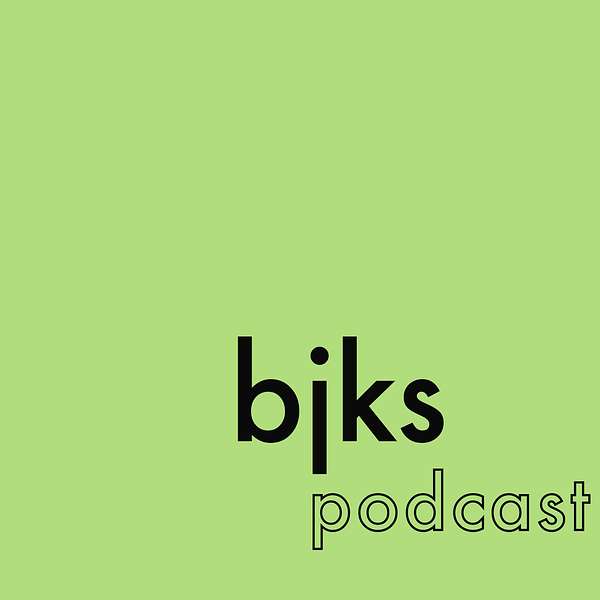
BJKS Podcast
A podcast about neuroscience, psychology, and anything vaguely related. Long-form interviews with people whose work I find interesting.
BJKS Podcast
75. Paul Smaldino: Modeling Social Behavior, the value of false models, and research beyond traditional disciplines
Paul Smaldino is an Associate Professor of Cognitive and Information Sciences at UC Merced, where he studies the evolution of behavior in response to social, cultural, and ecological pressures. In this conversation, we talk about his new book Modeling Social Behavior, everything related to formal models of social behaviour, and Paul's path to where he is today.
Support the show: https://geni.us/bjks-patreon
Timestamps
0:00:00: Paul's new book 'Modeling Social Behavior'
0:04:42: Paul's somewhat circuitous route to doing what he does today
0:25:54: Why so interdisciplinary?
0:36:58: The importance of (metaphorical) violence in modeling
0:46:26: Newton's model of gravitation ignores almost everything
0:52:11: Exact vs inexact sciences
1:00:02: From simple to complex models of cooperation, and the complementarity of simulations and equations
1:11:48: When is formal modeling appropriate and when is it too soon?
1:27:47: A book or paper Paul thinks more people should read
1:32:46: What Paul wishes he'd learnt sooner
1:36:20: Any advice for PhD students or postdocs?
Podcast links
- Website: https://geni.us/bjks-pod
- Twitter: https://geni.us/bjks-pod-twt
Paul's links
- Website: https://geni.us/smaldino-web
- Google Scholar: https://geni.us/smaldino-scholar
- Twitter: https://geni.us/smaldino-twt
Ben's links
- Website: https://geni.us/bjks-web
- Google Scholar: https://geni.us/bjks-scholar
- Twitter: https://geni.us/bjks-twt
References
Previous episode with Paul: https://geni.us/bjks-smaldino
Axelrod & Hamilton (1981). The evolution of cooperation. Science.
Boyd & Richerson (1988). Culture and the evolutionary process.
Friston (2012). The history of the future of the Bayesian brain. NeuroImage.
Giraldeau & Caraco (2000). Social foraging theory. Princeton University Press.
Giraldeau & Gillis (1985). Optimal group size can be stable: a reply to Sibly. Animal Behaviour.
Gleick (2004). Isaac Newton.
Glimcher (2004). Decisions, uncertainty, and the brain: The science of neuroeconomics.
Hamilton (1964). The genetical evolution of social behaviour. Journal of theoretical biology.
Kauffman (1970). Articulation of parts explanation in biology and the rational search for them. PSA: Proceedings of the Biennial Meeting of the Philosophy of Science Association.
Kay (2010). Obliquity.
Nowak & May (1992). Evolutionary games and spatial chaos. Nature.
Smaldino (2023). Modeling social behavior: Mathematical and agent-based models of social dynamics and cultural evolution. Princeton University Press.
Smaldino (2017). Models are stupid, and we need more of them. Computational social psychology.
Smaldino, Pickett, Sherman & Schank (2012). An agent-based model of social identity dynamics. Journal of Artificial Societies and Social Simulation.
Turchin (2003). Historical dynamics: Why states rise and fall.
Wimsatt (1987). False models as means to truer theories. Neutral models in biology.
Wimsatt (2007). Re-engineering philosophy for limited beings: Piecewise approximations to reality.
Zukav (2012). The dancing Wu Li masters: An overview of the new physics.
(This is an autoamted transcript that contains many errors)
Benjamin James Kuper-Smith: [00:00:00] You have a book out very soon. It's about, I think, a week now or something from today, right? Um,
and I guess by the time this is out, uh, it will be around the publication time. I've been looking forward to a book for quite a while. I think you announced it like, was it like a year ago or something like that?
Um, half a year ago?
Paul Smaldino: Yeah, I don't remember exactly when, but been eagerly anticipating this.
Benjamin James Kuper-Smith: Was it something you always wanted to do? Or was it because you've been writing this kind of quiet, I mean, the first time we talked, it was very much about kind of how to and why to do, uh, modeling and they were already very much written in a kind of educational. Like didactic kind of way.
Paul Smaldino: I'm not sure. I think that I felt, as I learned how to do modeling, I felt like I had something to say and add to the conversation. And I remember when I got hired at UC Merced. They asked me, you know, are there any classes that you would particularly like to teach and I thought about it a [00:01:00] bit and I said, you know, I'd really like to teach a course called something like modeling social behavior. And they said, would it would this be an undergraduate or graduate level course? And I said, let's do both. And. I started, especially for the graduate level one, um, putting together, readings, I, I looked for a book that kind of did what I wanted to do and, and was close enough to the course that I wanted to teach, and I just, there wasn't anything. There, there are many good books on modeling out there, but there wasn't any that, um, took the perspective that I wanted to take and, and presented all the material, or at least enough of the material that I wanted to present. So. I started notes about, uh, you know, what I wanted the students to know. And I think this, I don't think this is a particularly unique to me. I think many, many textbooks and, and related books come out of sort of pedagogical experiences, [00:02:00] courses. So I was putting this together, I was like, Oh, I'm basically writing a book. And maybe I should just write a book.
Benjamin James Kuper-Smith: And then it was, Oh, I just have these notes. I'll compile them quickly into a book. And then it takes two years to do it. So
Paul Smaldino: Oh my god, I remember when I first started writing, and I kind of outlined the book, and I thought, you know, I'm sure editing will take a long time, but I thought, I bet I could have a finished draft of this book in six months. And
Benjamin James Kuper-Smith: that?
Paul Smaldino: it was more like three years. Uh,
Benjamin James Kuper-Smith: Do you want to maybe just say like, yeah, what the book's called and roughly who it's for.
Paul Smaldino: Yeah, so the book is called Modeling Social Behavior. Subtitle mathematical and agent based models of social dynamics and cultural evolution. I wrote it as sort of textbook on what models are, and then working through lots of example models and model families, uh, I worked through [00:03:00] a number of sort of what I think of is really important or seminal models in understanding things.
Thank you. Social behavior, not all of social behavior, obviously, but, uh, enough aspects that I thought, you know, this provides a really good foundation for lots of social and behavioral scientists broadly defined. so I, I sort of wrote this as a graduate level textbook for 1st and 2nd year graduate students, uh, in the social sciences, but hope is that it's. interest to other people, I can using this from an upper division undergraduate course, or just as a sort of primer for self study for anyone interested in building these kinds of models. So, you know, the 1st chapter goes through the philosophy of modeling. And then I go through a number of different modeling frameworks and ideas about collective behavior, segregation, contagion, polarization, cooperation, norms, [00:04:00] uh, the scientific process, and social networks. Uh, and I end with, uh, sort of a chapter on how to integrate these kinds of models with an empirical research program.
Benjamin James Kuper-Smith: Yes, I mean, I guess we'll, we'll be mainly talking about the book and modeling and all the things that I'd like to, to learn about this as someone who's not very experienced and probably could profit from taking your course. Last time we talked about, uh, kind of why, particularly, there was a lot of question about like, why would you need to do this?
We, uh, explored your cubist chicken story a little bit. Um, so I guess we don't need to do that today. It's, I think it's literally the beginning of last episodes. I'll link it in the description. But yeah, um, before we kind of do that, I was curious, like, uh, I usually like to look a little bit into the background of the people I interview to kind of, uh, see kind of how they got from, I guess, just how they got to where they are today.
And you have a particularly kind of interesting short bio on your website. Um, so I'm [00:05:00] curious, Uh, if you don't mind that we kind of just take that journey in a few minutes. So kind of like you studied physics first. Uh, what did you think you were going to do with that or why did you choose physics?
Paul Smaldino: Sure, um. I, I think, you know, I came of age and I, I started college university, uh, in the late 1990s it was, I think at least for me a very optimistic time I, I sort of thought, well, I could do anything. And am I interested in? I'm interested in sort of, was very kind of Head in the clouds or idealistic and I thought I'd like to understand and, you know, the fabric of the universe and very, very humble goals and, uh. I was, uh, in high school and I was, uh, the summer between my, um, junior and senior, my, my last, right before my last year of high school, uh, I read a book by Gary [00:06:00] Zukav called The Dancing Wooly Masters, An Overview of the New Physics. And it's sort of a pop science book about quantum mechanics and relativity. And I thought, is the most interesting thing I've ever read. I want to know more about this and understand the nature of the universe. And so I called up my high school and I said, I'd like to enroll in AP physics, which is sort of a college level physics class that they offered. And I, uh, switched from, I think, marine biology, which is what I was originally signed up for. So I went off and I, but I went to Wesleyan University, which is a kind of a liberal arts college in New England. Which is this, there's this sort of type of college in the US, especially in the Northeast it's, it's very much not a concentrated program in physics. Like, I certainly took physics classes and enough to major in it.
And I took some math classes, but [00:07:00] I took a lot of other classes and, uh, all the, I, I
Benjamin James Kuper-Smith: Like, like what?
Paul Smaldino: so this is the thing. I'm now a professional social scientist, uh, slash cognitive scientist, And I took zero cognitive or social science classes in college. All my classes were math and physics and then humanities.
