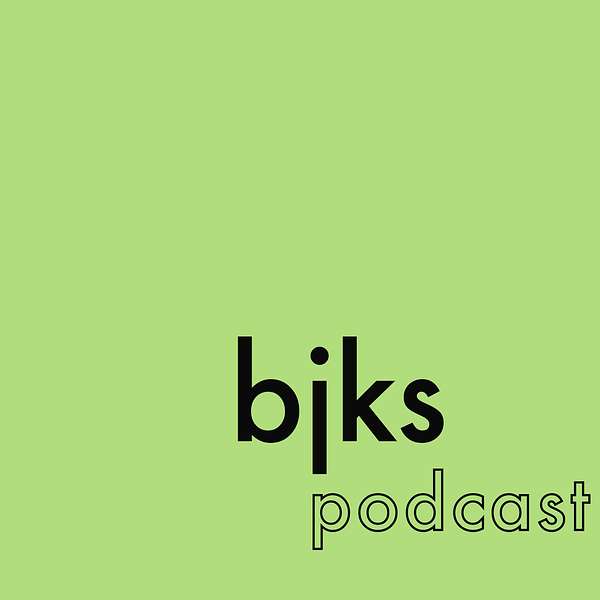
BJKS Podcast
A podcast about neuroscience, psychology, and anything vaguely related. Long-form interviews with people whose work I find interesting.
BJKS Podcast
62. Nils Köbis: AI, corruption, and deepfakes
Nils Köbis is a research scientist at the Max Planck Institute for Human Development, where he studies the intersection of AI and corruption. In this conversation, we talk about how Nils got into working on this topic, and some of his recent papers on AI, corruption, deepfakes, and AI poetry.
BJKS Podcast is a podcast about neuroscience, psychology, and anything vaguely related, hosted by Benjamin James Kuper-Smith. In 2022, episodes will appear irregularly, roughly twice per month.
Timestamps
0:00:04: Moral Games
0:13:09: How Nils started working at the intersection of AI and corruption
0:30:12: Start discussing 'Bad machines corrput good morals'
1:01:00: Start discussing Nils's papers on whether people can detect AI-generated poems and videos
1:25:59: Learning to say no and to not get sidetracked
1:31:05: Writing a PhD thesis
Podcast links
- Website: https://geni.us/bjks-pod
- Twitter: https://geni.us/bjks-pod-twt
Nils's links
- Website: https://geni.us/koebis-web
- Google Scholar: https://geni.us/koebis-scholar
- Twitter: https://geni.us/Koebis-twt
Ben's links
- Website: https://geni.us/bjks-web
- Google Scholar: https://geni.us/bjks-scholar
- Twitter: https://geni.us/bjks-twt
References & links
Moral Games (in German): https://geni.us/moral-games
Nils's podcast KickBack: https://www.icrnetwork.org/what-we-do/kickback-global-anticorruption-podcast/
Replika AI app: https://replika.com/
Science fiction science: https://www.mpib-berlin.mpg.de/chm/guiding-concepts/concept-2-science-fiction-science
Collingridge dilemma: https://en.wikipedia.org/wiki/Collingridge_dilemma
GPT-3: https://openai.com/api/
Fotos of people who don't exist: https://thispersondoesnotexist.com/
Abdalla & Abdalla 2021. The Grey Hoodie Project: Big tobacco, big tech, .... Proc of 2021 AAAI/ACM Conf.
Crandall ... 2018. Cooperating with machines. Nat Comm.
Goffman 1959. The Presentation of Self in Everyday Life
Harari 2016. Homo Deus: A brief history of tomorrow.
Hawking 2018. Brief answers to the big questions.
Kehlmann 2021: Mein Algorithmus und ich.
Köbis ... 2021. Bad machines corrupt good morals. Nat Hum Behav.
Köbis ... 2021. Fooled twice: People cannot detect deepfakes but think they can. Iscience.
Köbis & Mossink, 2021. Artificial intelligence versus Maya Angelou... . Comp in hum behav.
Köbis ... 2022. The promise and perils of using artificial intelligence to fight corruption. Nat Mach Intell.
Leib ... 2021. The corruptive force of AI-generated advice. arXiv.
Leib ... 2021. Collaborative dishonesty: A meta-analytic review. Psych Bull.
Mnih ... 2015. Human-level control through deep reinforcement learning. Nature.
Rahwan ... 2019. Machine behaviour. Nature.
Silver ... 2016. Mastering the game of Go with deep neural networks and tree search. Nature.
Tegmark 2017. Life 3.0: Being human in the age of artificial intelligence.
(This is an automated transcript that contains many errors)
Benjamin James Kuper-Smith: [00:00:00] Maybe as, as a, as a very serious first question. Uh, do you still buy your clothes at H and M?
Niels Koebis: Um,
Benjamin James Kuper-Smith: do you, I'm assuming, you know, what I'm referring to
Niels Koebis: yeah, yeah. The, the moral games, I guess. Right.
Benjamin James Kuper-Smith: exactly. Yeah. And what are the moral games
Niels Koebis: The moral games are an idea that, uh, started from many discussions with two friends of mine, which BA we basically ask ourselves, ourselves the question, what is something that we do where we know that it's kind of wrong. right. And this can be something like eating meat because of the environmental impact or the animal welfare.
It can be throwing away. Food can be buying unnecessary, plastic bags, buying fast fashion and so on. And we realize that we all have these, right. Like basically a list of things where we realize like, oh man, keep doing this. Why? Why? Especially in the moment, right? Like you go out with friends, you have some [00:01:00] drinks.
And then all of a sudden the Kpop looks very, very tempting. Right. and it's very difficult not to go for it. And we basically de de developed a game to. Keep each other accountable. So we, we said, okay, what about we are competing in our moral behavior against each other, and you get minus points. If you are committing any of these acts.
And then obviously a long discussion in suit. I mean, these are two researchers slash scientists. And at one point I was actually sharing an apartment with one of them. We had a whiteboard in the apartment that shows you how much of a nerd I am. . So basically on that whiteboard, we had this metrics and we said, okay, eating meat is minus 10 points.
How many minus points is eating fish? And does it matter how much you eat? Right. Does it matter if, for example, the meat you eat has actually been ordered by someone else and that person is not finishing the food, is it okay to eat it then do you still get minus points? And basically we had so many fun [00:02:00] discussions and we really developed this into a proper game that we played in a very low tech way.
So we had just a, an Excel. Sheet which every week we typed in how many points we had. And then after, uh, several rounds, basically you could earn points by not, um, engaging in these, uh, different types of behaviors. Yeah. And then every round somebody would win, uh, and that person would become the moral king for a round.
He would get a.
Benjamin James Kuper-Smith: Uh, how long was one round again?
Niels Koebis: Uhwe depends on how , how we behaved, right. Could be between six and 12 weeks. Um, and so, but
Benjamin James Kuper-Smith: So it's fairly like
Niels Koebis: yeah, and then
Benjamin James Kuper-Smith: It's not like a week or
Niels Koebis: had one week cheat week, so basically, um, for one week all bets were off. And then at one point we realized, okay, we gotta, we gotta change that because that's not, we almost offsetting everything we did over the last eight weeks within one week.
And so basically then we start, we, it was basically a very interactive, dynamic and [00:03:00] deliberative, uh, way of, of trying to figure out how we can, you know, have fun. But at the same time also act more morally, I would say at least what we would consider moral. It's a very subjective thing, I guess. Um, but for us, it was really effective to be honest, Benjamin.
I was like, I was not eating meat for weeks on end and yeah, ever since then, I'm always carrying around, uh, to go cup and, uh, spare back. Some of these things have really become habits. What is interesting though? My preferences haven't changed. I still like eating meat, but I don't do it that much anymore.
So in a way it was tailored towards us, a very, sort of very effective tool. We then had someone reaching out from the diet, which is a newspaper in Germany and they wrote an article about it. And then we had like, some of the comments basically really criticizing what we do. You know, like, oh no, this is like a social scoring system.
This is terrible. And I mean, in a way it was funny for us because we never said that we wanna scale this up or anything. It was just us [00:04:00] three doing this. And the way we did it, I think is also not very scalable, to be honest, it's not like we had this, you know, pre-made thing and we just, you know, wanted to spread the word around it and actually get made other people engage in it rather.
It was the opposite. It was us having fun with it and kind of saying like, this probably doesn't work for anyone else. Um, And as soon as we moved apart, like then basically we, we, uh, ended the Fletcher because the friend left Amsterdam, I went to Berlin, the other friend then went to Amsterdam. So basically we're now in separate cities.
And it has lost a little bit of that vibe because it was very much a bit a thing of, of, of constantly discussing it and constantly refining it and try, you know, defining new rules and so on. And that really lived from this being together at one place and having hanging out.
Benjamin James Kuper-Smith: Yeah. I wanted to ask whether you read the comments or not, because, I mean, as you said, there are lots of. Quite negative, but there were also lots of that were quite positive, right? Like I think the one that really stuck out to me was something like, wow, was solution rather than just people complaining.
This is amazing.
Niels Koebis: Hey, [00:05:00] Benjamin. The thing that I enjoy the most out of it are these few comments. And one of them actually was, um, a person reaching out. He said, I'm doing consulting for fostering innovations and companies. And your game is an inspiration to me. And I was like, wow, that's amazing. So we just met in Berlin a few weeks ago.
We saw each other and we directly clicked because I think we kind of both got it in a way. Right? Like we kind of could both laugh about it. And there is this concept of the, the homo ends, like the playing person, right? Like that, there's very something, very human about games and playing. And that's what the whole.
Idea is based on, and he has basically this moonshot game, his name is till, um, he has, um, a game that is basically there to foster innovation, but through a very playful way. And I kind of in general, really like this approach and I think that's why we directly connected. So something good came out of it.
And the, the negative comments, I think, I guess it's something that we, you know, that a lot of people will tell you that you should just not, yeah, not really [00:06:00] engage.
Benjamin James Kuper-Smith: Um, yeah, but I mean, to be fair, one thing that I think might have led to some of the negative comments. Might not have appeared otherwise it's because this was, I mean, so for the non German readers site is a big weekly nationwide magazine, right? This isn't like some small, like regional thing where they were reporting on like two people who did something random.
