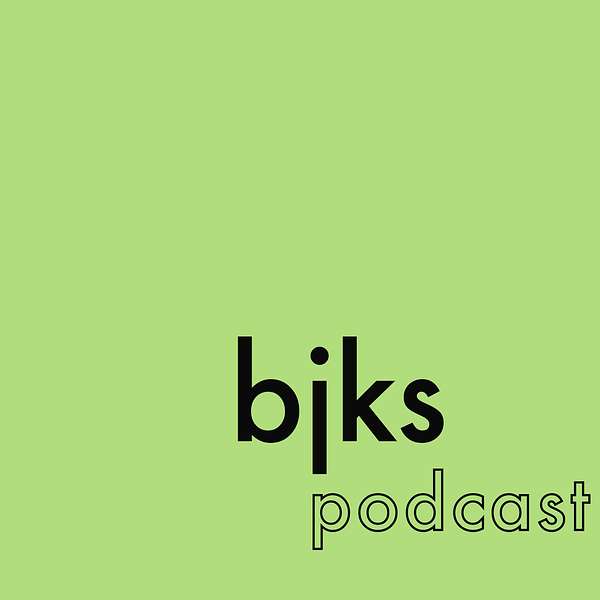
BJKS Podcast
A podcast about neuroscience, psychology, and anything vaguely related. Long-form interviews with people whose work I find interesting.
BJKS Podcast
55. Angelika Stefan: p-hacking, simulations, and Shiny Apps
Angelika Stefan is a PhD student at the University of Amsterdam in the Psychological Methods group (lead by Eric-Jan Wagenmakers). In this conversation, we talk about her preprint 'Big little lies: A Compendium and Simulation of p-Hacking Strategies', which she just uploaded to PsyArXiv. We also discuss how she created the Shiny App that allows users to play around with the simulations and run simulations that didn't make it into the paper.
BJKS Podcast is a podcast about neuroscience, psychology, and anything vaguely related, hosted by Benjamin James Kuper-Smith. In 2022, episodes will appear irregularly, roughly twice per month. You can find the podcast on all podcasting platforms (e.g., Spotify, Apple/Google Podcasts, etc.).
Timestamps
00:05: How did Angelika start working on her paper 'Big little lies'
05:22: P-hacking and human error
07:47: Different p-hacking strategies
29:34: What are good solutions against p-hacking?
40:56: Future directions for this kind of research
45:32: How to make a Shiny Apps
Podcast links
- Website: https://geni.us/bjks-pod
- Twitter: https://geni.us/bjks-pod-twt
Angelika's links
- Google Scholar: https://geni.us/stefan-scholar
- Twitter: https://geni.us/stefan-twt
Ben's links
- Website: https://geni.us/bjks-web
- Google Scholar: https://geni.us/bjks-scholar
- Twitter: https://geni.us/bjks-twt
References and links
- Stefan, A., & Schönbrodt, F. D. (2022, March 16). Big Little Lies: A Compendium and Simulation of p-Hacking Strategies. https://doi.org/10.31234/osf.io/xy2dk
- Wicherts, J. M., Veldkamp, C. L. S., Augusteijn, H. E. M., Bakker, M., van Aert, R. C. M., & van Assen, M. A. L. M. (2016). Degrees of freedom in planning, running, analyzing, and reporting psychological studies: A checklist to avoid p-hacking. Frontiers in Psychology, 7. https://doi.org/10.3389/fpsyg.2016.01832
- Their Shiny App: https://shiny.psy.lmu.de/felix/ShinyPHack/
- Shiny tutorial videos: https://shiny.rstudio.com/tutorial/
- As Predicted preregistration template: https://aspredicted.org/
[This is an automated and uncorrected transcript that will contain many errors]
Benjamin James Kuper-Smith: [00:00:00] Yeah. I mean, I guess we were talking about your paper, big little lies, a compendium and simulation of P hugging strategies. Maybe just like as a, as a, as an easy way in kind of, how did you start working on this project? Yeah, it seems little so to me, that's you, I mean, you did, from what I can tell you did your bachelor's and master's in Munich and now in Amsterdam, but this project is with someone from Munich. So I'm curious whether that like started before your PhD or yeah. How that kind of.
Angelika Stefan: So the project actually started during my PhD, but it has been a working progress since a couple of years already. So, um, Felix Rimbaud as my co-supervisor the co-supervisor of my PhD. So I'm still. Together with him a lot. And so it started out with, we wanted to do something metal, some metal science study, and we wanted to look at maybe something like P hacking and meta-analyses.
And, [00:01:00] uh, so I started looking at literature and I started looking at the P hacking literature and what people had, had written about P hacking. And, um, I started realizing that. Actually a lot of, there's a little clarity about the definition of P hacking because everyone basically in their articles comes up with their own definition of P hacking, uh, which is usually a definition by example.
So P hacking is when people, uh, try to, uh, get a significant result using, um, Such as, uh, and then they name a couple of examples for P hacking, such as, uh, deleting outliers or using multiple dependent variables and reporting on only one of them or using a different imputation methods. For example. The issue was that everyone had a different collection of strategies that they named.
So there wasn't a lot of unity and those P hacking [00:02:00] definitions. And this is basically where this project took. It starts that we started, uh, just doing a big literature review, basically to look at these definitions and collect all these different P hacking strategies. And then. We first just collect them and then also decided to simulate each of these strategies and see what it does with the data and with the distribution of, of P values with, um, the false positive results, et cetera.
So this was how the paper started and we. Already thought that these, all these strategies that people mention actually have a different, a different effect on, for example, the rate of false positive results. And, um, so this is what we also showed with the simulations [00:03:00] and try to make, um, accessible to other people by providing this shiny app.