So I took film and art and history and philosophy, mostly. loved those subjects. most of my friends were film majors and theater majors in college. I had a couple of other physics major friends, but, um, most of my friends weren't science people, they were arts people. And so, you know, I think what happened was I graduated college with a physics degree, didn't know what the hell I wanted to do.
Benjamin James Kuper-Smith: So you didn't want to be a researcher in...
Paul Smaldino: I didn't really think about it. Like I said, it was a very optimistic time, and I was [00:08:00] really kind of very enamored with the sort of be here now philosophy, where I was just sort of. the moment and studying the things that appealed to me and reading the things that appealed to me and doing the things that appealed to me.
And then all of a sudden college was over and I had to actually go get a job. I, I, I very specifically remember a few days before my graduation, I was at a party and I started realizing in a couple of weeks, I'm going to be home and this will be over. And I have no plan. And I a bit of a crisis. And I threw, I sort of was like, what should I do? Maybe I should become a, I should do something. I like helping people. I like doing something kind of pro social. I like science. I should become a doctor. And so I basically through some family connections and just sheer force of will I bullshit my way into a position as a lab tech in an immunology lab at Sloan [00:09:00] Kettering Cancer Center in New York. And. having no biological training at all. And
Benjamin James Kuper-Smith: Right, it was maths and humanities.
Paul Smaldino: maths and humanities. I bought myself an immunology textbook so I could catch up on what the hell we were doing. And we'd read it at night. I got fired from that job after about three months. I didn't do anything that terrible.
They were just like, you know, we've got people. Banging down the door for this job. And you're, you don't really seem like you want to be here. I was like, that's fair.
Benjamin James Kuper-Smith: Do you agree that you didn't want to be there? Like, did you?
Paul Smaldino: absolutely agreed. It was very clear it wasn't for me. I was, uh, it was very high pressure. was very, I wanted to have a job where I could tinker and solve problems. And this was very, like, very brass tacks. Let's get down to business. Here are very clear things we need you to do. And it was, there was no fun for me. And I, it was very, like, if I screw [00:10:00] up, then. You know, it people were relying on that lab for, you know, the development of cancer
Benjamin James Kuper-Smith: Heh heh heh heh.
Paul Smaldino: a good fit for me. It was way too like, I, oh, my God, people who can do that work. I salute them so much. It's so important, but it's, it's,
Benjamin James Kuper-Smith: Yeah.
Paul Smaldino: not for me. is actually, like, the history of, I don't know how, how detailed we want to go and sort of my early work history, but like. It was basically a long period of trying things out and then being like, this is not for me. I, I spent some time moved, went back to the arts and I started working in film and music and I, the next job I had was as a studio manager and assistant engineer at a recording studio that worked on music for television commercials mostly, which was really, it was really fun.
I really liked that job a lot. Uh, but ultimately I, I, it was just like, I couldn't really hack it.
Benjamin James Kuper-Smith: By Hackett?
Paul Smaldino: I, I [00:11:00] didn't want to be an engineer. Well, an engineer is, is a lot of work and I, and I, I couldn't really find an in to doing that full time. Maybe I could have persisted. I think I wanted to be an artist and, you know, sort of be a musician, but I, I don't have the, don't know, regimen or persona that, you know, uh, makes for, for that kind of person, I suppose the same with film.
I did some work as a production assistant on some films. And this is actually, I don't know how detailed we can go, but like, this is a funny story. I had been working on some films and I did, uh, I got hired on a short film as a production assistant. And, uh, I got to be friends with the, the director of photography and by the end he was having me, I was basically his assistant camera person.
I was setting up shots and he was like, you know, you really have a talent for this. You should pursue this as a career. [00:12:00] so I kind of did a little bit and I remember I had been offered a job, but it was unpaid as an assistant camera person on a feature length film. It was going to be a horror film shot in 35mm in Brooklyn, and I was running out of money, and a friend of mine was like, you know, I have a, I work at a, he worked at a, this is back before smartphones, there were like, cell phones, you could buy ringtones, and,
Benjamin James Kuper-Smith: Yeah.
Paul Smaldino: and games for smartphones, and there was a, he worked for a company that produced these, and basically me a job working for that company, Uh, but he's like, you have to let me know by the end of the week. I also had this unpaid job offer as a direct, uh, assistant camera person. And so I, I got on the phone with the director of photography and he was like, you know, they're screening the last movie I shot at this theater and why don't you come and you can come to the screening and then we [00:13:00] can have coffee after and talk about the job. So I, I said to my, made myself a deal. I said, if this person has talent. And I would benefit from working with them. If this movie is well shot, I don't even care if it's a good movie, but if it's well shot, I will take this job and I'll scrounge together enough money to live for another month. And if it's not well shot, I'll take the job with the cell phone ringtone company.
And I went and it was, the movie looked awful.
Benjamin James Kuper-Smith: Heh heh heh heh. Yeah. Heh heh heh heh.
Hmm. Heh
Paul Smaldino: boring, flat, sort of soap opera lighting, all medium shots, just nothing interesting at all. And I was like, I can't, I have to turn this down. And that was the last job. worked in film again, which is perhaps ironic, but I worked in that job. speed this up, but uh, I ended up with a job at a film and video transfer company where [00:14:00] people would bring in their old 8mm and 16mm film, like You know, here is a bar mitzvah from the 1960s that I found in my grandmother's attic. And can you put this on a DVD? And so my, I was doing that and it's, it was a fun job.
Uh, pretty low pressure and I was in the back room by myself a lot working, doing, running these films. the, the job was just half a block from the Strand Bookstore in New York, which is one of the, the sort of biggest
Benjamin James Kuper-Smith: heh
Paul Smaldino: bookstores and has a large used bookstore, uh, book selection on the street. So they had all these old paperbacks that were 48 cents a piece, or five for $2. So I would walk there on my lunch break and I would come back with like 10 books at a time, spending $4 on 10 books, and I would just read a ton. And I would take my lunch break and I started reading more about, you know, human behavior [00:15:00] and, and thinking more about people and I would take my lunch sometimes in Union Square and just watch people walk by and I think I started thinking more about people and, and trying to understand them and realizing I didn't understand that much about them.
I had thoughts, but I felt like it was all just speculation. And I, I, the other aspect of that time was I was, I ended up at a lot of parties where there would be a lot of kind of stonery conversation around like, man, you know, the world would be better if everybody just did something or things were just like this. And I, you know, very idealistic and I like conversations like that, but two questions kept coming to my mind. One was. Would it be better if things are really like that? Like, there are a lot of assumptions that go into thinking things through. I think, you know, given how interconnected [00:16:00] everything in our world is, if we change certain things, then that's going to have a lot of downstream consequences, and it becomes difficult to think through all those consequences. And two, let's say it is. Let's say it really is better. You can't just snap your fingers and then it, the world becomes that. Thank you. You have to get there from where we are now. So, what are the processes by which the way the world works changes, and people change their behavior? So these were the driving questions I had in my mind, and I kept thinking about them, and because I didn't, I had never taken any behavioral, or social science classes, The only thing I knew about was psychology, and the only psychology I really knew about was clinical psychology. So I decided to go study clinical psychology, and I applied for PhD programs in clinical psychology, and THANK GOD I didn't get into any of them.
Benjamin James Kuper-Smith: heh.
Paul Smaldino: And I, I got a [00:17:00] job as a psychiatric nurse's aide at a hospital. It was at Bellevue Hospital in New York. And the only place they were hiring was in forensic psych.
So it was, you know, the people who also in the corrections program, they were, you know, in jail for some crime, usually not convicted, but they had been arrested for something, sent to Rikers Island prison in New York, or just brought directly to Bellevue. then I was just there helping take care of them and, uh, it was a very illuminating experience for me.
I learned very quickly that much like the Cancer Research Center, I shouldn't be involved in direct care of people. I was much more interested in the way the thing that was fascinating to me. There was not. The patients and their abnormal psychology, but the system of the, the sort of corrections facility and the institution of the [00:18:00] mental health facility.
I found that most interesting. Doctors who came into the ward only, you know, once or every week or 2 very often mistook me for a patient because of my demeanor, I guess. but through that, I ended up getting a, uh, a master's degree in psychology.
Benjamin James Kuper-Smith: Which means through that.
Paul Smaldino: Well, during that process, so I had applied for, um, A degree in clinical psychology and didn't get in, but at the recommendation of a family friend, I also applied to this master's program at the new school in New York and got into the master's program because as many people know, it's easier to get into a master's program than a Ph.
D. program because you have to pay for it. And I did that. And I had a great meeting with, um, the great Marcel Kinsborn, who's a cognitive neuroscientist and neurologist, um, who is my professor there, and I started talking about all the things I was interested in and he, he kind of paused and he looked at me and [00:19:00] he said, well, you're in the wrong school. So, finish the masters because you're already here, but you should get yourself into a research lab at NYU or Columbia and get yourself into a research university that's doing more things that you're interested in. And I did, I ended up working in a. Cognitive neuroscience lab at NYU for a year. It was really fun.
I learned a lot. I learned that I didn't want to be, uh, doing psychophysics or, you know, perception, memory kind of stuff. And then looking in PhD programs, I'll speed it up, but I, I got, I found this weird guy at UC Davis named Jeff Schenck, who was doing these like computer models and robot models of rat collective behavior.
And I was like, I don't know what this is all about, but this sounds cool and interesting. And I think I wrote this application that must have been in, it must have been insane. I don't have a copy anymore, but it was like, I'm interested in decision making and the brain and animal behavior and evolution and robots and complex systems. [00:20:00] And I think most people were like, all right, this guy doesn't know what he wants to do. No. And I think Jeff was like, this guy doesn't know what he wants to do and is all over the place. We can use this. so. I got accepted to UC Davis and it was incredibly serendipitous because I was in the psychology program there. Didn't feel like I fit in in the psych program at all, because I was interested in all this other stuff and doing these computational models and starting to get into evolution and game theory and social dynamics. at the time, especially, UC Davis was this hotbed of activity in cultural evolution and human behavioral ecology, you know, these amazing people like Pete Richardson, Richard McElreath, Monique Borgerhoff Mulder, Bruce Winterhalder, you know, other people, Mark Lubell, an incredible collection of people and the cohort in that lab of other grad students, you know, most of my Friends from grad students, a lot of them are from that program and not from the [00:21:00] psychology program.