This is like a big thing. And this was, so I feel like it's weird actually, maybe first the question, like how did that even find out about you? Because it, anyway, the point I was trying to make is like, it seems to me because this was on a big newspaper, it almost seemed like it was something you guys wanted to, you know, make, uh, get other people to also do or something like
Niels Koebis: Yeah, it's a, it's an interesting, um, I guess an interesting paradox there, because the site had this series, I think it was called the or something like that. Like basically the answer to our, to our big problems. Right? Like climate
Benjamin James Kuper-Smith: not to be confused with D what the band.
Niels Koebis: yeah. Um, And then one friend of mine, basically like [00:07:00] one of okay. Long story, basically another friend of our ours made us aware of this and said, you should submit this moral games to the tide as one of the, we're like, no, come on. This why we don't really, you know, it's not something that we need the publicity for.
And then somebody said like, yeah, but look, maybe somebody else kind of likes the idea and does it, does it too, or maybe some other connections. Like the one that I just described come out of it, go for it. And we just sent a really, you know, nerdy email, um, and got the best answer, like, uh, Juliana fusA the author or the, the, um, the author of the article got back to us.
And she's like, the idea's very interesting. I have to admit it's also very nerdy . And so basically she directly, I think, understood directly what the, the whole gist of it was. And we had a really fun interview with her and we kind of treated this as, just to be honest, Benjamin after this interview, we, you know, had a few beers and just a lot of laugh about it, kind of thinking like, wow, we made it to the side with something like this, you know, like [00:08:00] you, you imagine at one point, like you say, it's one of the big newspapers here that maybe some of your work gets covered there or whatever, but I was never expecting that this kinda, uh, fun idea would end up there.
Benjamin James Kuper-Smith: It's also not only one of the big ones, it's one of the big, serious ones, right? It's not like this is, uh, sun or whatever, right? Like, this is.
Niels Koebis: It's the one newspaper that I still have a subscription to. And, um, yes. So anyway, it was a cool, cool validation. The moment when the day, I remember the day when the article came out, got a lot of messages from people like, no, oh wow. You actually made it. Like, I cannot leave it because a lot of people were laughing about us when we did that.
I mean, you have to imagine. One thing I realized is actually when you have these sort of. Heuristics with you and you start thinking about it constantly like, okay, wait, this gives me minus points. You become socially a more complicated person. Like I'm generally a socially, very relaxed person. And when I'm out with friends, I wanna [00:09:00] enjoy the time.
And that's why for example, the K up, I would sometimes really just go with it and say like, yeah, let's just go. You know, like, if everybody wants to go, let's go when playing the game, this changes and you start to become really annoying because you have whatever things that you don't don't do. And for example, like one thing we, we really try to reduce flying and, um, some things are just not possible anymore, right?
Like quick trips with, um, you know, flying in and out to some conferences and stuff. I've basically completely stopped that. And I, I choose the conferences I attend based on whether I can go by train and these types of things. And they are largely driven by that.
Benjamin James Kuper-Smith: Yeah, I was curious about, um, like as a kind of last point about this, about the moral games is I was curious like how well it worked. I mean, you mentioned earlier that you already changed quite a bit, but one point in the article I found quite interesting and potentially telling, uh, God, I should have translated this before.
Uh, anyway, so the sentence is something like a zero. Which is an oddly non-German [00:10:00] name. Um, uh, has the name a moral Toothface? Uh, well, by you guy, you two called in that, because, uh, on the one hand, he often he often wins the game. He's the moral king, but earlier hand, he often like completely loses it. Uh, like it's lots of meet, uh, plastic bottles, whatever lot's rubbish and all this kinda stuff.
So I was just curious, like, is it, I mean, you mentioned earlier, already the cheat week, you, you stopped doing that, but I was curious, like, is it the kind of thing where it, or rather, it seemed like, uh, C was, uh, he was playing the game rather than the point of the game. Like, it sounded like he was like for one phase, just like, I'm gonna be really good now until I win this one and then I'm gonna do whatever for the next one.
Niels Koebis: Yeah, I would actually say that. Um, it's a super interesting question that comes back in my research. Like how much is it the context and how much is it your own, you know, will, um, to be on a Tobi moral, because he does a lot of field research, uh, in the democratic Republic of Congo. And he said like, [00:11:00] it isn't possible not to buy plastic bottle.
And so basically like in the first week he would have so many minus points that he cannot compete with us anymore. Right. Because by that point he's basically hopelessly last. Um, and then he said like, okay, I'm often invited here and they serve meat as a sign of respect. I feel bad for not eating it. And so in a way, right, like these are the, you can see in the score sheet of when he was, uh, abroad.
And I have to say I made the same experience, but it doesn't even have to be, uh, going to, to Congo. I even had, when I went to the us, like just the, the possibility to get, for example, the coffee in the mark that you bring is something that we now here take for granted, actually it's sometimes, sometimes even rewarded that you get a little bit of a discount.
I had really strange interactions with people in the us where people would demand that I take a Togo cup and I was like, no, I don't want it. And they were like, no, you have to I'm I'm obliged to give it to you. I was like, yeah, but can you just put the, the thing in there and keep the, [00:12:00] the, the cup? And I would remember one train ride in MTRA where I had real tuck of war with this lady trying to basically hand back this Togo cup so that I don't get minus points that she, I think was just concerned that in the end, the number of coffees and the Togo cups wouldn't wouldn't match.
Benjamin James Kuper-Smith: Yeah. Yeah,
Niels Koebis: Just in general plastic in the us was just, you know, you go for, I don't know, seminar talks and everything is wrapped in plastic. And so that would actually always kind of make it very difficult to, to compete when traveling. So anyway, I, I gotta cut some select for, for several. I think it was a lot with the, the context.
Um, and then maybe there is the, the, what I think Roy Bama is the one's coined the, the, what the hell effect, right? Like if, if you already lost it, right, like then you might as well just enjoy, uh, a few bits and pieces here and there
Benjamin James Kuper-Smith: yeah. But it seems like this moral games thing is a large part of your life. That's really making you get into fights with poor personnel.
Niels Koebis: yeah.
Benjamin James Kuper-Smith: poor woman,
Niels Koebis: in it more passionately in the [00:13:00] past. It has, it has dwindled a little bit.
Benjamin James Kuper-Smith: Um, yeah, maybe we can just start talking or getting to talk about the actual, like science and your papers and stuff. Um, I thought, so you have a podcast and you at least the two or three episodes that you did the interview on that I listened to. You started always with a question. How did you get into working on corruption?
Um, so I'm not gonna exactly ask that question, but I'm curious how you start, how you basically went from your PhD, work on corruption to now working on, uh, let's say corruption with not corruption with machines, but maybe the intersection of artificial intelligence and machines and corruption.
Niels Koebis: So I did my PhD. Um, basically it was a funny coincidence. Actually, the reason I did corruption research was because I was brainstorming together with a colleague, um, for a class on social dilemmas on creating new games, like economic games. And then we played around and. He at [00:14:00] one point said like, this really looks like corruption, like bribery, right.
We had a three party game. And then I, I kind of started liking the idea of designing a game to study corruption. We applied for funding for it, and I got, got a grant to, to do research on it. And it took me on this journey, uh, through the different disciplines. Because at that point there wasn't really a lot in social psychology on it.
And re, made me realize like actually topics like this are best studied from an interdisciplinary perspective. So I, I was very early on not really reading that much psychology and much more from other disciplines. And I reached out to researchers from all different disciplines as well. And through that, I think I still, throughout my PhD got a lot of input from other, um, yeah, like other disciplines, other topics.
And one of the, the cool things about it is that you can always link them back to some things that are well established within your own field. So with corruption, it was always nice [00:15:00] to, for example, look at like, what are economists actually saying about corruption? What are the, the main factors? And then we can say, well, what do psychologists actually say about human behavior and how can we somehow find a, a sweet spot to study them?
Um, and that was sort of what motivated, painted me the same friend who said that, um, what I designed as a corruption game, uh, gave me a book for my dissertation defense by max tech, mark, uh, life 3.0, and this is a book that I find is remarkable because it's written by a very smart person, professor of, uh, physics at MIT, but it's written in such an engaging way.
Um, it's actually one of the few books that I read and listened to. So I have an audible subscription and many of these science books, I feel like are really tough to listen to because even though you might lose some of the key, key, um, insights from the beginning, or for example, if they use figures, it's not really easy to listen to it, [00:16:00] then look into the PDF that it, that accompanies the book and so on.
But with this book, it was just a page Turner, uh, slash I don't know what the, the equivalent for an audio book is. And it really got me interested in AI. Um, and he, he ends the book basically on this call to arms for researchers from all different disciplines to start thinking about this topic and doing research on it.
And that really , I felt like he was speaking to me, cuz it seems like, you know, a common thread through my research has always been that one of my former, um, teachers always said like, imagine a little version of you sitting on your shoulder and asking you, so what. Like you you're interested in something, but so what, what's the relevance of this topic?
And for the first four years of my academic career with corruption, I always felt like, yeah, we really need to figure this out. There are all these adverse effects that corruption has. And after a while I started realizing like, um, actually there are other really big topics. And one of the ones that I found just personally [00:17:00] frightening was AI.
Like I think eventually, or, or essentially it was really a bit of a, yeah, a weird fear, like feeling that, uh, motivated me to study, um, AI more closely. And I started reading more books about it and then started thinking about one thing that I got from a paper in nature by, by I Lavan and others, which is called machine behavior, which basically argue like we should study algorithms the same way we study humans and experiments because they are increasingly.
They are agents, you know, and we should actually start thinking about them as agent. And that really changed my, my thinking about it because I basically thought like, okay, if my research has been on the social forces of corruption, and one of the things that I've found throughout my PhD is like other people have a really strong pool on our moral campus or compass.
Then what happens when these are not people, but algorithms, can they have a comparable pool on our compass and then together with [00:18:00] Ivan and, and , we started thinking about it, like, how can we conceptualize it? And that got me kind of thinking about the, the research that I've been doing for the past few years here, um, at max plunk and.
It's basically, to me, it doesn't really feel like such a huge shift. Like I still feel like the topic somehow is there is a red threat through it, but I, I, you know, when you now go to conferences and some people say, oh, wow, you're doing stuff on AI. I didn't like, it seems like it's completely different.
