, I would remember essence of the paper and I would sort of forget the paper and it's all in my database, but it's like, [01:07:00] um,
Benjamin James Kuper-Smith: Oh, it doesn't have to be new, right? It can be an old, whatever you want.
Peter Bandettini: yeah, I mean, there's some, would actually, uh, there's some papers kind of along the lines of, of I was talking about that really are really inspiring papers. Um, what is a more recent one? It was in nature. by Evan Gordon. It's called, um, A Somatocognitive Action Network Alternates with Effector Reasons in Motor Cortex.
This was a paper that was cool for two, three reasons, or several reasons at least. Um, one is that it's a brand, it's, it's a new discovery about the motor cortex that I think is one of the. Most significant discoveries since Penfield, uh, first made his maps, um, and that is finding these areas in the motor cortex that are interspersed with the, uh, motor effector regions that to the rest of the brain that actually are connected to areas that are involved with, uh, you know, willing your [01:08:00] action or whatever, or goal oriented behavior. Um, it's a great paper, but what's cool about it, one, it used deep sampling, and two, it involved a little bit of serendipity that I really think is really important, uh, in fMRI analysis. And that's where Evan, uh, he was mentioning to me. the way he discovered this was he had this deep sample data set from a single subject and he was just kind of going along, uh, with the region of interest, just looking at how the rest of the brain correlated as he moved his, his cursor over the brain and he found, wait a second, there's this weird pattern that ever pops up whenever I run it over this area and just putting data through like a pipeline and putting into a matrix.
And then you wouldn't find that. You wouldn't see that at all. So you only see it by sort of of carefully exploring your data. So I thought that's a paper worth reading because it's a cool [01:09:00] experiment, because it uses great methods, brand new finding, and it involves some, lost art of sort of, you know, just looking at your data really, really carefully. Another one that I thought was just beautiful. I remember some of these papers are just not an objective feeling of how good it was, but it was how I felt when I read it. Um, when I read, uh, Yuri Asan's paper, um, it's in science in 2004, uh, intersubject synchronization of cortical activity during natural vision. I, I was like blown away. I was like, this is new. This is really a great idea that is going to shift how a lot of people Uh, do fMRI. It hasn't quite completely caught on, but I think, uh, that paper's worth reading. Alex Huth's paper, which I referred to as well. It was a Neuron in 2012. Great methodology paper.
Also blew my mind because the results just completely in the [01:10:00] face of everyone who says, Oh, language area is here. and, and in fact, there's, there's more to the story. And, uh, that paper suggests that. A really cool paper sheds some light on. Resting state. There's not many papers out there that do that.
But this amazing paper also by the Wash U group, by Newbold in Neuron in 2020, they did something really cool. They took a subject, uh, looked at the resting state and then They put their arm in a cast for two weeks
Benjamin James Kuper-Smith: Okay,
Peter Bandettini: and,
Benjamin James Kuper-Smith: okay.
Peter Bandettini: and, and during this time, they're measuring the resting state connectivity between the left and right motor cortex and found the correlation went way down, uh, between left and right cortex.
So that suggests, uh, it gives a lot of insight about the nature of resting state. It sort of reflects the ongoing. Regular activity that's happens during the day [01:11:00] between areas, and so the degree that it goes down sort of shows how areas are becoming pretty quickly disconnected each other, and it's also suggestive.
He also noticed that there is these pulses. That every once in a while would go through the other motor cortex, sort of suggesting potentially of a, of a mechanism of plasticity, uh, saying, okay, even though it's not connected, there's still the other motor cortex or somewhere saying, okay, wait a second, are you there or not?
And you know, what's, could you do something? It's like, in some sense, that's how I interpret it. That it's sort of, there's so much in that paper and that's called plasticity and spontaneous activity pulses in disused. human brain circuits. So think they got like three motivated volunteers to put their arm in a cast for two weeks.
So,
Benjamin James Kuper-Smith: They must have gotten paid well, yeah.
Peter Bandettini: yeah, well, I think once again, I think it's all part of this, uh, this midnight scan club. It's like, there's, it's a great [01:12:00] model
Benjamin James Kuper-Smith: just briefly, what is the Midnight Scan? You mentioned it earlier briefly, but, uh,
Peter Bandettini: yeah, yeah, it's, it's really worth mentioning. Cause it's an awesome model, uh, that you basically have a group of. PIs, postdocs, graduate students at Wash U who are super motivated and they can only get on the scanner because it's a, maybe it's a clinical scanner or something, or maybe the, the scan, the, the, the amount they pay for the scan time goes down like 90 percent at night. So they jump on it at midnight. And they all scan each other over and over and over and over again, and they've produced some stunningly good papers just doing that and, uh,
Benjamin James Kuper-Smith: Okay.