And then since. We saw a lot of variation in those, uh, P hacking strategies. We also thought, well, let's at least think about how we could use this to also evaluate current methods that are out there for, uh, P hacking detection and basically for dealing with P hacking in the analysis of the literature, or also in preventing PI.
Benjamin James Kuper-Smith: Yeah. I mean, you mentioned quite a few things already that we could talk about later, especially I want to talk about the shiny apps and, uh, all sorts of things that you mentioned, maybe just first, briefly, what exactly is your PhD about? Like, I know I've seen, like you do refuse like stats or Metro science things or have done them in the past.
Is, is this kind of like a central part of it or is it kind of as a side project that grid of hands or is it.
Angelika Stefan: Well, [00:04:00] so I'm doing my PhD with the psychological methods unit at the university of Amsterdam. And my main supervisor is in a gemba Mackers who, um, works a lot on basing statistics. So, um, the main focus of my PhD is actually on bays and statistics specifically on experimental design and on price specification in phasing statistics.
So this is not exactly in line with what I, with what I usually do, but I think it still. Kind of fits in because it is still thinking about how we can improve methods that are used and like very broadly in psychology and how we can obtain more trustworthy, stable, reliable, credible results. So I think it very, very broadly it fits in at least.
What I'm going to try and argue for [00:05:00] my, for my dissertation as well, but, um, no, I think, I think it still fits in and, um,
Benjamin James Kuper-Smith: Yeah. I was just curious whether you, uh, I don't know whether all your, all the other stuff you've been working on recently is also on that, or wait. Yeah. So different than, but maybe do you want to define P hacking then fit? Like how you define it in this paper,
Angelika Stefan: Well,
Benjamin James Kuper-Smith: you have to think about it.
Angelika Stefan: We, we very broadly defined it as, uh, and I, I looked it up, um, actually in the paper. So this is a literal quote, as any measure that a researcher applies to render a previously non-significant P value significant. So that's our broad definition. And then. You get to the, for example, um, and all the strategies.
So, um, we basically, we try and have this one very broad definition and then go into the specifics with the strategies.
Benjamin James Kuper-Smith: Yeah. And, um, I guess there's [00:06:00] this one paragraph that kind of very early on that jumped out to me. And I think that if you don't mind, I'd actually just read briefly because I think it kind of sets the scene quite well. That P hacking is not just something people do intentionally because they've had people.
Um, but that it's a bit more ubiquitous. Uh, so there's from page four. You're right. It should be emphasized that not every research engaging in packing is fully aware of its ramifications. There are many degrees of freedom and statistical analysis, and usually there's more than one right away through the proverbial garden of forking paths.
This arbitrary and is constitutes an ideal breeding ground for biases and motivated reasoning that can provide researchers with a subjectively convincing. To justify the analytic choices in hindsight, therefore P hacking is not necessarily an intentional attempt at gaming the system, but can also be a product of human fallibility.
And it, yeah, it's just sitting there like that. That was an important thing to have in the early on, because I
Angelika Stefan: definitely. I think so, too.
Benjamin James Kuper-Smith: these papers can so often seem like it's like pointing fingers saying like your, your [00:07:00] intentionally committing fraud and these kinds of things rather than, um, Yeah. It's, it's, it's, it's always interesting.
Like now, especially when I go through those 12 strategies that you have in here and, you know, often you get like, yeah, I've seen that often written the papers or something, but some of these strategies and some of them, I think often seen as almost not, not exactly, but for example, like the, um, sorry, now I keep forgetting how you call these strategies.
Um, the. Democratizing variables and various transformation and that kind of stuff to me always seems like stuff that a lot of people just do anyway. And I don't think they necessarily do it out of bad intentions. I don't know. I'd like to discuss some of the strategies and kind of what the effects are.
Maybe. Yeah. Maybe I'll just start by asking, like, what does, is there like any strategies that really surprised you here, the effects of it where you thought like, oh, this is like a small, like, you know, it's a strategy that people that can have an effect, [00:08:00] but like it's probably not going to be, have that much of an effect.
And then suddenly you saw the simulation results and you're like, oh wow. That's, that's much more than I thought this is a much bigger problem than I initially thought it was or the other way around. Yeah. I'm just curious, like, was there, did you have any surprises there when you did this?
Angelika Stefan: So I think. One strategy where the results surprised not only me, but a lot of peoples also based on some responses I got on Twitter was the variable transformation strategies of basically just transform your, your variables, uh, log transform, or take the inverse, uh, take the square roots, um, et cetera.
And that this can have such big effects. I. Did not really expect that post-talk again. Um, I mean, I didn't preregister through my expectations. Um, so post-talk, it seems [00:09:00] sensible. That's using transformations on data that is normally distributed and then computing some regrets. Would create significant results just because you introduce a correlation structure.
So I think you could, even someone, someone told me, uh, in our lab meeting recently that you could even show this mathematically. I hadn't even thought about that to be honest. So it seems like, yeah, this is something you could very. You could have known without the simulations, but that I was personally surprised about that.
The effects are this big, some something else. I think I was also surprised about was outlier exclusion that almost always has. At least a decent effect, even though we were very conservative in the way we implemented outlier exclusion. So we didn't just say [00:10:00] exclude this one data point that makes the regression, uh, the connection between the ver between the variables stronger.
Um, but we just excluded points that would be marked as outliers by popular outlier detection methods.
Benjamin James Kuper-Smith: Yeah. I mean you, yeah. You're actually like you didn't, you know, I guess like the, the, the outline exclusion that can often seem very bad as well. You feel like people are kind of making up reasons for why they excluded this participant or that participant. Uh, but you didn't do that. Right. You just had like generic,
Angelika Stefan: We just had yes. Yes. And it was perfectly like the data was, was drawn from a normal distribution. So, um, we didn't have any skewed data. Yeah. And with some of these outlier detection methods? Yes. Yep. Yeah.