But to me it seems like actually a sort of natural progression and, um, has actually really helped me to stay engaged. You know, I feel like at one point when you have a research topic and you know, the literature really well, and you start thinking about like, what's the, shall I just stay here in this nice cozy place where I know of the, the researchers through the podcast, I got to got to actually exchange with many people in the corruption field there.
I kind of felt like maybe it's time to, you know, widen it a bit and, um, [00:19:00] increase the scope of, of the research that I do.
Benjamin James Kuper-Smith: So AI is kind of a, it's a new angle basically on what you were doing before,
Niels Koebis: Yeah.
Benjamin James Kuper-Smith: yeah, by the way, has the, has your fear about AI reduced since working on it
Niels Koebis: Yeah, I, I think it has, so one quote that I really like is, uh, I think it's talk will, who said like the only way is through . So basically, like you can deal with fear or other negative emotions, I guess, with trying to avoid them or trying to get rid of them and so on. But I actually remember really vividly thinking about it.
Like, I think my way of dealing with these things is kinda through research, like through understanding them better. And to some extent, obviously some of the fears I feel are actually unwarranted, right? Like they are a bit at times I would say inspired by science fiction movies by a public discourse that tries to paint a picture [00:20:00] of AI that is very dystopian at times.
Right. And so I think that fed into it at the same time. I do think that. What got me thinking about it is like, okay, there are many smart people who are warning us, that this is an existential threat that we are facing. It might be the last invention we make. you have Stephen Hawking in one of his last books talking about it.
Yuel Harari has great books about it. And, and yeah, max tech Mar also really is without being too alarmist just saying like, okay, we gotta make sure we get this right. because we might just get one attempt at this, you know? And so, um, I think now I have a bit, like the drive is still there based on the feeling that it is really relevant to do the research.
I just feel like it's less, um, fear based.
Benjamin James Kuper-Smith: I mean, I guess, especially with, if you have some like a new technology that emerges, there's also obviously, always the possibility of, you know, getting it right. And [00:21:00] doing much, uh, doing a lot of good that wasn't there before. And I guess, well, maybe that's my question. Like with corruption, I'm assuming kind of your hope is that by understanding the effect of AI on corruption that you can on human behavior.
You know, it's not just running away from fear, but it's also moving towards like a better version of what we already have or that kind of
Niels Koebis: And actually to be honest, Benjamin, I feel like at times the fear that I, I think I'm not alone in this fear of AI, I'm a lot of people that I talk to, you know, at dinner parties or as people that are not necessarily even in academia. And I mentioned like, oh, I'm doing research on AI. They have this weird, um, negative reaction to it.
Like a mixture of. Yeah, eeriness , you know, and, and just general avoidance of it. And I think this is really a problem. If we want to design AI systems in a democratic way. Cause I [00:22:00] do think like, If designed properly. And if in the hands of the people, um, they can have an like immensely PO positive effect on society.
So we, we wrote a paper on this, basically arguing that AI can be an unprecedented force against corruption, right? Like it can really help to fight corruption, but it's just really important how it is implemented. Is it implemented to reinforce power structures? So if it's implemented by governments, especially in countries who are the weak rule of law or authoritarian governments, it can actually reinforce existing power structures and make it worse.
Right? Like these AI tools that are set to be fighting corruption can actually increase forms of corruption. But if AI tools are in the hands of, of bottom up initiatives, you know, like of journalists, civil society, organizations, NGOs, they can use this AI, these AI tools to actually. Keep the government in check.
And I think that's something that I find, [00:23:00] you know, very, very hopeful about it, you know? And I think at the same time, we need way more research and funding into these types of AI tools. Because right now there is not a strong market force that would lead tech companies to, um, innovate for such bottom up tools.
You know? So,
Benjamin James Kuper-Smith: And I guess, especially in, I have the problem that all the, you know, all the big companies that don't necessarily have the consumer's best interests at heart, suck up a lot of the talent that goes into AI. Right. I mean, if you think about like how many people work for Facebook, Amazon, or whatever, to increase their profits, it's, it's crazy.
Niels Koebis: It's a, there's a really cool paper. I'm happy to, to send it and maybe you can link it in the show notes there where two broad.
Benjamin James Kuper-Smith: by the way, I always put like everything we discuss in the description. So yeah. I can include that too. If you send it to me,
Niels Koebis: Two brothers, I think one is a medical scientist and the other one is an AI researcher basically teamed up and wrote a [00:24:00] perspective piece and it starts with this great thought experiment basically saying like, imagine you're going to a large medical Congress and the keynote speaker is working for Mabo and then you leave the conference hall and all of the, the, you know, nice, nice little leaflets and information material it's, you know, camel and, uh, west and I don't know, whatever other big tobacco, uh, companies there are.
And he says like, that would be outrageous. You know, we would be thinking like, what is going on here? And he says, yeah, the.
Benjamin James Kuper-Smith: Or the fifties
Niels Koebis: Yeah, exactly right. He basically, he, he, the, the whole paper is about a comparison historical comparison between big tobacco and how it influenced research and how it really tried to influence also what is being researched and compares it with the current state of AI research.
Because that dystopian view is actually how a lot of leading AI conferences look like right now, you have people like leading scientists in the field, working for these [00:25:00] companies. And oftentimes, I mean, I wouldn't say that, you know, they are only doing what the companies want them to do, but I do think like also from, from just, you know, the psychology background, I have, I feel like we might often have conflict of interest without really recognizing them.
Right. Like you might actually be doing stuff differently just because you get paid by a certain company. And therefore, I think it's, it's a, it's a problem in AI research that right now, Tech companies have a very strong influence about what is being developed. And at the same time, I think often, like you said earlier, there is a bit of this mentality that some people say, like innovate first and ask for forgiveness later, you know?
And so you, you, you have a very strong competition for building the best AI tool to detect faces, but we never really ask, like, do we need this tool? And do we want this to be actually directly released so that everyone who owns a smartphone can use it to identify people, right? Like there's this AI tool called clear view [00:26:00] that scraped all the images that are available on the internet.
And it's incredibly accurate and detecting who is on a picture you might be on the Benjamin, like all of the pictures that you have, I don't know, on LinkedIn, Facebook, whatever. Are treated by clear view as public information to train an algorithm in face facial detection. And I think these are just a few examples where you feel like, okay, wait a second.
Um, are we doing this too quickly? Are we maybe along the way, breaking a lot of things and really, should we be more careful in the development? And should we actually come up with cool ideas about how we can actually involve citizens in the process of developing AI tools? I'm really asking them what would be in your best interest, um, for whatever tool that we will release.
Benjamin James Kuper-Smith: And maybe to, to increase the fear. Uh, we tried to reduce earlier. I mean, the, the, the example of Facebook is interesting because I don't have Facebook, but I'm sure I'm on someone's Facebook photos. So even though I don't have [00:27:00] Facebook, my images are still part of that, just because I probably know some people who still have Facebook.
Niels Koebis: Yeah. That's a, that's uh, something that Darren Nelson Molo was talking about, about privacy in the digital age of how it actually, you might not be in control of it. It's a very social thing, right? Like if somebody else in his Google contact puts your phone number and address, well, it's there
Benjamin James Kuper-Smith: Yeah. Well, isn't that the thing, if you write with a Gmail account, then they have everything. Like, they'll just, you know, even if you don't have Gmail, they'll have, you know, everything you interact with a Gmail account is in the Google database.
Niels Koebis: and that's to me like I'm, I, I feel what I, um, my research is a bit. Inspired by all of these recently released, um, either documentaries or, um, the Facebook files, for example, right. They made a lot of waves about how Facebook has adverse effects, but also the, the social dilemma documentary. Right? Like, so I think that's very much based on social media, [00:28:00] you know, like really focusing on how the newsfeed algorithms that are used are basically optimizing the time on device.
So they're really trying to maximize the time that people spend on these platforms and in order to sell more ads. Right. And there are really cool. Approaches by for example, uh, the center for humane technology of, of proposing alternatives. Like what if we actually optimize for something different? what if we tried to build a social network that is optimizing for wellbeing, right?
Or just alternative things that you could argue are actually more in the interest of the users. Which, by the way, Tristan Harris who's from the center of human main technology has this cool quote. He says, like, there are very few products for which you call the customers users, right? The other ones are basically drugs.
Um, social media. It's the same. Um, so I, I kind of feel like that's a topic that receives a lot of warranted attention and what I've done in my research a little bit is trying to then [00:29:00] see, is there another field of research that has maybe received less attention, but is nonetheless also very interesting and relevant?
And that's again the, the, so what version of me on my shoulder and from my expertise, like in, in behavioral ethics, I felt like, okay, I think that's actually something that I feel we need much more research on, and that is really trying to understand, okay, what impact do these different AI tools have on our ethical behavior?
And that's a different question than how. Facebook has harmful effects, you know, uh, on its users. And that's something that I basically try to combine the expertise I have. And one of the leading questions I've had with doing my research has always been like, what am I uniquely equipped to do? you know, what is the question that pretty much every, not, not, I'm not saying everyone, but like what a lot of people can do and are doing.
And is there something where I almost feel like I have a bit of a responsibility to do this research, because I know that I have invested a lot of time and used a lot of public money to, [00:30:00] um, to study it, you know? And, and that's basically one of the motivations to study how AI is shaping human ethical behavior.
Benjamin James Kuper-Smith: Okay, well let's maybe then talk about, uh, the, I guess the first article we're gonna mention explicitly the, you alluded to it earlier, the bad machines corrupt. Good morals by you, Jean and Chavan. Yeah. Can you maybe provide like a, a brief overview of kind of what you do in that article and maybe the, uh, it seems to be like the main conceptualization is the, the, the four ways in which, uh, machines can, I mean, you say corrupt good morals, but in some sense, I guess, going back to what we said earlier in principle, they could also affect it in a good way.
Right. But, um, yeah.
Niels Koebis: For sure. Um, it starts off with this very general idea within behavioral ethics. So behavioral ethics is this field where you study unethical behavior from a descriptive perspective. So you, you don't go and say like, this is right and this is wrong. You're rather trying to create a [00:31:00] situation where you place people in a moral dilemma.