Peter Bandettini: really pushed the field. So, so anyway, this is one of them
Benjamin James Kuper-Smith: Yeah. Okay.
Peter Bandettini: Uh, Oh, one other one, I just happened to, it's an oldie but goodie that I think has been missed, uh, by most of the field, completely. [01:13:00] that is a paper by Seji Ogawa, uh, was the first author, in 2000. It's one of the last Uh, papers he wrote where he was first author on something interesting, it was called An Approach to Probe Some Neural Systems Interaction by Functional MRI at Neural Timescale Down to Milliseconds. And what he did was something really clever that I'm actually kind of surprised more people haven't done, uh, and that is this. It turns out that everywhere in the brain there's, there's an active inhibitory process. If you have one area active, it inhibits another area. And he actually found that in rodents, you know, with left and right four paw, you stimulate one four paw, it will, it'll send an inhibitory pulse to the other four paw.
And he was able to measure this inhibition. By tuning the delay between left and right forepaw activation and seeing a dip in the activation at about 50 millisecond delay. He also did this in the [01:14:00] visual cortex. It turns out the visual cortex feeds back on itself at a rate of about 200 milliseconds and um, that inhibitory signal is optimum at 200 milliseconds.
And so, So if you tune that, you see an optimal milliseconds. So there's so many cool things of looking at cross network, uh, communication and inhibitory effects that, and since then, I've seen nothing come out and I'd like to try to pursue this as well, but, um, was a great paper. Anyway, I think
Benjamin James Kuper-Smith: Yeah.
Peter Bandettini: I'll end my papers with
Benjamin James Kuper-Smith: Exactly. Enough papers to read for everyone. Um, uh, second question, something you wish you'd learned sooner and kind of how you learned it or what you did about it or
Peter Bandettini: You know, in terms of the science or in terms
Benjamin James Kuper-Smith: I don't know, whatever you want. It could be, I don't know, I mean, uh, often it's something slightly, not exactly personal, personal, but something like, it's just something like, oh, my career would have, uh, not necessarily my career would have been better if I'd learned this sooner, but something that was, I don't know, holding you [01:15:00] back for a while or,
Peter Bandettini: interesting. Um, I mean, there's not too many instances. I, I, I like to think that I, I mean, I've done the best I can to try to make the most of, you know, everything I've had and to try to, you know, push the field, but I think that, I think I would have tried to, I mean, there's a lot of ideas in the field that were floating around that I sort of thought are ideas.
I, I, I probably should have pushed harder on some ideas, uh, um, to maybe get them going faster, maybe, or, I another thing, I, I, I think what happens, and this is something that maybe it's a twist on your question. When I was analyzing data and, uh, writing code and things like that, a lot of ideas came to me when I was very close to the data and now it's still, I still, I feel like I'm a good supervisor, but I, I sort of, I regret a little bit falling away from hands on. Uh, and I think that I really respect people [01:16:00] who are also PIs who are writing grants, who are still doing the coding and the analysis. I think it, it really leverages a lot more power in terms of trying things and doing things as opposed to, luckily, I have really good and, graduate students, so I could just talk to them and they'll do it better than I ever could. But still feel that I would get. Um, I'm still trying to figure out better and more ideas, uh, if I were still, I'm still trying to do that. I'm trying to find, eke out time in the data to, you know, learn Python and do various other things, but one thing. Um, yeah. And there's a couple of other things that were just blatant missing, uh, a result.
Like for instance, when early in the, the, the days of fMRI and, and resting state, I remember actually looking at resting state data. I was a postdoc at MGH and I was just looking at resting state, trying to figure out whether it's real or not. And I saw, uh, you know, the famous default mode network, [01:17:00] and that was before the default node network was a thing. And probably a couple of years later, Rachel and several, and, and, uh, Mike Gracious sort of established it. I remember seeing it. I remember seeing it and like, Oh, that's just probably visual cortex, something, I don't know. And then it just went on. And that's an example of where I didn't, I didn't like sit down and say, Hey, what is this?
This is a thing. And, and that's an example of where I didn't, you know, pay close enough attention to my data and where I, you know, it just got discovered and everything. That's fine. I don't really care, but, uh, I mean, it's great that, uh, but it's sort of, it was a funny thing. It was an example of how I'm, how I actually just blatantly missed something and, and just went on and, and then someone else, then it became something huge.
So,
Benjamin James Kuper-Smith: but do you, is there, um, I don't know, I don't know, How could you have kind of done that differently in hindsight? I mean, also asking, because this is now the second time I've had something like that on the podcast, one of my early interviews was with Kate Jeffrey and she worked on spatial navigation and [01:18:00] rodents and that kind of stuff.
