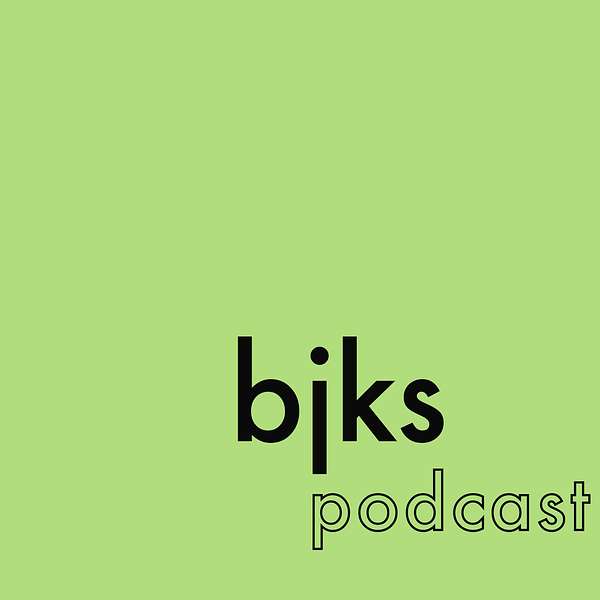
BJKS Podcast
A podcast about neuroscience, psychology, and anything vaguely related. Long-form interviews with people whose work I find interesting.
BJKS Podcast
66. Rafael Pérez y Pérez: Story Machines, Creative AI, and Mexian serenades
Rafael Pérez y Pérez is a professor at the Universidad Autónoma Metropolitana, Cuajimalpa, where he studies computational creativity, in particular in relation to computer programs that can write stories. In this conversation, we talk about MEXICA, the story generator he has been working on for most of his career, his newly released book Story Machines (with Mike Sharples), the advantages and disadvantages of different approaches to creating stories with AI, what the future holds, whether large companies like Amazon are working on these topics, and much more.
BJKS Podcast is a podcast about neuroscience, psychology, and anything vaguely related, hosted by Benjamin James Kuper-Smith. You can find the podcast on all podcasting platforms (e.g., Spotify, Apple/Google Podcasts, etc.).
Support the show: https://www.patreon.com/bjks_podcast
Timestamps
00:05: How Rafael ended up doing his PhD on artificial creativity in Sussex
07:00: Why did Rafael create MEXICA? / A more human system for generating stories
24:45: Many approaches of generating stories
30:46: Is a combination of symbolic and connectionist approaches (neuro-symbolic AI) the solution to creating machines that write stories?
33:23: Why might GPT-3 not work for stories or The risk of singing a Mexican sereneade to a Norwegian
43:38: Are there fundamental barries for AI writing convincing fiction without actually living in the real world?
47:54: Is Amazon developing AI to write fiction?
53:59: What will happen in the next 5-10 years of AI writing stories?
Podcast links
- Website: https://geni.us/bjks-pod
- Twitter: https://geni.us/bjks-pod-twt
Rafael's links
- Website: https://geni.us/perez-web
- Google Scholar: https://geni.us/perez-scholar
- Twitter: https://geni.us/perez-twt
Ben's links
- Website: https://geni.us/bjks-web
- Google Scholar: https://geni.us/bjks-scholar
- Twitter: https://geni.us/bjks-twt
References and links
Chat GPT: https://openai.com/blog/chatgpt/
Mnih, Kavukcuoglu, Silver, ... & Hassabis (2015). Human-level control through deep reinforcement learning. Nature.
Mueller (1990). Daydreaming in humans and machines: a computer model of the stream of thought. Intellect Books.
Pérez y Pérez & Sharples (2004). Three computer-based models of storytelling: BRUTUS, MINSTREL and MEXICA. Knowledge-based systems.
Propp (1968). Morphology of the Folktale. University of Texas Press.
Sharples & Pérez y Pérez (2022). Story Machines: How Computers Have Become Creative Writers. Routledge.
Sharples & Pérez y Pérez (2023). Introduction to narrative generators. Oxford University Press
Turner (1993). MINSTREL: A computer model of creativity and storytelling, PhD Dissertation, University of California LA.
[This is an automated transcript that contains many errors]
Benjamin James Kuper-Smith: [00:00:00] Yeah. Um, yeah, so I mean, I guess what we were talking mainly about, um, your, and I mean your book Story Machines, which I have here as you can see, lots of notes, um, and that you wrote together with Mike Sharples. I thought maybe to kind of start talking about that and get the ball rolling, I thought maybe I'd ask kind of how you got into this and maybe, maybe we can start with like, what is the School of Cognitive and Computing Sciences at the University of Sussex and how did you end up there?
I mean, you are from, from Mexico originally, so how did you end up in Sussex to your masters?
Rafael Perez y Perez: Yes. Well, in Mexico you studied electronics and computers and when I finished my undergraduate studies I wanted to, to go to study a postgraduate course to some place. And I found Sussex and I really like it because he was, um, they. With multidisciplinary teams. And for me that was [00:01:00] a key aspect. I, I think it's extremely important to work in an interdisciplinary environment.
So I thought it was a good option. So I went there to study my, my master in knowledge by systems. Uh, one day, uh, we got an email from one of the young proce professors there asking if someone want to write his dissertation for the masters about computers and creativity. And I was like, wow. What computers and creativity, what is this?
For me, creativity, all my life has been very, very important. It's a, creativity is an important word that I have heard my entire life. So of course I answered that, that nail. I ended doing my dissertation about, uh, using a neural network to evaluate some. Basic music. Uh, so I, I fell in love with that. I, I really, really like it.
So I went to talk to that [00:02:00] professor and told him that I would like to study a PhD because about computers and creativity. So we were discussing, he agreed, and that professor was Mike Chalis. start to work together and we, we, we got along very, very well. We had a very good relationship. So after I finish my PhD, uh, we still in contact and we decided around 2001 that we want to write a book together about narrative pages.