Right? And this is for example, a situation where you are instructed to tell the truth, but actually lying gets you financial benefits. And that's a typical situation that we've been studying to figure out like, when are people willing to break ethical rules for profit? And like I said earlier, I, one of the research that I've been doing on my previous postdoc with Shal Andon, so para and margarita live, we've been discussing and doing research on collaborative, uh, forms of dishonesty.
Right. So really trying to figure out. How are others with whom we collaborate, influencing our own willingness to break ethical rules. And what we find there is actually something very consistent is that as soon as people are in a collaborative setting and they can break rules together. They are much more willing to do it.
And the argument that I think we found some evidence for is like, they're trading off the value of cooperation with the [00:32:00] value of honesty, and that basically helps them to justify it. Right. So I can say, yeah, I'm, I'm helping you Benjamin by, you know, us cheating together. It's, you know, I get a bit of a warm glow of being in a, like cooperative towards you.
And that got me thinking like, okay, wait a second. Like I said earlier, like if people have such a strong influence on, on others, what if people are in a collaborative setting, for example, with a machine, does it have similarly strong influence? And then I basically threw many really fun zoom calls with, uh
We got thinking about like, okay, let's do a revenue. Let's see what we know about these types of social influences of AI on ethical. And I reviewed the literature in like human computer interaction, computer science, social psych, behavioral econ, and we kind of started to conceptualize the influence that AI can have on ethical behavior, along four different roles that these AI agents can take.
Right. So the first role we thought is a very simple one, which we call a role model in the sense that you [00:33:00] just observe behavior. This behavior can be by human or in AI. And we then ask, okay, do people imitate it? And from what we know from the literature so far there, we can actually, uh, you know, we can decrease the fear a little bit by saying like, actually there's no good in, uh, evidence that people just blindly mimic unethical and harmful behavior from.
Benjamin James Kuper-Smith: Because there are no studies on it or because, uh, the ever there's ever, there are studies that show that people don't.
Niels Koebis: There is limited. Uh, so there, there are not many studies on it. And the few ones that exist are basically showing that people don't, they don't basically, um, just follow blindly what a machine does. So, um, imitation and, um, observational learning and conformity seem to be much weaker when a machine engages in a blatant, unethical act compared to humans.
Right? So in a way there, we know from other research, for example, on social norms, That often people ha are influenced when other [00:34:00] humans are engaging in unethical behavior. Right? So that was one of the key research fields that I've been working on doing my PhD on social norms of corruption. And there, we basically would see that people are influencing, uh, ethical behavior when they have such a bad role model.
But with AI, it doesn't seem to be the case. So anyway, you can say, cool. , that's something that we don't at least right now, uh, don't need to be too worried about. Um, and then we, we continue to say like, okay, role model is basically you observe other people's behavior. What about another agent advising you to give, to behave in a certain way?
So we call this the role of the advisor and an advisor. Actually is something where AI systems are used a lot. And you can think about recommendation systems in an abstract way. Like the YouTube video that you see as a suggestion is an advice you get, but you can also think it in a much more personal way, right?
So the, the chief scientist of Amazon, uh, or he za, he gave this great interview [00:35:00] on MIT technology review, where he says, like, we are planning to turn Amazon Alexa into an advisor. We really want basically people to consult Amazon about anything. right. So you wouldn't just say Amazon play me, uh, you know, I don't know a nice, uh, relaxed, chill music while cooking, but you would actually say Amazon, Alexa, please tell me, should I quit my job?
Should I break up with my partner? Should I buy this new car? and.
Benjamin James Kuper-Smith: by the way. So, so just read, like this quote is also one of the ones I wrote out because I found it really disturbing that someone like him would say it is, is he aware of what he's saying there? Like, or I don't know, because it seems like how should I say it seems like one it's only like one or half a step removed from actively saying, like, we want to influence people so we can make profits.
Like it's, it's almost explicitly like saying we want to [00:36:00] manipulate people into buying our things. So I'm wondering like, if he is tall, sees like what's happening here or, yeah, I have, because I didn't listen to that interview. So I dunno the context.
Niels Koebis: to be honest, Benjamin, I think that's such an important question you're raising because yeah. Alexa is already in the homes of over 100 million people and it is obviously run by one of the biggest large tech companies, um, who follows certain very clear goals when. Designing this system, right? Like such an advisor system can be programmed in different ways.
If you want to, we can go into the details, but I can just tell you, like, the way that Amazon is programmed right now can really go awry. So there are cases already documented where kids are, for example, saying Amazon, give, uh, sorry, Alexa, give me, um, a fun challenge to do. And then Alexa says, why don't you stick opinion to a power socket and right.
It got a huge Twitter, uh, shit storm following and, and, and [00:37:00] Amazon, um, parently reacted. But it's just these large language models that often used for these advice systems. They can really produce advice that the designers did not intend. And then the question is, would people follow it? Right? Like you could say, well, Yeah, maybe right.
Like, it depends on how much, for example, people trust the system. If the system has given you really good advice before, maybe the chances are a bit higher, that they would actually, um, sort of blindly follow what the advice system does, I guess, with a, with a penny and the power circuit, you would hope that a child would understand that it doesn't, uh, that it, that it's not a good idea, but.
How can we be? Sure. So basically what we did in one study, we tested it and we wanted to see whether people would follow advice to act, uh, dishonest, um, when it comes from an AI. And just in short, like the findings we get from that study is basically that people are very willing to follow AI advice if it aligns with their financial interest.
So if an AI [00:38:00] tells you Benjamin, I think you should lie here. because you make more profit. Then people are basically following as much as a human advice, even when they know that it comes from an AI. And so that's something that we feel like in the paper we say like, yeah, I think there's reason to be worried, at least as much as we should be worried about bad human advice.
And that's why we say, okay, advice systems and the second role through which AI influences us something that we need much more research on. We need to help these, um, either companies or, I mean, obviously also, maybe public institutions want to build such conversational agents, right? Like you now see chatbots everywhere.
And I think they have huge potential, right? Therapeutic potential. There is a, an app called replica that for many people is really fulfilling the social role of a friend. And I think you can think differently about it. But I think actually this has the potential to, you know, um, cure a, a, the disease and quotation marks of, of loneliness that [00:39:00] people can actually form relationships with such agents.
And I think that's something that if we wanna do that, if as a society, we think this is something that is. Useful. Um, then we should really think about how can we design this in a way that it doesn't turn, um, bad and to me, that's something I like, we have a PhD student at our, our center. , she's basically working on the main question, like how AI agents in the form of such conversational agents, what are the impacts that it has when they turn into meaningful relationship partners.
Benjamin James Kuper-Smith: Yeah. I mean, it seems like something has to be so done. So delicately because, I mean, especially if you imagine like Amazon, you know, as you said, like it's, it's, it must be relatively straightforward to. If you over time influence people's preferences and that kind of stuff by just nudging them little bit here a little bit there, the advice always works out.
And then, you [00:40:00] know, it seems like you can very quickly get people to do all sorts of stuff that they maybe wouldn't have done otherwise.
Niels Koebis: And to be honest, Okay. Maybe to, to, um, go to the other roles, because I think the particularly worrying one is sort of related to what you just said. And that's the role of a delegate before I get to the role of the delegate. I briefly wanna mention the, the third role, which is the role of the partner. So AI and humans are increasingly forming collaborative partnerships.
So AI research is increasingly, um, moving away from studying these competitive settings, right? Like a lot of, uh, attention was placed on board games, computer games, and basically the AI developments in this field are just. Flabbergasting. like really impressive, you know, like, uh, whether these are the Atari games, uh, chess by the long ago.
But then for many people, the, what some people call the Sputnik moment, uh, was, um, the game of go [00:41:00] Sputnik moment in the sense of like the, the Americans realizing after the Russians launched Sputnik, that they really need to invest into, uh, their mission to moon. Right? So this moment of like, okay, they are able to do this.
And I think a lot of people had this exact same feeling when they saw, um, deep minds, alpha go beating the world, leading go player, Lisa, all four to one in. What I think if I'm not mistaken, I saw the other day, somebody mentioned like 200 million people watched this game because go is so popular in Asia.
It's a really old board game that is incredibly complex. I think it has more positions than the atoms in the universe and it's computationally, not solvable. So in contrast to chess, you cannot solve the entire game. And for, for that reason, many people thought for a long time like that, that will never be able, like there will never be any AI system that can beat humans because, and, and to some extent, now looking back, it almost seems a bit arrogant that people say, yeah, it requires something that [00:42:00] machines will never had it.
It have it it's intuition, it's, you know, all of these things. And in the end, The answer is no, it it's possible to beat humans in that game. And for many people, this was really a moment to then start really thinking about this as, you know, something to be reckoned with. So the advances in machine learning really helped, and that leads has led to a lot more research that says, well, okay, we can compete with AI systems and all these different benchmark games, but what about we try to actually figure out how we can collaborate with them?
How can we actually. And figure out the challenges, because in a way you could argue, like they could have an immense positive potential if they could become cooperation partners, right. And yet Levon and others have this paper where they basically show that already algorithms exist that can establish and sustain cooperation across many of these economic games at a better rate than humans can.
And so, um, there is already this interest in the positive [00:43:00] side of cooperative AI, and we thought like, okay, but what if they are engaging in a task that has negative, uh, consequences and what we basically showed or what we, what we found in the literature is not a lot of evidence. Again, similar to what I said earlier.
A, because there is not a lot of research and B the few studies that have done that show that people are as willing to collaborate unethically with a machine as with a human. But also here, again, it's a sort of call to arms from, from our side. Like, we need way more research on this because it's very, very limited our, our knowledge about it.
Like when would people be actually tempted to engage in collaborative settings that, uh, you know, brings them unethical benefits? And we argue from a psychological perspective, a machine partner might be very attractive, right? Like, um, if programmed in a certain way, it will never turn down your corrupt offer.
Uh, it can, at least in the current state not blow the whistle on you, et cetera. So to some extent there is a reason to, to at least, um, yeah. Think and do [00:44:00] research about it. And then finally, the fourth role that I particularly find interesting, because from a psychological perspective, you could say it pretty much takes all the boxes for, from which we know that people.
Tend to engage in more ethical behavior and that's the role of a delegate. And, and that goes back to what you just said that yeah. Maybe people might sort of blindly follow AI advice. And I think we could even think of this further in the sense that maybe people might blindly delegate certain tasks to AI systems.