So that was really like where I, I sort of found a place and. From there, I just kind of became a social scientist, which was, I did a postdoc in this strange center popped up and then was gone a few years later at Johns Hopkins called the Center for Advanced Modeling in the Social Behavioral and Health Sciences run by Josh Epstein. And I was, I emailed him cold and I just said, I saw you have a big grant. Do you have any money for a postdoc? Do you want to hire me? And he was like, Oh, interesting. Let's talk and then I was the first person hired at that center, uh, which was wild in Baltimore, uh, and then I came went back to UC Davis and they did a postdoc with Richard in anthropology. And that's where the sort of modeling science out of, um, that I'm into. And we can talk more about that. And then I gave a talk in the communications department while it was there, because somebody heard I was, I had opinions about modeling [00:22:00] and wanted to talk, you know, convinces the communications department that modeling was useful, so he asked me to give a talk and I was like. Is there a job? he said, no. so I said, well, then it doesn't matter what I say. So I'll say whatever I want. I wrote the talk that I really wanted to give, which was ended up being a, the chapter, uh, are stupid and we need more of them. So I wrote this as a kind of like, I'll just say what I think. And at the end of the talk, a guy came up to me and was like, are you looking for a job? Uh, and I was like, yes. And he goes, well, we have a postdoc. we, you know, need to hire and it would be a joint appointment in political science and computer science, you know, doing these modeling multiplex networks. So I ended up based on that, an interview for the second for this next postdoc, like the next week, which was lucky because my postdoc with Richard only lasted a year. Um, that's all the money there was. [00:23:00] And. I worked there. So, as you know, it was, uh, the, the Hopkins center was technically in the school of medicine. So I guess I went from physics to psychology to medicine to anthropology to political science slash computer science then there was this job ad for a faculty position in, uh, computational science and data analytics broadly defined at UC Merced. And I came in and discovered this department called Cognitive and Information Sciences, and they were like, well, we want to do cognitive science, but also network science and social science all wrapped up together.
And with a very, you know, a focus on complex systems and computational modeling. And I thought, well, that's sounds like a good place for me and they hired me. so that's where I've
Benjamin James Kuper-Smith: So you could just reuse your previous application to, uh, what was it? Jeff Shank. Like I like all of these things.
Paul Smaldino: I mean, it was, uh. [00:24:00] I remember I had missed the deadline for the application for that job. And so I thought, well, that's fine. I nevermind. I won't apply. then a friend of mine sent me an email and said, look, they've extended the deadline for this job. You should apply, but it's due in two days. And so I, I, I think I threw together that application in like something like two hours and just was just like, all right, here you go.
Here's who I am. Let's see what happens. And yeah, I guess right place, right time, right fit.
Benjamin James Kuper-Smith: It's really weird. I feel like in my case, I mean, I've, I've written a few applications even, uh, you know, over, over the years for like scholarships or whatever. And I think the, the only ones that worked were the ones that I had to write in less than a day, often in a lot less than a day. I don't know. It seems to always, those seem to work, but unless you, but you can't plan for it.
You can't wait until the last day and then write. It has to be like.
Paul Smaldino: No, I think that there's something about, like, Okay, you need to tell me why you are a good fit for this. Right now. And that doesn't work if you're not actually a good fit. [00:25:00] But if you are a good fit, sometimes, like, the first, the first things that come to mind end up being the right ways to connect. I don't know, maybe this, maybe this is all nonsense and it's not actually a good strategy.
Benjamin James Kuper-Smith: It's probably just me, uh, justifying why I procrastinate and everything. Um, cause they're like, well, that one time it worked. So better do it again. Yeah. Yeah, but it's great. I mean, uh, I, I, you know, I know that you have your, what is it? Paradigmatically promiscuous scientist or something like that is your tagline on your website or something.
Um, you really are like covering, I think you still have to enter law, like a legal department or something. I think then you've kind of got a university covered like from,
Paul Smaldino: Yeah, well, you know, this is all time.
Benjamin James Kuper-Smith: you know. Yeah, it's kind of interesting. So I'm, I'm trying to, you know, at some point tie this back to the book and I guess that kind of does give a fairly, um, I should put it, your, your background in various fields does match the examples you give. [00:26:00] From, from the book and what the individual chapters are and all that kind of stuff.
I'm curious, was that, kind of, what was the, um, I mean, the question basically is why. I'm just trying to make it slightly more intelligent. Um, and kind of, uh, I'm wondering about, like, did you try and, like,
Paul Smaldino: or why is the book this way?
Benjamin James Kuper-Smith: yeah, why is it, like, why not, you know, go, like, okay, I'm going to write something for people who are interested in only the evolutionary parts or something like that.
So then I have one book that all of those people... Well, like, versus maybe now someone says like, Oh, I don't, I don't care about that topic. So...
Paul Smaldino: right. Well, to some extent, I think there are a lot of books that already exist of that vein. I was, you know, I sort of over time became interested in questions about human, mostly human social behavior, and The dynamics of culture and the interplay between individuals and their social [00:27:00] and cultural environment, how cultures change over time. And I thought, well, there's, there's a lot that I, you know, want to do here, but I also found, you know, some of the old school social science, like sort of economics based, um, approaches or political science based approach is kind of dry and. disconnected from large, more fundamental or foundational theories about why people behave the way they do in the first place.
What kinds of things can we expect? I mean, economic models, at least microeconomic models often assume just kind of, well, we're going to assert that people Have these kinds of preferences or these kinds of proclivities, and then we'll model out, you know, what the optimal behavior is or what the outcomes will be based on those.
And that, that's a very fruitful way to model. But ultimately, you have to make assertions about where those preferences or other kinds of [00:28:00] features or our behavior come from. And most economists don't really have a theory about why they are the way they are. I was really taken with approaches from, evolution and behavioral ecology, because once you have, you know, evolutionary dynamics at your disposal, you have to think, well, everything that is now came from somewhere, came from something else. And what are the forces that shape it in the way that it does? And are evolutionary dynamics. It's not just the dynamics of genetic evolution. also have cultural evolution, which works in a, in a, in different ways. And I was really inspired. I mean, like I mentioned, I, I had the great fortune to be UC Davis, uh, and interact a lot with Pete Richardson, one of the great founders of sort of Sort of father figures of modern cultural evolutionary theory. [00:29:00] Richard McElreath was there, you know, no slouch in his own right. Also, you know, he was a student of Rob Boyd, uh, one of the other sort of greats of, of cultural evolution and, you know, you had Monique Bergerhoff Mulder and Bruce Winterhalder, sort of really important people in human behavioral ecology, thinking about how to use ecological models to think about human behavior And the more I got to learn about what they were doing and the perspectives they were taking, I just thought this is the correct way to think about all behavior, including human behavior, where you start with. needs, biological needs, and then you kind of work through how those needs are met and then what the limitations on their them are. And we can talk more about that in more detail. also, I mean, you see Davis, especially at that time was just magical because also it had this incredibly good. does, but this incredibly good animal behavior program and, uh, so not only [00:30:00] ecology and evolution, but animal behavior. And I learned a lot. I took, you know, course fundamentals of animal behavior was, as I was getting into modeling, I. Started reading a lot of books and, and papers from behavioral ecology.
One of my all time favorite books of modeling books is this book by Carrico and Giraldo 2000 called social foraging theory. Now the whole book is basically a series of mathematical explorations of ideas about like, how do groups. Form. How do animals join and leave groups? What is the optimal group size that balances their needs and the cost of being group? What, how do groups maintain certain sizes when the incentives of members to join new groups are different from the incentives of current members to keep out new members? You know, if, if I'm exploring something and you want to learn [00:31:00] from me, what is the sort of optimal frequency of sort of innovators or producers versus copycats or scroungers? And so it was these, these series of, of really fascinating questions. And then. As I started interacting more with social scientists and social psychologists, I, I. I was fortunate to, to start interacting a little bit with, with these social psychologists, Cynthia Pickett and Jeff Sherman, who were interested in social identity from a kind of old school, social psychology perspective.
Um, I mean, it was, uh, uh, they were, they were interested in this perspective called optimal distinctiveness theory, um, developed originally by Marilyn Brewer. And it was this idea that like, Well, it's very intuitive in a sense. It was about like, well, why do people express the identities that they do? Well, it's because we have these drives for, we want to belong.
We want to assimilate with others. So we will [00:32:00] therefore adopt the identity, you know, that gives us a sort of group affiliation. But also, if we were all in one group, then we would get crowded out. We would feel just sort of, you know, Submerged in the group and we couldn't assert ourselves and we couldn't differentiate ourselves from others.
So there's also this sort of opposing need for differentiation from others. And these 2, you know, opposing forces lead to a sort of when they're balanced. That is the optimal level of distinctiveness that you're the group you express will be. And it's a very kind of social psychology theory, which it's it.
It makes sense when you kind of describe it verbally, but. When you think about why those drives should be there and whether, well, why those drives should be there is an evolutionary question and is a good question. We can get to that. But, but, you know, a more fundamental question was the verbal theory that Brewer had been describing for a while, which is sort of asserted that, well, when these [00:33:00] opponent processes. Are both in action, then, and everybody individually has an optimal level of distinctiveness they want to express. Does that actually lead to everyone being able to do so, given the fact that everyone is also doing this together? So if everyone is, like, expressing different identities, joining and leaving groups, based on these individual level drives for distinctiveness. Do you end up with a population in which everyone is in a group that maximize or optimizes their distinctiveness? And nobody knew. So, they found me because they knew I was interested in modeling and social behavior. And we built this very simple model. In a way, the paper is out there in the world. It's written from a very kind of naive perspective.
I had so much to learn at the time when I was doing it, but I just sort of built this model that could [00:34:00] belong to groups and they all had like an optimal level of distinctiveness that they wanted the group to be relative to other groups and they would join or leave groups based in an effort to maximize that distinctiveness. And lo and behold, what we found was they didn't maximize their distinctiveness. What ends up happening is they all ended up in groups that were too big. Because groups grow at a rate that's smaller or slower than the small groups shrink. so what ends up happening is by the time the big groups are too big, the small groups have gotten so small that they're less appealing than the big groups.