Benjamin James Kuper-Smith: Why. W I'm just curious. I mean, this is kind of specific random question, but why did you, [00:11:00] um, start with, uh, so I found a set of critical you'd have the number of outlier detection methods, and you just start by with three, right? Like that's the minimum you do in the figure?
Angelika Stefan: Oh yeah.
Benjamin James Kuper-Smith: I guess like, what I'm curious about is also like whether any specific outdoor detection methods, what particular.
Prone to doing this or something, just say that, you know, increasing the false positive rate or whether it was kind of more by stacking, like several on top of each other that kind of led to, um, this kind of effect. Did you see what I mean? Like, because I don't know whether I've seen many papers that use many different ones or I dunno, I guess I don't pay that much.
Press attention. No baby.
Angelika Stefan: Well, I guess, I guess if someone did use this as a P hacking strategy, you would only see one outlier detection method reported in the paper and the. The other ones would fall out that wouldn't be reported. So what we did in the paper was take, we had, I think it was 12, [00:12:00] uh, outlier detection strategies, and we just made a random draw out of these.
So we use three random outlier detection methods out of those 12 in the shiny app. You can actually also try it out with single specific methods. Um, And I don't think I actually did this. So I am, uh, I would also be curious about the results. So I would, I would think that the strategies that always find outliers are probably also the ones that, um, can have the highest impact,
Benjamin James Kuper-Smith: Right. You mean the ones that just generically? Yeah.
the most, the most participants.
Angelika Stefan: Yeah.
Benjamin James Kuper-Smith: Yeah. Okay. I didn't, I didn't see, I got paid around a bit with the shiny apps, but not like all of it. And I didn't do that one. And I guess it's cool that you can just choose any combination or individual ones of those 12 and just kind of play around.
Angelika Stefan: And with, with these [00:13:00] simulations, I think this was also one, one of the reasons we wanted to have a shiny up for people to play around with, um, because you always make the. Very subjective decisions on what simulations parameters do you use. So I think we just use three outlier detection methods as a minimum because we thought, well, that's probably what people would do if they do slight P hacking.
Um, but, uh, a lot of this is just based on our. Subjective assumptions of what constitutes P hacking and also on our personal communications with people in our field. And, um, well also our subjective assessments of what people would be w how much time people would be willing to spend on one P hacking methods, even if they intent, like, if they intentionally.
P heck using one of these [00:14:00] P hacking strategies, then how much time would they actually spend on, um, like using multiple outlier detection methods? How many do they even know? Um, something like that, but it's, it is, it is very subjective, which is also one reason for having the shiny app.
Benjamin James Kuper-Smith: Yeah, it's. Negatively and specifically to one person like the maximum, I guess, would be what Brian went dead. Right. Just like trying as long until you basically find something, uh, then you go like, ah, seek good science. I found a significant
Angelika Stefan: It also, I guess it always depends on your motivation. Right. And how much time, how desperate are you? Um,
Benjamin James Kuper-Smith: also a trade-off right? Like at some point it just gets quicker to collect new data, more different data, right? Like at
Angelika Stefan: well, I don't, it also depends on how costly is your data collection procedure. Anyway, I mean, This, this would actually be an interesting topic, I think for. Uh, metal signs [00:15:00] study, like for maybe for a survey, um, researchers who, um, or people who identify as having engaged in P hacking at some point in their career.
And how much effort did they use on a certain method, a certain one strategy, or how many strategies that they, that they use? Uh, what did they actually do? I would be interesting to see that and I, uh, didn't really find any, any results.
Benjamin James Kuper-Smith: Like what's the most time efficient way of P hacking. And it just really, you know, it takes you like five seconds and you, you can definitely double your false positive rate. Um, yeah. I mean, I guess your, your. You're explicit in the paper about this, uh, that this is not a tool for P hacking. Um, and obviously on Twitter, half the people, it seems made that specific joke that now they can,
Angelika Stefan: I think literally everyone I've told about this project, I was like, [00:16:00] I'm doing this project on P hacking. Oh. So
Benjamin James Kuper-Smith: I wonder why.
Angelika Stefan: I wonder why, so what exactly is your motivation and how what's so what's the best strategy.
Benjamin James Kuper-Smith: But what is the best strategy? I mean, to actually take this as a serious crime. I mean, I guess we can, we can frame this question once in terms of this, like a silly joke and once in a, maybe slightly more serious way, which is kind of what is a, uh, so the, the. The city joke approach would be like, if I want to P hack, what's the best way of getting away with something like, what's something that I can use.
That's kind of accepted in the field. Uh, it's maybe a bit difficult to detect from the results. Um, it really increases the false positive rate. We can also phrase this question and the other way and say like when reading a paper. Pay particular attention to something that may be signals. Someone is doing something slightly, um, or, you know, whether intentional or not is doing something that might lead to an increase in the false [00:17:00] positive rate.
Do you see what I mean? Like kind of what's the, yeah, I guess just something, when you see it in the paper that that should really make you go. Hmm. Do I still trust this?
Angelika Stefan: Um,
Benjamin James Kuper-Smith: yeah.
Angelika Stefan: for me, one of the, um, most dangerous P hacking strategies is optional stuffing. Um, because a lot of people who are not, I think a lot of people who are not following all the, um, metal signs, debates, and statistics, and didn't have like a lot of statistics education don't really know that optional stopping.
So stopping once your P value is below a certain threshold. So stopping conditional on your P value is actually.