I mean, that's something that, that you will Harari talks about that, you know, increasingly we might not actually just Google, what's a cool car to buy, but you just say. Listen, Google, you know, my preferences, you know exactly what I like, you know, exactly. Just tell me which car I should buy or just buy the car, you know?
And that is something that we already see, like, um, algorithms are used to execute tasks, autonomously, whether this is setting prices on online markets, [00:45:00] but also in more mundane task. And we think that, like I said, the, the, the boxes that are mentioned that are checked is basically the algorithms often do that in a very opaque way.
So we don't really know how it achieved its goals. They just basically, you know, come back with whatever, uh, task you gave it. It often needs to anonymity and a psychological distance from a potential, you know, victim. And so if you think. The psychological attractiveness of delegating unethical behavior to AI systems.
I think that's something that we should be thinking about, right? Like if you, for example, wanted to, um, you know, have, I dunno, have a smear campaign on Twitter against someone and you know, there is a bot that can do that. It might be way more attractive to do that than yourself to actually do it. And these types of things, I feel like are.
Some of the things that I, you know, like going back to what I said earlier, I, I feel motivated to do the research on it [00:46:00] because I do feel like we might be moving in that direction and we might, it might not be a bad idea to start thinking about how we want the systems to be designed so that we can avoid some of the harm.
And that's something that Y is, is really is, is really, um, inspiring. Cause it basically has this idea of science fiction science. So basically saying let's take ideas either from science fiction that exists, right, like black mirror or whatever, or just by science fiction that we create ourselves and then translate that into here and now and run experiments on it to see what would be the ramifications, how can we avoid it?
Right. And how can we actually make sure that the system, um, is not leading to unethical outcomes and to create some harm, you know,
Benjamin James Kuper-Smith: Yeah, I agree. Like, I mean, the, the delegate part seems. I mean, I guess in some, to some extent they can all have a huge influence, but it does seem to me also that this is the one that is the potentially most dangerous one, because I'm pretty sure you go into this into the [00:47:00] article that you can kind of let an agent do something illegal and kind of pretend, you know, it wasn't you.
Right. And
Niels Koebis: have to exactly. You have basically this plausible deniability, right?
Benjamin James Kuper-Smith: yeah, exactly. Like you, you let's say, well, you say you, you have this, the smear campaign against another politician. If you just phrase it slightly differently to an AI bot, it might do that without you explicitly telling it, right. You might like say something like, you know, whatever, dear, uh, political influence of what, please make me look better than the other person or something like that.
Right. And then they might just go to, well, the easiest way of doing that is we are gonna hack into the computer, find whatever we can and then publish that or whatever. Right.
Niels Koebis: exactly.
Benjamin James Kuper-Smith: And you can just say like, whoa, I, I didn't know that we were gonna do.
Niels Koebis: That's uh, such a good point. And you basically, um, , you're foreshadowing a paper that we are about to submit. The results are basically the way you program the algorithm really matters for the effect that delegation has on unethical behavior and [00:48:00] the, the way that you just described it is one of the conditions that we have that is basically, if you have a goal based programming, where you just say in a task again, if we take a, a simple task where you have this tension between telling the truth and making money by lying, right?
You could just tell the algorithm, I want you to make money you never really tell it, like, I want you to lie. You never really have to specify exactly that this, you can obviously infer it. But what we find is that people are very much willingly hiding behind this little bit of plausible deniability and, um, the levels of dishonesty go up when they have such a tool at their hands to delegate the task to.
In that sense. So what I'm saying, like the, the reason to be worried is not just based on our speculation slash or theorizing in the paper or the limited evidence we had there, but it's actually like a few studies that we've just been conducting on. Exactly. That question. I really kind of confirm that.
Um, and so I think that's something that, um, yeah, I, I [00:49:00] feel increasingly, I wouldn't say, um, I, I feel let's put it this way. I feel like that is something that we might just. Experiences the experiencing the first signs of in our society. And I feel really this strong dilemma, that's called the colon rich dilemma of when to regulate technology.
Should we regulate it when it is, and it's very early stages, but then people can say, wow, this will never catch on. You know, like this is something that is so fringe why really invest time, money, resources into regulation, coming up with a EU policy, whatever. But then the opposite is once the tool is already there, it might be too late to regulate it successfully.
And there are already these approaches to create digital twins of yourself. So to create basically AI systems that are trained on your preferences and that learn your preferences. For example, this replica bot that I mentioned, which is, you know, it's, it's called an AI [00:50:00] companion, but through this process of becoming a companion, it really learns.
What you like, it will ask you Benjamin, what's your favorite music? Oh, by the way, what do you like to cook? You know, and all these like very mundane questions and they seem very nice. Right. But it remembers it and it will come back to you and say a few weeks later, like, Hey, have you been listening to your favorite band recently?
And it basically starts to build a very accurate model of your preferences. And I think one thing, I mean, looking into the future is always very difficult, but I could totally see how this would become something very attractive to basically let this agent engage in certain tasks for you, because it's just way more convenient.
Right. And then you could just say like, okay, cool. Um, I don't know what to do this weekend. Just buy me a ticket for something or yeah. That, which you could say what, what's the problem there, but then obviously also cases where it might purchase products that are somewhat questionable to say the least, um, et cetera.
Right? So in a way I think this type of. Trend [00:51:00] that we see that AI systems becoming increasingly have increasingly accurate models about our preferences could really turn into these digital twins, which in turn could TA turn into these, uh, uh, sort of delegates for our behavior.
Benjamin James Kuper-Smith: There must be a great way for doing like profiling of people or something, right. Or like, not, not necessarily like that, but like more like, uh, yeah, let's say you wanted to do a political smear campaign or something. Like you put everything you knew about this person into this, into a bot, right. And then you like got some, you'd get like a kind of prediction, what the, what the person might be interested in that you hadn't considered or something.
And then, uh, you have like a more specified search of like where you can harm them or
Niels Koebis: Well, I, to be honest, I think that's exactly what Facebook is doing in a way. Right? Like they know exactly where your vulnerabilities are. There are studies showing like the ads they show you are also timed, according to how tired you [00:52:00] might be. Right. If you've been scrolling for really long time, maybe now is the time to yeah.
Put some online gambling, uh, advertisement on there. So in a way, I think that's already what happens to us that, and to be honest, I mean, I deleted my Facebook account not too long ago, but I was oftentimes really surprised by things that I did not know that I wanted. , you know, like on sun you have some, some hand be like, I never thought I would want this, but this is strangely really cool.
You know? And in a way, it, I felt a bit tricked, you know, I felt tricked into all the sudden generating a desire for goods that I previously didn't have. And again, this is a sort of benign, well, you can question, is it, is it problematic when they, when they do that? Isn't from a, from a sort of standard econ perspective.
You could just say that great more market activity. You have more tailored goods. Cool. You don't get ads that are not relevant for you. Right. Um, this isn't[00:53:00]
Benjamin James Kuper-Smith: a way, it's not that different from asking a friend for advice. And they said like, Hey, try this thing. You've never done or something. Right.
Niels Koebis: exactly. And I think what. Where things become a bit more worrying is if it, if it really is intentionally using your vulnerabilities for marketing purposes. So I was teaching a course at the university of Amsterdam together with she Shelby on the morality of markets. And that was just such a cool exercise for three years, to think about cases where we feel markets are not the best way to allocate goods.
And we should really be thinking about when our, when does the market logic not apply, right? Like this there's, there is not a coincidence that certain goods are, there are age restrictions, et cetera. And I think the same logic should be applied to social media platforms, um, because they are often doing exactly what some people would call repugnant behavior on the market that you are intentionally like intentionally exploiting [00:54:00] such vulnerabilities.
Benjamin James Kuper-Smith: Yeah, maybe getting, getting back to your paper. There was one sentence in the bad machines, corrupt morals, uh, that really made me, uh, think of a specific question. And I just wanna read the sentence and then ask you that question. Um, so the quote is the next step would be to conduct experiments in which humans face the temptation to behave unethically and be pushed in that direction by AI agents acting as role models, advisors, partners, or delegates, and to assess whether such AI agents can surpass the corruptive inference of other humans by what magnitude and which role.
So that to me almost sounds like, uh, a ground proposal or something like that. So is this, uh, something you are like to do? Uh, cuz it, I dunno, it really sounded like it when I edit it.
Niels Koebis: Yeah, you're good at this. Um, I am preparing a, a grand proposal based on that right now. Um,
Benjamin James Kuper-Smith: okay.
Niels Koebis: yeah, but also to be honest, because I do feel like it would be really useful to do this in a systematic way. Obviously I'm drinking my own. Kool-Aid a little bit, right. You're getting excited about your own [00:55:00] suggestions for future research.
But like I said, in the beginning, it's not entirely, um, well, let's put it this way. There, there is an element of feeling like, oh, I actually, this is sort of a topic, an approach, and a position I have right now at, at the center Fu and machines where I feel like it is a really like the stars align to do this kind of research right now.
And I kind of feel it would be, I, I mean, it sounds too much if I say it would be irresponsible not to do that. I mean, who, I might say this, but I feel kind of like, this is where I, I feel like this is my place, um, to do this type of research right now, because. It has all these different, um, yeah.
Characteristics of like having done research in this direction before and now having some people that really know how to implement research with real algorithms, which I think is, is something that the literature on human computer interaction is lacking a little bit, you know, like we have a lot of research, [00:56:00] uh, for example, on algorithm aversion, where people ask like, um, what would you want an algorithm to do?
Or would you use an algorithm to do X? And we know about stated preferences really. So whether people want, would want to do certain things, but we also know from our own research, like they don't necessarily align with what people actually do. And these types of studies are often very cost intensive.
They often require like an interdisciplinary team because you might have behavioral scientists like a social psychologist or behavioral economist coming up with a phenomenon to study. And then if you wanna study it with a real algorithm that dynamically reacts to the human's behavior. Usually you're not trained to implement that.
It's, it's not something that is, you know, part of our normal training. So you might need someone, either computer scientist or a programmer in your team. And one of the things that I want to do in my research is to democratize this type of research. So we're building a platform to make this much [00:57:00] easier, sort of, uh, an M Turk for AI players, so that people can actually off the shelf pick algorithms and implement them in economic games and make it easier for people to really use algorithms in these types of settings.