So the book was to be called Creativity in Computers, computer Based Storytellers. That was the, the title. We thought it was, uh, going to be around 100 hundred 50 pages and we thought we were going to be able to finish it. On May 31st, 2 0 0 2. So different things happened and we were not able to, to, [00:03:00] to do this thing.
We had to wait 20 years to achieve this goal. 20. But, um, three years later, we, we wrote together a paper, uh, where we compare, uh, three computer models, Mashika, my storage generator, uh, Brutus and Tre. And that, that paper was important because somehow give us a good idea how the boot could be, you know, how we could develop this further into, into a, a book.
But while time passed, and we have to wait until 2017, Mike was in Mexico City in those days. He was here at my place and we were talking and we start again to talk about the importance. Of writing this book. So we agree that when we finish, as soon as we finish our current business, our commitments, uh, we will start to seriously talk again about doing this book.
So [00:04:00] in September, 2019, we were in London. I was, uh, at Mike's house. Um, finally we start to seriously talk about this project. The project, uh, the idea of the project was to write a book that allow people who were not experts to understand better how all these new technologies, particular narrative generators work.
Because I think it's very, very important for our society to be informed about this, these, uh, developments because they are having a really, really strong. Effort in society and there are a lot of misinformation around a lot. So we thought it was very important. So we agreed to write two books. One Story Machines is the one who is more general.
It describe past, present, and future of narrative generators and [00:05:00] gives a global view of the of the field. And the second book, who is going to be published in few months in July by Oxford University Press. It describes in much more detail how each of these systems or some of the most important systems for narrative generate, uh, generation works.
Um, The second book also is intended for people who not are experts in computer science, but of course they need to have some knowledge. Yes. I mean, it is not, you have to have some background, but not, you don't have to be an expert in, in computer science. So that's how this, that's the discussion we had that that day in, in London, in, in 20 ni 19.
So we agree in working this, in this project, these two books, and we start to, to, to work in the, in, in, in, in the project. And, and what finally we have finished it, both of them and we are very, very happy. And, uh, the first to, [00:06:00] to be, uh, published was story metrics.
Benjamin James Kuper-Smith: Yeah, so maybe to, to make it more specific. I mean, the book is very much about, at least that's the way I look at it. It's very much about. There's kind of, it seems to me there's a goal to try and create machines or like, you know, computer programs, ai, whatever you wanna call it, that can write stories, um, you know, maybe even entire novels that people enjoy reading and that kind of stuff.
And it's, um, yeah, and as I said, you yeah, you just grab kind of like how it started the early days and what's happening today and yeah, it's funny that we're talking right now, I guess it's a bit of a coincidence, but I mean, in the last few weeks it seems like chat g p t has really become quite famous in the news and it all kind of, this kind of things because it can, you know, produce very well text that seems quite human in that sense and to, to go back.
So it seems like to me, by the way, [00:07:00] one question, you call it mek Mashika, but sound not a
Rafael Perez y Perez: No, it's Exactly. S h like
Benjamin James Kuper-Smith: And the country, you say the same way or.
Rafael Perez y Perez: in Spanish we say Mexico. Mexico. But the, that sound doesn't exist in Spanish. So that's the reason. The Spanish, when they arrive to Mexico, they use this, uh, x no to, to, to represent that sound because it's not a sound we use in Spanish. So that's the reasons with X.
Benjamin James Kuper-Smith: Okay. So it seems to me that that project then started very early on that since you started working on creativity and computers. Yeah. Maybe to provide a bit of the history of kind of these things, like when you started, um, working on
Rafael Perez y Perez: Mm-hmm. Perfect. Very.
Benjamin James Kuper-Smith: There you go. Um, kind of what were you trying to do with it that hadn't already been done?
Kind of what was the, [00:08:00] the new aspect that you were trying to add to existing program?
Rafael Perez y Perez: When I started my PhD, there were some interesting programs around.
Benjamin James Kuper-Smith: So this was like 2000, when was this? 2000, 1990.
Rafael Perez y Perez: I first, I studied my master from 92 to 93, and I started the, the PhD in 1993. So there were some programs around two that I really, really like. One is called Minstrel Program, writes shorty stories about the King Arthur on the Knight of the Round Table. And the other that I really like is called Daydreamer.
It, it simulates a woman who has, is having daydreamers about daydreaming, about uh, having, she's in love with Harrison for, so she, she starts to manage the things that she could do with Horizon for. So all those are, are the narrative. And those programs, all those and others, were based in a technique that is called [00:09:00] problem solving.
That basically the idea is that you have initial goal, you have a final goal, you have a set of operators or actions that your characters can perform in order to move from the initial estate to the goal estate, to the final estate. That's the essence. And they did very, very interesting things with that.
But that technique didn't, um, I, I, I felt that it was necessary to explore other options because when we write, it's true that sometimes we have goals, clear goals, that we want to achieve that, but some other times that's not the case. Some other times, idea just came. Yes. And we just start to write. I, I, I read a lot about how writers write, how they.
what they say about writing? No. How, what writers say about writing, and some of them describe this, these ideas that, for example, they don't know [00:10:00] what is going to happen, no. That the story just develops, and that kind of stuff is not represented in in problem solving because as I mentioned, you have a final goal.
You have clearly the goals you want to achieve. So what I wanted was to develop a system that was not based exclusively in problem solving. I wanted to generate something different that represent that process where we just start to generate ideas without a specific goal. All this was shaped by my conversation with my s because.
He in those days was finishing of developing this, um, cognitive model of creative writing. Uh, and, uh, his ideas feel very well with this concern I had because he says that writing is a process that has two estate. One is call engagement. [00:11:00] The, the other is called reflection. The typical example of engagement is when we're, we are daydreaming ideas just flow.