Benjamin James Kuper-Smith: Less appealing because,
Paul Smaldino: Less appealing because to join one of the small groups would put you so far below the optimal level of distinctive, like you would be too distinct.
Benjamin James Kuper-Smith: I see. Oh, because they're in a lot of the, yeah, okay.
Paul Smaldino: Yeah, it would be too distinct. So you end up, your options are join a group where you're, you don't have any sense of belonging or be in a group where you have a hard time [00:35:00] differentiating yourself a little bit. And so ends up, everyone ends up being in the same group. And we let, you know, also I did some stuff with networks and we're like, okay, well, people are on networks and they're all responding to different local environments, local groups of people, then you can get closer to this optimal level. But the general, the baseline finding of everyone being in two groups, I later discovered this paper came out in 2012. This was a principle that had been discovered by behavioral ecologists in the early 1980s. Okay.
Benjamin James Kuper-Smith: What's it called there?
Paul Smaldino: It's just called optimal group size uh, you know, where people like Geraldo and, uh, Sibley wrote these great, yeah, these great papers, uh, in the journal animal behavior, I think from, from the early 1980s. And that was also a lesson where it was like, wow, like they're thinking about these things in a very deep way. And what was really cool is in the journal, there was this back and forth where people were like, oh, look, you get [00:36:00] this result. And then someone else was like, yeah, but if you make different assumptions, that result doesn't hold. So I was like, okay, well, so now we can refine the theory to think about, it's not just everyone who, you know, if there's an optimal group size, everyone's in a group that's too big. It has to do with the sort of shape of the fitness function that relates to, What it is to be in groups and and how when you deviate from optimal, bad does it get?
And how quickly does it get bad? If you go too big or too small the shape of that curve will affect sort of how. much you end up overshooting, uh, the optimum. And in some cases you can get right there if the shape of the curve is kind of this asymmetric thing. If it's like not that bad to be in two small groups, but it's very bad to be in two bigger groups. So it's a good lesson in assumptions.
Benjamin James Kuper-Smith: Um, yes, I thought we could, [00:37:00] uh, go through some of the You know, talk about some of the chapters, let's say that, uh, that are in your book and kind of discuss some of the points that I had was reading it and, uh, maybe to start off kind of, uh, in your, in chapter one, it's called doing violence to reality. Um, I was curious whether you could, uh, first maybe just expand on, uh, what, what kind of violence you advocating for and whether it's necessary.
Paul Smaldino: So, yeah, to be clear, uh, it's, uh, I'm talking about metaphorical violence, um, well, so the book is about modeling and about building models and specifically models of social behavior and the bulk of the books or chapters. There's 11 chapters in the book and chapters, you know, 2 through 9 are. All sort of deep dives into let's, let's build up and work through some concrete, some models and code them and analyze them and study them and talk about how they [00:38:00] work and why, I wanted to open the book with a sort of philosophical perspective.
And why are we doing this at all? What is this that we are trying to do? How does it help us do science? there is um, a great quote that I, I opened the chapter uh, with uh, an epigraph from Jakob von Uxkuhl. Who is this, I
Benjamin James Kuper-Smith: No, sorry, I'm, I'm grinning just because I think he's German and I have no idea how to, I grew up in Germany. I have no idea how, it's, it's a really weirdly written name.
I actually don't know how to pronounce it.
Paul Smaldino: I I Xkuhl,
Benjamin James Kuper-Smith: Ux, yeah, I don't know, it's, it's a Y, wait, let me just,
Paul Smaldino: I I
Benjamin James Kuper-Smith: it's this. Yeah, but the funny thing is, I, I also thought like, I don't know how to, Ux could, because, yeah, anyway, doesn't matter, sorry.
Probably Ux could, but yeah.
Paul Smaldino: yeah. Okay. So let's say Usku, was this kind of, I remember learning about him when I took animal behavior. He was this sort of proto [00:39:00] ethologist before ethology was even really a thing where he, you know, he was very interested in relationship between organisms and environments, uh, and did some really cool, uh, experiments and studies, but he had this amazing, amazing, Quote, and I'll just read part of it.
I have a longer version of it in the book, but he says, you know, if we want to make reality and therefore truth useful to science, we must do violence to reality. We must introduce the distinction which does not exist in nature between essential and inessential. In nature, everything is equally essential. By seeking out the relationships that seem essential to us, we order the material in a surveyable way at the same time, then we are doing science. So, this really stuck out to me in an amazing way, and I thought, this guy is bang on. Science is not really about the quest for truth. It's because... [00:40:00] I don't know.
To some extent, truth may be impossible. It's a philosophical question. We can get down into the weeds, but I know what kinds of truths, et cetera. But ultimately, right in science, we're trying to build up explanations for the way things work and why we see what we see why we observe what we observe. Uh, we want to, we want to sort of catalog. Observations and the things we discover in the world and find out sort of what there is in the world. And that's part of science too. But then we want to explain what it is we're seeing and why things are organized the way they're organized and ordered the way they're ordered. Why, you know, certain events lead to other events or associated with other things and. If we really tried to get a full explanation, like, the full explanation involves the fundamental fabrics of the universe, right? It has to do with quarks and m brains and [00:41:00] strings and, you know, nine dimensional space time, it, it becomes completely useless if we're interested in things like behavior and, you know, social, All right. Right. What we end up wanting is an explanation that's based on the things that we can measure and the things that we care about. This really relates to, um, the sort of the great complexity scientist, uh, Stuart Kauffman has this, uh, paper from the 1970s that, It's very influential to me called on the articulation of parts, explanation of biology and the rational search for them. it's a terrible title, but a great paper. And, uh, you know, what he is saying is, you know, very, very much in line with what von Uxkuhl is saying, which is that if you want to build up an explanation of anything, you have to your system and break it down into parts. And. [00:42:00] Those parts are not going to be a complete list of all the parts that are really there in the universe that are involved in the system. There are going to be a subset of those parts that are important to the kinds of explanations that you want and the kinds of questions you want answered by that explanation. And so, by breaking down the system and sorting it into the parts that are important to the theory and the parts that I don't care about, That allows me to build up scientific explanation.
Von Uschel is absolutely right here. He's saying this distinction between the essential and inessential is not a part of nature. In nature, it's just, it is what it is. But if we're trying to build up an explanation, we have to say, well, some of those parts are going to be key to my explanation. And some of them I'm just going to ignore because they're not sufficiently important. And because I can't, my know, little brain is only [00:43:00] big enough to hold some of the facts of the universe and not all of them.
Benjamin James Kuper-Smith: Yeah, and I guess, I don't know how, I mean these are maybe separate points, but I guess also to some extent also you can only measure so much if you want to relate it to any actual observations, so like there's no point in having something that requires all these different inputs that you can't measure at the same time anyway.
Paul Smaldino: Right. I mean, yes. And it helps us when we, when we think this way, it, so, I think basically all science is like this. And I, you know, I make the argument in the chapter that Modeling is a thing that most scientists do, whether or not they're building mathematical computational models or not, right, because we are always, every scientific explanation is coming up with a description of a system that is incomplete, that is a model description of the system, where you take the parts that you think really matter to your explanation and you focus on them, and you allow yourself to sort of ignore, [00:44:00] at least for the moment, Other things that you know are part of the system, but you were going to say, we're going to ignore that for now. And so, you know, behavioral experiments are models because they're of the sorts of scenarios that we're actually interested in, but they're not the real scenarios. They don't have all the components of them. We're saying, well, let's distill it into this. And, you know, models are kinds of models. we say, well, I want to study physiology and I can't cut up a person, but I can, you know, mess with a mouse brain. And the fact that the mouse brain is similar enough to my brain allows me to draw inferences, even though a bunch that's missing or different from the mouse brain. And in the kinds of scenarios, I'm putting the mouse in, in which I'm studying them.
Right? And so. Yeah. When we do, when we're trying to come up with explanations [00:45:00] of social phenomena, of our explanations have these limitations to them. And what the kinds of formal modeling that I try to talk about, or well, that I do talk about at length in the book are way ways that can start looking at different kinds of questions and different kinds of social systems and think, well, here's. Here's an example, or here's a candidate distillation or decomposition of the system into a set of parts. Those parts will have properties. The properties that we care about won't be all the properties those parts really have in reality, but they will be the ones that we think are essential to the relationship or the phenomena. They'll have relationships and behaviors or actions they can take that will be oversimplifications that will be. know, distillations of what we think are important, and then we can use the model to work through the consequences of our assumptions about this limited set [00:46:00] of parts and their properties. And they're always going to be incomplete, but by doing this in an iterated way and exploring various extensions and on different models and using multiple models to understand different aspects of phenomena, we can kind of triangulate to richer and more precise explanations.
Benjamin James Kuper-Smith: Um, could you, I think also in that chapter that like somewhere there you mentioned Newton's model of gravity as one of the kind of classic examples, and I think that's maybe a fitting example for the point you just made. Would you mind kind of expanding a little bit on that and how there, you know, Newton ignores basically everything, but still comes up with a very useful model.
Paul Smaldino: Right. I think I was trying to come up with an example of, uh, when I was. I want to talk about the value of models, you know, you can go back to kind of the roots of modern science, which come out of, you know, the time around [00:47:00] Newton, I mean, people talk about Newton as being sort of one of the first modern scientists, partly because, you know, he was one of the first people to be published in the Journal of Philosophical Transactions, the Royal Society, which is like the first academic journal. But so, yeah, Newton had access to all this data that other people did too, right? That Kepler, uh, presented that Tycho Brahe was, um, had gotten, um, it was all this, you know, at the time, the highest resolution data that anyone had about the motion of the planets and Kepler there. very convincingly to many people, including Newton, that these, uh, the planets seem to go, not only do they orbit the sun, but they orbit the sun in elliptical orbits, not circular orbits. And Kepler came up with some sort of crazy, like, kabbalistic explanation for why that could be. Um,
Benjamin James Kuper-Smith: the cooler explanation.
Paul Smaldino: yeah, I mean, it's very, [00:48:00] like, you know, uh, yarn on tacks on a cork board. But Newton. up with this idea about universal gravitation. And I learned, James Gleick has a great biography on Newton.