Benjamin James Kuper-Smith: You collect data and you continue to test kind of your yeah. And then suddenly you get a signal you can go out and you say, okay,
Angelika Stefan: Yeah, exactly. And I think, I think this is something a lot of people don't really realize is such a bad idea, but it's, it is really, really bad idea because if [00:18:00] you do this long enough, you will eventually you're guaranteed to get a significant P value. And it is something that you could, of course, if there was a pre-registration you could probably see it.
But if there is no pre-registration you basically, it's very difficult to infer from a study and everything can sound very, very, yeah. Everything can look very good and reasonable, but then, um, the results are, are still flawed and the error rates are.
Benjamin James Kuper-Smith: I guess this also something that. In many papers. Isn't just like, you know, you don't necessarily always say why you connected this many participants with
Angelika Stefan: Yes,
Benjamin James Kuper-Smith: So if that isn't there, then you have no idea whether it was whether they looked at the data at all before or not, or anything.
Angelika Stefan: exactly. Whereas I think with, for example, dependent variables, even if there is no preregistration, if [00:19:00] someone focuses on, uh, on a, on a dependent variable that sees. Unintuitive or is something that not a lot of people in the literature have done, then you may become suspicious.
Benjamin James Kuper-Smith: Yeah, that makes it, yeah, I guess it's, it's This kind of thing that. Independent of how strong the effect of it is It's just something that's much harder to notice, um, than the other stuff. Yeah. That's, that's the one thing that I guess just surprised me in a way, I guess it shouldn't, um, some, some things you mentioned earlier that like, in hindsight, it's not that surprising in a way, but like that yeah.
The larger, the sample size with option stuffing, the more you increase the likelihood of getting a false positive, just because you have more opportunity for it to happen, basically that if you're in your thirties, Yeah,
Angelika Stefan: This is also something I think I found, um, at least slightly surprising in general, how middle, large sample sizes are protective against P [00:20:00] hacking. And I would have wished that like, it will be better because yeah, large sample size solve so many other problems, but, uh, for P hacking it in, in many cases, it just, um,
Benjamin James Kuper-Smith: Yeah. I mean, that's a, there's also a point I wrote down that I wanted to address. Like, I really thought that it would, yeah, just having largest, I mean, you have large sample size, right? Like we're not talking about, I guess online studies, 300 people isn't that much, but if you run a lab based study, Testing 300 people could take quite a lot of time, but so that's, I mean, like that's not for, for at least an individual study, that's not even the kind of sample size I would even really consider.
Um, it's just, you know, I guess the kind of studies we do are like, I don't know, 50 people or something like that. And then, okay. We have a few studies, so then overall it gets a bit more, but, um,
Yeah.
I was surprised that even having such large samples of, um, [00:21:00] Basically, you only really get, if you do an online study or if you, I know Yeah.
Some sort of survey or something
Angelika Stefan: Hmm.
Benjamin James Kuper-Smith: sent out to lots and lots of people you wouldn't even reach. And even then it just didn't really make much of a difference in most of the cases. But I guess you already kind of alluded to it. It's not, you know, P hacking is one aspect of good science and, uh, or I guess P hacking is one sign of bad signs, but I guess you said.
Sample sizes are good for lots of other things. It's just for packing that had to do that much after like one thing that, that also really, I think I mentioned earlier that really surprised me was just the kind of, you have every transformation and just get it. I just don't know how to say democratizing, just making variables, discrete, how that.
Uh, you know, making reference discreet didn't have a huge, like upper limit in that sense. Right. It kind of always seemed to be around like 12% false positive rates or something like that.
Angelika Stefan: I think, I think this also depends on how [00:22:00] we implemented it. What we did was to use a median split then. Cut the middle strategy where we cut out the middle part and left over only a comparison between the, the most extreme groups. And I know that I think we had, of course the, the continuous variable design.
So we didn't have a lot of variations in there, like cutting it into four or five or six, um, discreet. Groups. So this, this might be one of the reasons for why there's only so little variability, but I think this, this one was a really, really difficult one to implement in a simulation because once you start, where do you stop?
We decided to keep it minimal in that case and see what, what comes up.
Benjamin James Kuper-Smith: Yeah, but I think it also makes sense, right. Because it seems to me that the median split. Maybe not, I don't know whether it's still that common, But I've read a lot of papers that [00:23:00] use that. And it's often in cases where you go, why did they use emergence? But they, they could have liked that there was no reason to do this.
They could have just analyzed the continuous data and.
Angelika Stefan: But again, it doesn't have to be malicious intent. It can, it can be just an auto. Um, you read it so often, then it's just an automated reaction to copy what you read in other papers.
Benjamin James Kuper-Smith: Yeah, but I guess it's, it is. I think I've always been fairly critical of that because to me it always seemed like just, I mean, sometimes sure there are reasons for doing it, but often not. And I think that's one of those, one of those strategies to me, that where I thought like, it really increases the false positive rate and it's something you see it on quite a lot, so that's not good. Yeah. Um, by the way, are there any, I mean, so you focused on these 12 tragedies. 12 sounds like it's a suspiciously. [00:24:00] Nice number, a good dozen. Uh, did you have like a few more, that just didn't seem that important that you didn't include because the paper was already long enough or something, or to make it kind of
Angelika Stefan: We had a few, we had a few things where we were on the fence, whether it is P hacking or not. So I think that the last one that didn't make the cuts was selecting effects from an ova, for example, and then, uh, basically focusing your attention away from one main effect to the other main effect or two or an interaction effect.
And, um, Basically at the last moment, we decided not to include it in this, in this list because we felt that it was, um, more harking than P hacking as a hypothesis hypothesizing after the results are known. And you would still see, probably see the whole Inova. So it is very, in a way, very [00:25:00] transparent. Yes.
We felt that in a way it doesn't really fit in there, but it was really very close to making the cut.