We don't have control of how these ideas arrive. And the other state reflection is a very analytic, very much problem solving oriented process. Yes. So the combination of both is what produces the creative processing humans. Of course these ideas are very old. No, they have a long history, but my was able to collect all them and put them together and, um, incorporate new elements to all these ideas and make something really coherent.
So inspired by all these ideas, I use it as a framework to build mahik, especially because this engagement state was like something for me. Like, yes, no, we need to represent something different. No, no, that, that sounded to me more like the way we humans work, not more like that problem, mechanical problem solving approach.[00:12:00]
So that was my goal to try to produce something different. So I started, uh, I started to, to think a lot about that, not because this, um, framework, not the engagement, reflection, uh, community account of creative writing is very interesting, but it's very general. No, it's a, a very good framework, but , there are a lot of gaps there that you need to fulfill in order to build a computer program.
So, Um, there are, uh, many things I can describe about that, but we can, we will need like seven, several weeks to go into too many details. So I will just say that one of the things I did is to think how we can represent disengagement process, this automatic process where the system just starts to associate ideas without using gold.
How we can do that. And my solution was to represent the emotional relations and cl and conflicts [00:13:00] between characters as a way to associate ideas. And for me, that's one of the main contributions of the system. So in that way, we, I was able to generate sequence of more or less coherent uh, ideas and then reflection.
Goes into the stage to fix all the gaps there, no, all the gaps that happen during the generation process. And then we have a coherent story. And that was something important because before all programs at the end use some kind of predefine it, um, structure to be sure that the output was going to be coherent.
And Mashika didn't use that. No meshika. The, the story just emerged as part of all this process I, I'm described. And I think that was, uh, something very important, something very different to what happened before. [00:14:00] Uh, so that was like the main motivation, not to, to do about doing something different to what was in that moment because problem solving has had a really, really huge influence in the development of, um, automatically storytelling.
And I think, well, I think I achieved the goal. So, uh, I'm, I was very happy with the, with the results. And of course during all this year I have been working in improving and improving and improving the system because it's, uh, endless. No, this is an endless process. We know so few about how the creative process work, that always, there are lots of opportunities and ideas there, but that's how did.
Benjamin James Kuper-Smith: Mm-hmm. , it seems to me that, I mean, so you mentioned that. The early versions often had a very problem solving kind of approach to writing fiction or short stories usually. And in the book you also mentioned lots that take, I can't remember which is which anymore, [00:15:00] but you know, they take like lots of fox stories or um, or different kinds of stories and then kind of try and get the essence out of them and then try and retell a story in that style.
Am I correct then in understanding that, uh, Mashika then tries to it? It's, I mean, one thing that sets it apart is that potentially the stories are more creative than if you just be, you know, if you just kind of retell stories that you put into the system. Or do you still kind of start with that large base of like, you feed lots of stories into the system and then from there it learns to retell stories.
Rafael Perez y Perez: Let me first answer this last part. The idea of Maka is to represent some of the ways we work in order to generate a story that means, That Meshika needs some knowledge. I mean, we are able to know stories because we have [00:16:00] experience, we have lived, we have knowledge. Yes. So MEA is not part of the MESHIKA model to represent how we get that knowledge.
So we assume, or I assume that already has that knowledge. So to do that, I provide some information to the system and the main idea of that information, that Mashika is able to register how we people act on their specific context that are described in terms of the emotional links and conflicts between characters.
For example, from these stories I provide to Mashika Meshika register that when someone is in love, what is something logical to do, for example? Yes. Because that, that, by the way, is very social. No, it depends. In, depends a lot. It is not the same how people behave when are in love in, in the uk, in Germany, or in Mexico.
No, it's, it's very, very [00:17:00] different. No, um, when my father was young, he and his mates used to go to seren eight girls around the, around the, the city, you know, with the guitars and play, you know, the romantic song, something like that. I have never seen something like that. I don't know if it happened, but I at least I have never seen that, something like that in.
In, in the UK where I live five years, so this is very social, which is very interesting. So I provided stories not to provide words, I don't work with words, but to provide information that the system can then recollect and use it to know between quotation, how to continue given a specific context in terms of this emotional conflict.
That's the kind of information I, I provide and as I explained before, because Meshika, uh, um, is assumed that Meshika already has that knowledge. It's not just a newborn. Yes. So yes, I, I, I am, [00:18:00] I use that information to generate, is that more creative, that other techniques? Oof. No, that's a, um, it's a complicated question because we don't have really a, a universal definition of creativity.
I mean, the best we have is that creativity is something that is new and is novel or interesting. No, something new, something novel and something interesting or useful. That's the more or less the general definition, but that even that has some problems. No, uh, what is novel is not that simple. So, um, I wouldn't at all say that one system is more creative than the other, but definitely the, these different approaches, not the approach that msika follows.
The approach that problem solving follows the programs based on problem solving. Follows and the new techniques based on deep neural networks, they contribute in completely different ways. They provide different [00:19:00] information, different perspectives, and at the end, all that are important because as I said, we don't understand creativity, we don't understand how we work, so we need as much information as possible to start to figure out things.
Benjamin James Kuper-Smith: Yeah, I mean you, for example, you uh, sent me two stories, although I think there's also one story is in story machines. You reproduced it. I mean, it would be too long to read the entire story here now, but I thought there's, I mean, so what's interesting to me is about these stories that, um, Meshika produces, is that it's very much, it reads a little bit like almost a synopsis of the, like an action point list almost, right?
I mean, for example, this one story I have in front of me, which is story 18, for example. I mean like, yeah, just to read like a little bit of it unexpectedly, the lady saw the princess had the sacred knife that was stolen from the temple, so there was no doubt she was the murderer of the odd priest. The princess [00:20:00] produced in the lady conflicting feelings.