And what I remember learning from that book was that Newton wasn't the first person or the only person, at least to come up with this idea of something like an inverse square law. So he said, all right, there's universal gravitation and things are attracted to each other in a way that the force of that attraction will diminish. with the inverse square of the distance. Apparently, Robert Hooke had thought about this idea. Some others, I think, um, uh, Christian Huygens had come up with this idea. But Newton is the first person, and he's also the first person with the mathematical chops to, to work, work out the math. But he writes it down, and he says, all right, well, So let's imagine I've got these, these sort of simple laws of force that I've come up with where, you know, force [00:49:00] equals mass times acceleration, or, you know, something like that. And let's imagine that objects are attracted to each other with a force that's proportional to the product of their masses and inversely proportional to the square of their distance. What happens? Well, what happens is you get elliptical orbits, and actually, depending on the distances and the sort of scaling rules, you can actually get any of the comic sections. So circles, ellipses, hyperbolas, et cetera, um, parabolas, but one of the more stable ones is our ellipses. And so he's like, look, if you assume an inverse square law. You get all the elliptical orbits and. out this is really good at predicting where all the planets are going to be. And even though, you know, so the model is woefully incomplete, right?
Like, [00:50:00] Newton doesn't, he's not building a model of the entire solar system. First of all, they don't even know about, like, half the planets the time, right? I think they knew out to Saturn. And I think that's it. So they know about six planets they don't know any about any of the other moons. I don't think, I mean, maybe, maybe they know Galileo, I think discovered some of the moons of Jupiter, but he's not even building that he's not building a model of six planets. building a model of one planet and one sun. Because that's all you need, right? What he's doing is saying is imagine. One object that's super massive and an object that's way less massive, they're moving near each other. What happens? turns out that the little thing will go around the big thing in an orbit that's elliptical. And so this is a very simple model that includes just one planet and [00:51:00] one, and it's not even like a complete model of the planet, right? He's modeling the planets and the sun as point masses. There's nothing that there's, they're not modeled as spheres that have, you know, other kinds of inertia and angular momentum, et cetera. It turns out this is really effective and, you know, even though it's later replaced because like all models, it's, it's kind of a wrong model. mean, Newton was never able to really explain why why gravity existed, why this universal gravitation was there. He was just like, well, let's just it is. I mean, and a couple centuries later, Einstein comes along.
It's like, what if like the fabric of space time is just, you know, by mass, that would lead to something that looked to our eyes like gravity, which is a pretty cool idea. And it's accurate than Newtonian gravitation, but Newtonian gravitation is so good and the math is way easier than general relativity that, you know, most of like the [00:52:00] space missions use variations on Newtonian mechanics, not Einsteinian gravity, because it's, it's good enough to get, you know, a rocket to move to the moon. So there's, I use this as an example in the book of a very simple kind of wrong model that still gets us a long way. But, you know. a big gap between a model like that and a model of the social sciences, and this gets it, you know, I talk about this when I talk about, uh, inexact versus exact, uh, sciences, I think that this is, this is a distinction that really should be taken as a continuum more than a discrete, you know, categorical thing, where some sciences are more exact and some sciences are less exact and, of physics, string theory accepted, but most of physics is pretty exact.
And by this I mean the relationship between theories and measurements is nearly [00:53:00] one to one. So, you have a model, a theory in physics, you write the theory down as a set of equations, and the terms in those equations have units. The terms in those equations are the measured quantities. The terms of the equations are things like mass, and current, and charge, and flux, things that you can measure. And so, a theory in physics is really about the relationship between measured quantities. Consider the social sciences, where our theories are about things like utility, and happiness, and cooperation, and, you know, inequality. Identity. Oh my God. Identity. Yeah, these things are way harder to measure. You can measure them, but every social scientist and behavioral scientist knows that there is, even if they don't admit it, but most of them will, they [00:54:00] know that there is a chasm between the concept of Any of these concepts as they think of them in their head, as we mean them when we use these terms verbally, and in what we're able to measure. Therefore, we are stuck with this dilemma with our, when we try to model these things. Do we model the things that we try to measure, or do we model the things, the real thing we're trying to explain? And I think this is actually one of the things that The kinds of formal models that I talk about in the book are just really helpful for because of the training in, you know, uh, social behavioral sciences, especially in psychology, but other fields too, is really about the first kind, the sort of physics kind of, uh, uh, the models as relating measurable quantities.
I mean, this is what all our statistical models are. statistical model is taking in the data that we have and relating them to each other and trying to find [00:55:00] regularities in their connections. We get into problems because a lot of these associations that come out of the models don't have real causal, uh, weight. But beyond that, the problem of causality is a huge one and is very big. But also, ultimately, If we have lots of data and we build up a statistical model that's based on that data, what we're doing is finding relationships between measured quantities and not between real conceptual factors that we're really interested in, ultimately interested, and will help us generalize theories beyond just these particular measurements. So instead we can build formal models and into those models we can try to capture more about the essence of what the things we're interested in are do. So, if I build a model of identity, instead of just saying, well, here's my measure of identity, and here's how that measure of [00:56:00] identity relates to my measure of cooperation, can say, well, do I actually think identity is for? And what do I think identity does? And therefore I can build a model in which identity is expressed in that way and think about the predictions that a model of identity expressed in that way will have on other factors like assortment or cooperation or contagion. Uh, it's, it's ironic because I, so identity is actually one of the areas I'm, I'm very active in, in terms of my research.
I work on and I build models of the dynamics and evolution of identity and, and, uh, related factors like signaling and norms. Um, I don't have a lot on identity in the book, partly because I felt like I needed to. Build up a foundation first of some of the precursors for identity. What are, you know, what are the things that identity acts [00:57:00] on? And so the, the book focuses more on, on topics like contagion and opinion dynamics and cooperation and coordination and norms without overly focusing on, you know, and I, I do a lot of, so every chapter in the book, most of the chapters has a, a section in it that's called going deeper. And so every, every chapter basically ends with 2 sec, I, I, I have 3 actually have 3 sections that basically every chapter with some exceptions ends with when it's called reflections when it's called going deeper and when it's called exploration, uh, exploration is, uh, just is a is a series of homework problems.
Basically, it's like here do some things with these models that I didn't show you how to do, but you can use what I showed you explore them more and answer some new questions. Reflections is sort of thoughts on the limitations of the model's assumptions. So we've worked through some models, those [00:58:00] models make assumptions, those assumptions have limits. What are some thoughts, you know, what are some of those limitations and some, what are some, you know, Uh, caveats that we should have before drawing conclusions from these models and then going deeper is trying to be a pointer to work that takes those ideas and goes farther with them and expands on them and builds on them, takes them in new directions. And so, yeah, I mean, it will be a slightly biased, uh, as I think anyone's book will be slightly biased from their perspective. But yeah, so I try to just not just be like, these are the models. You're done, but it's more like these are the models that I felt like I had the time to go over and I think will be good foundations.
I was really focused on foundations with this book. And then here are some pointers for ways in which or places if you're interested in these topics. Here are some different [00:59:00] things that different researchers have done with these ideas and take them in new directions. You should go check them out. But at the heart of this book, I really thought of. If I was training a collaborator, as I, that's basically what I'm doing with my students, especially my graduate students, like. want them to be my collaborators. I want us to work together on research projects, but I need them to know certain things. I need them to have certain skills and be familiar with certain techniques. So, in my building this course up, I was started with a perspective of like, what do I want my collaborators to know as a foundation what, what are the models that I need them to know? What are the techniques I need them to know for modeling? I gave them everything I could think of, this book would have been three times as long. So I tried to distill it as like, well, what are the really essential things from which that will give them enough of a scaffold upon which we can, [01:00:00] we can go a lot farther?
Benjamin James Kuper-Smith: Yeah, I think that's, that's how I also saw it. I mean, I, I should put it. Um, as you said, like in the middle, you have the like scientific contents on the outside. That's kind of a bit more of a philosophy of science and modeling and that kind of stuff. Though the one I read, um, from the contents, one particularly was the corporation one, because that's the one I'm most interested in.
And yeah, it was kind of interesting to see how you kind of. I hope this is accurate representation, but you kind of take, like, the early cooperation research of, like, how do you even model agents interacting with each other in an environment where, um, exploitation, exploiting the other person is often beneficial, like, how can in this kind of environment.
cooperation happen, um, which is, you know, the kind of early research from the Axarods tournament and Martin Novak's early simulations and that kind of stuff. And then you kind of make it a little bit more complex, add a little, you know, what if the, it's not like, well, I don't have to go into the details, but like, yeah, you kind of add complexity step by step and then in the end kind of [01:01:00] have this long list of like, here's some other stuff you could do, just have fun with it.
Paul Smaldino: Yeah. I mean, one of my sort of early entries into this kind of modeling were these models of cooperation. It's, it's a topic that I've, I've worked on for a really long time. Like, My PhD dissertation had four chapters and three of them were about, you know, cooperation models, two of which were about the Prisoner's Dilemma, one was about the public goods game. And so I, you know, this is a topic that I have a real, like, deep love for and affinity for. And I, I, There's clearly there's so much nuance to cooperation and so many factors involved in understanding cooperation in both humans and other animals. But I really felt like, well, we have to start at the basics and we have to understand just let's take ultra, you know, altruistic cooperation is costly, but provides a mutual benefit. So how do we get, how do we get that benefit to be sustainable when, um, [01:02:00] Individuals can exploit cooperators take advantage of them. And I thought, well, what's great. What I love about this chapter in particular is that it provides an opportunity to say, well, look, here's a model. can work through some, some examples of, of, you know, we take the Prisoner's Dilemma game and we'll show a Prisoner's Dilemma game is such where there's a of mutual cooperation, but it's costly. So I pay a cost to help you. If you pay a cost to help me, the benefit is better, is greater than the cost. So we both benefit. if I pay the cost to help you and you don't pay the cost to help me, then I get screwed because I've paid the cost and gotten no benefit, and you've gotten the benefit and not paid a cost, so you do way better. But if then I say, well, screw that, I'm not helping you either, then neither of us get anything. So we're better off both cooperating with each other. Um, then we [01:03:00] are in defecting, but no matter what we do, I'm always better off defecting if we're only gonna play the game once. So, I think that logic, you know, it provides a real opportunity to start exploring different things.