Benjamin James Kuper-Smith: Yeah. Yeah. I can see why you looked at it And why you didn't include it because then you kind of have to change the narrative of what you're doing. Right. Because you kind of have to justify why you suddenly don't care about that.
Angelika Stefan: Yeah. Yeah. And this is, this is exactly where we set the boundary between P hacking and harking. So with at least in our opinion, with the P hacking strategies that we included on the paper, P people wouldn't have. Needs to change. The storyline ni would need to change their hypotheses. Whereas if you actually look at a different effect, you probably need to rewrite your whole introduction.
Benjamin James Kuper-Smith: Yeah, exactly. And I guess you're not actually changing the p-value right. You're just, you're just focusing on.
Angelika Stefan: Yeah. Yeah. Switching, switching phones.
Benjamin James Kuper-Smith: Okay. Any other ones or I'm curious now, it's just also just interesting to see like the, [00:26:00] in a way, like, I guess if you, you know, I'm, I'm not a Mehta scientist, but I'm just interested in this from, from the perspective trying to do well and not doing, not doing bad science.
Um, but it, it's kind of interesting. So like in a way, this. So this is slightly like definition thing, right? Like, is it Hocking is a P hacking, like, from my perspective, it's like, well, it doesn't matter too much, but that is kind of interesting to see kind of what the boundaries are of these things and kind of.
Angelika Stefan: Yeah, I think, I think it's, for us, it was also a learning process because people mentioned P hacking, harking and publication bias. Use it usually in one sentence, but still as different questionable research strategies. And for us, it really made us think about what are the differences, whereas an overlap where can, um, P hacking overlap with harking, uh, P hacking overlap with publication bias.
Yeah. So this was also one, one little contribution we try to make with this paper paper to just separate these [00:27:00] concepts a little bit better. Um, yeah. As to other strategies that didn't make it. We try to be as inclusive as possible. Um, so it, it's not like we have this big dumpster full of P a P hacking strategies that didn't make the cut.
That's not, not at all the case. We really try to keep everything in there that people at least repeatedly mentioned as a P hacking strategy. One thing we. Didn't talk about in this paper was in several papers. It was mentioned that people would do, self-selecting sort of, uh, an individual publication bias.
For example, selecting studies. Um, I say I did three studies and two of them were significant and one, uh, Uh, non-significant results. And then I, I have write it, I write up a paper and I write up only the significant results. And there are people who also call this P [00:28:00] hacking in a way it's links in with the reporting strategies that we mentioned in the paper.
So deciding what P value to report, if you calculate it, lots of P values, but then again, um, The analyses we did were using a single data set. So we didn't look at what if people collected multiple data sets and then, uh, reports P P values out of multiple data sets.
Benjamin James Kuper-Smith: I'm wondering like how much different that is from subgroup analyses. Cause in some sense, couldn't you say you've apparently if I remember correctly, your subgroup analysis, basically you say like we have this large sample of participants and now we split it up into different groups. Isn't that kind of.
I know, but you're still reporting all of them. Yeah. You're not, you're not like pretending one of the SRS is different yet.
Angelika Stefan: No, but it's, it's definitely similar.
Benjamin James Kuper-Smith: Yeah. So, so people shouldn't do that because I have a study where one isn't significant of [00:29:00] anything. No, no, no.
Angelika Stefan: I'll always be transparent, always report
Benjamin James Kuper-Smith: inconvenient.
Angelika Stefan: No. Um, no, just, I would always recommend transparency.
Benjamin James Kuper-Smith: Yeah, the problem is we, we made the error of pre-registering it. So now we have to reform. Um, yeah, no, but I actually like it because it forces you also to think more about what you're actually doing, rather than just saying like, ah, whatever didn't work. Yeah. I mean, maybe Aisha would just briefly mentioned that.
Talk about the solutions then. I mean, you already mentioned that large sample sizes aren't as much of a solution as we might might've expected, or at least hoped for maybe shall we start with one of my recent episodes with, was with Chris chambers about registered reports. So how, how do they kind of fit into this whole thing?
Are they a solution for P hacking specifically or.
Angelika Stefan: I'm a big fan of registered reports. I think they are great if [00:30:00] it, if it was for me, almost every paper could be a registered report. At least if it reports confirmatory results, I think with P hacking, it sort of has the same caveat as previous preregistration. And pre registrations protect against P hacking as much as they are able to limit your, your analytical flexibility.
So I think what our analysis show is that you can basically use any P hacking strategy. If you, if you just use it aggressively enough, you will still. Get a very high probability of false positive results. And I think this is an issue for pre registrations because if we assume malicious intent and I just didn't, preregister [00:31:00] something that allows me the flexibility to, uh, use one of these strategies.
Then I can still. Engage in P hacking, even though I have a fairly compelling preregistration. And I think this is an important message or for also for, for me, it was an important learning because it really means that when it comes to P hacking, I won't necessarily assume that everything that is pre-registered can be.
Not everything that is not, not saying, I mean, probably most people who do preregistration and registered reports and stuff. I don't assume that they, they P heck that's usually not the same crowd.
Benjamin James Kuper-Smith: It's not your first assumption. Yeah.
Angelika Stefan: but it doesn't it's, it's not as good as at protecting against P hacking as it maybe should.
Benjamin James Kuper-Smith: But it seems to me like a retro report would [00:32:00] then if the peer reviewers do their jobs actually be like a good, you know, they can kind of safeguard that people actually specify. For example, with, with respect to these 12 strategies. What they're going to do to, to then actually limit the flexibility that you do have.
So it seems to me, I mean, I, I completely agree with you. And I feel like over the last few years, I've also met some people who I think. As much in favor of the open science movement and kind of did some things because they kind of felt they had to go along with it. And then, I mean, I didn't, I don't know, maybe they wrote great preregistration, but I feel like if you're kind of just going along with it, because if you're pressured to do it, then you might not be as specific as maybe you should.