You know, this kind of like description of what the feelings are is of course very different from what you might read in a novel. And so I'm curious, like how would you, you know, to make that an engaging story? You wouldn't usually just. Describe the emotion in this like, very, uh, direct way. Uh, but you might try and get it more indirectly.
So I'm curious like what's, what's missing from the, like, how can you add that basically to the program to take it kind of to the next level
Rafael Perez y Perez: Um, let me explain something, two things. One is that Meshika, what really is, is a plot generator. It's what it is. It's not a language model. So the goal of meshika is not to produce nice language, uh, no stories will not link nice language, but to produce seconds of coherent, interesting, and novel eh [00:21:00] ideas, sequence of action.
That's the main goal of Maik. And eh, that's because Maik is a research tool. To try to under understand better how we work to pro, to be able to produce that sequence of interesting, novel and coherent ideas. That's what I'm really interested. So in this case, one thing that I really like is that Msika has some knowledge about the emotional status of the characters.
And that's the reason I thought it was interesting that in some specific points, the system make a reference to that emotional status of the characters. And that's the reason That phrase that you read is there No, in that moment now it would be better to describe it in another way. Yes. And it's very simple in Mashika because [00:22:00] Mashika use templates to generate the final output.
Because as I just mentioned, it's not a language model, so it use this basic templates and then you can put elaborate ways to describe things. Yes. In very, very different ways. For me, the important thing is that to recognize this emotional situation and do something about that either included in the story or they sudden done to include it wherever.
But that for me is like the core of, of, of my goals. No, it's like how, how this happen, how we humans realize about that, how we can using this information, make something to happen in this story. That's the kind of things Magicka is trying to represent. That's the reason this phrase is like that. It's simple because if I use very elaborated phrases somehow, I don't want people think that that was [00:23:00] produced by, by Maka, because Maka is not, it's called no.
So, but it's simple to do it. It's not a, a big problem. Not to put more, something more, eh, no, the, the princess, the heart of the princess was beaten fast because she knew something was wrong here, for example, I don't know, I'm just saying a word, uh, phrase.
Benjamin James Kuper-Smith: Okay. But that's interesting. Yeah, because I then I slightly, uh, um, misunderstood the point of msika because I thought it was an attempt to write stories rather than, I mean, it sounds to me almost as if you are using, as, if you're trying to understand the human mind by modeling part of the creative
Rafael Perez y Perez: the goal. Exactly. That's the goal. No, trying to contribute to the under understanding of how our mind goes when we produce short stories. That's the idea. So I, we have these [00:24:00] ideas, the engagement, reflection plus all the other things like the emotional relation between, so that's the kind of knowledge we need to make sense of the world.
Make sense of the world. So for example, based on machi, I'm claiming that, eh, this use of emotions to represent relationships are essential to be able to make sense of the world. So that's the kind of stuff I I I'm trying to do. That's the goal of Machi.
Benjamin James Kuper-Smith: Hmm. Okay. Okay. That really changes my, yeah, . It's funny, like when you think something is has, you know, this particular purpose, but it actually has a very different
Rafael Perez y Perez: and that's, that's important. I'm happy we're talking about that because one of the themes, I'm a little bit concerned with all these, um, cover in the media about programs like, uh, chat gpt and before G PT three and all that, that people start to think that that's the only kind of [00:25:00] models that exist for narrative generation and their goals are the only goals.
That people follow when they are working in narrative generation. And that's terrible mistake in my opinion. And I think it's very important that society is aware about that. So in the story machines, in the book, we explain different ways that have different goals to produce narrative. Of course, we include G P T three, we include an explanation, but we also describe the engagement reflection model and a mahik.
And we also describe the problem solving programs like the one I was mentioning earlier and minstrel, um, the Dreamer. And also we describe other tech, uh, techniques like, like story grammars, not based in the work of Vladimir Prep, not about the the Russian folk stories. So it's important that people understand that no, there are different goals, [00:26:00] different perspectives, different ways to generate that.
Not only the one who have a lot of money to make a lot of publicity about their own systems. We are other also there who have been working for many, many years doing this kind of stuff.
Benjamin James Kuper-Smith: You know, that was really the, I mean, not necessarily the most interesting aspect, but the, the, the one that surprised me most, let's put it that way, about the book was that, um, I studied psychology and neuroscience, let's say in the last 10 years. And, Through neuroscience. I mean, I was, I also did my masters at UCL where, you know, de Harbor with Deep Mind and a lot of the people from Deep Mind, uh, came from and still have lots of connections to that.
So that's kind of what I always, um, what, I mean, you could almost say like what I grew up with as the idea of what artificial intelligence is. And of course they've, I, they've done lots of great things and made lots of advances, but, so when I thought about, you know, machines that write stories and these kind of things, I [00:27:00] thought that was like, yeah, as you said, like the only way that you can do that.
And when I opened your work, I was like, ah, there's all these other approaches that have been going back for decades or I mean, sometimes centuries, right? These like old mechanical machines that people use to create Latin poetry or whatever. Yeah, it was really interesting to me to see like, oh yeah, this is, uh, I, and I guess as you alluded to, most of the people have been missing out on kind of the other half and.
Potentially also an equally important half because that's especially where the structure for the story comes from that maybe is, well, I don't know actually whether like G P T three and chat G P T have really been used to actually create stories and novels. I don't actually know. But um, yeah, the kind of more structural aspects that these other systems use are actually, you know, just as important.
But yeah, as you said, they're not really
Rafael Perez y Perez: Exactly. [00:28:00] Yes. I'm very happy to hear what you are telling me because that's exactly the goal of the book. I mean, people need to understand that the, the field is much, much bigger than just, um, machine learning. There are a lot of other things there. Uh, All these approaches have, um, limitations and have a strong aspects, all of them, including deep neural networks, all of them.