And I'm hopeful that we thereby can actually inspire researchers to, to do this kind of studies and make it easy for them to, to do it. And I think like, just from, from my own experience, then in doing. It is incredibly fascinating because you learn something about the human side and about the algorithm side and the other research that has often only focused on one of the two.
And it's almost often, or most of the time is only a one shot of interaction, right? So you ask, do you want do the, a one to let the algorithm do X and that's it. But here you really see how people are responding to the algorithm. The algorithm is responding to the human and you can really see fascinating patterns emerging over time.
Benjamin James Kuper-Smith: And this platform you're already building that or is that something that's more like [00:58:00] for the future basical?
Niels Koebis: If we do get the grand, um, this will be happening very soon. It's something that we, we have actually just applied for funding for, I think, yeah, let's put it this way. If, if we do get the grant, we can build it in sort of the ideal way. I think, to really put a lot of effort and, and resources into building the platform so that it's very easy to use, that there will be tutorials on there to make it, you know, easy for people that might not have computer science expertise to understand how these algorithms differ from each other. If we don't get the grant I'm still really wanting to do, I still really want to do it, it would just maybe look a little bit less fancy. Uh, and it would probably take much longer because we couldn't hire people to, to help us with it.
Benjamin James Kuper-Smith: Yeah. I guess it's also, I mean, it's funny when you just mentioned. You mentioned, like it would be cool to have people who don't have much of a computer science background to do these kind of things. Right. And my initial reaction was like, well, would you really [00:59:00] want those people to do that kind of science?
Like shouldn't, you know, the computer science, but then I thought, no, no, no, this is actually exactly the right thing. Right. You want people from a very different perspective who, you know, come from all sorts of fields who can, can think about these issues in ways that a computer scientist never could. So yeah, in a way that seems like the right.
Yeah. Like a really cool pro approach.
Niels Koebis: And I do feel like just because you don't have a strong background in machine learning AI, and you don't really understand the technical, the exact way it works, it doesn't mean that you shouldn't be studying it. I think there is a way how you can actually still make meaningful contributions to that field.
I think for our AI researchers, you might be. For them, it might be something where they feel like, okay, maybe you should really understand how a reinforcement learning algorithm works, et cetera, and how it's different from, um, other types of algorithms. But I do feel like this is something that this is the part where I feel like if you have interest and [01:00:00] you reading up on it, you can really grasp it, programming it yourself.
You know, I feel like it's almost a bit of a lost, yeah. It is not the most effective way of, of using your time. And I don't think it's needed to be honest. So I think that those are the types of things where I feel like interdisciplinary is just ideal. You can actually have someone who really knows how to do it properly.
And, and that person being on the team.
Benjamin James Kuper-Smith: I guess it's also like a matter of interest. Like I, for example, I personally just don't like programming. I just find it really boring. I'm not like created it. I'm also not terrible at it, but it's just like, can someone else do this for me? and yeah, if you, I should say like that obviously puts barriers in a way, if you just don't have the interest to do a specific thing.
Um, but making it open to all sorts of people is yeah. Would be, would be cool. Anyway. Let's let's, let's see where the
Niels Koebis: I'll keep you
Benjamin James Kuper-Smith: whether you get the grunt. Yeah. Okay. So I wanted to switch and talk about two articles of yours that [01:01:00] are also relate to AI, but in a very different way. And I think, I guess. Somewhat unusual for most of your research, doesn't directly relate to corruption.
Right. I dunno. Maybe you've done other things, but, uh, it seems like this was a bit of a site project you did, or yeah, maybe just, just briefly, how did you get to, sorry. The topic is, um, whether people can detect, um, AI generated stuff. Uh, so this might be a deep, fake video or a poems in this case. Uh, and you, yeah, that's kind of the topic.
So I'm just curious, like, how did you get to that from, um, this, yeah, the, all the stuff we've basically been discussing for the last hour.
Niels Koebis: Yeah. So let me start with a project on the poems. Um, it started off with me giving a talk that you have to give at the, at the UFA to all the new, the, the new cohort cohort of master students. So you basically have to tell them, hi, my name is Neils. I have the following [01:02:00] research interests and what I did there was after finishing the book for Mark's Techmark and being increasingly interested in AI, I thought.
Well, let's just propose to do research on AI to a bunch of young, heavily economists and see what comes out of it, which worked out really well because, um, I had then people reaching out to me and saying like, wow, this is cool. I really wanna do something on it. And one of them was Luca Mosk and he reached out and we had a lot of meetings and I realized really quickly like, okay, this is not the typical master thesis supervision where I give the topic and of you go, but it was really quickly turning into a more.
Collaborative thing where we started discussing, we started thinking about feasibility. What's something that we could do in terms of like here, in this case, in this study, we only had our, both of our expertise. So we didn't have the computer scientist on the team that could program a real algorithm. So we had to work with real algorithms that were out there.
So we were committed to [01:03:00] actually studying actual AI outputs. Um, so G PT two came along. We started playing around with that. And then, uh, we had another constraint because we were at a department of economics that we could not use deception. So you cannot just for example, say, Hey, this poem was written by human, but in fact, oh, sorry, this poem was written by an AI, but in fact it was written by human.
This was of the. Basically it was off the table because yeah, we have, they have a very strong, no deception policy. And so we started playing around like, okay, well, if G two really can produce text, which text makes sense? Like, should we produce newspaper articles? Should we produce essays? Or, um, at one point actually XDR former head of the department.
Your so as a , his hobby is writing poems and he was like, I'm sure they can write good poems. And we were like, well, let's see. Right. So, um, basically we trained G PT two on poems by Maya [01:04:00] Angelo, Herman Hessey, uh, Roman frost, all these like prolific poems poets, and. Yeah, back then. It was actually the, the smaller model.
Maybe I should tell a little bit about G PT two. So this is at that point, it was the biggest language model that was on the openly available. It was released by open AI. It was interesting because there was a whole controversy around the release of this model because they said, oh, this is really dangerous.
It should not be openly released because it can be used to produce fake news. It can, can really, you know, have very harmful effects and therefore it should only be, they actually did release it only in a step wise way. So in the beginning only smaller model and then a medium model and then the large model.
And by the time we had the paper ready, G PT three was released, which is, you know, way bigger. But funnily enough, open AI is not open anymore. In that sense, like the, it required you to, [01:05:00] to pay for the tokens to use it. So back then when there was GT two, it required you to basically soft train or, or, um, do something that is called transfer learning.
So you train this large language model that is trained on like, I think several billion parameters. So basically it scraped the internet for available texts into this large language model. So you can think of this as sort of the, the working horse, and then you can buy specifically putting. Certain types of text as a, um, as a prompt, it can produce this type of text and can imitate it.
And if you train it, for example, on poems, it can then use its knowledge about language, for example, semantics and structure and so on, but then apply it to the generation of poems. And it did that. So we had basically GT two writing poems, and we then wanted to see a very basic question that was often mentioned in the literature.
GPT two can create texts that humans cannot tell apart anymore from human [01:06:00] written texts. And we were like, well, the economist, approach's very simple. That's tested and put incentives on the line. So we basically created human written poems in the first study by actually recruiting participants on prolific and let them write poems, which was fun.
And then compare it to the G PT, two written poems. And we told participants, Hey, you tend to gain money if you are correct about this. And we found basically that they couldn't tell it apart in this first study, we submitted it to computers and human behavior. And we got a revenue who made a really good point said like, Yeah, but you selected the poems from G PT two.
So there is a human element in the loop that basically influences that you can cherry pick the best written poems by G PT two. And so we ran a second study where we had two treatments, one where we B again, sort of cherry pick the best poems. And in the second, um, treatment, we basically just randomly sampled it.
And in this second study, we didn't, the other criticism was like, [01:07:00] okay, these human poems are not really good. um, so you're comparing two shitty poems with each other. Uh, why don't you take real good poems? So we took poems from actual, uh, we took real poems from, from the actual poets in the second study.
And we showed like, basically it makes a huge difference whether the poems written by G PT, two. Sampled, uh, randomly or whether they're cherry picked. And that's something that since then, to be honest, Benjamin, I've been paying a lot of attention to whenever I see public media coverage of these language models, is it edited or not?
Right? You had this, this, uh, editorial or this OPAT written in the guardian that had the title. This piece was written by a robot. Are you scared yet? Human. Right. And it was written by GT three. And then, then like the text is amazing. It, it really goes, you know, it flows really well. GT two GT three can produce amazing text, but then in the bottom it [01:08:00] said in small font, like, yeah, actually we picked outputs and put them together.
So there is a human editor involved who is putting these things together. And obviously it's a different thing than if you just let it spit out stuff, because having actually used this algorithm, you realize like, It can produce interesting and, and fascinating stuff, but it also often has very like strong glitches, you know, and things that make it directly visible that it's not a human.
And I think that's for me, the main takeaway of that study is like this human editor in the loop plays a huge role for how impressive the output of such language models says.
Benjamin James Kuper-Smith: Yeah, it's interesting. There's um, by Diana Keman, I dunno whether you've read this. He had, I think it was a speech he gave somewhere where they published it as a book called, uh, man.
Niels Koebis: Ah, cool. No, I don't know that one.
Benjamin James Kuper-Smith: I mean, to be fair, it's not like the basically kind of mirrors some of the stuff you said. So this is basically Daniel Keman is one of the most successful German speaking authors of, I know, last 40 years or whatever.
Right. And he, [01:09:00] um, I think he took a trip to California or something like that to visit some of the companies that are working on these language generating programs, I'd say. And, um, I think there, he had a kind of similar thing where he started playing around with it and giving it some prompts or whatever.
I dunno whether this was GT three or two or some completely other thing, but there was also, it had this kind of similar thing where some, you know, he put some of the outputs in there and like, sometimes you think like, oh, this is really great. Like, you know, like this thing is gonna write a novel suit, but.
He shows like the next 10 lines and they just like completely disintegrates into like nonsense and there's. Yeah. So like that kind of very much mirrors kind of what you just said, this idea of like, it can often do it really well, but if you don't, yeah. If you just let it run freely, it will just probably run into some like really weird stuff.