And you kind of just say like, oh, we're going to do, you know, just like a very vague period of station, but it seems to me like a registered report could then actually say that the potential. That you just addressed with P hacking and retro reports, you should be able to Cain to largely avoid. Let's say with, with [00:33:00] having peer reviewers, actually look at it and say, Hey, what's the low exclusion criteria, please.
Angelika Stefan: yes, I think, I think that definitely makes sense, which is also why I think registered report is. Even better than just a preregistration. Um, also because you, I mean, it's, it's so valuable to get feedback from reviewers and to still be able to change your, your study, uh, based on their review or feedback.
And I think, I think that's also also just great, but also in terms of the pre-registration, I mean, it is also just so easy to forget something and again, P hacking does doesn't have to, doesn't have to happen with malicious intent. It can also just be hindsight bias. And I think it's great. If, if reviewers look at these, all these aspects of flexibility, analytical flexibility, and try to improve the pre-registration tour together with the.
Benjamin James Kuper-Smith: Yeah. In a, in a way it seems to me almost like you could use the [00:34:00] 12 strategies you have here. Not exactly as a checklist, but as something where you got, do I state specifically what all of the DVS I measured or do I.
You know, in the pre-registration of registry reports, say, whether I'm going to do it in transformations, if X or Y happens or whatever, it's like, in a way you can just, you can almost use this to, to protect yourself from hindsight bias and these kinds of things. Um, because Yeah, I agree. Like it's so easy to just forget to specify some.
But if you have like a list in front of you that says like at 12 things you shouldn't forget then, um, I mean, I guess the OSF already does that to some extent, right? They have like, uh,
Angelika Stefan: they're uh, preregistration templates. Um,
Benjamin James Kuper-Smith: all of these in there, or I didn't, I didn't check.
Angelika Stefan: So usually registration templates are a little bit broader. So they would, for example, also ask you to specify the sample size [00:35:00] and specify what your overall design as what the stimulii are that you want to present, et cetera. So depending on. What version you use and, uh, how extensive those pre-reads pre registrations are.
They, they even have more aspects that we didn't explicitly mention. We didn't design. I agree that it is like, it probably gives you a. Idea of what to fix in a, in a preregistration, but it, like, we didn't design this as a, uh, pre-registration template. So I think, um, it's always, it's always better to use one of those pre-registration templates specifically, if you were working in a field where there's already a field specific pre-registration template, because I think they, uh, they thought of so many more things than we did.
Actually, we're just thinking about what could be, what could people do when they P hack? [00:36:00] Um, so, uh, I think I would, I wouldn't use one of those.
Benjamin James Kuper-Smith: Yeah. I mean, I agree. Definitely. It's just, um, I guess more like, in addition to that, Yeah, I guess, as you said, like those.
I mean, there are some templates that are super intentionally super vague or like very, very minimal. Right? The as predictive, whatever it is, where they just said, what's your sample size? What's your DV or something like that.
Angelika Stefan: But still, I think, I think that's, that is still a much better than no pro pre-registration because at least, you know, um, especially with, I think with as predicted your preregistration becomes public after a certain time. So you would know that there was a study that at least was planning on looking at this research question, which is already super valuable information for meta analysis, for example, because then you could.
Email the authors and say, Hey, where are you? Results.
Benjamin James Kuper-Smith: yeah, exactly. Um, I think [00:37:00] as an additional thing. I mean,
Angelika Stefan: Yeah.
Benjamin James Kuper-Smith: I wonder, Yeah.
maybe moving, I'll try it just next time. Have your paper, uh, next, next to the computer. And
Angelika Stefan: There's actually a really nice paper from, uh and, uh, from 2016, it's called degrees of freedom and planning, running, analyzing, and reporting psychological studies checklist to avoid P hacking. Um,
Benjamin James Kuper-Smith: sounds like a checklist of, yeah.
Angelika Stefan: Has like, it just lists a lot of flexibility, points of flexibility. So in comparison to our paper, it doesn't look very closely at each strategy. It doesn't do simulations, but it's, it has this huge table somewhere in the middle that lists so many degrees of flexibility on. This is also a great space as for, for pre-registration because you can basically just go, go long and say, okay, okay, fix this, fix this, fix this, and basically set your [00:38:00] check marks.
Benjamin James Kuper-Smith: Okay, that sounds pretty good at how a look at that then. And also, I guess, for anyone who's listening, who hasn't listened to this podcast before, there's I always like put references to stuff we mentioned in that description. So. Sort of that you can just go to the description of the episode and I'll put it there.
Um, I'm wondering whether we should talk a little bit about the combining of packing strategies to some extent. It just seems to me like the more you do, the more likely to get a false positive in a way it's like a very simple story.
Angelika Stefan: Yeah, it is just the only thing you can really add is that the additional benefits is it's not, not, not exactly additional. So, um, if you did the same two strategies with the same in the same intensity, then if you look at the effects of this strategy as, uh, on its own, then the combined effects are smaller than the sum of the single strategy.
Benjamin James Kuper-Smith: So it's diminishing returns.
Angelika Stefan: [00:39:00] yeah, the diminishing returns.
Benjamin James Kuper-Smith: the more ups than what, the harder you have to work at it, uh, before. I also like the term ambitious P hacking makes us sound very good. It's like, oh, look at these people with ambition. I tried so hard, I guess. Sorry. We would brief, you're talking about solutions. Um, You were probably fairly happy that using base FAC I mean, given the other part of your research, I guess you were fairly happy that base factor seem to, I mean, if I remember correctly, it seems like, uh, effect sizes and based factors were actually some things that, especially in combination with large sample size actually did help.
Right. Were you nervous when you ran that analysis or.