So it depends what your goals are. If your goal is to try to understand the mind or to try to contribute to the understanding of the mind, like is the Mahik case, then system like GT three are very bad for that. . People don't know really what is going on inside the system. So if you want, your goal is to generate very impressive test with a lot of different, different topics.
Of course, GT three is really good at that. No, it, it generates something very, very impressive things, you know, but, so it shows the power [00:29:00] of pattern recognition and statistics, informational models to produce this kind of, of text, you know, so, It's important to know all this because we as society need to be aware of all the good things about these technologies, but also about the risk that they can present.
And to have the proper information about how they work will help us as a society to be able to, to push for the, the good things. No, and and to alert from, Hey, be careful with this. Be careful with that. No, so that's the reason I believe it's so important that society understand better this kind of system and don't believe that only there is one company or two companies that develop artificial intelligence and all their systems are the only ways, because the way many journalists report this is in that way.
No, it seems that only, uh, [00:30:00] two, three companies are doing artificial and that's not true. No. At thought.
Benjamin James Kuper-Smith: Yeah. Um, yeah, it's funny, like, I guess most of the questions I prepared were around trying to create machines that can write stories. But now that you've kind of, at least to me, clarified like the purpose of Mashika, it almost seems like that's going in a direction that, uh, well, I mean, I guess the book is still like Story Machines is still very much about like trying to, in a way, like it's a survey of the different approaches people have taken to write machines that can write stories.
Yeah. I'm just curious, like, is there. . It seems to me then that there's a little bit, these two different, like, conceptually different approaches to creating those machines. The one is, you know, the more conceptual, um, structural, the ones like, like yours and the previous ones, and then the, the current more famous ones are [00:31:00] the ones that are built on lots of data with language and all this kind of stuff.
I mean, is the, I'm assuming there must be like people trying to combine the two to kind of get the best of both worlds, right?
Rafael Perez y Perez: Yes. Traditionally the first type, like mak, like t um, this other system based on problem solvings are symbolic systems. No, that they are classified. A symbolic systems, and we have this connection in models, you know, this, uh, like all those based on DM neural networks and this, this kind of stuff. When the results of using neural networks started to appear, there was this huge, no huge boom.
This, all these articles have been, been published in the last years, knowing the last month for some years now, no showing and talking and describing what is going to happen. But also slowly the, all the limitations of this system are also starting to merge because as I mentioned before, all of those systems have [00:32:00] strong points and we point that's the nature of, of, um, science and the development of.
These kind of programs, um, something that is starting to slowly take more force is this idea of combining both, not the symbolic systems with the neural system. No, they are calling it neuro symbolic systems. This idea has been for a long time, it's not something really new, but now they're trying to do it also in, in narrative generation.
Uh, we will see where it goes. I think really what we need is something different, not the combination of both, but the function of both to generate something new. That's where I will, I think, is the direction we need to go back. Of course, I don't have to answer, know how to implement implemented, but, um, because clearly we need to, to cover the gaps that the different system.
But there's still a lot of things we need to sort it out. So that's the reason I think [00:33:00] we need something, something new. But yes, certainly the next step is how to combine them, how to, to see how we can work with these different approaches together and start to get better results in, in, in different aspects.
So definitely service is the root.
Benjamin James Kuper-Smith: Yeah, I mean, so one thing I was thinking about when I, uh, started preparing for this conversation is like, basically why might something like, uh, GT three and that kind of approach, why might that not work so well for stories? And the answer I got basically, I'm curious what you think about my answer, , whether that makes sense or it's kind of a stupid answer.
But the basic res answer I thought, um, why it might not work, is because the main problem is data so that they don't have enough data. So my, the, this is based on the following idea. So from what I understand, the reason that. Let's say a company like [00:34:00] DeepMind, you know, was super successful with the games is because a computer can play billions of games, you know, against each other, uh, against itself and can get feedback, you know, every few seconds that it can use for the reinforcement learning or whatever agent they use.
Now, that's very difficult to do if you're trying to write, let's, I mean, let's take the extreme case. You're trying to write a novel f to get feedback about how good the novel is. I e how good the, uh, the AI is performing. In writing a novel, a human has to read the novel and then tell you like how much they like it or what aspects they like of it or whatever, which means instead of, you know, a supercomputer or a cluster, being able to, you know, run billions of games in a few days or whatever.
now you need people to assess basically the quality and to provide the feedback manually. Therefore, you [00:35:00] just can't run a system that is, that requires that much data. Does that kind of make sense? I mean, I guess, I mean, especially like at the big structural level of a story, right? Because you need to read the entire book to say whether you like the book or.
Rafael Perez y Perez: Yes, yes. I think you are in. Direction to play a a a game is very different. To write a story, not very different activities. No games have very specific rules, very clear rules that you can represent and you fall to write a story requires a lot, a lot of different things, not just that requires understanding of different situations, social situations, cultural situations requires also to understand how we humans behave.
Um, uh, emotions I mentioned before. No, for me, stories are emotions. No, they, they, these are in very essential part [00:36:00] and nothing of that is represented in programs like G P T, no, in, in, in programs using Transformers, the, those systems. First, they don't know even that they're writing. They, they, they cannot reflect about what they are writing.
They don't have knowledge of representation of the world. What they do is they use this very, very sophisticated statistical information to generate the next phrase, the next phrase, not Transformers now allowed to take a whole context of linguistic elements to decide how to continue the narrative. As I said, uh, storytelling is much, much more than that.