And the way I've chosen to explore this is to put the model on a square lattice, on a grid. imagine, you know, playing the game is this sort of, you know, idea, yeah, very similar to some of the early work by Martin Novak and Robert May and others, and find when you put agents on a grid and you have agents play with their near neighbors on the grid, actually very easy to get cooperation. And the question is, well, what's going on? Why is that? It seemed like cooperation, altruistic cooperation, which is this costly cooperation, seems like that would be very difficult to get, but on the grid, it comes up very easy when neighbors are playing each [01:04:00] other. And you realize, well, it's because the neighbors are playing each other, and the neighbors remain neighbors forever in the game. And so little neighborhoods where there just happen by chance to be more cooperators are all cooperating with each other. Neighborhoods where there just happen to be more defectors are not cooperating with each other, they're doing worse. And so the parts of the grid with more cooperators are doing better because there's a cluster and that cluster can spread. So then I say, well, now what happens if we start messing with the spatial correlations and build up a little bit of complexity say, well, What happens if, uh, we disrupt the spatial order? And I do this by showing you how to randomly swap the locations of agents on the grid at a rate, at a some given rate.
And so if that rate is very low, Like, you know, half a percent, 1 percent of agents swap every [01:05:00] time, then you still get a pretty high amount of cooperation. But as you start increasing that swapping, you disrupt the spatial order. And so the the neighbor you played with at one time is not necessarily the neighbor you play with another time.
Agents are moving around. And what happens is you then start approximating it closer and closer to what Physicists would call a well mixed model where agents are just interacting at random, and when you have that, cooperation cannot sustained. And so I use that insight, the sort of spatial modeling, the visualization of it, to provide this insight.
And playing with this model also provides, you know, some instruction on how to code up these evolutionary simulations. But then I say, well... seems like a very, you know, maybe a general principle, but these models are very bespoke. Like, it's a square grid and agents are only playing with this many neighbors.
And, you know, what is there a more general way to [01:06:00] think about this? And that's when I say, well, it turns out we can use algebra and not simulations. Right? And let's think about, let's use algebra to think about what the expected payoffs to cooperators and defectors are. Okay. And we can have a term that is, what is the rate at which individuals tend to interact with the same type of agent as they are, so cooperators tend to interact with other cooperators, defectors tend to interact with other defectors, above chance, because chance is just the well mixed model, just it's a well mixed model, then the probability that you encounter a cooperator, if you're also a cooperator, is just Well, it's just the frequency of cooperators in the population. you're picking one at random, but imagine there's some mechanism for assortment so that more often than than not, or at least more often than chance, you do get to pick a cooperator. Maybe a mechanism is you're interacting only with close kin. [01:07:00] And so you tend to share genes. And so cooperators have similar genes for cooperation, or maybe there's a spatial structure where you tend to interact You know, locally, or maybe you have a cognitive mechanism by which you can find other, you know, cooperators, or you prefer members of the same type. It doesn't really matter in, in the way this particular framing is done, but we have a term for this assortment and you can show mathematically that if assortment is high enough. Then that's what does it. That's what allows cooperation, or one of the ways in which cooperation can outperform defection is if assortment, uh, is sufficiently high by any number of mechanisms.
And what we end up doing is deriving Hamilton's rules. This is an inequality that was introduced by W. D. Hamilton in the 1960s. Um, but expand on it. And then, you know, talk about some other mechanisms for cooperation. So I worked through another whole example about reciprocity and other kinds [01:08:00] of contention strategies, tit for tat. And we do, we go back to the simulations and say, let's simulate tit for tat and see what happens. And look, tit for tat doesn't need spatial assortment to do well. Tip for tat will do well even when we disrupt the spatial assortment. So now let's prove this. Let's go back to the math and think about how to write down equations that capture what tip for tat is doing and show that The functional form of tit for tat and the of reciprocity looks basically identical to the functional form of Hamilton's rule.
And that's because reciprocity is a kind of assortment. It's not assortment of types, but it's assortment of not isn't about reciprocity. Co operator is only interacting with other co operators, but it's about the benefits of co operation being directed preferentially to other co operators. And so doing this back and forth modeling allows me in that [01:09:00] chapter to kind of show both like the relationship between simulation modeling and mathematical modeling and the relationship between different kinds of mechanisms that can promote cooperation in this context. And it really highlights a point that I try to make throughout the book, which is that agent based modeling and sort of equation based dynamical systems modeling. are not or opposing or competing methods. These are very complimentary methods. And so, I mean, I remember early as a graduate student reading and I was just getting into this kind of modeling reading some paper in a psychology journal that was about, you know, raw raw agent based modeling and it was saying, well, this is why agent based modeling is better than equation based modeling. And over time, I've just come to vehemently disagree with that, that baseline perspective, which is just. are not competing things. Agent based models can give you insights and [01:10:00] allow you to explore heterogeneity in ways that are much more difficult with equations, but equations can give you precision and allow you to get closed form solutions and give you lots of, you know, sort of quick insight when you can do the math. And often, the best approach is to sort of get an insight from an agent based model. Try to distill it down to its essence in an equation based model, take the equation based model as far as you can until you can't do the math anymore, and then simulate more. So it's, it's a really back, it's, it's much more of a back and forth approach than an either or approach. And I try to promote that in the book. I will say, the last thing I'll say, uh, uh, about this is that, I've really written the book for an audience that is interested in modeling and wants to do modeling and wants to learn how to do modeling, but doesn't necessarily have a deep mathematical background.
I've tried to limit the mathematics to algebra and probability theory. You know, I talk a little bit [01:11:00] about calculus and differential equations, but we don't, you don't need it for the book. Similarly, like, the computational models are designed to be simple and replicable by anyone who can code. I also use I use NetLogo in the book because it's free, it's open source, and it's sort of a software package that is very much designed for building agent based models, but I also have a section in the preface I already saw called why this book uses NetLogo, but you don't have to, because I really tried to make the lessons general and generalizable to people who don't use it, um, but I, I, the book comes along with a GitHub repository that has all the code That's in the book.
So you can, you can grab all the code for any of the models I present.
Benjamin James Kuper-Smith: So I had one question about kind of. In some sense, like when a formal model is applicable or when you should use it, when you shouldn't use it, um, and I thought, or maybe even which [01:12:00] person should use it, which person shouldn't use it. And I, I was reminded when I thought of this question of a passage I read in a paper, um, that I think contrasts these two approaches between formal modeling, not formal modeling very well.
Um, so this is from, uh, Sir Carl Fristen wrote and like a kind of, essay kind of thing almost, um, where as lots of people know, he uses lots of maths in his, uh, in his work. Uh, but he did a postdoc with Gerald Edelman who did not appreciate mathematical modelling. And so I thought I'd just read, um, this little abstract because I think it's kind of nice.
Um, so the passage goes, As a student of probability theory and quantum physics at Cambridge, I'd assume that all formal theories should ultimately be cast in mathematical terms. Edelman, on the other hand, considered this as mathematosis. and about as desirable as halitosis. When I pointed out at an early group meeting the formal links between value learning and dynamic programming, there was genuine fear and horror in the room about how Edelman would respond.
His [01:13:00] response was to rusticate me to the library for a period of six months. My task was to sanitize my thinking and immerse myself in the writings of the great biological thinkers from Mayr. And I find this kind of interesting because Gerald Edelman, uh, for those who don't know, won a Nobel Prize in Medicine for Something about antibodies.
I don't know, but, uh, someone who was obviously clearly very successful and then moved to consciousness research afterwards. And I'm just kind of curious to kind of how you, in a sense to kind of comment on that, like when mathematical modeling is appropriate when it isn't. Yeah. Kind of what you make of this kind of clash of ideas.
Paul Smaldino: it's interesting. Um, So, I'm not a purist. I think that you don't always have to build a model. Uh, there are certain questions that are strictly empirical questions. Like, I want to know how many of something are there in the world, right? Or I want to know, you know, what happens if I do such and such?
Or why do people, you know, what do [01:14:00] people do when this happens? And some of those questions are questions that I, require you to actually go out and test things and measure things and look and engage with the world. those questions can often be model driven. You can use models sometimes if you have, you know, a good enough theory to make a prediction about the kinds of things you, uh, might find or to constrain the kinds of things you might be looking for, but you don't always have a good enough theory. Um, and I think that this is really where it gets into the weeds because when we are starting to learn about systems, new systems, new areas of inquiry. Part of our job is just to describe what the hell it is we're trying to talk about and say, all right, what, what, what is there in the world? What is, what is this thing that I'm trying to describe? are [01:15:00] these phenomena? What kinds of phenomena are there? These are not modeling questions. These are, these are empirical questions and they're questions not just about measurement and data, but also about, you know, thinking and debate, uh, because I think one of the main challenges of science in general, but social science in particular is about resolving ambiguity. Language is inherently ambiguous. The words we use, you know, and the sentences we use have multiple possible interpretations. to some extent, this is A feature and not a bug, right? It's very useful for us. There's a great literature in the communications, uh, literature call on and in political science on what's called strategic ambiguity and the use of ambiguity as a strategic device.