Angelika Stefan: A little bit. Um, but it's also, it's clear that if you, if you generate data under the null hypothesis, so with the knowledge. Then eventually the base factor should just show evidence for the null. But of course, the question is when, when does it do that? And to be fair, [00:40:00] the analysis we did in the paper, I'll also not super extensive.
Um, it was just one way of showing. Okay. So based factor can help, but only if it's in combination with a large sample size, So we didn't do, full-scale a really big analysis because that, that would have that, that would have been another paper. Um, but I think, I think when it comes to, what can we do against P hacking?
I think looking more at effect sizes and base factors already helps also because it takes away the focus from those wretched P P values.
Benjamin James Kuper-Smith: Yeah.
And I guess like, what you did is also more like pointers, right. And like certain different directions of things that people have tried and saying, like, does it seem like this helps or not rather than like, fully answering that question? Yeah.
Maybe I kind of last thing about the study itself, kind of I'm curious the, you know, in the end you say [00:41:00] the last sentence is our compendium and simulation of picking strategies can be viewed as the first step in this direction.
Um, I'm just curious, are you, are you making, are you yourself doing more of those steps or is that most of the thing that you're leaving to Felix or other people or.
Angelika Stefan: I will first try and finish my, uh, my, my PhD thesis.
Benjamin James Kuper-Smith: Okay.
Angelika Stefan: And then we'll talk again about that. Um, but I think, I think there are lots of interesting research questions coming out of this. And, um, I mean, I already mentioned one, uh, What do people do if they P hack and, um, basically just doing, doing a survey, seeing what, what there's a lot, we still need to understand about what actually happens out in the wild basically.
And we almost make assumptions in simulation studies. And I think it is very important to check how valid these assumptions are and confront them with empirical data. So that's something that. Would it be very [00:42:00] interested in looking at, I also think there's a lot you can still probably still do on, uh, with, with regard to P hacking detection methods.
So in, in the paper we said it is, it is very difficult and, uh, because you always have a mixture of effects, you have a mixture of P hacking methods, et cetera. But I think that it will be interesting whether there are some. Possibility to separate P hacking from publication bias in the literature. So I think, I think there is a lot you could do in that way as well.
Benjamin James Kuper-Smith: What are some good strategies of detecting P hacking in a single paper? I mean, I think. But that most of these strategies are more for like a field. And you can say like, look, all these, you know, collectively this field seems to be hacking away, but how can you detect via hacking in a single vapor?
Angelika Stefan: Well short answer. I think you can't, unless, I mean, you can have a, as [00:43:00] you said, for example, it is very suspicious. If someone uses three different outlier detection strategies through throughout the paper, at least as a reviewer. I would ask why, um, is there any specific reason apart from that your P and P value is significant now?
Um, I don't think, but I, I think these are mostly just gut feelings or suspicions on, uh, I don't think there's any analytical methods that can tell you based on the single paper. This is probably P hacked or not. I think I saw something at some point that where people try to train a deep learning algorithm on, uh, papers that were P hacked or not.
I don't know how they knew in the first place, like what, what their training sample was. And then you could probably. Use that deep learning [00:44:00] algorithm on the paper and get some probability of it being P hacked. But then I, I'm not sure how, how good that works. So I wouldn't really trust this, uh, to be honest.
Um, but it's an interesting thought that people might reveal themselves by using certain language. If they do P hacking.
Benjamin James Kuper-Smith: Yeah. I wouldn't trust that too much.
Angelika Stefan: No me neither, but I, I think I saw it somewhere. I don't know the reference though. So, um, may have been a dream.
Benjamin James Kuper-Smith: But maybe, I don't know. It's a very specific dream.
you have. Yeah. You know, you're deep into your PhD in Metro science. If you
Angelika Stefan: This, this happens
Benjamin James Kuper-Smith: what you dream of papers that don't exist. yeah. I mean, I guess to some extent, the whole problem with people doing bad science. I guess the difficult thing, it is really, really difficult to, to detect and to even prevent, you know, as I said, like [00:45:00] often just forget stuff and then analyze things with a bit of, I mean, like my, the first study I'm I did in my PhD, we're kind of finishing it now.
And, uh, that's like basically for two years it's just been lying around. And so, you know, after two years suddenly you're like, I kind of thought I wrote everything down and luckily we preregistered because otherwise God knows while I was there. So he's three years ago. Um, can we talk briefly about shiny apps
Angelika Stefan: Yeah.
Benjamin James Kuper-Smith: I've seen, yeah.
I mean, I've seen them around occasionally I've used them, but I've, I've never actually made one myself. I think I might've looked into it briefly, but I'm just kind of curious, like, how do you, how, how does one make a shiny app? Kind of what's the process? Is it easy? Is it a year of your life? You wish you.
had back?
Well, what's the, Yeah.
What was it?
Angelika Stefan: So I think it depends on your, our literacy. Um, if you already use our, for running analyses and, um, doing other things, maybe [00:46:00] writing our markdown files, et cetera, then I think it's not such a big step. I made my first shiny app during my masters, actually from a master thesis. And, um, I think it took me. A day to figure out the basics.
Um, there are very good tutorial videos from the shiny R studio people that I found extremely helpful back then. And basically I just created. The structure of this shiny up and everything based on those tutorial videos. And then I tried to fill it, fill it with my own content. So with every shiny, shiny app, every shiny app basically has two parts.
One is, um, the user interface. And then the second part is the server side. And, um, so with the user interface part, You basically tell R or shiny where all the boxes should be. Um, what the panels should look like. Uh, [00:47:00] where do you want to have a figure? Um, do you want to have a slider or, um, multiple choice menu, for example, then with the service side, you, the.
User interface with content. So if you say I told shiny, I want to have a plot in my main menu, and then I fill it with information with data. Okay.