So that's the, the reason is I think we are far away from achieving really, uh, real results about storytelling systems like maka. Others like minstrel try to represent these kind of aspects. For example, Mr. In str, the program in STR [00:37:00] has a model of how creativity works. I'm not going to describe here to avoid technical details, but it has a specific representation with details about how the outer Turner thinks the creativity works, and it includes some representation of the man, some representation of of characters.
In the case of Maka, I explained already to you that Meshika represents this relation between characters. The new version of Maka is able to evaluate its own output. Of course, this evaluation doesn't compare to human evaluation. We are still far away from that because. , I mentioned this before, I will repeat it several times.
We don't understand how our brain works. We don't understand how our mind works. The evolution process is something very complex. It's not that simple as sometimes we seem to believe, [00:38:00] but at least there is a model there, the how to evaluate the stories it produces. So Mashika puts a grade between one on 100 about the stories it, it generates.
So sometimes it's very happy with its own story. Sometimes it says, oh, this is rabbits not put, or four or three that happened because it has a completely different, let's say, philosophy, how to represent this process. No, it's not just a lot of data that you extract the patterns and that makes these statistical associations.
Uh, so I believe that you are right. We cannot go further so far because we need. Other information that is not possible to obtain from huge amounts of data like the one, like the one I have just described. No, it's not possible to obtain from that, from relationships between this huge amount of data. For example, understanding of how the [00:39:00] the world works, understanding how our emotion works, understanding that in Mexico you are in love, at least before you used to serene a girl and things like that.
No, I wrote a song to, to make my wife fell in love with me, for example. No, I mean, so people
Benjamin James Kuper-Smith: for your family.
Rafael Perez y Perez: my wife is from Barcelona, so I have to keep nap her. Uh, I have to convince her to come here. Uh, so I did a lot of things between those. One of those was, uh, to compose her son for her. So to serene.
Once I serene her from Mexico, I, I was with a group of friends. They played the guitar. I played the guitar. So I, I told them guys, we, we are going to Seren girl tonight. Oh, perfect. In which neighborhood she lives, they ask, I said, she lives in Barcelona. What? Yes, in Barcelona. So we telephone and we spent like 30 minutes in a house friend singing for her.
And of course she [00:40:00] was crying because she had never received before. A, sorry. Because it's social, it's something different.
Benjamin James Kuper-Smith: Yeah. Yeah.
Rafael Perez y Perez: So this kind of stuff cannot be represented or cannot be just model in a computer system base. Just huge amounts of data of linguistical. Yes. Data could, linguistic elements, no words, paragraphs and so on.
So I think you're right. That's the reason it makes sense to start to combine these approaches. No, the reason I believe we need something new is because, okay, we are going to combine them. So if we are successful, maybe we will have some representation of the world with this huge capacity to produce language.
Not that language model have, but still there are too many gaps there. Then we need to not to connect. There are many things aspect that we need to connect. No, I don't see how, for example, thinking of magic, I don't see how my representation of emotional relationships [00:41:00] and conflicts can be useful in a system base on the neural network.
We need to, to see how we are going to make these connections, you know?
Benjamin James Kuper-Smith: It's funny, I just, I just try to imagine what would happen in Germany if you, uh, came up with a bunch of guys and you'll start to play the guitar in front of someone's house. And I'm trying, they'd probably just like , not sure they'd call the police, but they'd be, probably the neighbors would be very annoyed with the whole situation that it would be disturbing the peace and quiet of the neighborhood or something.
But, and I, I mean, what I mean, what's funny about it is that I can definitely see how, let's say you train, uh, a system on all sorts of stories. I can definitely see how the system learns. Let's say you put lots of Mexican stories into it, it learns like, ah, this is a, you know, an important part of love if you don't specify that it's in Mexico, and then it will add that to any story that might be in a different place.
Just that it would seem completely out of context in that situation. It might still work in Germany, I don't know, but [00:42:00] it would be a very different thing to do than, yeah, maybe in Mexico, um, which would make the story just seem a bit weird if you just pretended that that was normal
Rafael Perez y Perez: Yes, all these kinda things is the ones we need to, to, to justify because, and to represent so the system can manage them properly. Because in one way it can be very, , interesting not to have a guy in, in, in the UK or in Germany, seen in a Seren eight, but it wouldn't make sense to expect the same answer, for example, for the, from the girl from, uh, that in Mexico.
No, it wouldn't make sense and the system needs to understand that. So let me just tell you quickly story. When I arrived to England, I made this Norwegian girl and I was telling her about ceremony and she told me if someone seren to me, I will start to laughing and laughing on like, like, what is this? So maybe assistant could have a [00:43:00] Mexican playing a Seren eight in, in Nor, and then the logical answer will be that the girl start to laugh.
No. And to make fun of the guy because something completely strange. No, that could be something. Yes, but you need to have all. Social context, that knowledge about the culture. So that's the kind of stuff we still need to represent properly in, in, in these kind of systems. And definitely system based. Uh, love of data, just linguistic data, uh, cannot represent.
Benjamin James Kuper-Smith: Mm-hmm. Yeah. I mean, like one question in general is whether a computer can ever write, you know, a story that people would actually find meaningful. And I think one criticism or like one. Reason that people say why this is not possible is because you have to have this experience of actually existing in the world and knowing all the small details that you can only really get to by living in the country, [00:44:00] uh, or not the country, but like in the world and experiencing these things.
And yeah, I mean, is this like a fundamental barrier that, that is gonna prevent computers from ever being able to actually write convincing stories? Or is it maybe not that important if the stories? I don't know. I mean, there's lots and lots of previous stories that they can maybe use to figure out what a makes a good story.
Um, yeah, I dunno. Is that a fundamental barrier that I don't know, or, or can that be circumvented some.
Rafael Perez y Perez: Uh, you know, it's just, I think there are a lot of things related to this question. In one hand, I just mentioned that I provide information to Mahik, I provide the stories. use that stories done not to get worse, but to get information about the world. Um, from that information generates new stories, stories that different to the ones that used to feed the system.