Like, for example, you know, if I'm a politician, I can make campaign promises that are very ambiguous and therefore you, you can't say that I didn't live up to the promises because [01:16:00] actually there's many possible interpretations, but also in more quotidian things like building coalitions, if I'm trying to build, get lots of people to sort of go along with me and I'm sort of ambiguous about what our goals are, then different people can sort of map on their own interpretations of that and whatever the favorable light is and it can be consistent. whatever everyone thinks we're trying to do and sometimes that that works really well because it's okay if we're not actually trying to do exactly the same thing. If it's close enough that we can all or get our goals sort of mostly met. When we're doing science, right, we're trying to ultimately reduce ambiguity, because we're trying to build up explanations that replicate, that can be communicated clearly to others, that can translate from one scenario to another, or at least specify why they won't translate from one scenario to another. And therefore, precision is sort of, in some sense, the opposite of ambiguity. As we develop [01:17:00] theories about the way things work, we can become less and less ambiguous as we know what to exclude and what not to exclude. And on the pathway to reduced ambiguity is formal modeling, because when you build a formal model, you have to say the relationship is this. It's not that it's this. You have to say the parts are these. They're not those. They're these. Now, formal models don't entirely remove ambiguity because. The model itself being a model has to map onto the world, and there can be still some sort of residual ambiguity, but in that mapping, like, what actually does this represent? But this is a surmountable problem as then you grapple with, okay, how would you test this kind of model? How will we refine the model to resolve these ambiguities? Once you can identify the areas of ambiguity, you can start trying to hack [01:18:00] away at them. Early on in the development of scientific theories, sometimes I think the maintenance of ambiguity is very valuable, and this might have been what Edelman is talking about, like the sort of neural systems he might have been interested in. are very complicated, very complex. he was probably using, I mean, I know him mostly for his work on Neural Darwinism, which is a terrible name for an interesting idea. But if you look at it, it's very vague. It's a lot of it is very ambiguous. It was this sort of idea of well, neurons are kind of like Darwinian populations and through feedback, you know, synapses that provide a fitness benefit will be selected for and strengthened and, you know, synapses that don't provide a benefit will be, you know, sort of. extinguished and go extinct. And in this way, the [01:19:00] brain learns and organizes in an analogous way to, our Darwinian system. So I think this is what Edelman is probably interested. And, you know, it's possible that someone like Friston might have been very quick to say, let's build a mathematical model and formalize these things without a full appreciation of sufficient nuance Like without a sufficiently nuanced appreciation of the system to build a model correctly.
And you actually see this not that infrequently with people with very strong mathematical training, who sort of jump into modeling systems that they're not deeply familiar with. People, let's say, that come from math or physics or computer science, much great modeling work has come from people trained in physics and math and computer science. But there's also okay, You know, some people in come from those fields with a sort of, say, arrogance that because their mathematical ability allows [01:20:00] them to analyze, build and analyze complex models, the modeling itself, the act of modeling is. Disregarded as, uh, something that is a real challenge. So, okay, we can just model this simply as this kind of system with this, these properties.
That's the model. Okay, now I can use all my fancy math to solve the model. And the problem is, yes, you've solved the model, but the model a good representation of the system that you are trying to understand that allows you to ask the questions about it that you actually want to know? This is something that I think. Uh, I, I've had to really emphasize in my teaching because there's a tendency for people to underestimate difficult it is to design a good model. And in my perspective, this is actually the hardest part of modeling. So when you are getting into modeling, when you're learning to model, it feels overwhelming.
It [01:21:00] can feel overwhelming and very challenging to. You know, do the math to write the computer programs to, you know, run the analyses, run the scripts, know, all the technical stuff feels very difficult when you're first learning or can, Once you develop those skills and you build a competence with how to do these things, becomes not that, not that hard.
And this, I've seen this happen over and over again. And I, and I will say it probably happened with me when I was starting out too, where you learn how to model, you develop your chops, you learn the math, you learn the coding, and then you say, aha, modeling is easy. Now I can model. I can model anything. Let me at them.
Give me all the problems. I will model them. And. You know, one of the things that I've worked really hard to do is continually engage with not just other modelers, but social scientists, cognitive scientists that are not modelers, but have really deep understandings of their study [01:22:00] systems. Because I can present a model and then go and talk to an anthropologist and say like, hey, does this model that I've built capture the relationships that you are interested in or we've been talking about in the system that we're trying to explain and they might say, well. It's, you know, yes and no, uh, this is actually a real, like, here's an important thing that's not in your model or your model assumes this and that assumption doesn't really hold for most of the scenarios that we're interested in. And so. Working hands in hand with, you know, uh, in a collaborative way, modelers and empirical researchers who aren't necessarily modelers, uh, can do a lot more.
I don't think there should be modelers who model and empirical researchers who do empirical research and they never talk to each other and they're sort of off in their own corners, right? These, these people, these groups need to talk to each other because [01:23:00] modeling should be part of all of social and behavioral science, but you also need The deep knowledge of the systems so it's possible that what Edelman was reacting to was a perception that fristin was a little bit and I don't know the system at all.
So this is all
Benjamin James Kuper-Smith: Yeah, actually me neither. Right. This is, it was just a kind of tidbit that he wrote that I thought related. Yeah.
Paul Smaldino: uh, yeah, so it's possible he was reacting to fristin's, let's say, rush to model something before properly understanding the system he was modeling. And that is actually something that I think is a reasonable objection. Okay.
Benjamin James Kuper-Smith: So should one only start learning to model once you understand something?
Paul Smaldino: No. Uh, it's all... No. Because...
Benjamin James Kuper-Smith: Oh, I triggered something.
Paul Smaldino: No. Because ultimately, the answer is because science is [01:24:00] hard. And learning anything requires... Like, getting a deep knowledge of anything... You know, requires learning it at multiple perspectives, multiple scales, multiple levels of organization, multiple different like different analogies, different metaphors, different models, both formal and non formal for different systems.
You learn a ton of facts. You learn different verbal ideas. You learn different modeling techniques. And I don't think that there's 1 correct pathway mastery. I think that you can start as a modeler, and then say, well, you know what, I've gotten pretty good at the math chops, but... I feel like I need to learn more about the systems that I'm trying to model before I can feel confident in the models that I'm doing. And that was, I mean, to me, that was kind of my path. I mastered, I don't know if I mastered, but I got very good at modeling, formal modeling way before I felt very [01:25:00] confident and I'm, I'm always, I always feel a little bit like a dilettante, but, uh, it may be related to the, the breadth of the, of my interests. Before I felt comfortable enough with social science to start asking really deep questions. A lot of my early modeling work is very abstract. It's just saying, look at these models I built and look what happens when I explore them. And look who's the kinds of things that happen. I think that's really interesting it took me a while to build models that were connected enough with empirical research and verbal theories and in social science fields I could say, well, look, now I've got something that's really about human behavior, and we can start taking this to empirical data because it makes some predictions about kinds of associations we should see. So that was, that was very early. That came later for me. But I think there's other people who work really well. They've developed a deep expertise in a field. [01:26:00] then they say, well, you know, my data is sparse. Or I have questions that I, I am not able to answer or I have a theory that I would love to, like explore, you know, whether it works in principle and what the, what those constraints on that theory are.
I think I need to build a model. I need to learn how to do this. There are, there are certain fields where the models are very directly connected with data, where we have both rich theories about the way things work and rich enough data that we can sufficiently calibrate the models. This is a whole other can of worms. You know, uh, certain kinds, areas of epidemiology are really good examples where the theory is both strong enough and the data sets are rich enough that you can build models that can even make predictions about things like, you know, interventions, urban dynamics, urban planning is another area where the sort of mapping between the models and, uh, And the data is [01:27:00] strong enough and the theory is strong enough that you can use these to be sort of fairly precisely map, uh, what's going to happen using a model. in most of the social sciences, it's a bit more abstract and we're saying like, well, when this kind of scenario occurs, we should see more of this kind of behavior. this kind of constraint will lead to these kinds of behavioral outcomes if incentivized. And I think. Considering how much of the social sciences and behavioral sciences are, have results that are basically like, our hypothesis is that A is different than B, then I think we're doing fine.
Benjamin James Kuper-Smith: Okay. As some of this might know, I have some recurring questions now, for the past five or six episodes or so. First one is, uh, what's a book or paper that you think more people should read? This could be a hidden gem, something that's been forgotten, something that was never read in the first place, but you somehow found it.
Or something that's super [01:28:00] famous, and... It's so relevant that people should read it again.
Paul Smaldino: I love so many, there's so many papers and books that have been influential to me. think I will say though, the one I mentioned earlier, Stuart Kaufman's paper, I found the title, Articulation of Parts Explanation in Biology and the Rational Search for Them. Like I said, terrible title, but amazing paper, um, published in, uh, the proceedings of the biennial meeting of the Philosophy of Science Association.
This is the conference proceedings that eventually became the journal Philosophy of Science. This is, uh, published in 1970. And I think this paper is so cool because... What it really talks about is the process that is, I think, underappreciated, which is hypothesis generation. So, so much of science education is about, how do we test hypotheses? what Kaufman is focused on in this paper is, where do these hypotheses come from? He talks a lot about the relationship [01:29:00] between You know, hypotheses and modeling, and he actually says, you know, once when we, we build a hypothesis, what we have to do is decompose the system into parts and their relations and come up with some idea about how these fit together and then, and again, this is 1970, he says, and then we can build cybernetic models of these things explore their consequences.
So, I love that. Uh, relatedly, yeah. You know, on the philosophy tip, I mean, anything by Bill Wimsatt, by William C. Wimsatt is, uh, just truly excellent. There's a really, uh, amazing collection of his Thank Papers in a book called philosophy for rational beings, uh, that I can't recommend enough. Uh, there's a paper in particular, a particular relevance to this stuff, which is called, um, false models as means to truer theories, uh, from 1987, where he, he [01:30:00] talks about sort of. the uses, uh, that false models, the models that are not accurate representations of reality can still get us, and he uses a lot of evolutionary biology as his examples, but, uh, it's, it's a really tremendous paper. Uh, I, I would also, I, I'm, I realize I'm answering your question with multiple things, but, you know, I think that there's, there's so much really, there, there are some really cool. Books about sort of early attempts to use mathematical and computational modeling to understand historical dynamics and cultural evolution that I think should be viewed as as real classics. The most I'll just name 2. The 1 is. Boyden Richardson, 1985, Culture and the Evolutionary Process, where they sort of work through mathematical models, trying to think about, like, not only do humans have these two transmission engines, both genetic transmission and cultural [01:31:00] transmission that shape our traits in this interacting ways, but the way that we transmit things Culturally, socially through learning and teaching that's based on an evolved psychology.
So how would natural selection a psychology that provided for certain kinds of social learning and social transmission and what kinds of these, what, which of these strategies would be adaptive in different kinds of scenarios. It's a really tremendous book that is widely cited and not read enough. Relatedly, I, he just, he's been doing in the news lately. His recent book is very good, but Peter Turchin wrote this book in 2003 called Historical Dynamics, which. Is again using sort of dynamical systems models and some some agent based models as well to think about patterns on the rise and fall of empires and how sort of civilizations come up and go down and what kinds of patterns [01:32:00] lead to societies getting bigger and then dissolving. And he, he does this really interesting thing where he kind of formalizes ideas that were developed all the way back in, like, the 14th century by Ibn Khaldun, who's this medieval Arab scholar, and he built these really cool models. I, you know, in an early exercise, when I was, you know, reading the book, I went and I, I replicated, uh, the agent based model that he describes in that book, and it was really cool to watch these little empires on my screen, you know, emerge and, and go away. Uh, and, and he's really taken this sort of field of modeling historical dynamics very far in the last couple decades, so I'm very inspired by that, that work.