Benjamin James Kuper-Smith: Because that sounds pretty easy from the way you described it. I mean, I guess, uh, where you're already familiar then with our, when you started.
Angelika Stefan: I did have some experience with are generally making shiny apps is easier with any, you would think, um, the only hurdles in my experience are that you have sometimes. Deal with reactive values that are inherited from, from JavaScript. So basically you have something like if someone pushes this button, then this reacts of value [00:48:00] changes to one.
Benjamin James Kuper-Smith: value.
Angelika Stefan: So this is, um, it's basically a value that is defined by what a user does. For example, button presses.
Benjamin James Kuper-Smith: Okay.
Angelika Stefan: So sometimes it's, it's sometimes a bit difficult to debug these reactive things, uh, because you, you can never really test all configurations. And sometimes the error messages are not. Easy to understand.
Um, then, uh, I usually just go on Google and stack overflow and look, look up what other people say. And, um, so I think, I think it's easier than most people think, and I think it's a great way to display information and, uh, in an interactive.
Benjamin James Kuper-Smith: Yeah. I mean, it always looks like it's, I don't know. It looks like it would be a lot of [00:49:00] work, um, when you see them, because it always seems. I mean, I know that there are lots of tools for boat on websites and all these kinds of things. And in principle, it could be relatively straightforward, but off this thing is, you know, isn't straightforward in practice, but that sounds good.
Angelika Stefan: Yeah. So, I mean, I can, uh, I can tell you, I actually looked at my app code, so I have two code files, one for the server and for one for the user interface. And my user interface code parts is 400, about 450 lines of code. And the server side is 380 lines of code. So it's
Benjamin James Kuper-Smith: The question is how efficient is your code
Angelika Stefan: that very much depends.
Benjamin James Kuper-Smith: people 450 lines can mean almost nothing. But
Angelika Stefan: Yeah. Um, yeah, maybe this, this revealed a lot about how efficient my code is.
Benjamin James Kuper-Smith: but it doesn't sound like a lot, I guess. It's
Angelika Stefan: Um, yeah, so this is actually what I wanted to say. It sounds like, because I think, I think the app looks fairly [00:50:00] complex. Um, But it also, you makes use of all these simulations functions that we already defined for the R package.
Uh, so basically what we do is just to say, um, to create a user interface for those simulation functions, I feel like it, it looks more complicated than, well, roughly 900 lines of code.
Benjamin James Kuper-Smith: Yeah, which is, you know, again, I mean, I also haven't done much in us, so I don't know exactly how that's done a little bit now. Um, but. Okay. I guess if you, if you say it's easier than one would think, then I'll just take that. That sounds good.
Angelika Stefan: Yeah, would, I would really recommend, uh, looking at those R studio tutorial videos, um, because they're, uh, they were awesome. Maybe we can also link them in the.
Benjamin James Kuper-Smith: And I'll find someone put them in the description. Uh, do you have to, don't have to pay for this? I think I once looked into it and it seemed like you had to pay for it. It was for like hosting the thing or something like that, or is it like through the university or.
Angelika Stefan: You [00:51:00] only need to pay for it if you want to host more than, I dunno how many apps on the shiny app server, but you can, so we have, we have the advantage that Felix has. Um, if it, for my co-author. Has basically his own server, um, that is connected to the LMU system. So the, uh, university in ma in Munich, though, he as hosting the app on his own server, but you can also feel free host apps, um, up to a certain number of apps, um, on the, like, just with a user account on the shiny app server.
And you can always. Create as many apps as you want, if you just use them on your own computer. Um,
Benjamin James Kuper-Smith: Yeah. Yeah. Sorry. I just looked at the, at the Euro. It is shiny dot P S Y. And I moved out to east slash
Angelika Stefan: exactly.
Benjamin James Kuper-Smith: Yeah, Okay. I mean, I don't know whether that will ever do anything with. [00:52:00] It seems to me like these kinds of apps are particularly useful. If you have a kind of large data set with lots of options, where in a paper, just, as you said earlier, you kind of have to make, if you put it in a paper, you have to make decisions and reduce the space of things you've mentioned.
Talk about. So I think for those things, it makes a huge amount of sense. Not sure whether I actually have anything like that, but yes, it was nice to click around. Kind of see what people did.
Angelika Stefan: Yeah, I think it's also nice for teaching. Um, if you want to explain some concepts in statistics or.
Benjamin James Kuper-Smith: Okay.
Angelika Stefan: Like P hacking, then it's nice also for students to have an app to play around with. And, um, I think it, I get the impression that students really like it and hopefully fosters, um,
Benjamin James Kuper-Smith: Yeah, especially, I mean, I haven't, So it's the, you said you, you Russ in our package. So what did you use? What [00:53:00] package.
Angelika Stefan: So we, with this, with this paper, we both created a shiny app and an R package. So basically you can go, uh, go to GitHub and download the R package, and then you can use the R package for simulating P hacking and redo some of our, uh, our simulations.
Benjamin James Kuper-Smith: And that's largely equivalent almost to the shiny apps.
Angelika Stefan: It's largely equivalent. Yeah. So the R package has a few more options, but, um,
Benjamin James Kuper-Smith: I guess what I want to say. It's just like for teaching, it's pretty cool then that people, you know, you can have like a quick click around and then if you're really interested, people can like, look a bit more into the code and how it actually works. And also learn like these kind of simulations with it or something like that.
Angelika Stefan: maybe at this point I should also stress that the R package is only for simulations. It doesn't do P hacking for you.
Benjamin James Kuper-Smith: you. have to do it yourself. Although I, I there's [00:54:00] this big header that keeps looking at me, which is obvious watering thou shall not be, heck see if you're very clear on that. Um, don't do it.