Can those stories [00:45:00] be meaningful for, to someone? I will say maybe no. Maybe just, it depends also in the other person, it depends on the expectation of the other person. It depends on the, it depends on a lot of things. Yes. So in that sense, I don't think that necessarily can be a barrier. Three can also produce something that makes you reflect, made you think, made you see in different ways.
Something, no, the system doesn't have an idea that it is producing that in on you. But , but it can happen as well. No, but of course we humans. The way we write and what we trans, uh, transmit to the readers is very different. I mean, computers don't, don't have any opportunity at the moment to have, um, this kind of experience, not the, the rich experiences we have when we communicate.
No. But maybe in the future we'll be able to have [00:46:00] systems with much more rich experience with all this cultural and social knowledge. I, I don't see that necessarily is a limit, you know, at the moment in this. But I think in the future can be incorporated, know if we find the right way to do it. So that's the reason I said that.
This question has like different perspective, different verses. Another thing and I want to mention about your question is that in the book, um, this is, uh, Mike's idea, he was reflecting, Mike was reflecting what happened if. The computer tell us stories about its experience in the internet. For example, no computers, no.
All the ways that happen, what will happen there? No. The computer will have an experience and it is going to be completely different to have we have lived. I think it's an interesting idea, uh, to think about. No, uh, sometimes people ask me [00:47:00] if I want Mashika to write to a human and no, no, not really. What I would like is that Mashika Cat could produce its own style and that some people feel attracted to that style, but that I, I'm not pretending to, to produce outputs that people think it's, uh, produced by a human.
That's not my. Yes, that's, that's another perspective. Also know that some of us are not interested to just produce this reproduction of humans. How are we going to reproduce a human Nowadays, we don't have any way, but we can produce system that generate interesting things, knowing everybody knowing that that's a computer generated output and it has a specific characteristics that belong to computers.
Benjamin James Kuper-Smith: Yeah, I mean, so one thing I was curious about is, Almost kind of where in the future the development of these new systems can come from. [00:48:00] Because I mean, so, so from the way it seemed to me from the book is that often these projects are one person or very small teams kind of working on something on their own or in very small groups and then producing these systems.
And this is of course quite different. I mean this is, you know, most of that's, you know, in the past, in the seventies, eighties, whatever. Right. That's of course quite different from, you know, big teams like Deep Mind or opening AI or whatever who have lots and lots of people working together to, to have, yeah, of, I mean just very fast advance, right?
Because you have so many more people and I dunno, so I have like one, uh, basically my, I'm wondering whether there are big companies, especially Amazon or Apple, that might be trying to develop something like this. And I'm just curious kind of whether you think. This is the case or not? I mean, for example, for Amazon.
My, the reason why I think for them it would be very interesting to do this is because they, I mean, if you [00:49:00] think what they are as a company, they have, they display books, right? Because when you search, you get all kinds of different things. They distribute the books to the people. If you use Kindle, they actually, you know, show you the, the, the book on itself.
And they have the ratings from people who are doing that to have the feedback. The only thing they kind of don't have is the creation of books itself. But basically if they manage to do creation also they'd have like the entire cycle, of fiction, right? From producing it to bringing it to the people, selling it, getting feedback on it.
Also on kind. what people highlight, how long they stay on a page, what the rating is at the end, all these kind of things. And it just seemed to me like it would almost be crazy if Amazon didn't spend a few million a Euros or dollars a year to just like throw together a few computer scientists, linguists writers, to produce a system like that.
Because if they achieve it, the payoff [00:50:00] would probably be enormous because they could, you know, sell large parts of the entire fiction writing system, um, without having to pay copyrights to authors and all that kind of stuff. I'm just curious, like, does that, anything like that kind of, do you know of anything like that?
Or is this what I'm saying? Uh, a bit.
Rafael Perez y Perez: No, no, no. I don't think it's silly at all. As you said, and you're completely right, most of these systems have been developed by, uh, very, very small groups. So individuals, no. Many of the systems are PhD dissertation, norel. Um, they Dreamer and others are PhD dissertations because in general these are, this is basic research and they don't have so far a clear commercial application.
The reason all these companies you mentioned are invested so much now in deep neural [00:51:00] negro Negroes, because they. A commercial application, as you mentioned, they see monitor, no companies, the purpose of companies to generate money. No. And so when that happens, then of course they're very interesting and they have this army of scientists and engineers working in, in all these developments.
And I think what you describe it is one of the goals of developing programs like G p T, no chat, G P T or g PT three. And soon we will have G P T four. That's, uh, that's the duration they are aiming because you describe it very well. They will have the control of the whole, uh, creative processing, writing, uh, and that means a lot of money.
So yes, there are, I, I believe that's, um, one of the, their main goals, which is not as scientific. So the companies as, as long as they see that they can get some profits, will invest a lot in, in that, in that [00:52:00] technologies. Um, ideas like the ones I work with will don't have that kind of support. Of course not, because as I said, they are don't, they're not have any evident commercial application, but , that's the reason.
No, we, some of us work in universities. That's the place where we can develop this kind of stuff. Not that uh, maybe someday they will have some other kind of commercial application. But for me, the important thing is to try to contribute to the understanding of how we work. You know? So, but you are completely right.
I think your description is very precise.
Benjamin James Kuper-Smith: Okay, cool. Yeah, I was, I always wondered just whether that was like, yeah, a crazy idea I had or something sensible. But it's interesting, I never thought about it. It was like I never thought about it, that the, um, the old ones were not that the, that the emphasis is, you know, between academic basic work and commercial applications.
I never quite drew that distinction so [00:53:00] clearly. But that's of course, a hu huge reason why, uh, like, well, why these differences exist.