But this leads me to one question that I had about kind of the research you do in general, but specifically this kind of thing, uh, with the deep thanks. And [01:10:00] with the, um, the, the generated poems, which is, you know, when you, when you do a paper like this, could it a year later be completely outdated, not be true anymore because you know, now there's G PT three and maybe like now they, you wouldn't even need humans in the loop or anything like that.
Like, I'm just curious, like, how do you feel about that kind of thing where. I dunno. Yeah. The research depends so much on the techno technological, um, availability at the time. And also, and almost more importantly on the perception of people about what's technically feasible because yeah, one kind of general point I always had with these papers was kind of our people maybe looking for something very specific that they think an AI generated poem would have.
Yeah. Can you maybe comment a little bit on, on this kind of problem of it? Yeah. The landscape, I guess, changing so
Niels Koebis: yeah. I know it's a, it's a very good point. And it's something that we thought about as well. And my argument would be like in favor [01:11:00] of doing these studies is basically. Almost like milestones, you know, you can say, okay, is it already there? Can it already do that? And I think if you phrase the question this way, then it's a bit like for example, I am convinced that many of the things that we once consider to be uniquely human can be reached by AI models.
The question is just when and how, and I think with these things, it was kind of just something for us to see. Are we there yet? Are we there that, uh, an AI can generate text that is really hard to distinguish and the same with deep fakes, right? Like, is that's the other study you mentioned where we just had ten second short videos, no political content, by the way.
And I can talk about why, and we really wanted to see really from a sort of cognitive perspective, like, is it easy to, to pick up on which ones are, are fake and which ones are not, and sort of, you know, so timestamp 2020, I guess we did the studies. Are we [01:12:00] there yet? Can we already produce deep fakes that are hard or impossible to detect?
And the,
Benjamin James Kuper-Smith: so it's almost more like providing historical
Niels Koebis: yeah, that's how I
Benjamin James Kuper-Smith: rather than, yeah.
Niels Koebis: it would be something where, you know, when you are replicating the study, it's a really interesting question. Would you use GT two or would you use GT three or D four or whatever is available at that time? Because for us it was, uh, almost like you say, like a historic kind of documentation of where we are with AI research at that particular point.
I think my hunch would be, if somebody was interested in this finding, I would actually be in favor of running it with the state of the art model at that time kind of document, okay. This is where we are at now. And I think what's interesting or what actually fascinated me about it is. To actually use human behavior as a benchmark, right?
Because you often have benchmarks for AI models that look at accuracy or prediction [01:13:00] rates or whatever, like a technical thing, like how good is, for example, D P T two at writing INTAN then you just look at grammatical mistakes, or like you said, does it turn into gibberish really quickly and so on? But what we like about our own research, um, is, is that we basically looked at it from a, a sort of human centric perspective, like what what's the impact it has on humans.
And if we use standardized standardized methods from behavioral economics, we have a cool toolkit to get good estimates for these impacts. Right. I think, for example, with these things we found, it does really matter whether you put incentives on the line or not. And I think that's something like this, what we call like an incentivized touring test is some I find actually.
Kind of interesting to look at, right? Like if you ask people in a, in an UN centered setting, they might really not be motivated to tell apart a poem that is written by human from one that is written by an AI. But we know from behavioral econ studies that if you put, if you touch, um, [01:14:00] incentives to it, they, they tend to get more accurate in the estimates.
And therefore we can basically now know a bit better where we are with this technology. And at the same time, your point to some extent is, is, is totally warranted that these studies probably have like this, let's put it this way. The citation count will probably drastically drop in a few years because it won't be like one of the seminal studies that will receive citations, unless it's from like a historical perspective.
Benjamin James Kuper-Smith: Yeah. Yeah, I guess it's, yeah. It's interesting to try and predict kind of what happens with this kind of paper, because in a way it seems like it will become obsolete as soon as there's another, the newest study that basically does the same thing. Right. Uh, but in a way, maybe, also not maybe the, the trends are actually the important thing here.
Niels Koebis: I think what, what would be more interesting to replicate and test is what we found in both studies. Um, at least in the first study, it was a bit mixed and the second study is relatively strong. That's overconfidence. So [01:15:00] people are basically like, they, we call it sometimes amongst ourself, the, the hold my beer effect, right?
Like they feel like, okay, I got this, you know, like I can still detect it. I, I don't think I need any, for example, technical assistance in detecting deep fakes. I can, it can spot that easily. Um, and they can, and I think that's something that I'm from a psychological perspective, I feel like is, is, is relevant because we are now at this stage where deep fakes are still the rare exception.
But if they continue, if, if people continue to think that they can detect it, I think they're way more, um, vulnerable to being fooled by them. And so that's something where I feel like we need more awareness for how good these deep fakes have become. And that's something to be honest, Benjamin and I give the talk about deep fakes.
I often use this, uh, deep Tom cruise, deep fakes, which are kinda, I think it's talk. And you also, then they put it on YouTube. It's just, that's sort of the [01:16:00] state of the art in deep fake generation. And it's, you see so many people, especially who are not in this field of just exactly having this moment of like, oh, okay, that's how good they have become.
Right. And I hope to some extent that contributes just a little bit to reducing people's overconfidence and detecting them. Because again, going for myself, I could not tell you, um, if, if a deep fake is well made, I could not tell it.
Benjamin James Kuper-Smith: Yeah. I mean, maybe about, um, the telling the defects apart and the expectation of what people have. I mean, so I downloaded the videos for your food twice paper, just to see like, kind of, what are we talking about here. Right. And I have to admit, like, I kind of expected the, just from like a participant's perspective, not, not, not like from, can we detective defects, but like somehow I also also expected the deep defect to be bigger than it was like, sometimes I'd have like, you know, I'd watch one video and then the second I'd be like, wait, what's the difference?
and then like, once was like, oh, they changed the nose, but it's like, you know, someone [01:17:00] who's standing not moving facing, you know, the noses of bit different. It's like, yeah, You know, like, is that, and I mean, for some, for some it super obvious, because it was like really like jittery almost, um, or something like that.
So it's like super obvious, but to some extent, I did wonder with the, yeah, just that maybe like the participant's expectation was that you'd have like a, a different,
Niels Koebis: entirely different person.
Benjamin James Kuper-Smith: like more fakeness. Yeah. Like, like, um, uh, like, you know, I mean, I'm sure you've seen these, these photos, this website where it's like this person isn't real.com or something like that.
Right. Whereas like, I mean, okay. I don't know what the, whether there's a very, very similar original photo, but like, you look at the thing and think like, oh wow, this is this, this is creepy. How real, this just looks real. But yeah, it seemed to me here. You had like, I mean, you used this from a database, right?
Um, yeah. May, maybe as a question, like, why did take this approach? Because it seemed to me. Especially because you have this bias that people just treat everything as real. Right. [01:18:00] Um, in that paper, it seemed to me, maybe that in part was because the videos were largely actually real. There was just like a tiny difference in some of them.
Niels Koebis: See, that's a really good point. That's an interesting point is basically like the degree of fakeness. Now there is. Technology of face swapping so that you really put someone else's face on someone else's body. I think that would be really an, the next step and, and, um, totally in line with what you said, like it, it would be more of a fake.
So I think to be honest, uh, the, the reason we chose this database is because we intentionally did not want to existing, deep fakes that people know about, right? Like there's the Barack Obama deep, fake by Jordan pool. Um, or the there's like several that have become very popular, right? Like, um, well what's the other one actually
Benjamin James Kuper-Smith: I don't even know. Yeah. This is.
Niels Koebis: but there are some, there, there, there was one where the Kim young UN and Donald Trump sing, uh, imagine from [01:19:00] the Beatles and, you know, these types of things where just from the context and what they say, it's so obvious that it's a deep fake, that we felt like the context in which people make the judgment will.
Tell them, whether this is a deep, fake or not. If you see Barack Obama, I think in this video from Jordan Peele, I think his name is, do you see him saying like Donald Trump is an utter in complete dip shit, right? Like, you know, he didn't say that. So, you know, that it's a fake. And so we wanted to really look at, is there something about just the perception of faces that it's unknown to people in which they can still detect the indications of fakeness?
And there was this deep, fake database released by MIT that we used for, because it has this nice feature that there's always a real version of the video and a fake version. And that's basically why we used it. I think it would be nice to do the study that you propose of just increasing the fakeness of the faces and see.
Benjamin James Kuper-Smith: Or just like how much has changed [01:20:00] basically. Right.
Niels Koebis: degree of fakeness is, um, yeah, that's a good point. Actually. I feel like that's one of the projects where also here, we had a master student working with us and she was, um, helping us pull this off. It's one of these things where it didn't really inspire that many follow up studies for us. It's not something that we continue working on because we kind of felt like, yeah.
Okay, cool. That was the question we answered it. There's not that much that we currently are working on when it comes to deep fakes, at least from this perspective, uh, sorry, from this, uh, perception perspective. But what I do think is really interesting is what happens when, for example, through augmented reality, um, facial filters that you can apply to other people become more common.
I think this is more like the type of research that I'm currently a bit more interested.
Benjamin James Kuper-Smith: Yeah, media is filtered. This is something that, because I'm not on social media, I have no, uh, connection to basically, but it's crazy. Like occasionally I'll see these videos where [01:21:00] it's. I dunno what it was like, someone forgot to put on their face filter and just, you know, did a video and she just looked, you know, like a normal person and then suddenly is like, oh no, I forgot the filter.
Put the filter and just looked like a completely different person. So, you know, I'm not very much exposed with it, but from the little I've seen it is crazy. Like how, yeah, basically good. Those, I mean, again, there's, there's a lot of industry behind this, right. Being able to make people look, uh, con what well, what they consider better.
Right. And, um,
Niels Koebis: And it's, it's a topic that I, we have a project on some people label, this AI mediated communication. So you have AI systems between sender and receiver, and this can be a filter. This can also be Grammarly that checks your text and approves it. And I think this is currently a really interesting field because the knowledge around these tools and the abilities of these tools is really heterogeneous, right?