And she had a, she had a, this. image she wants posted on Twitter from 1994, where she, um, she said, like, here are some, like, recordings from, um, entorhinal cortex, where you have these, like, weird place cell kind of, like, activity, and it's, it's a grid cell, and you see, like, now, in hindsight, you're like, oh, I can see, like, a triangle from here, you know, you, you know what it is in hindsight, but at the time, she, you know, just didn't know what to do with this kind of weird signal.
Um, so I'm curious, kind of, this must happen quite a lot, that people see something, You know, in hindsight, it's obvious to see, like, what it is, but can you do the same thing about that at the time, or?
Peter Bandettini: Yeah, well, I mean, that's why you give the people who make those discoveries all the credit because they, they deserve it. Um, I, I think that, okay, so if I were, think I, I think that, you know, I was sort of rushing to establish something for a different purpose. So I think that I think what happens is that, you know, people get skilled at, at looking at the data and [01:19:00] seeing where things are different or don't make sense.
And yeah, I mean, I think what I could have done if I were like, Just collecting the data and I had the mental space to sort of sit down and say, huh, is, this is not like just the visual cortex. This is, I thought it was like empty or something, but it's like, and this is something different. Oh, let's, let's at least probe it a little bit.
Let's see what's going on here. And let's see how it, you know, I could have. You know, taking the time to delve into that and it, and I think that it's not huge leap, um, you know, it would have been a few weeks of trying some more experiments, of establishing it. I wouldn't have, right, making the connection of that Rachel did with his pet studies to say, oh, this area becomes less active. Uh, I don't know if I would, that would have happened. Um, maybe I would have tried a task to see how it activated or deactivated. Um, and also Jeff Binder, [01:20:00] uh, at MCW discovered that too later on by finding this constant area that always goes down with the task. But then it was Mark Grecias who said, Oh, this area is always active and oscillating you're at rest. So that was sort of a, it's hard to make it a formulaic sort of thing to make these connections. It's really hard. It just takes. Certain amount of serendipity, certain amount of skill, a certain amount of mental space, uh, to sort of recognize these things, if you're not pressing for like another result, um, I, don't know, I mean,
Benjamin James Kuper-Smith: because, I mean, obviously you could have just as well, you know, never gotten to the point where you did that because you spent all your time doing more analysis about something else that led nowhere, right? I mean, there's no, there's no guarantee that it would have happened, but I guess it's just generally the question when you know to move on to something and when you say, like, I think I'm going to stick with this for a bit longer.
Peter Bandettini: yeah, and I think it takes a certain amount of courage to, to say, hey, this is interesting where everyone else is like, oh, that's not [01:21:00] interesting or, um, you know, that's not, I mean, it's, I think it's takes a certain amount of, you know, you have to be willing to stick yourself out a little bit to make the, uh, But people who make the big discoveries are the ones who, most people say, Oh, that's just noise or something. Uh, or that doesn't make sense. Or, you know, everyone, it's easy to assess things that way. Um, but to actually make a creative connection. And I think there's still more to be plumbed in resting state. And I'm always trying to foster this approach of like, you know, let's just try to. Figure out how we can pull out this a little bit more and I, you know, once again, it's not a formulaic sort of thing and certain people just comes to naturally.
But, um. always helps everyone to sort of be able to sit back and reflect more deeply on aspects of their data that they wouldn't necessarily pay attention to.
Benjamin James Kuper-Smith: Yeah.
Okay. Final question. Uh, yes. I mean, any advice for people like me who are at the kind of PhD postdoc [01:22:00] transitory period? Um, yeah. Any advice?
Peter Bandettini: I mean, I think that the basic advice, aside from what I just mentioned about experimental, you know, looking at your spirits, um, is to, uh, read as much literature as possible. Less for taking the notes of, you know, what, knowing it, but more pay attention to ideas that come to you as you're reading the.
Papers. Um, I think I always do that. I, whenever I listen to talks or papers, I never take notes on the talk or on the paper. I always take notes on things that pop into my head as I'm listening to the talk, but I also think that the real leverage that that people that people are just getting their PhD or going on to, uh, assistant professorship, the real leverage that they can have in their position is if they span either disciplines or their career. Modalities, if you're really good at some technical thing, and you can ask neuroscience questions. Those are the people that drive the field. Um, you look at anyone, [01:23:00] you know, people like jackal ant or anyone who are just very much combining different expertise. That's where that's where. Things get driven.
And I think that's where the value, uh, of, especially in the field of mapping, that you're sort of resting on this sort of tactical backdrop, uh, where people do really well. If they understand the methods really well, combine them, understand the neuroscience field well enough to ask really interesting questions that might use the methods in a unique way, then you're golden.
And then you keep on doing that and you'll be fine.
Benjamin James Kuper-Smith: Okay.
Peter Bandettini: So.
Benjamin James Kuper-Smith: Yeah. Unless you have anything else to add. Uh, thank you very much.
Peter Bandettini: Oh, thank you. Thank you. This was fun.