Rafael Perez y Perez: And you describe one scenario, but there there is other scenario. For example, for video games. Video games is a huge industry producing lots and lots of money, and there are people trying to produce video games that can generate. Their own stories, not because imagine when you are playing with these systems and the system is interest, uh, intelligent enough to start to improvise the story based on what is happening.
That based on your own characteristic around that, that will produce a big thing. That's one of the thing we mentioned in the book. So a film, video games is another good example of how these technologies are trying to be used in order to produce commercial benefits.
Benjamin James Kuper-Smith: Yeah. Yeah, yeah. Yeah. As [00:54:00] always, the future is very difficult to predict. But, uh, what do you think is gonna happen like in the next few years? I mean, with, yeah. I mean it seems to me like with chat g p t, we, there's been, at least on the, like, on the, when it comes to the words the computer can put together and create coherent sentences and all that kind of stuff, there's been huge advances, advances in the last few years.
I dunno, like what do you think is gonna happen like in the next five to 10 years or something of machines trying to create.
Rafael Perez y Perez: Well, I think one of the things that is going to be clear for the developers and for the people, all the limitation this system have, which I think is important that people become aware of them and as a result of that will happen quite, we just mentioned earlier in this conversation, The next step is to start to mix the different approaches we have no, [00:55:00] that we know have had some achievements, but we need to combine them.
And I think that's going to be the next step for the following years. I think, uh, people will walk in that direction. It's going to be enough. I don't think, as I mentioned also earlier, I think we need something different, but we need to work, I mean, to move, to experiment and then see the new limitations and the new achievements, and then start to figure out how to, to improve all, all, all these, um, all these ideas.
That's how, how I see. Also, I think some people, few people will work with the idea of trying to represent. How our mind works when we are writing, um, in that direction. Also, I think one of the ideas is to mix this kind of system, but also to think in new theories, not new theories. That's the reason, the kind of research you do, I'm very interested about because I think I can get a lot of [00:56:00] really interesting information from that to try to implement in the system.
So the more that other areas like neuroscience and and other, and social sciences advance, we can also try to incorporate those ideas in, in computer models. So interdisciplinary work for me, it's also a key factor in the future because, All these systems we have been talking about basically are done for people from the computer science world.
And, uh, storytelling is something so interdisciplinary. We need to really work with other disciplines, not, not just from, from our perspective as computer scientists. So I think those are the, like the direction I see, uh, for the future in the near future.
Benjamin James Kuper-Smith: And for you personally, is that, I mean, are you, do you work, are you continuing working on, yeah, I guess, what did I say earlier? Trying to model the creative [00:57:00] process by creating a program that kind of tries to do that. Um, is this what you continue working on in that vein
Rafael Perez y Perez: Yes, yes, definitely. For me, me. Is, um, has become in my, uh, life project, no, the project for me, for the rest of my life, because there are too many things to do. There too many things. I, I'm still working in different aspects, improving the way Maka, for example, evaluates its own outputs on outputs, you know, um, the way, for example, I develop a model to identify when a plot is not emotional, coherent.
So there are a lot of ways we behave that follow certain patterns that are related to emotions, and it's very important to understand them in order to make coherent plots. So that's an example. Uh, for example, I also working with some artists in New York, because they are multimedia [00:58:00] artists and we want, they're developing a piece called a Stone of Madness that includes videos and writing and different stuff.
And we want mashika to be. Part of the, of the creative process. So we are working together so to, and we are modifying, um, the system can adapt to their necessities so it can produce things that can be part of their piece. So, um, this is a really, really interesting and huge, uh, challenge because, uh, we are now talking that the artists, Liam Bill, that's their names, uh, have to feel really comfortable with the outputs, you know, that to feel that really contribute to their piece.
So we are talking about real artists know, famous, very, very strict artists, which are really good. And of course they are not, they risk with this. So that's a really interesting way to put machine in the real world. And. What we can get from that. So [00:59:00] there are lots of things in the future, let's say, uh, my energy, how, how long it lasts to, to continue with this.
But, uh, but yes, at least at the moment, my plans are to continue in enriching this. And the reason is because I have created a really, in my opinion, interest in infrastructure with all this model, no, with the, with the mahik. And I think we need to exploit it, know to, to, to trade them the, the best way to, to see new, new ideas.
Uh, so that's my goal and I hope, um, I'm able to achieve it and to leave it to the community so they can still continue developing these ideas.
Benjamin James Kuper-Smith: Okay, cool. Um, yeah, I've, I've run through my questions. I don't know if there's anything else you wanna add at the end.
Rafael Perez y Perez: I will just say thank you so much for inviting me. It's been very interesting for me, this conversation.
Benjamin James Kuper-Smith: Well, thank you.
Rafael Perez y Perez: Um, I hope people realize that it's very [01:00:00] important to be informed about how this system works. I hope the book, the Story Machines Achieve this Goal and also the new book we will publish in few months.
And
Benjamin James Kuper-Smith: Mm-hmm. . Yeah. And what's that gonna be called?
Rafael Perez y Perez: yes, uh, in introduction to narrative generators. That's, that's the, the, the title. And I, as I said, you don't know, you don't need to know how to program a computer to read this coming book. And for us, that was a key aspect because we were as much people as possible to really get informed about all this to.
different aspects in that book. One chapter is dedicated to d neural networks and transformers to try to provide a good idea how it works, and also to the other, other techniques not problem solving. And maik, of course, we have a whole chapter about that. So I think with that, um, we as society will be in a better position to, to [01:01:00] push our politicians and all these people making laws to do the right work in Northern.
We can have or take advantage of the positive things that these technologies have and be very careful with the risks that this technology also have. And so I hope that in some way, this, this conversation contributes in that direction. Uh,
Benjamin James Kuper-Smith: I hope so.