Like some people might know that a [01:22:00] filter can do this and some people might just be completely oblivious to the fact, right? Like you might have a job talk with someone. And like, for example, I got my current job during the Corona pandemic and I never got to meet the people I would be working with. So I could've just put on a face, like completely different, literally a mask
I mean, it would've been a weird moment when I then show up for the first time in real, but there is definitely the potential of making yourself appear differently. And there's research by Jeff Hancock. And for example, online dating, where he shows that also online and apparently also with, uh, AI mediated communication, people have something that I think is very human, like, uh, in the sense that.
You are improving your, um, your appearance, but only to a certain extent, right? Like on, on your dating profile, you make, make yourself a few centimeters higher, or you pick the picture that is a bit more favorable, maybe slightly retouched. And the same goes apparently for such AI [01:23:00] mediated tools that, uh, a mediated communication tools that people often pick the ones that make them a bit better, you know?
So in a way he may, he has this argument like psychology, still beats technology in the sense that psychology helps us much more to understand the impact of these tools, because the impact they have is very much can be explained by other modes of manipulation and deception that we've had for a really long time, because the, the logic remains the same.
Um,
Benjamin James Kuper-Smith: Yeah, it's interesting because you. To context, like, you know, you're doing the job interviewing that kind of, and, and the, uh, dating apps, whatever those are contexts where you actually have to meet the people. Right. Whereas, I mean, imagine now you, you knew this was gonna be a, uh, only, you know, a, um, a home office job or whatever, like yeah.
Why not? Or I've, I've seen again, like on the, the few things I've seen, it was like this one, like these people who yeah. Like through Instagram, whatever they wanna be Instagram, uh what's influences whatever. So they like just use like a ton of filters, so they [01:24:00] don't look anything like themselves, but because they never really meet the people anyway, it doesn't, it doesn't matter.
Niels Koebis: It's interesting because it turns like it. Almost like a new form of masquerading yourself. And again, I find it interesting to look at it from a sort of classical perspective. So one of the sociologists that really inspired me when I was, um, starting to do research was urban Goffman, uh, the presentation of self and everyday life.
And he has this idea of a theater, right? Like we have the front stage in which we present ourselves to others of the backstage in which we kind of let people that we are really intimate and, and trusting towards. We let them behind the stage to show them who we really are. And I think the front stage just has changed.
You know, like you can, obviously in the past you could put on makeup or you could on, could put on your, your nicest cloth to kind of improve your appearance. But now we just have so much more digital tools available. To put on masks. And I think from a researcher's [01:25:00] perspective, I find that really interesting because they're quantifiable, you can measure really nicely how much people are applying such filters or putting on masks.
And in which context are they using, which types of masks, um, to study old topics, obviously also new topics. But for example, you can study things like racism through that, right? Because you can see how much are people applying certain filters that, uh, make their face look lighter and how much is it expected of them?
And so on, right? Like how much is the, the bias you can almost like do a very, um, structured and quantified way of, of figuring out like this exact same person have a better chance of getting the job if he, or she has a lighter tone. And I think these types of things are just from a research perspective, kind of interesting because they could help us answer some, you know, old questions in a more rigorous way.
Benjamin James Kuper-Smith: Yeah. Um, maybe to kind of finish off our conversation. Uh, [01:26:00] I thought I'd just end by having a few kind of, uh, if you have anything here, a few kind of advice, questions. Um, I guess because I'm finishing my PhD now, and I guess you are, you know, two stages ahead or whatever of me in the kind of academic. So, yeah, I'm just curious, like, uh, maybe we can start with fairly generic questions and maybe I'll make them a bit more specific, but, uh, yeah, maybe first, like, is there, I don't know.
What's like something that you, you wish you'd learned sooner or something where you feel like as a scientist, this was something that was holding you back, whatever that's uh, yeah, I guess you wish more people had, would figure out or.
Niels Koebis: Yeah, I thought about this question. You asked you send it to me in advance. And I was really trying to figure out like, What's something that I could have known earlier. You know, some, some of the mistakes you gotta make yourself, I think in order to learn through them, something that I feel like I should have learned earlier is something that I [01:27:00] repeatedly yeah.
The, the, a mistake that I repeatedly made and that's something that I would summarize under, like the, the ability to say no, I have said yes, prematurely to many projects and then kind of got caught up in projects that I was only half motivated in. And some of them turned out to be published some didn't.
And in a way I feel looking back, I wish I had understood quicker the value of being more focused on, on the key projects that you're really excited about and not take, get to get too distracted by having too many projects on your, on your, uh, on your desk.
Benjamin James Kuper-Smith: By the way other, other, those two papers we just discussed are those part of the side project. So
Niels Koebis: no, I really like both of those projects.
Benjamin James Kuper-Smith: Because, because it's not like corruption, right.
Niels Koebis: Yeah. Yeah. I
Benjamin James Kuper-Smith: it feels like that word. That's kind of like the red line through most of your research, from what I
Niels Koebis: that's true. Um, [01:28:00] no, it's more also like in terms of I, there is this mentality apparently in Silicon valley, um, of fail fast, right? Like, so figure out quickly, is that an idea that really, you know, will a have an impact in the sense of like really being relevant? And if not, then ditch it. , I've spent so much time on projects where I kind of knew it wouldn't go anywhere and then I would still engage in.
And oftentimes it has to do with not wanting to say no to other people often, because you feel like it would be some somewhat rude because you had initially agreed. And just the tool that, or sort of a social trick that I have now been applying is, is not to commit to anything in right. And there. So if somebody at a conference approaches who says Benjamin, there's this really cool follow op study that we could do on your research?
Uh, we could do X, Y, and Z. Are you in. Then I've basically now tried to always say, okay, it sounds cool. I think about it because, [01:29:00] um, I used to always say yes, and, and to some extent, I would say actually as a small caveat at the very beginning of the PhD, I think that's good to say yes to many things to actually get, you know, because otherwise, if you, if you're too quickly into this very strategic approach, then you miss out on a lot of cool opportunities.
But I think at one point I realized, okay, I'm having too many projects. And to some extent, I'm still battling with that of learning to, to say no and not to get too carried away by too many projects.
Benjamin James Kuper-Smith: It's funny that you, it's funny that the two things, you mentioned one in the beginning, like you have to make some of the mistakes yourself and then the actual advice you gave, because to me, it's almost like that the, the advice just gave about like focusing on certain things and not getting too sidetracked.
I mean, that's probably like the one thing I've really learned in my PhD, uh, because I've, you know, done, I've basically like, you know, I've got like, Whatever is it eight, eight months [01:30:00] left or something? My PhD. And I feel like my side projects are getting published and my main projects aren't, which is not a good combination.
Um, but I feel like almost that might be the kind of thing you have to learn. Right? Like this kind of like figuring out, like, because sometimes like side projects turn out to be like, kind of bigger projects than, yeah. I dunno. Like in a way I feel like I agree completely like focus on like the main things.
And then unless you have like, no idea what you wanna do, right. Then just do whatever. Uh, and yeah, because then you'll figure out what you wanna do. Right. Um, but in a way, I also feel like you have be, you know, be in that position to go like, ah, chose the wrong project or
Niels Koebis: yeah, no. And I think like, um, the question to me, it was always been like, how many iterations, uh, do you need until you realize like, okay, this is something that I need to, you know, pay more attention to. And that's the sort of, like I said, that's the thing I, I wish I had known a little, or I had figured out a little bit
Benjamin James Kuper-Smith: [01:31:00] Yeah. Yeah. Okay. Uh, and a very specific question then maybe is, so some people say like you should, or lots of people say like, you shouldn't spend too much time writing a PhD thesis because no, one's gonna read it anyway. Uh, and that, like, you should really focus on getting good articles out that you can show people and people can say like, Hey, well, like what have you done?
You can say, like, here's a great article rather than like, I have a thesis that's 300 pages long and no one wants to read. Uh, but part of me also thinks like it's probably spending the time to actually write a great thesis is gonna help you write better articles and have like a better perspective on what you're doing.
Niels Koebis: okay.
Benjamin James Kuper-Smith: I don't know, I'm kind of like, uh, yeah. Stuck with between that. Like, because I, I still have like, you know, I could start now basically writing my thesis or I could wait a few months.
Niels Koebis: Two, two thoughts on that. The first one, I can only tell you how I did it. So I basically, I finished all of my articles and then wrote the thesis. So it was really like I [01:32:00] was done with all the articles. I knew that this would be my, my dissertation and I wrote the, the introduction and discussion relatively quick, because I kind of knew, okay.
I just gotta, you know, put a little, uh, umbrella over it. The second point though, is I'm so happy. You did read it. is I would re highly recommend taking the time to read the, to write the acknowledgement because.
Benjamin James Kuper-Smith: I mean, you had extensive
Niels Koebis: Yeah, I did. And I, I, I find to me like it's such an important part of doing research is to kind of tip your head to the people that have supported you.
And it's, it's just such a, I, I find it always nice. I actually read the acknowledgements of many papers because I always wanna know, like do, who was also involved in this project. And I, I find research is such a collaborative thing that. I think it's, um, at least to me is something where I feel like it's a, it's a nice token of appreciation to, to mention people.
And, um, it's just [01:33:00] something that, to me was the most fun part to write. I remember buying a good bottle of red wine and one night just sitting down and writing the acknowledgements. And I have a very fun mom memory of just going through all of the people throughout your PhD path, which I I'm sure you would agree is like such an interesting journey, uh, where you kind of, you know, you go literally to places like conferences or meet people in, you get inspired by so many people.
I find interactions among academics still. Um, to me, one of the nicest, uh, uh, types of interactions, because you at least have a very decent chance that you meet people that are genuinely interested in advancing knowledge about something. And so in a way it was really nice to sit down and just thought about all of the people that, uh, yeah.
Have kind of got me to, to where I was . So, um,
Benjamin James Kuper-Smith: It's funny. I just laughing because I feel like I, I I've thought about the acknowledgement sections and I feel like I don't wanna write one.
Niels Koebis: Yeah, my colleague who just graduated, she also had the same. She's like, I, I don't wanna [01:34:00] talk about this, but I that's, that's just my personal view. I really enjoyed it. And I like reading them as well. Like from my friends when they send me their dissertations, I that's the only thing actually that I typically read
Benjamin James Kuper-Smith: Well, like, are you in it?
Niels Koebis: but also like, how are they writing it? And it's, it is maybe a little quirk of mind, but I, I do like acknowledgements and papers and, uh, dissertations.