Benjamin James Kuper-Smith: Yeah, the second question is anything you wish you'd learned sooner? Uh, this can be from your professional life, your personal life, just something that, Oh, some, some big life lesson you'd maybe like to share
Paul Smaldino: Well,
Benjamin James Kuper-Smith: or a small one. I don't know.[01:33:00]
Paul Smaldino: yeah, I, you know, my path is really winding one, and, I don't know if it's possible say there's there's one lesson that I wish I'd known earlier because I was sort of the winding path that got me to where I am, I will say that it took me a really long time to kind of discover. The means to even articulate the questions that I wanted to ask about the world, I think it's because education and and even just, you know, the way media and other kinds of things in society are clumped. Reflect a very antiquated disciplinary view where we have psychology over here, and these are the questions that psychology asks, and we have history over here. These are the questions history asks. You have anthropology over here. We have biology over there, physics over there, and then these are the sets of questions that they ask we are now in a [01:34:00] state of the world where are able to ask much richer questions, more nuanced questions that Are not psychology questions or history questions or anthropology questions.
There are human questions. They're questions about the nature of humanity and directions that you know humanity is going and how we got to where we are. And this work doesn't fit neatly into any of our traditional disciplines, which means that the only people who. Are asking questions, or most of the people who are asking questions and working in these areas are people that have meandered through multiple disciplines and found their way together to put these things. And by that time, they're old and it's late in their careers. how much more could you get? Uh, and how much, how much faster could we progress if we [01:35:00] can get these things into the hands of students earlier to get people thinking about these questions and these topics and these techniques earlier? So it's more like I, I, wish that, you know, we could restructure educational systems with an idea, you know, with an aim toward actually understanding human nature and human behavior. That draws on all the things we've learned over the last few decades, because if you talk to somebody in university now, it's, it's as if none of this work has ever happened because they've never been exposed to it. I think that if you expose people to this work, not until they are starting a master's program, that's Thank you.
Thanks. That's slightly tragic, not just for research, but also just like for society, because we have people who are in positions of power and leadership who can't draw on all the lessons that we've learned, and I think that's really too bad. So yes, my lesson [01:36:00] is, I guess my lesson is, uh, we need to redo everything.
Benjamin James Kuper-Smith: Nice.
Paul Smaldino: down. Start over.
Benjamin James Kuper-Smith: It's a nice subtle take there. Um, yeah, no, I mean, I'd love to like comment on things, but I don't want to take up more of your time. So I'll just go to the next, to the last question now. Um, yeah, so I'm, I'm finishing my PhD. I handed in my PhD two weeks ago and I'll start a postdoc in a few months soon. Uh, any advice for maybe me or someone?
In a situation like mine, I guess many people like that are listening to this podcast. Thank you.
Paul Smaldino: uh, well done on finishing. Oh, man. I don't know. I... It's a weird world we're in now. And, uh, I don't know if I have general advice for people in general. And for you, I would just say... Try to figure out the things you want to be doing, and the activities you want to be engaged [01:37:00] in, and the kinds of people that you want to spend your time working with, and try to maneuver yourself so that's the situation that you find yourself in as much as possible. And if that's in academia, great, and if it's not in academia, also great. It's a wide world, and uh, you know. Do, do the things that, that feel, uh, enriching to your life.
Benjamin James Kuper-Smith: Okay, I'll try.
Paul Smaldino: You know, there's a very hippy dippy answer right there, but, uh, it's,
Benjamin James Kuper-Smith: Yeah, but I mean, it is difficult to give specific advice to a population or even someone you don't know, right? I mean...
Paul Smaldino: I think that's the thing, right? Like, uh, what's your postdoc on is
Benjamin James Kuper-Smith: Uh, it's, it's going to be roughly, uh, I mean, so it's not a completely settled yet, so I don't want to be too specific, but it's, uh, let's say neuroeconomics, that's maybe the easiest way. Um, some [01:38:00] neuroeconomics with, uh, fMRI and brain simulation in humans.
Paul Smaldino: Yeah. All right. So neuroeconomics, great topic. Um, My specific advice for neuroeconomics, I don't know if this is gonna make the podcast, but it doesn't
Benjamin James Kuper-Smith: If you want, I mean, if you want to, I'll leave it in.
Paul Smaldino: advice for neuroeconomics would just be, you know, don't forget that the neuroscience ultimately corresponds to behavior. so the, you know, I'll tell you an anecdote. I got super into neuroeconomics when I, when it was new and it was when I was a master's student. So this would have been about 2006.
Benjamin James Kuper-Smith: That's a good time to get into it. Yeah.
Paul Smaldino: yeah, and I, I read, uh, Paul Glimcher wrote a book called like Decisions, Uncertainty in the Brain, the New Science of Neuroeconomics. And I read this book and I was super into it. And there were all these, there were all these studies that he reported about things like, [01:39:00] oh, well, there's optimal sorting between two options. That, uh, when, you know, one option is a lot of resource and one option is less resource, you don't want everyone even to the big resource because they'll run out. So this percentage have to go to this place. And we found neural correlates where the, you know, when animals were, a monkey was exposed to this task, like the neurons fired at a rate that was calibrated to the optimal, you know, Propensity to go one way versus the other, something like that. I thought, wow, this is amazing they did this study. And I went to one of my professors the great Marcel Kinsborn and I, and I showed him this study and I was like, isn't this incredible? And he leans back in his chair and I'll never forget this. He leans back in his chair and he says, well, it's a very nice, elegantly done [01:40:00] study. But we already knew that animals behave this way, right? And I said, yeah. He said, and we know that the brain controls behavior, right? And I said, yes. He said, so there's going to be a neural correlate of a behavior that we know happens. So the fact of its discovery is not in itself surprising. There was a, there was a big moment for me.
I mean, we learn a lot by knowing, like, let's say, for certain things, like, it's useful to know, like, where, in the brain, certain kinds of processing is happening or, you know, how it's connected to other things. But, um, There's often a tendency to just get very excited by neural correlates of behavior in their own right.
And, and I think that, like, I'm increasingly, like, it's important work. It's valuable to know these things, but [01:41:00] I think of it as more like, yes, that is some good accrual of data points science, um, and is, is how, how it should be treated,
Benjamin James Kuper-Smith: Yeah, no, I agree with you. I think this is like part of the Let's say one thing. I'm always very hesitant about is this kind of like I'm not particularly interested in Yeah, spending years and saying oh, it's it's that area that does that thing because that by itself Like I don't care about which area the brain does what in and of itself
Paul Smaldino: Yeah, I mean, I think you gotta, you gotta find out for yourself, what are the questions you actually want to spend your time answering? Spend your time trying to answer? Like, write them down. down the questions that, you know, and be ambitious. What are the questions that you want to know the answers to that you don't feel like you have a good enough current understanding of? And, [01:42:00] You don't have to pursue those things directly, but you can, you can shape your career, hopefully, in a way that everything, obliquely, moves you closer to answering the questions or a better understanding of the questions that you're trying to answer. Maybe, maybe that's the better answer to your question.
Benjamin James Kuper-Smith: Yeah, no, it's funny that you, you specifically mentioned the word obliquely because I had a quote that I didn't get to mention from a book called Obliquity by John Kay. Um, so it's funny that I almost actually quoted from a book that, or you, you said something that's very closer to that. Oh, well, his, his, uh, The, the, the book is called Obliquity and it's, uh, it's kind of interesting, but also a bit weird, but he had a, a quote on abstraction and, uh oh yeah, quote.
Just abstraction is a process of turning complex problems we cannot completely describe into simpler ones [01:43:00] that we think we can solve, but gorging, which simplification is appropriate requires judgment and experience. Our simplifications are idiosyncratic and subjective, and I thought I could maybe mention that into one part, but we never.
Paul Smaldino: I should read this book because I, the general, I just read like a quick synopsis and I'm like, yeah, I totally agree. Everything that I do, when I say like, you know, I tell people like what they say, what's what are you interested in most? And I'm like, these days I'm interested in identity the most and they're like, well, that's not what you work on the most.
And I'm like, but it is because I think to understand identity, you have to understand cooperation and you have to understand coordination and conflict and norms and group structure and identity. Like, this is what drives me crazy about the way that let's say, like, psychology is often organized. Like, you're like, yes, I study.
Yeah. Yeah. Uh, personality with a, an emphasis on neuroticism. And I'm like, Jesus Christ. Like, [01:44:00] I think that's insane. Uh, like, that should be. Like to only study that feels like, but, but you know, that that's like, even if that's your main question, like, you know, that that's linked to other things, right? what is our cognition for? It's largely for social behavior. The distinctions between the areas of research. Is my who don't talk to each other like personality psychology only publishes and personality journals and they read only that stuff and all like it's crazy to me this is why it's related to what I was saying before the way that disciplines are structured now reflects this sort of. Ancient medieval and almost, you know, understanding the sort of turn of the 20th century level of understanding of human behavior at the turn of the 20th century. We didn't know anything about human behavior and psychology and sociology, anthropology, economics. These are all new [01:45:00] fields and we're still learning things. But now our understanding what our potential for understanding human behavior is so much greater, but it requires. Transcription by CastingWords Breaking down these barriers between disciplines and I'm not saying we should get rid of all disciplines, but I think that the disciplines that emerge will look different than those disciplines because it needs to be in terms of, okay, how can we best organize our current study of behavior?
It's definitely not by putting the economist over here and the psychologist over there and the sociologist over there and the anthropologist over there, It's, yeah. There's so much overlap in the questions that these people are answering. It's like the parable of the five blind men trying to describe an elephant, except that's all of academia and, you know, one of the blind men is an economist and one's a psychologist, right?
And they're like, what is this thing called human behavior? I don't know. So, yeah, burn it all down, [01:46:00] build it up again from scratch.
Benjamin James Kuper-Smith: Okay, that's a good final word.